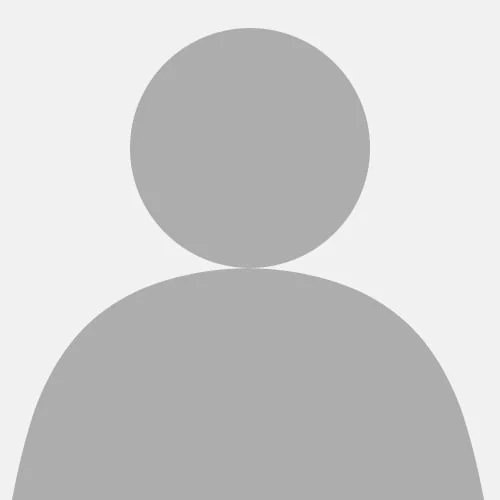
Maggie Mi
In the recent decade, scholars have been exploring the use of speech to aid diagnosis of illnesses (Brabenec, Mekyska, Galaz, & Rektorova, 2017; Brown et al., 2020, p.1). As coronavirus is a respiratory disease with symptoms that prompt pulmonic paralinguistic sounds, such as coughing, wheezing, and voicing, it is predicted that these features will have acoustic significances and patterns that can be used to train two Machine Learning models, namely, a Convolutional Neural Network (CNN) and a Multilayer Perceptron (MLP) for COVID-19 diagnostics. Importantly, the majority of previous works overlooks the (socio-)phonetic/linguistic foundations for analysis (Banerjee et al., 2019; Brown et al., 2020). Therefore, what makes this research truly original, is the sociophonetic experiments that will be tested on the system. These include, exploring which vowel carries the most ‘COVID-19 information’, the allophonic composition contribution to classification and whether anatomical differences due to gender, i.e., males having longer vocal tract (VT) length than females, would lead to significant differences in results. Rather than contributing the shortcomings of the system to algorithmic solution designs, it is just as important to consider VT anatomy and articulatory phonetics, to better understand what the data can reveal about speech production in COVID-19 patients.
Banerjee, D., Islam, K., Xue, K., Mei, G., Xiao, L., Zhang, G., . . . Li, J. (2019). A deep transfer learning approach for improved post-traumatic stress disorder diagnosis. Knowledge and Information Systems, 60(3), 1693-1724.
Brabenec, L., Mekyska, J., Galaz, Z., & Rektorova, I. (2017). Speech disorders in Parkinson’s disease: early diagnostics and effects of medication and brain stimulation. Journal of neural transmission, 124(3), 303-334.
Brown, C., Chauhan, J., Grammenos, A., Han, J., Hasthanasombat, A., Spathis, D., . . . Mascolo, C. (2020). Exploring automatic diagnosis of COVID-19 from crowdsourced respiratory sound data. arXiv preprint arXiv:2006.05919.
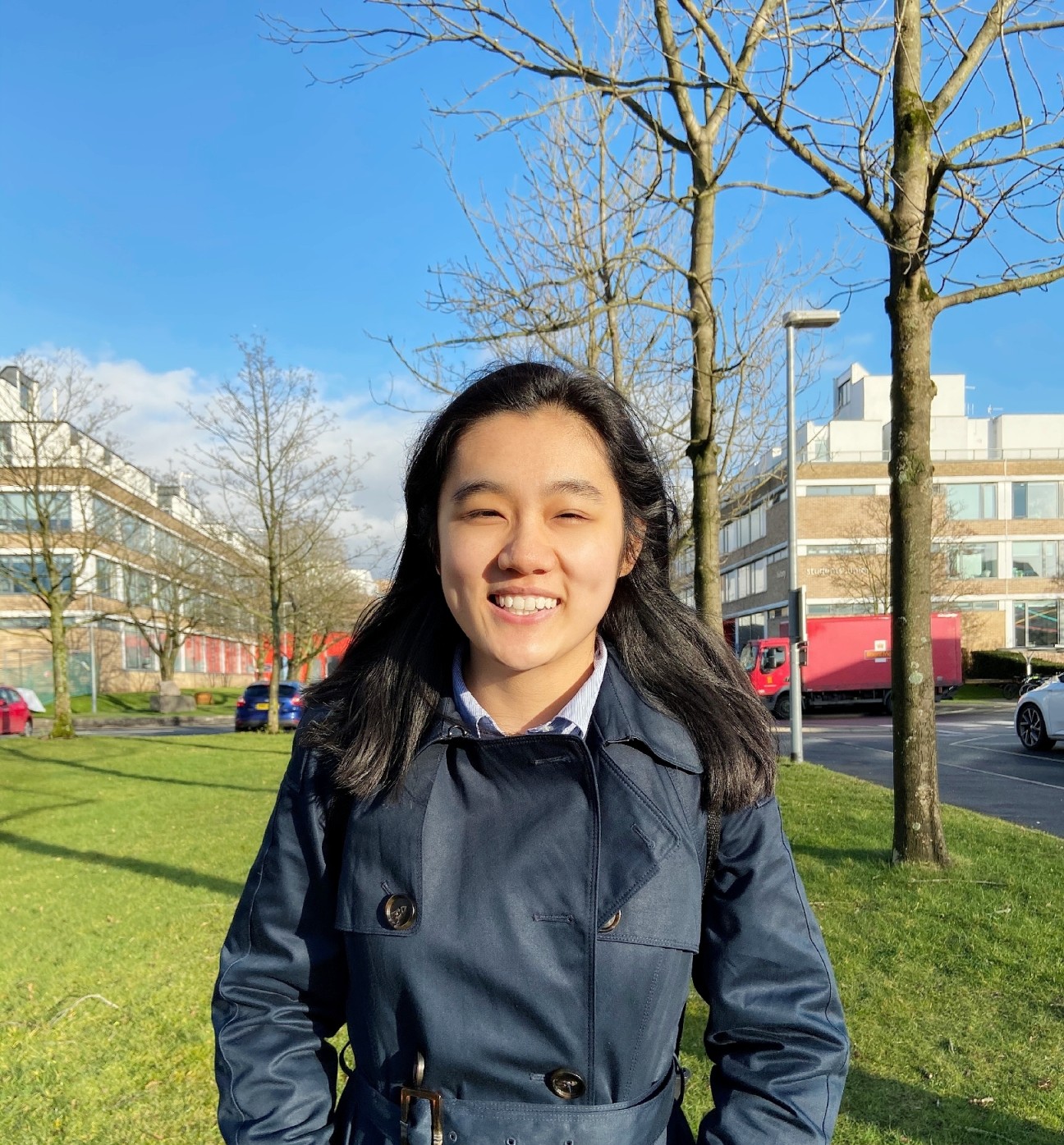
Maggie Mi
Diagnosing COVID-19 Using Speech
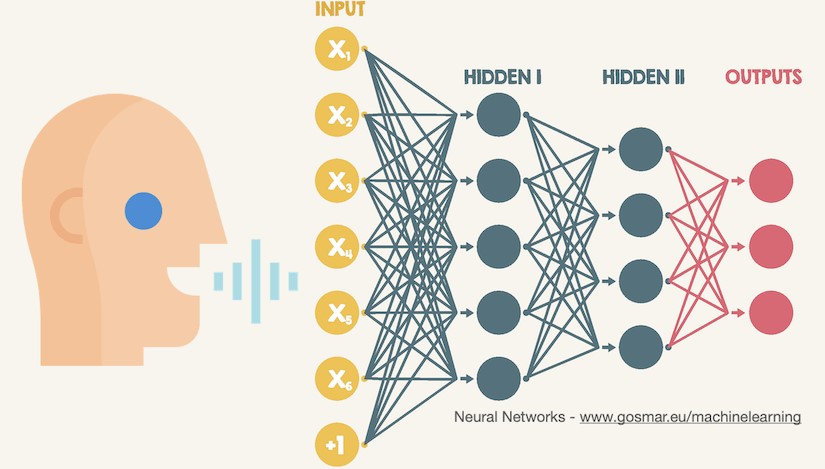
Research Rationale
Symptoms of COVID-19 include fever, cough, shortness of breath fatigue, headaches, and increased sputum production (Guan et al., 2020). These symptoms are related in speech and affect speech production. For instance, speech qualities, such as breathiness is directly influence by respiration. Moreover, numerous studies have found that respiration affects speech production and that various kinds of lung diseases, such as pneumonia, directly affects paralinguistic patterns (Lee, Loudon, Jacobson, & Stuebing, 1993; Ohala, 1990). Therefore, it is plausible that there exists a correlation between speech characteristics and physiological abnormalities that are caused by COVID-19 infection.
New drugs require extensive clinical testing before it is approved and deemed safe to be used on human patients. Therefore, to account for the urgency of the problem, the most suitable strategies involve controlling the spread of the virus are through means of “screening” and “testing”, and this response is mirrored globally (Hassan, Su, Sun, & Yih, 2020; Ibrahim, 2020; Josefsson, 2021; Van Nhu, Tuyet-Hanh, Van, Linh, & Tien, 2020).
Therefore, I aim to build a diagnostic tool for COVID-19 detection based on speech data and carry out socio-phonetic experiments on these models. Such a system is not only portable and require less time to output results, but it will also reveal speech features that are affected by COVID-19 that yet remain unexplored. Furthermore, the existence of such a system in hand with laboratory testing would be a life-saving resource for economically developing areas of the world that cannot afford modern medical resources, such as Reverse Transcription Polymerase Chain Reaction (RT-PCR) test kits and vaccines.
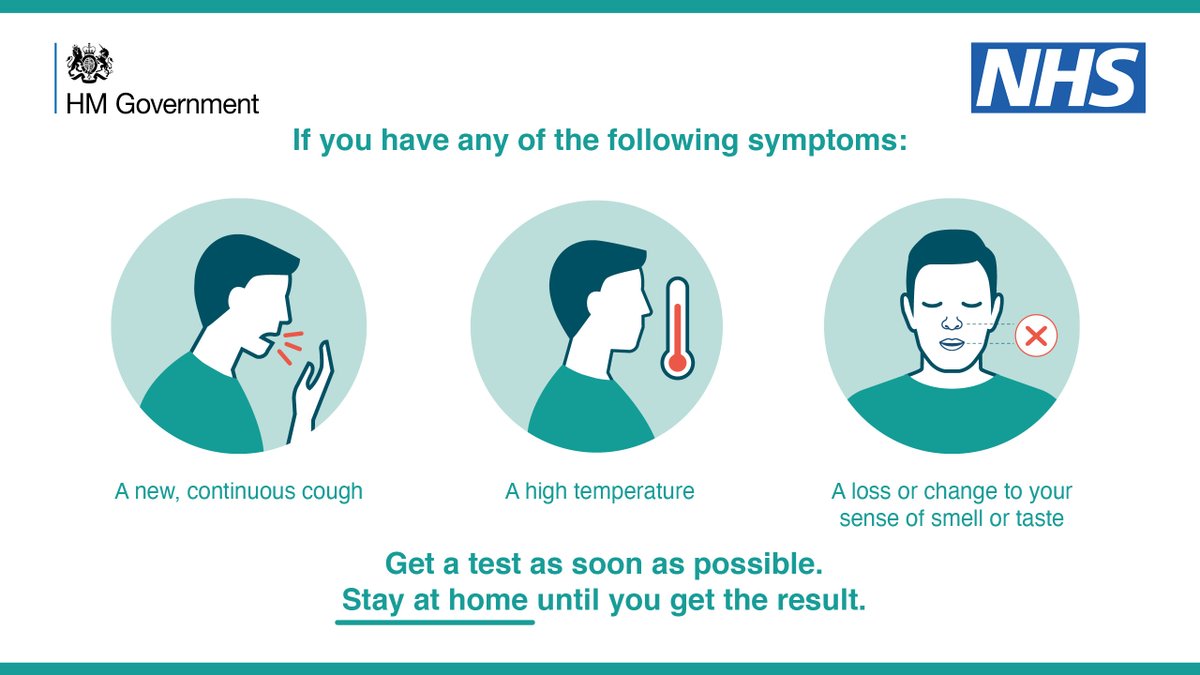
Twitter Post from the British Prime Minister Boris Johnson (UK Prime Minister, 2020)
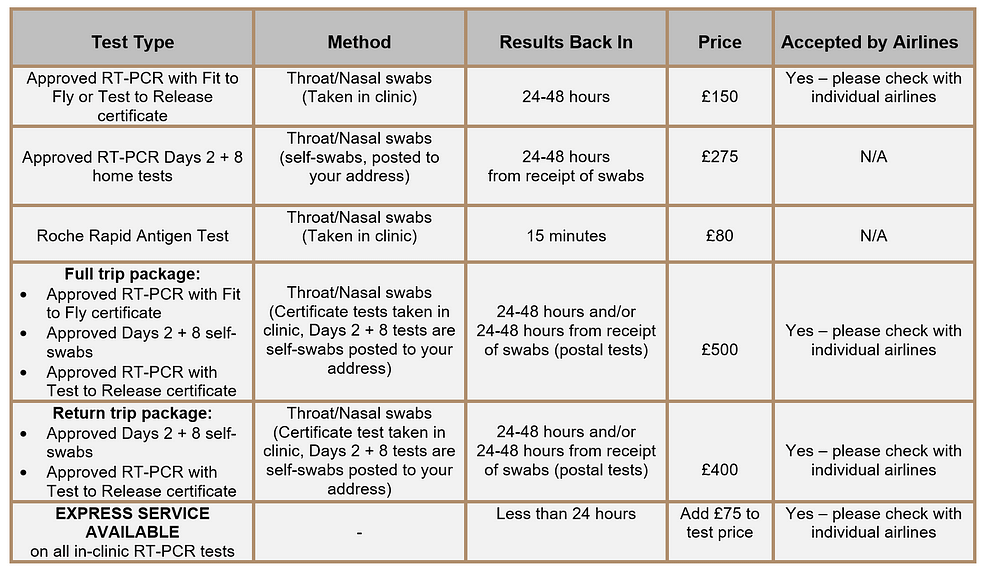
Prices of Test Kits taken from The New Foscote Hospital (2019)
Data and Methodology
The data used in this project is collected from Project Coswara.
Project Coswara (Sharma et al., 2020) is founded by the Indian Institute Bangalore. It attempts to build a tool for COVID-19 detection using speech samples provided by individuals, that range from breathing sounds, cough sounds to speech. The raw dataset of this project is made publicly available online and is updated frequently. The repository that is used for this project consists of crowdsourced data, spanning from 13/04/2020 - 16/01/2022. Moreover, the data is advantageous in terms of its coding and labelling. The health statuses of individuals were coded as:
- healthy
- respiratory illness not identified
- fully recovered
- positive mild
- positive asymptomatic
- no respiratory illness exposed
- positive moderate
Additionally, sufficient metadata of the data contributors were also collected, for instance, sex, age, and location of origin.
Therefore, this dataset was chosen because of its comprehensiveness; there are in total 2375 speakers who each contributed 9 different categories:
- Breathing sounds
- Fast paced
- Slow paced
- Cough sounds
- Shallow
- Deep
- Counting (in English) from one to twenty
- Fast paced
- Slow paced
- Vowels
- /i/ as in "tea"
- /eɪ/ as in "raid"
- /uː/ as in "food"
The data was then split into 80% training data 20% test data.
Methodology of Present Study
The basic idea behind this procedure is converting speech recordings to mathematical representations that machines can manipulate. The representations (i.e., vectors) are then used to train up Machine Learning classifiers to "sort" the unseen test data into categories, based on probabilities and likelihoods of the categories in the training dataset.
Preliminary Results
Task 1 is a classification task that identifies "COVID-19" speech and "healthy" speech. The Machine Learning classifier used is a Multilayer Perception and it showed an accuracy of 60.0%.
Task 2 aims to explore the accuracy of COVID-19 detection in the vowel data, and identifying which vowel carries the most COVID-19 information. See results in the live conference.
Task 3 aims to explore the accuracy of COVID-19 detection in varying paces of speech, and whether this has an impact on accuracy of diagnoses. See results in the live conference.
References
Guan, W.-j., Ni, Z.-y., Hu, Y., Liang, W.-h., Ou, C.-q., He, J.-x., . . . Hui, D. S. (2020). Clinical characteristics of coronavirus disease 2019 in China. New England journal of medicine, 382(18), 1708-1720.
Hassan, A., Su, Y., Sun, H., & Yih, W.-t. (2020). Proceedings of the First Workshop on Natural Language Interfaces. Paper presented at the Proceedings of the First Workshop on Natural Language Interfaces.
Ibrahim, N. K. (2020). Epidemiologic surveillance for controlling Covid-19 pandemic: types, challenges and implications. Journal of infection and public health, 13(11), 1630-1638.
Josefsson, K. W. (2021). Perspectives of life in Sweden during the COVID-19 pandemic. Journal of Clinical Sport Psychology, 15(1), 80-86.
Lee, L., Loudon, R. G., Jacobson, B. H., & Stuebing, R. (1993). Speech breathing in patients with lung disease. American Review of Respiratory Disease, 147, 1199-1199.
Ohala, J. J. (1990). Respiratory activity in speech. In Speech production and speech modelling (pp. 23-53): Springer.
Sharma, N., Krishnan, P., Kumar, R., Ramoji, S., Chetupalli, S. R., Ghosh, P. K., & Ganapathy, S. (2020). Coswara--A Database of Breathing, Cough, and Voice Sounds for COVID-19 Diagnosis. arXiv preprint arXiv:2005.10548.
The New Foscote Hospital. (2019). Private COVID Testing. Retrieved from https://thefoscotehospital.co.uk/private-covid-testing/
UK Prime Minister [@10DowningStreet]. (2020, 20/10/2020). Retrieved 06/03/2022 from https://twitter.com/10downingstreet/status/1318511921184841729
Van Nhu, H., Tuyet-Hanh, T. T., Van, N. T. A., Linh, T. N. Q., & Tien, T. Q. (2020). Knowledge, attitudes, and practices of the Vietnamese as key factors in controlling COVID-19. Journal of community health, 45(6), 1263-1269.
WHO, C. O. (2020). World health organization. Responding to Community Spread of COVID-19. Reference WHO/COVID-19/Community_Transmission/2020.1.
Acknowledgements
A massive thank you to my supervisor Dr. George Brown, for her continued support and patience through the years. I would also like to thank Dr. Andrew Hardie for his mentorship and the encouragement. I'm very thankful to Lily's insights on web page design and the tremendous support from my friends. Lastly, a special thanks to my parents, who set me off on the road of academia a long time ago.
Slide 1 image (max 2mb)
Slide 1 video (YouTube/Vimeo embed code)
Image 1 Caption
Slide 2 image (max 2mb)
Slide 2 video (YouTube/Vimeo embed code)
Image 2 Caption
Slide 3 image (max 2mb)
Slide 3 video (YouTube/Vimeo embed code)
Image 3 Caption
Slide 4 image (max 2mb)
Slide 4 video (YouTube/Vimeo embed code)
Image 4 Caption
Slide 5 image (max 2mb)
Slide 5 video (YouTube/Vimeo embed code)
Image 5 Caption
Slide 6 image (max 2mb)
Slide 6 video (YouTube/Vimeo embed code)
Image 6 Caption
Slide 7 image (max 2mb)
Slide 7 video (YouTube/Vimeo embed code)
Image 7 Caption
Slide 8 image (max 2mb)
Slide 8 video (YouTube/Vimeo embed code)
Image 8 Caption
Slide 9 image (max 2mb)
Slide 9 video (YouTube/Vimeo embed code)
Image 9 Caption
Slide 10 image (max 2mb)
Slide 20 video (YouTube/Vimeo embed code)
Image 10 Caption
Caption font
Text
Image (max size: 2mb)
Or drag a symbol into the upload area
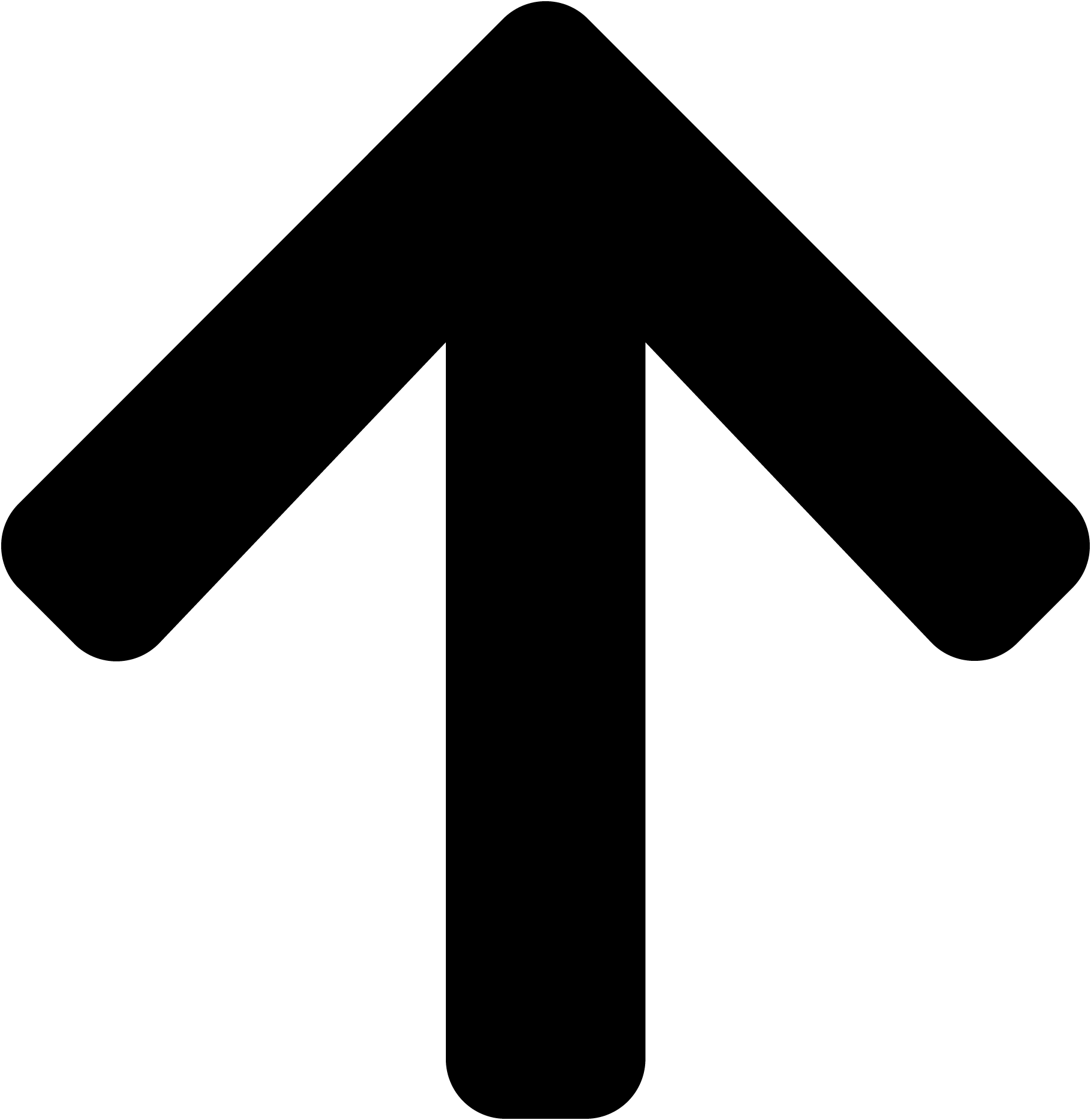
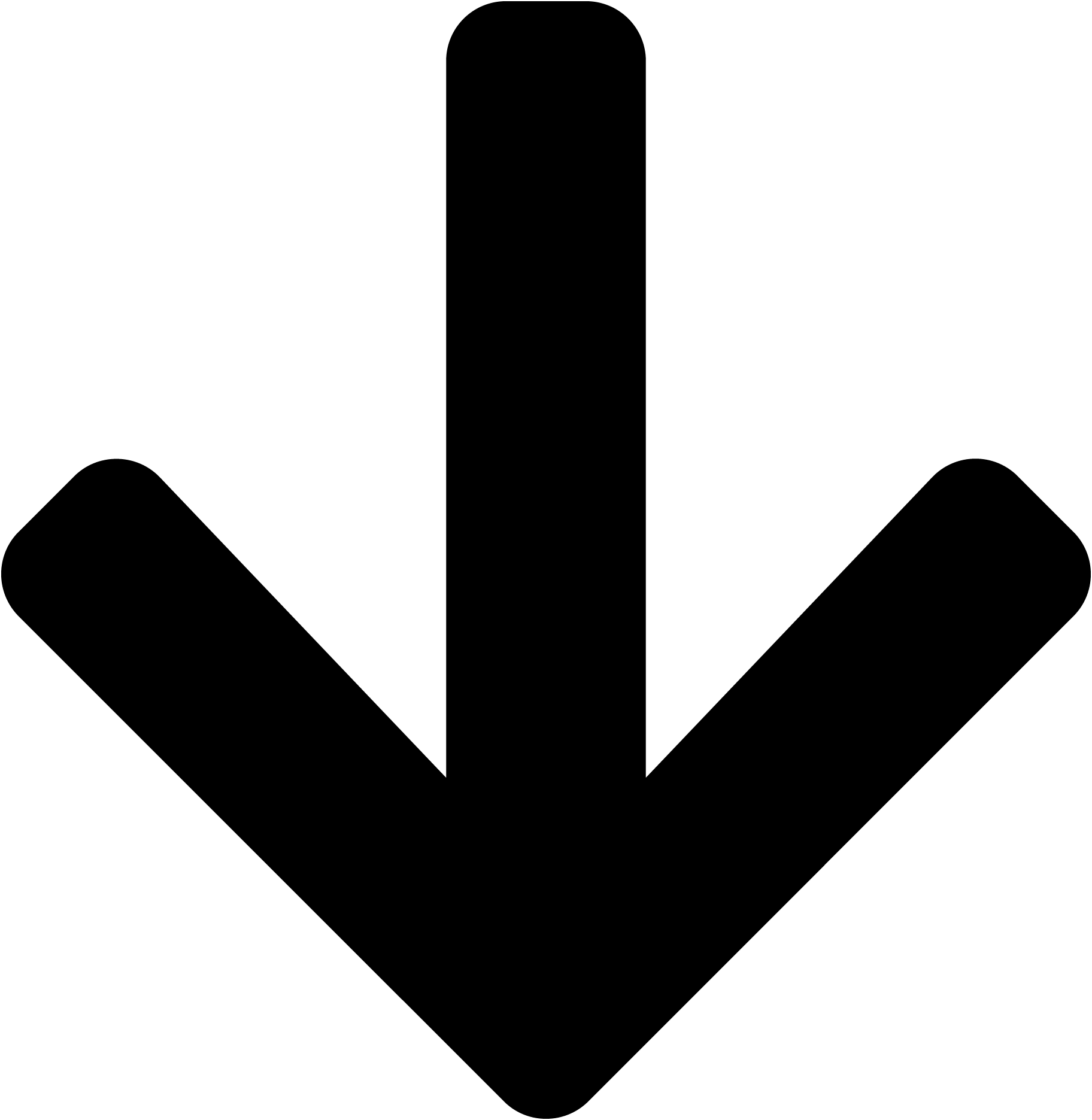
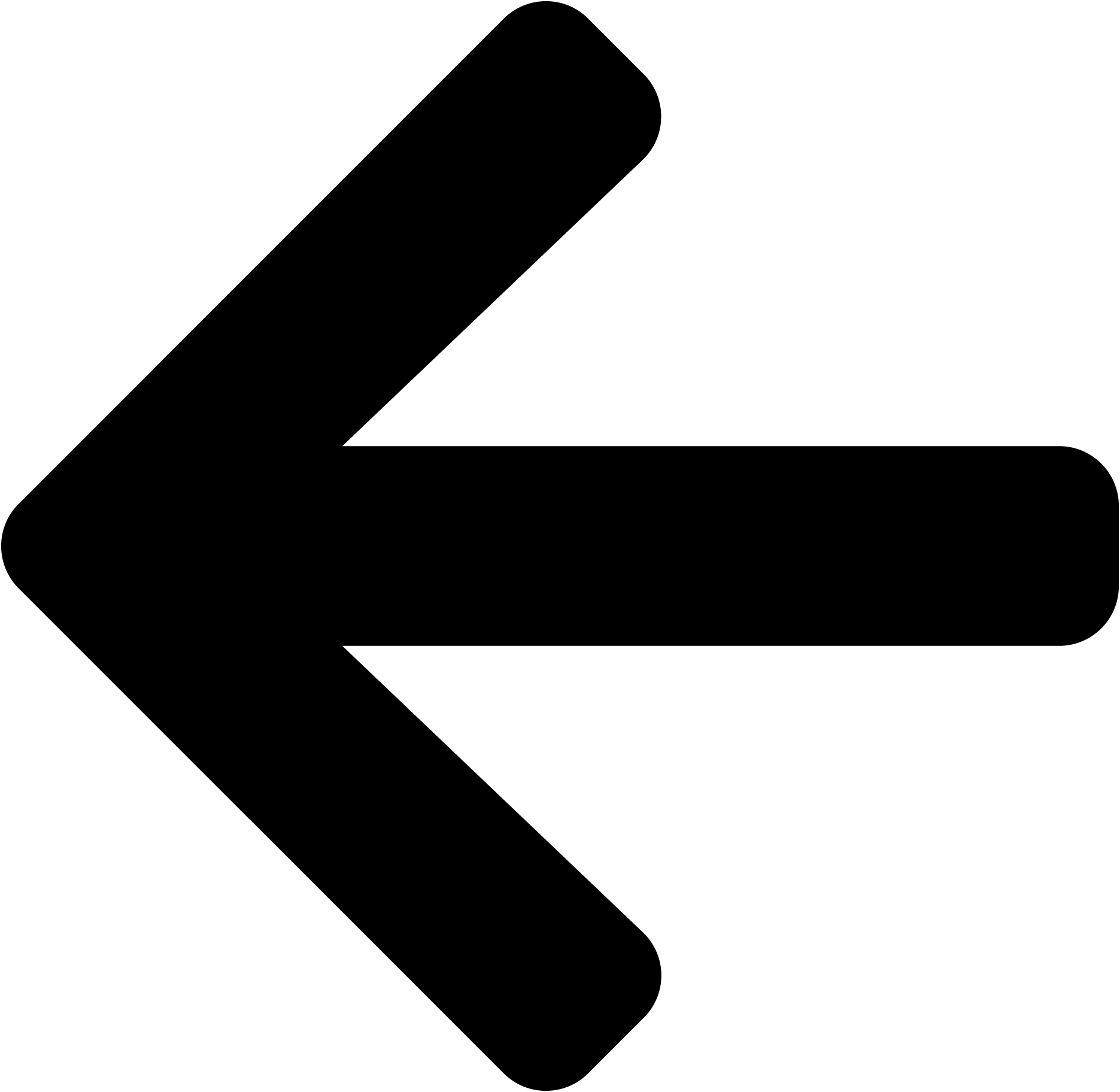
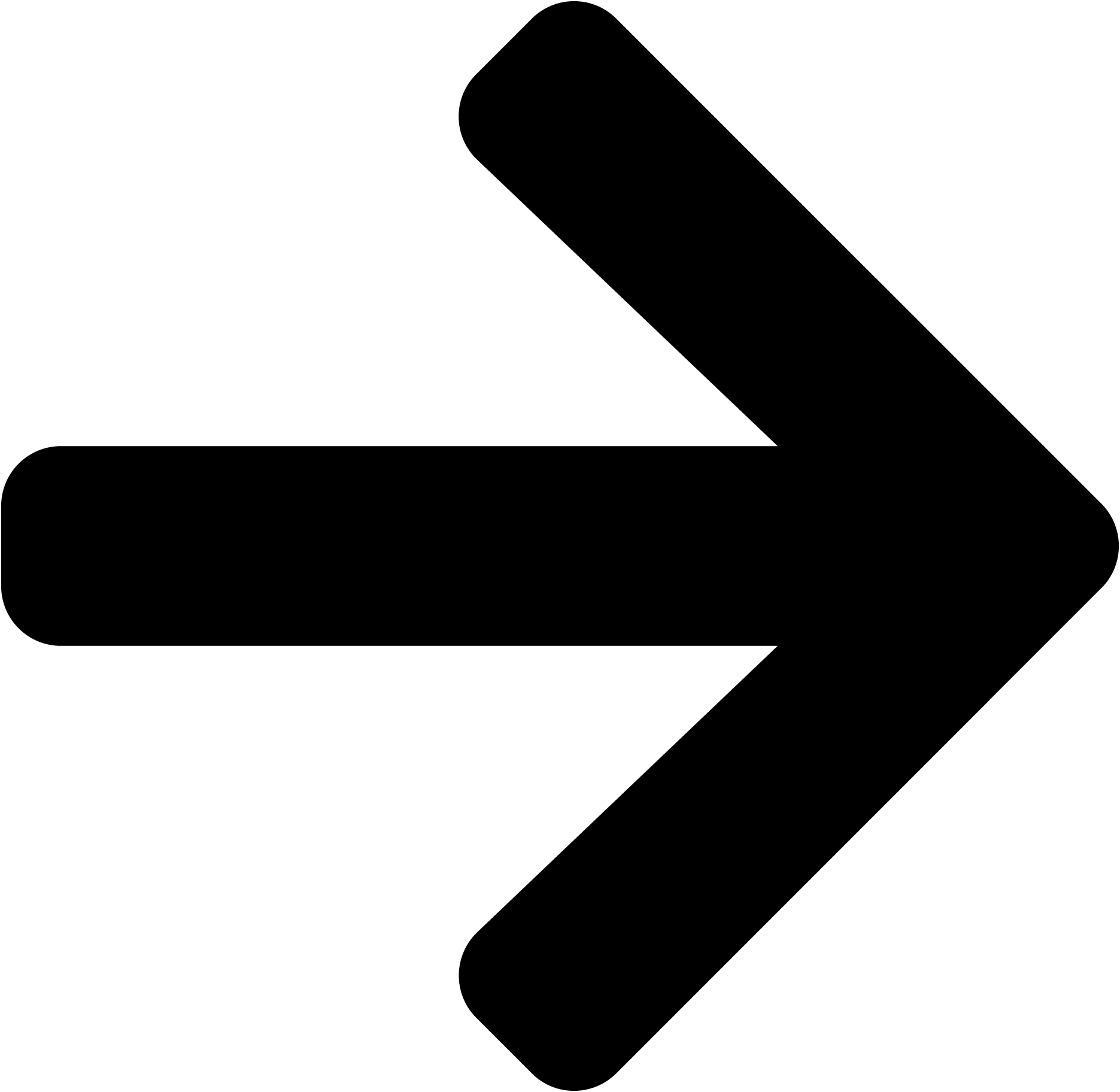
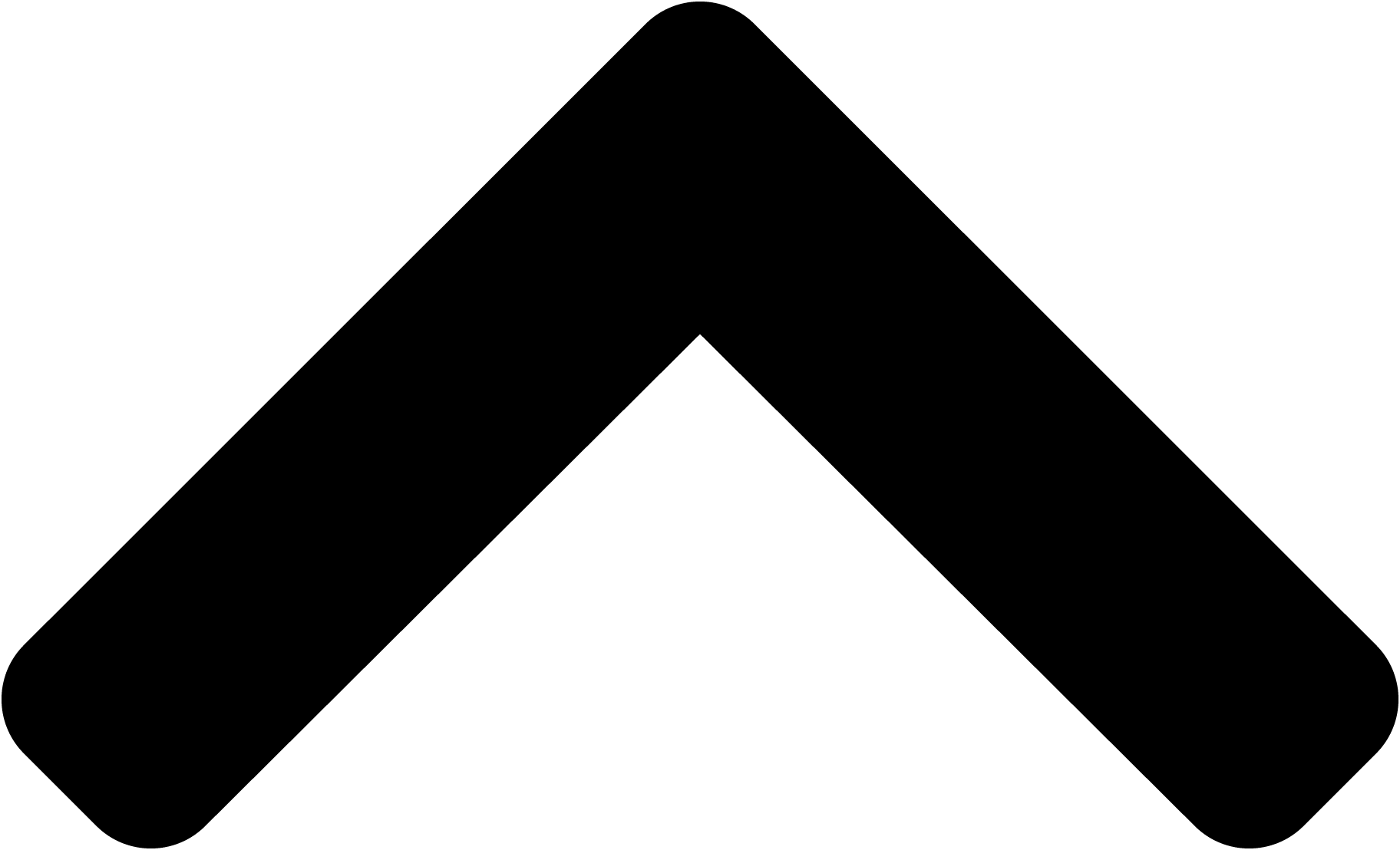
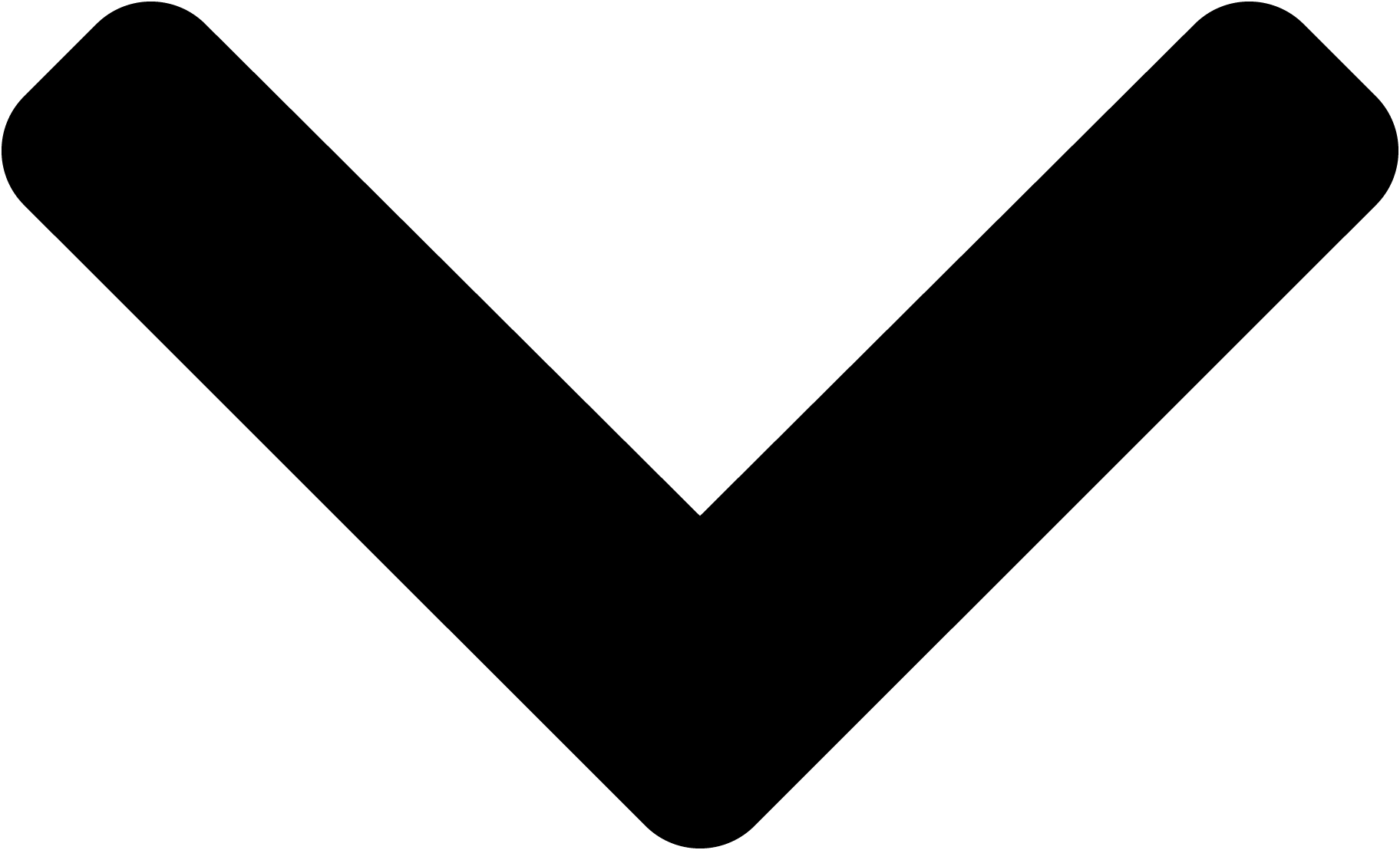
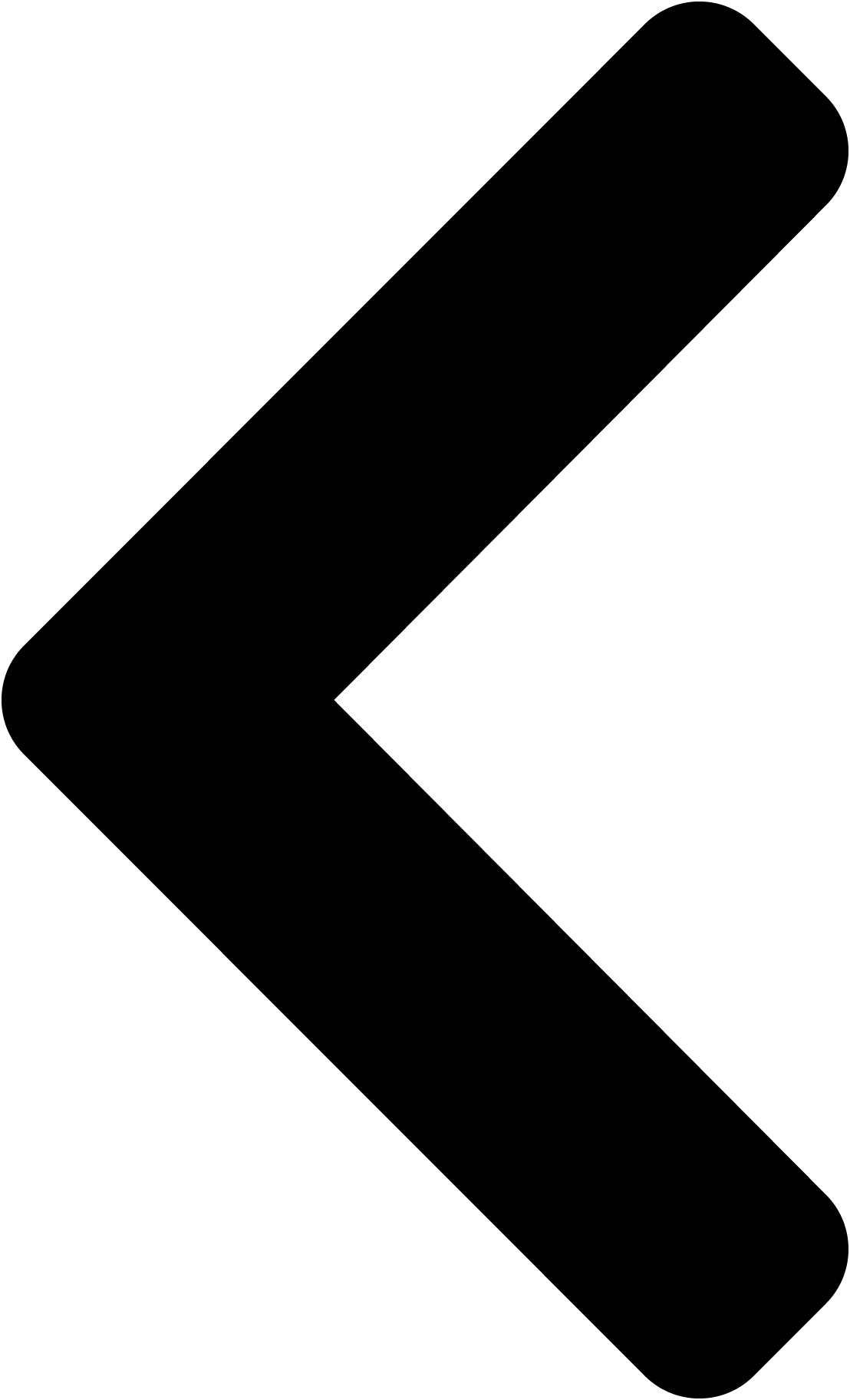
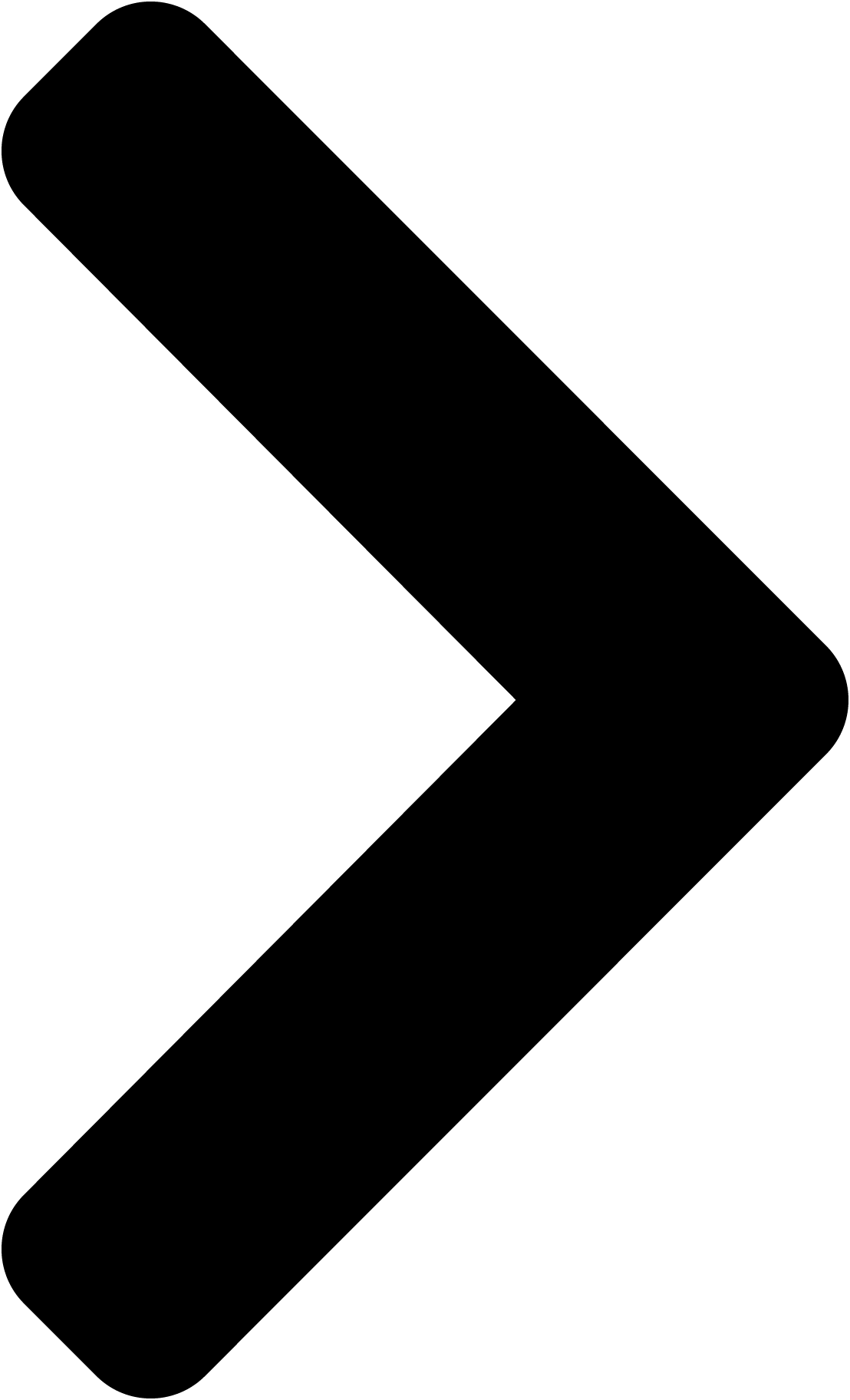
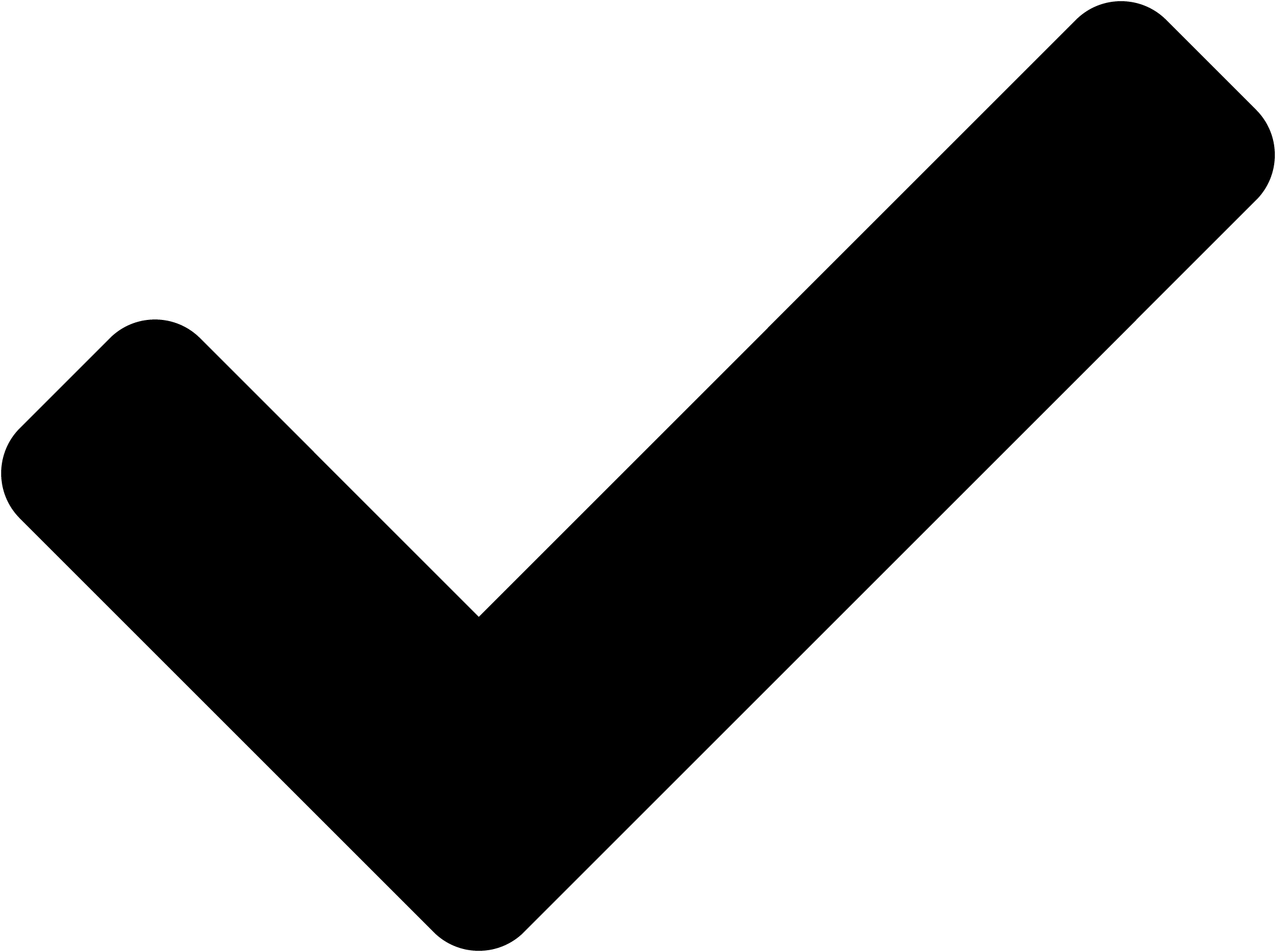
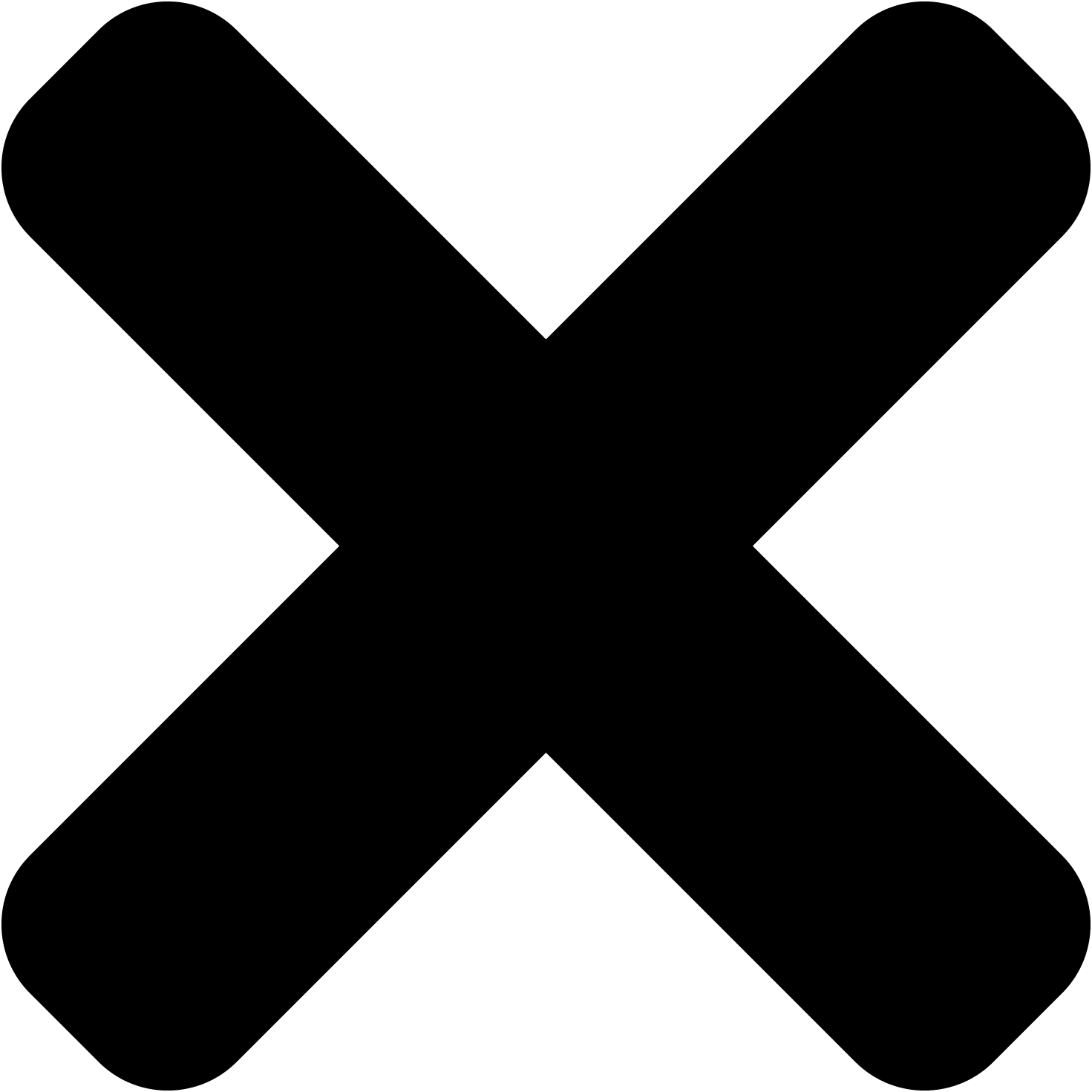
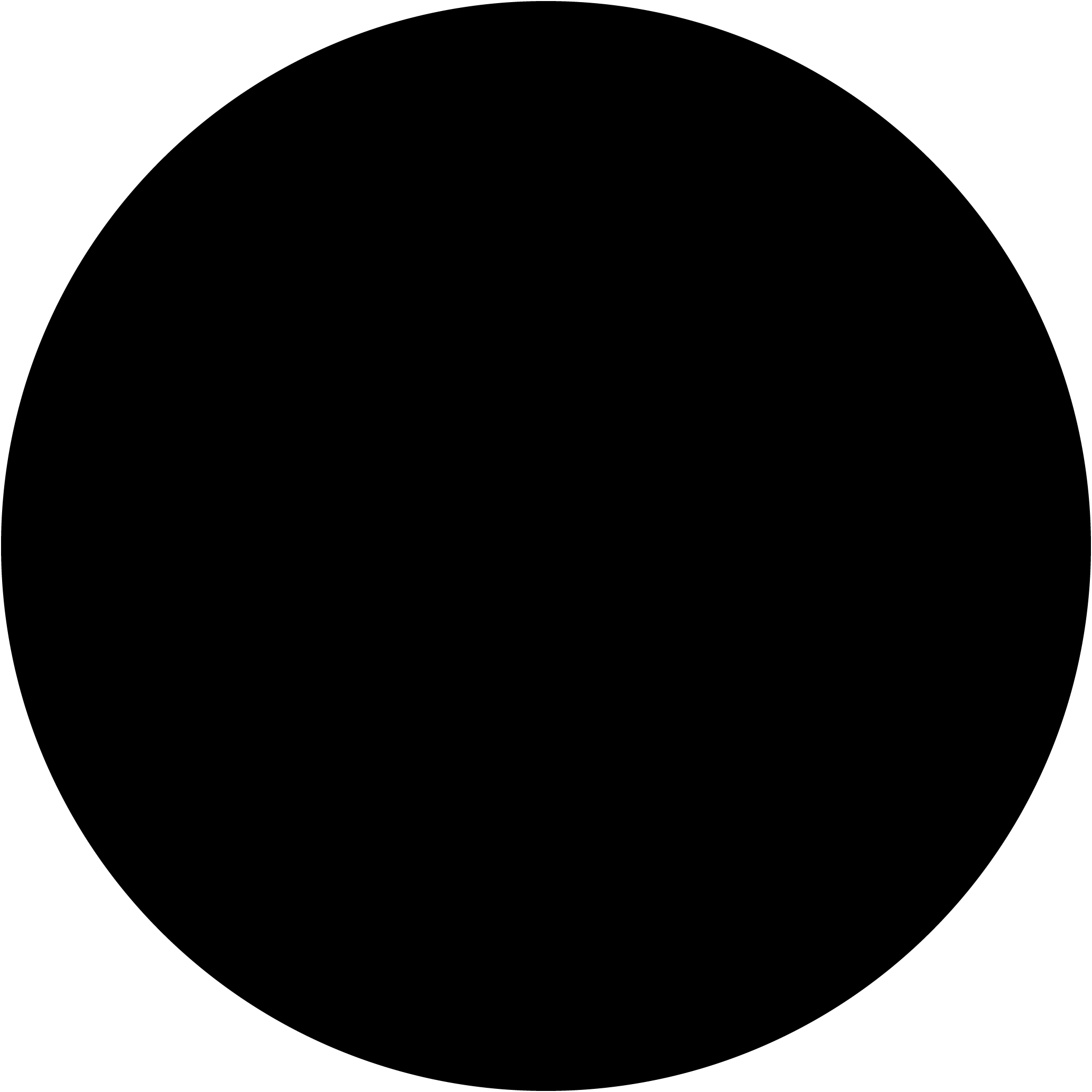
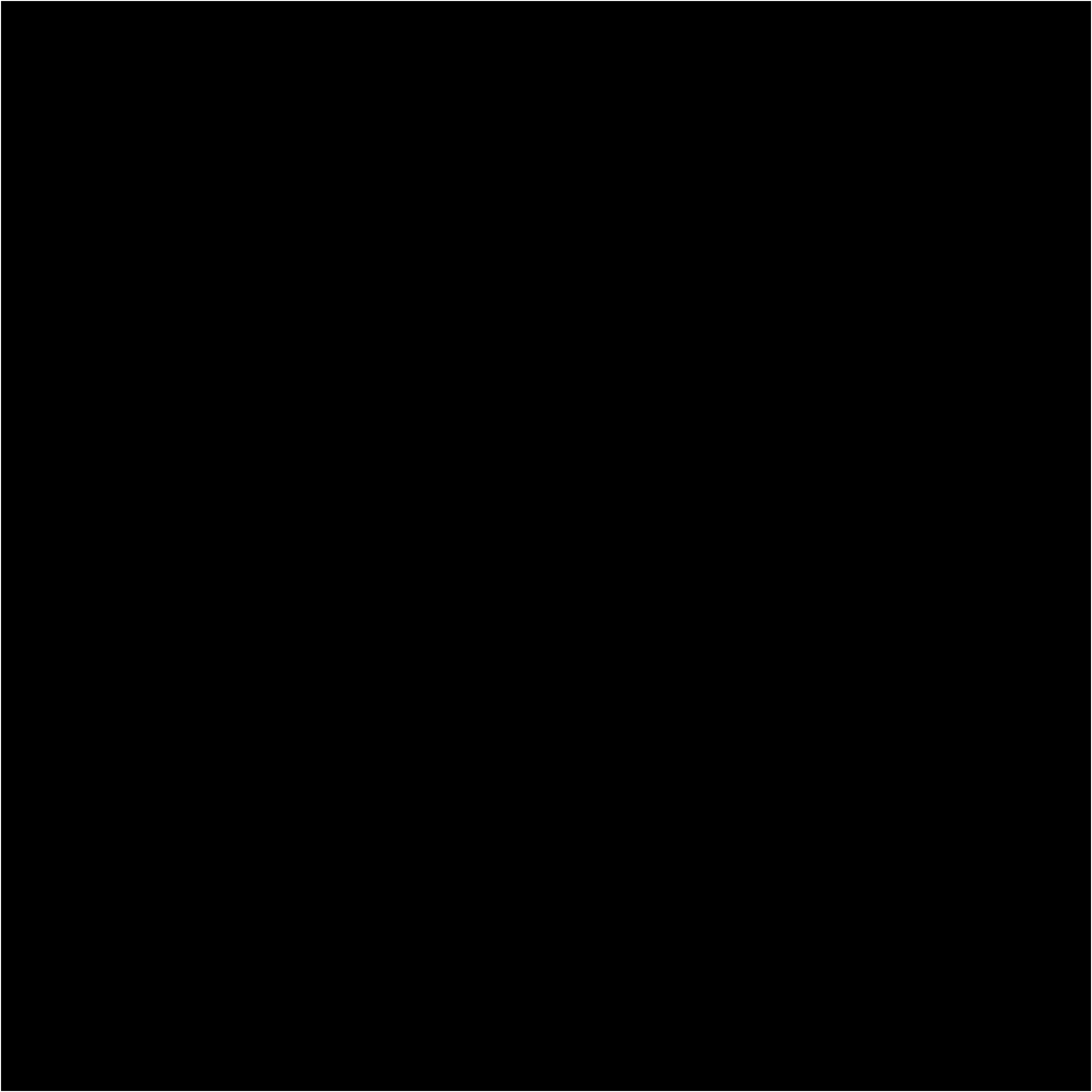
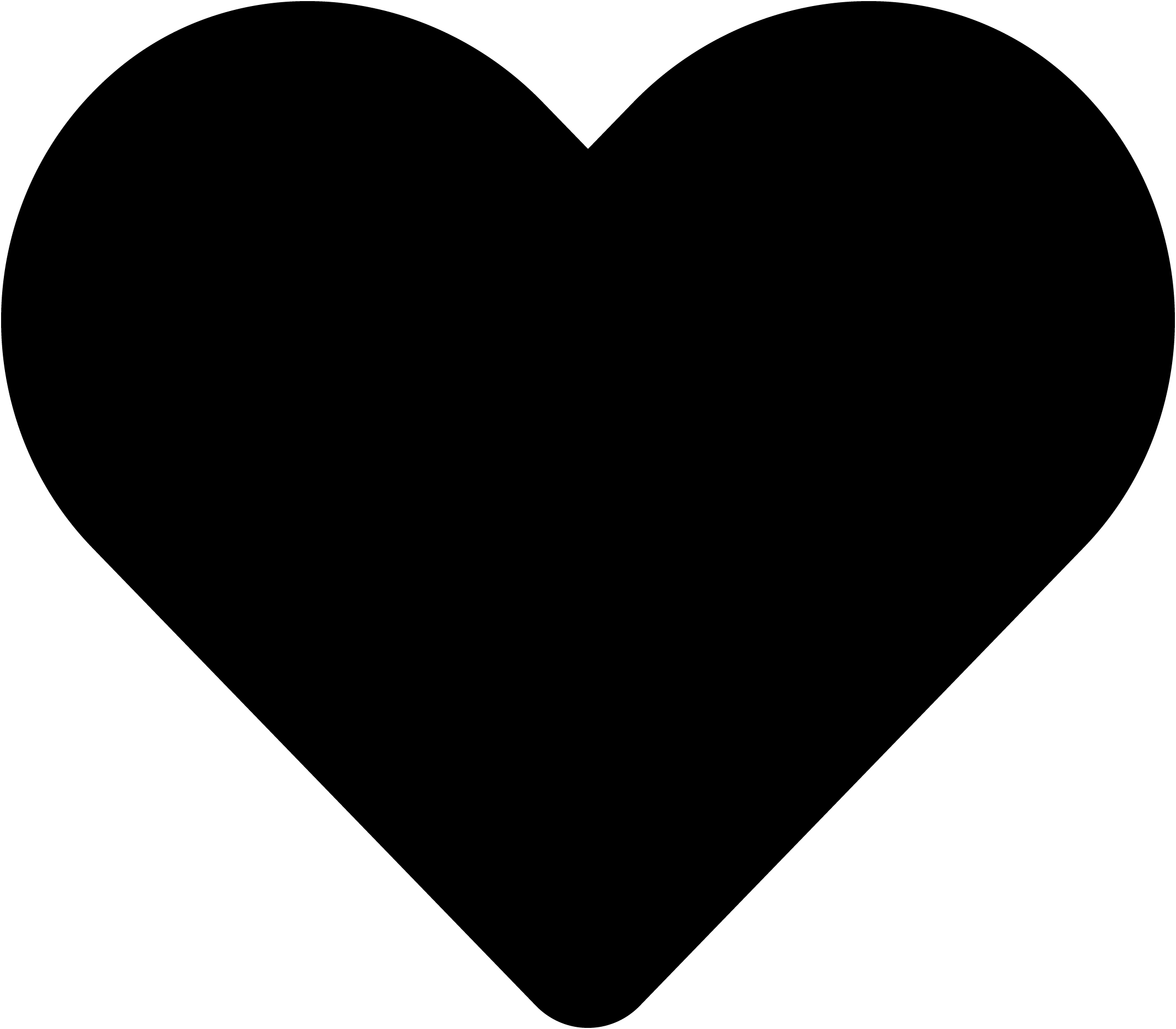
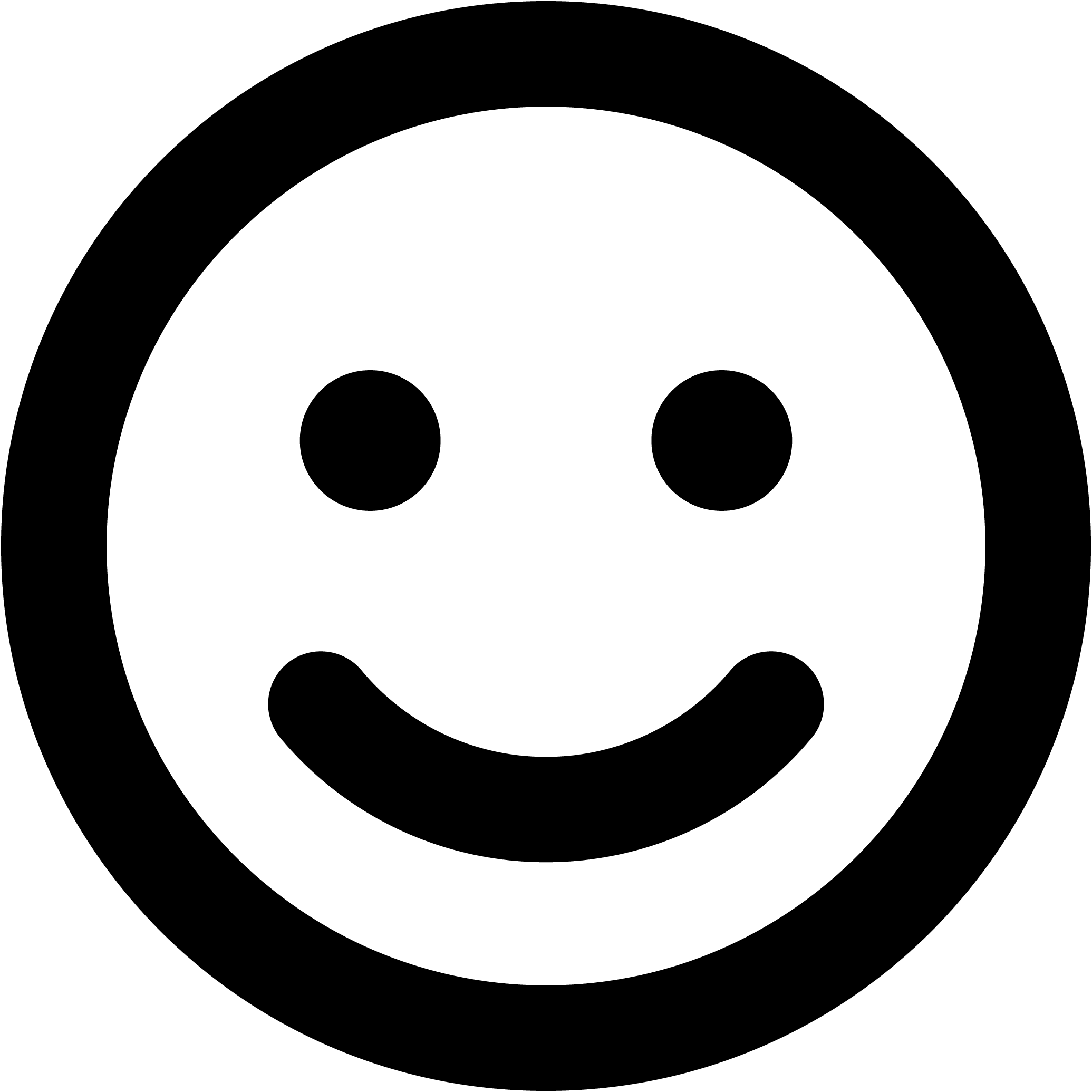
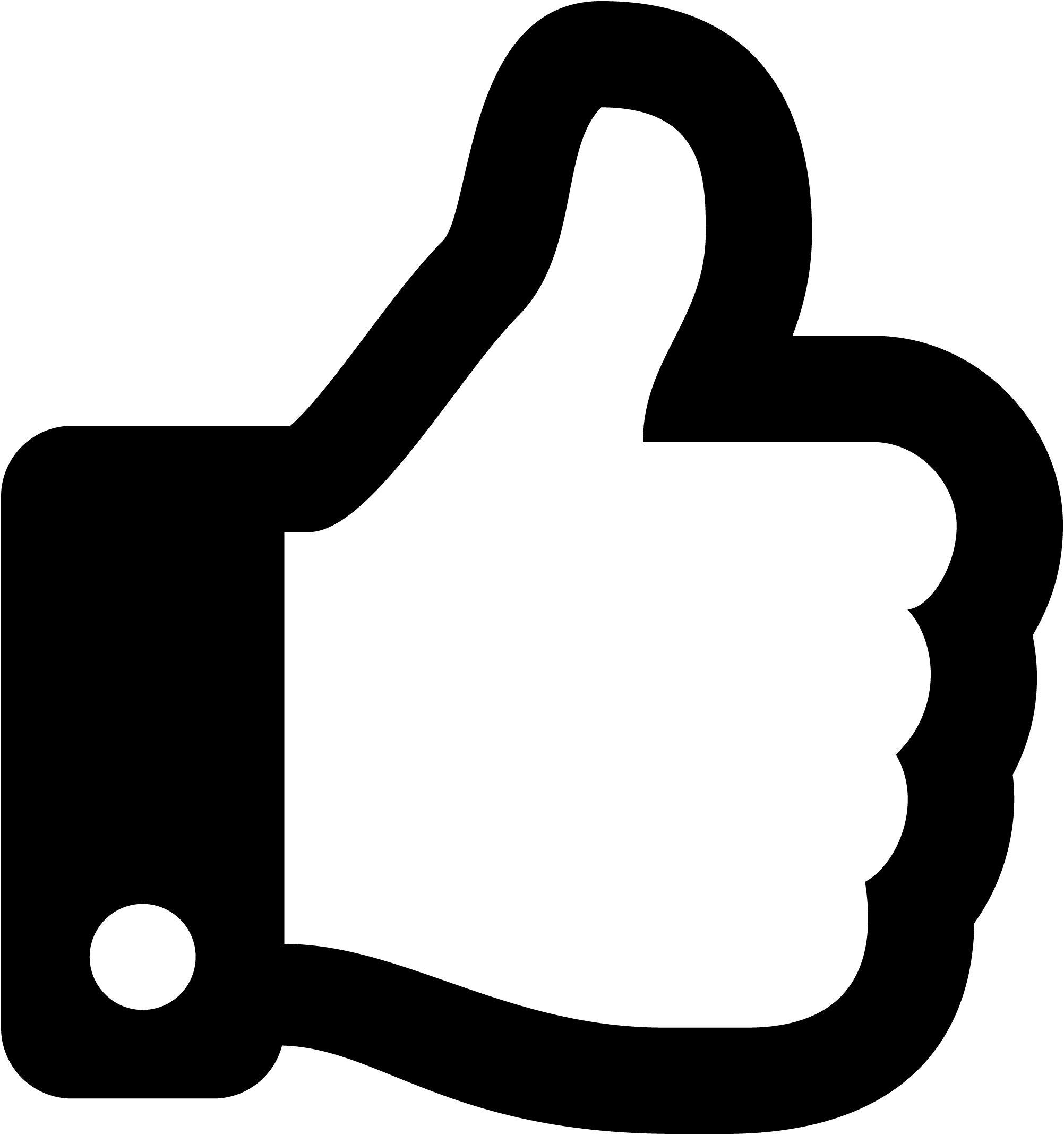
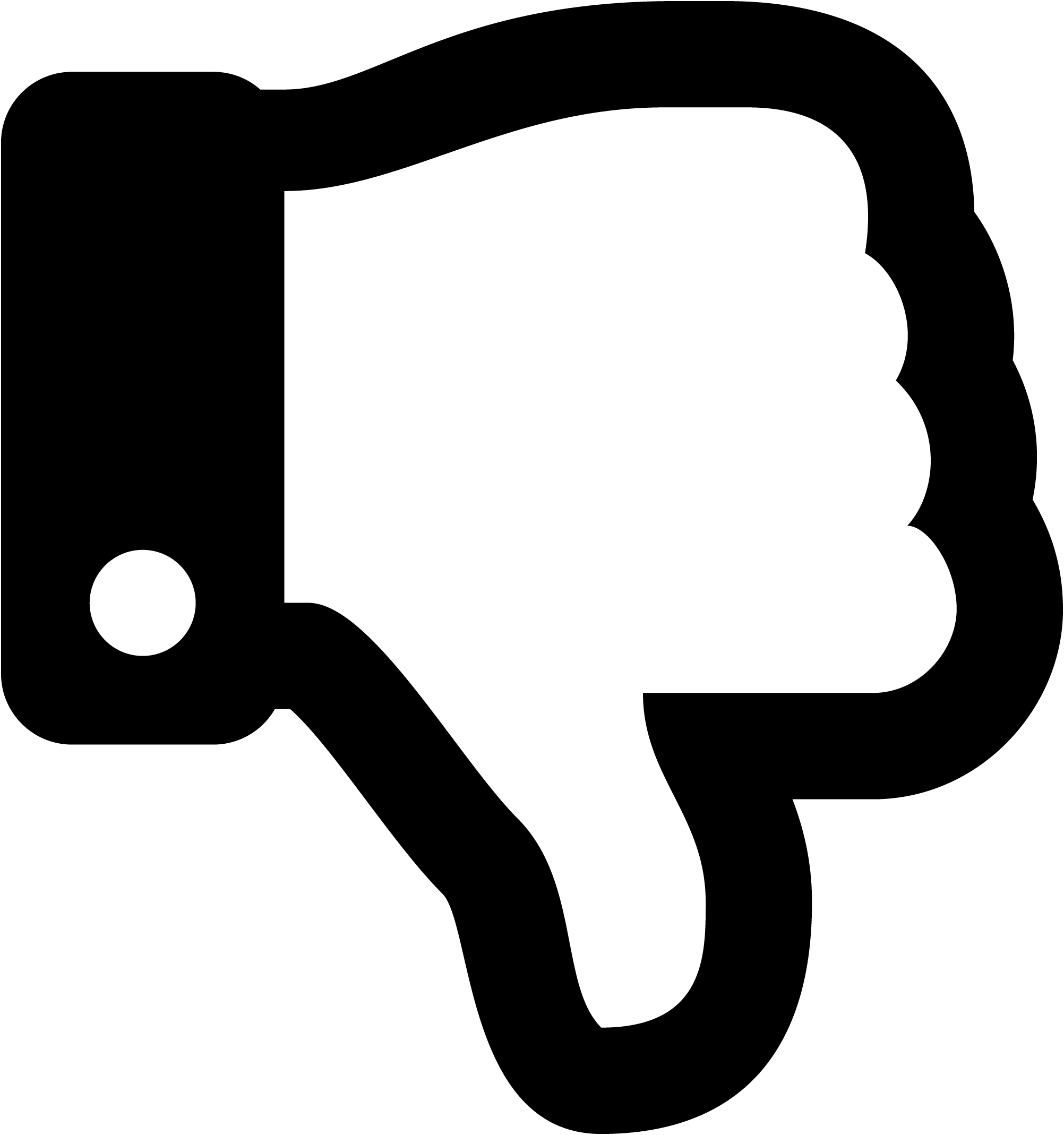
Image description/alt-tag
Image caption
Image link
Rollover Image (max size: 2mb)
Or drag a symbol into the upload area
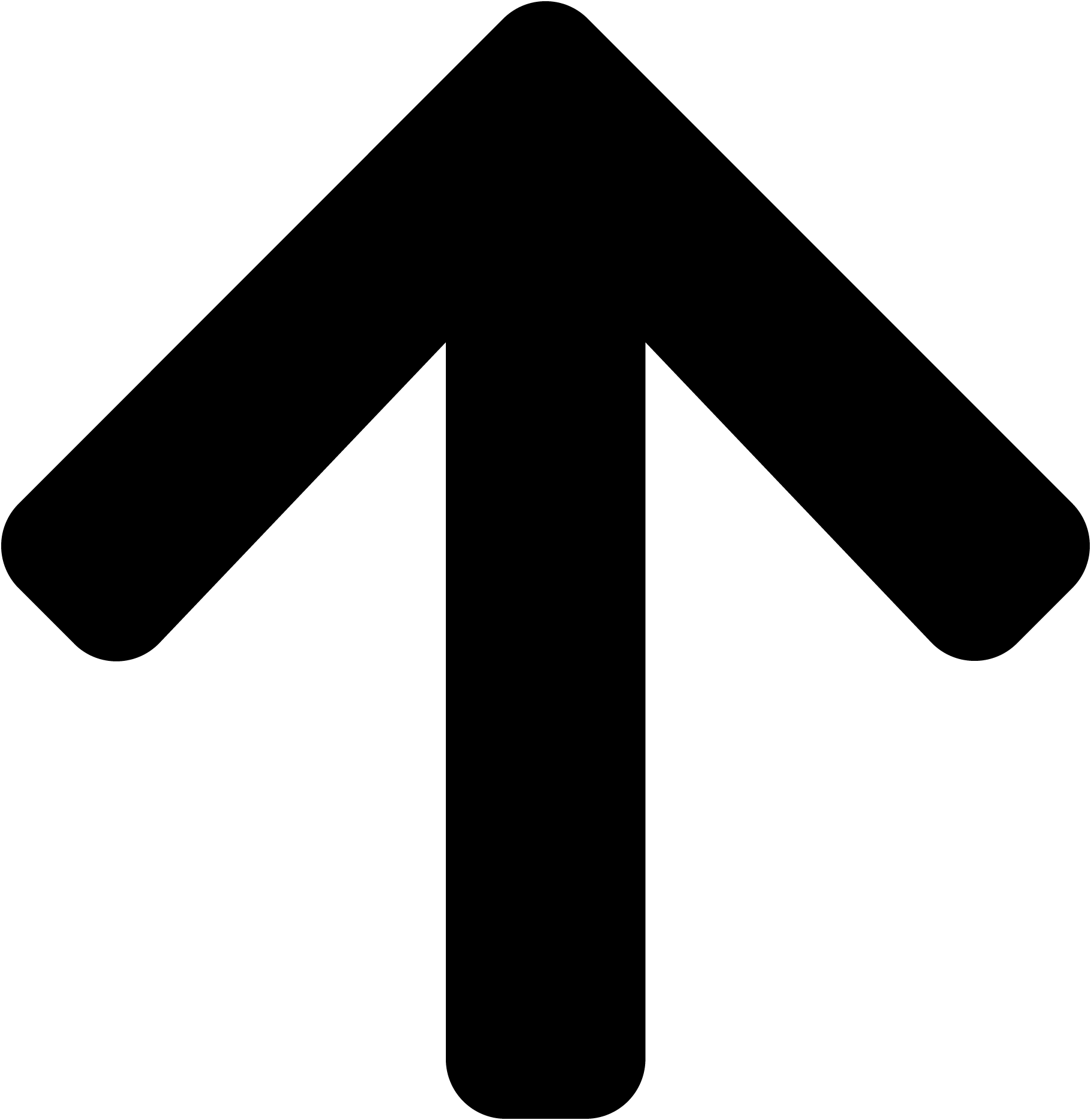
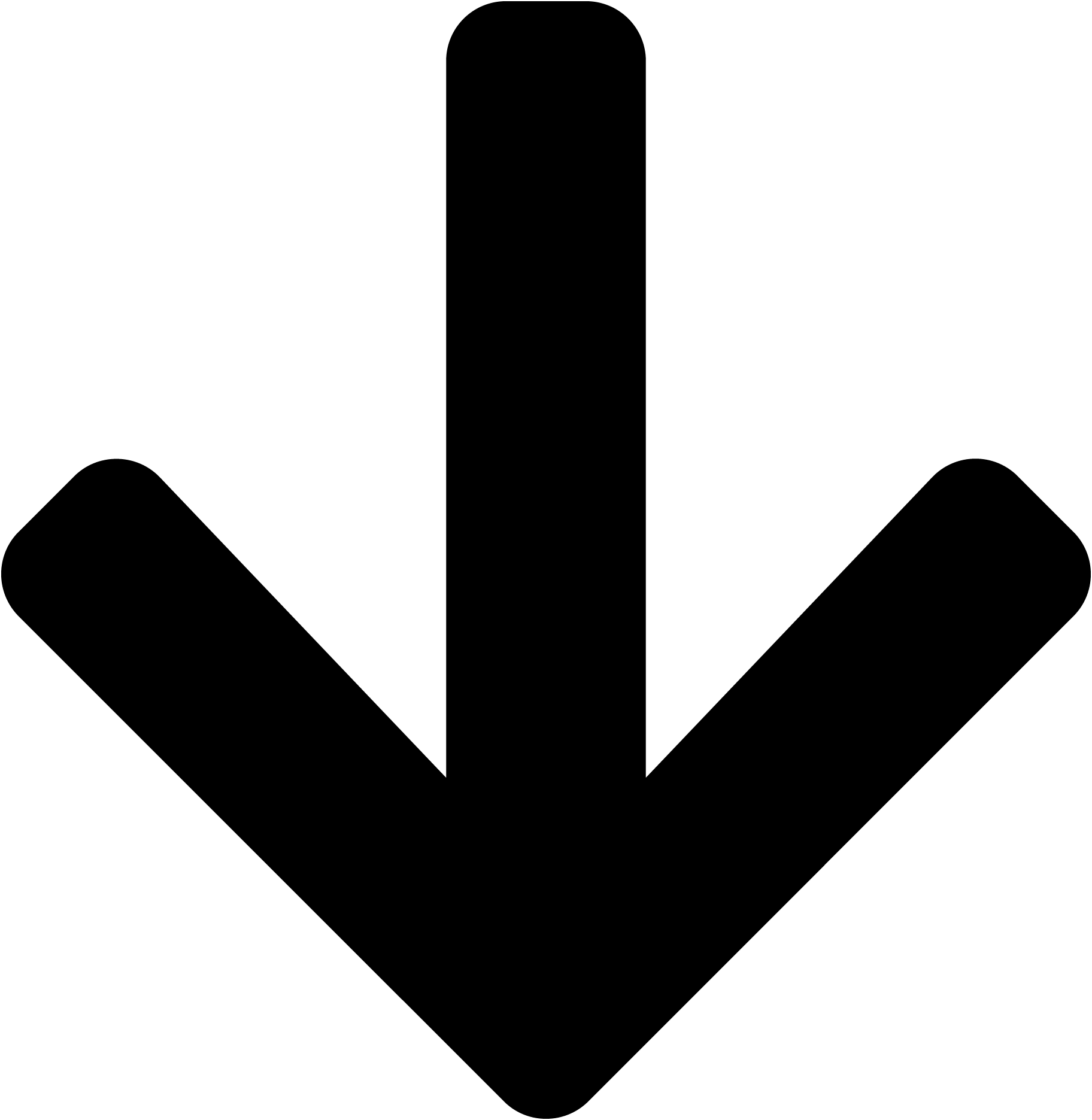
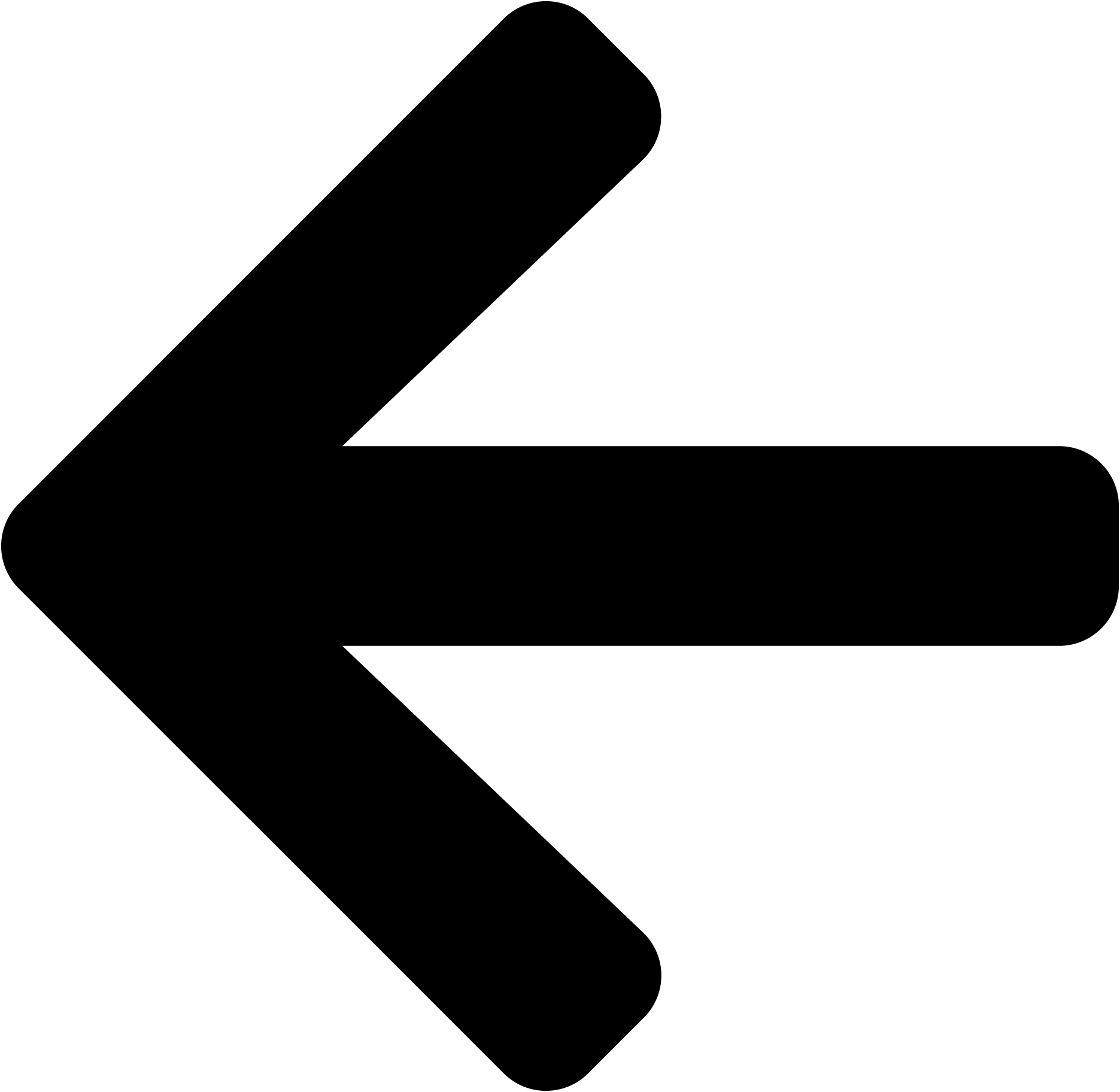
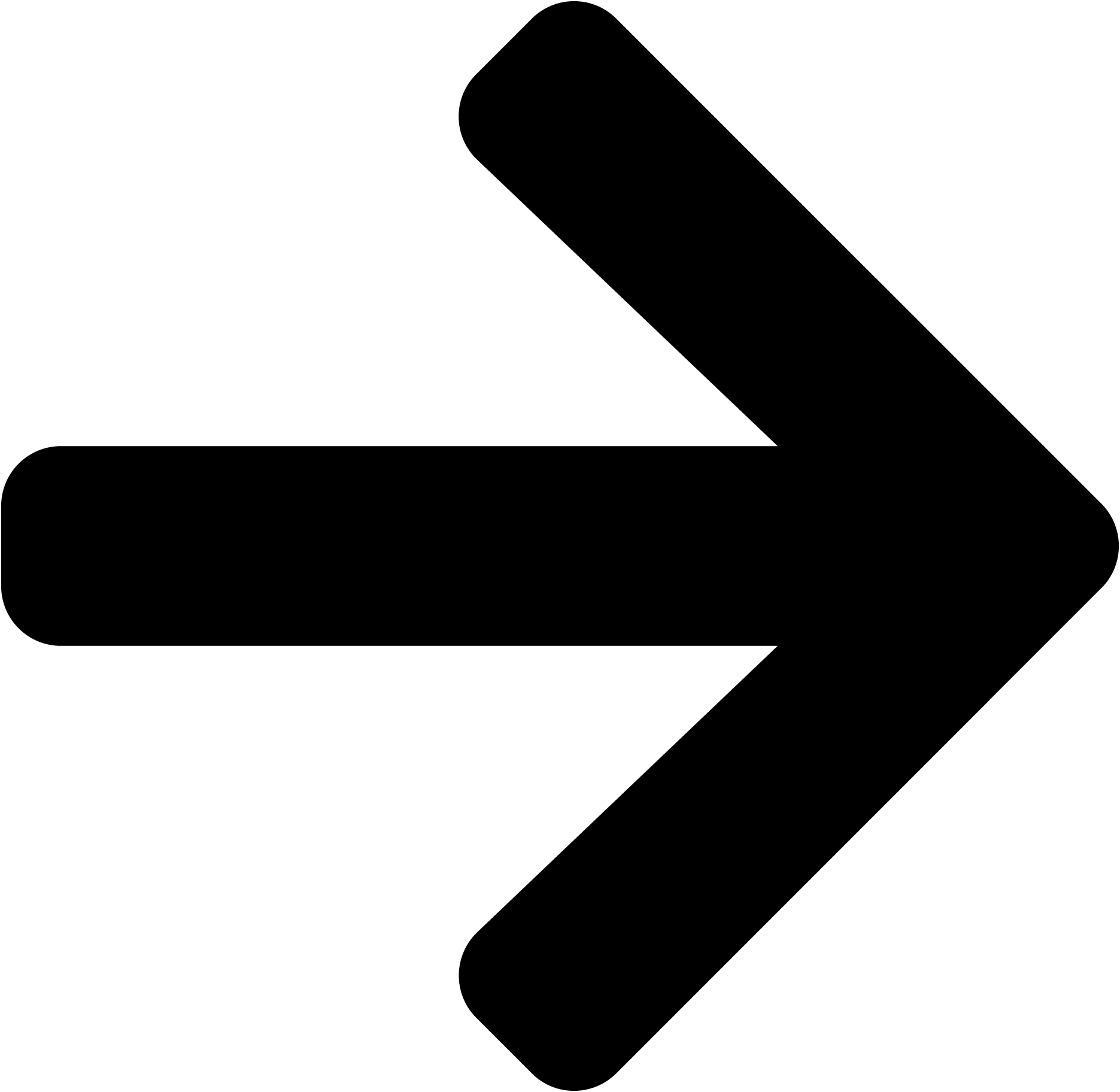
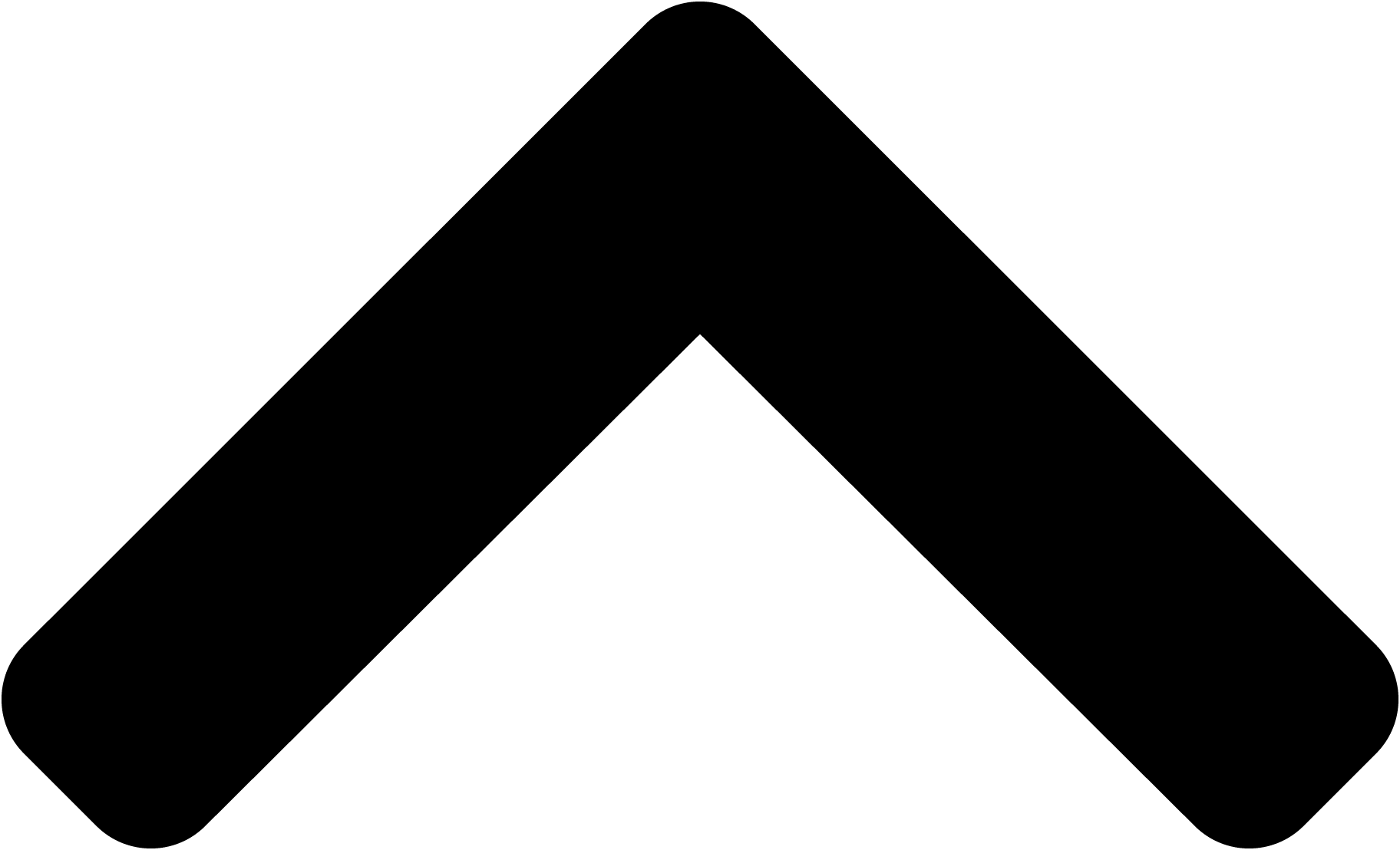
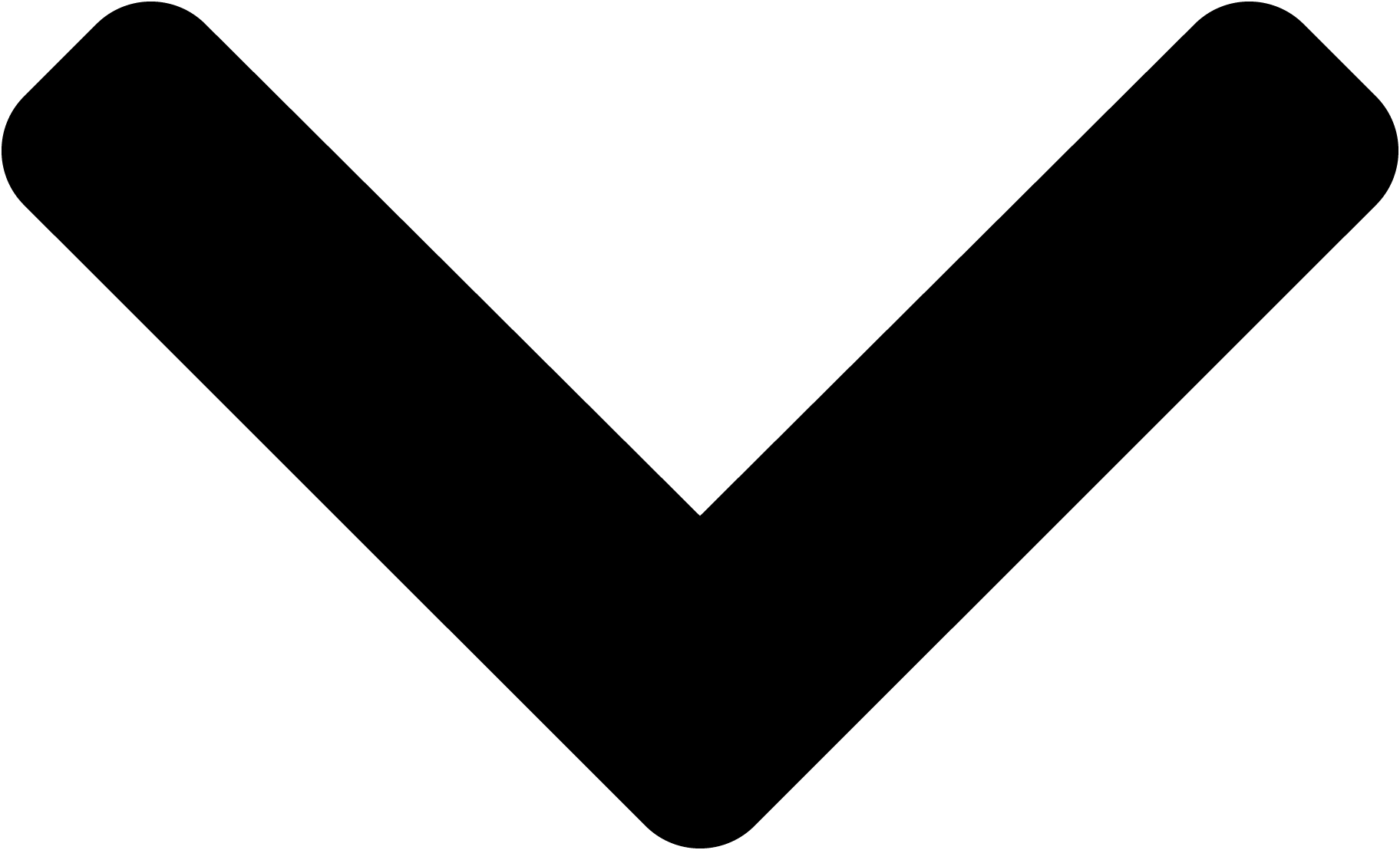
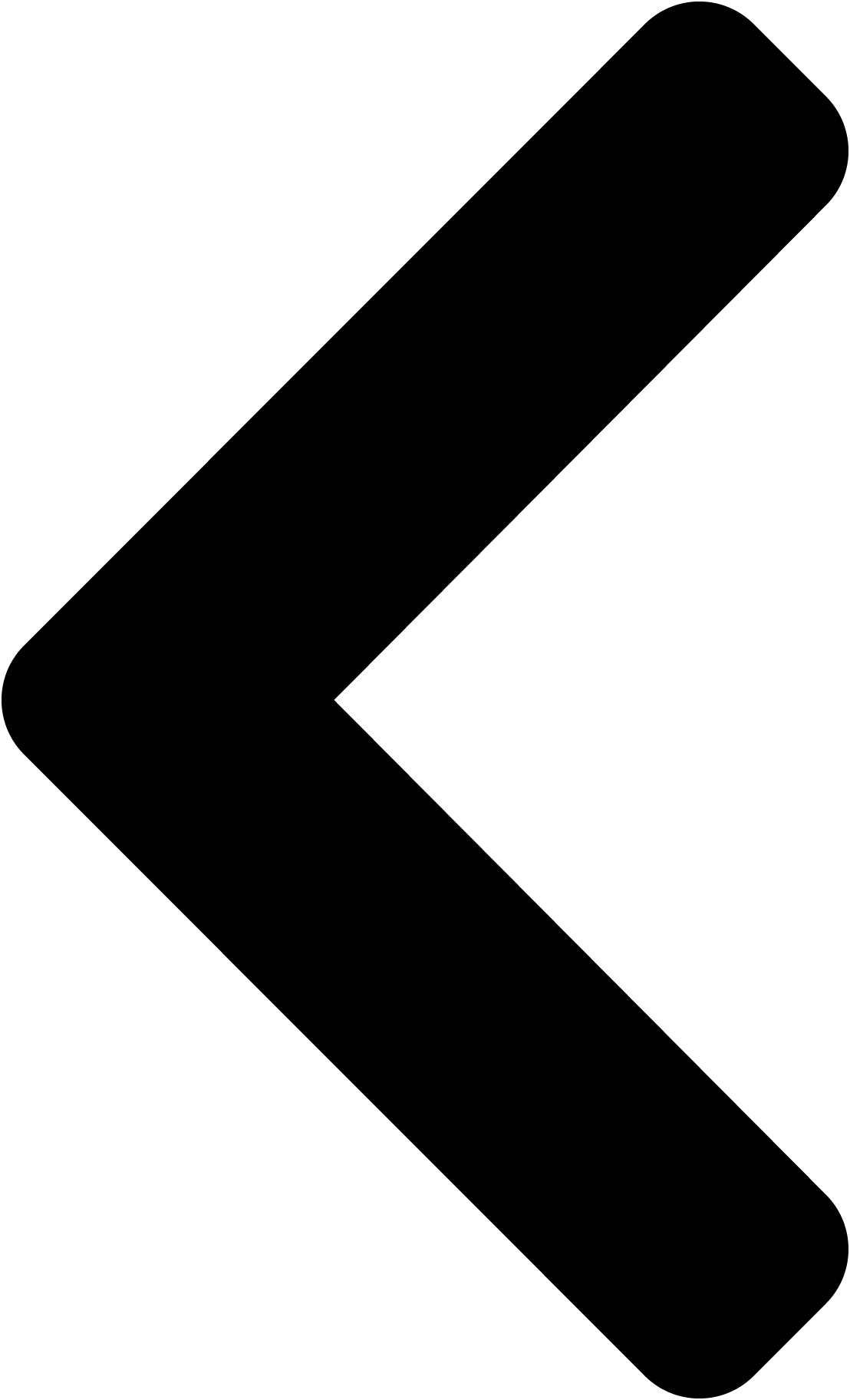
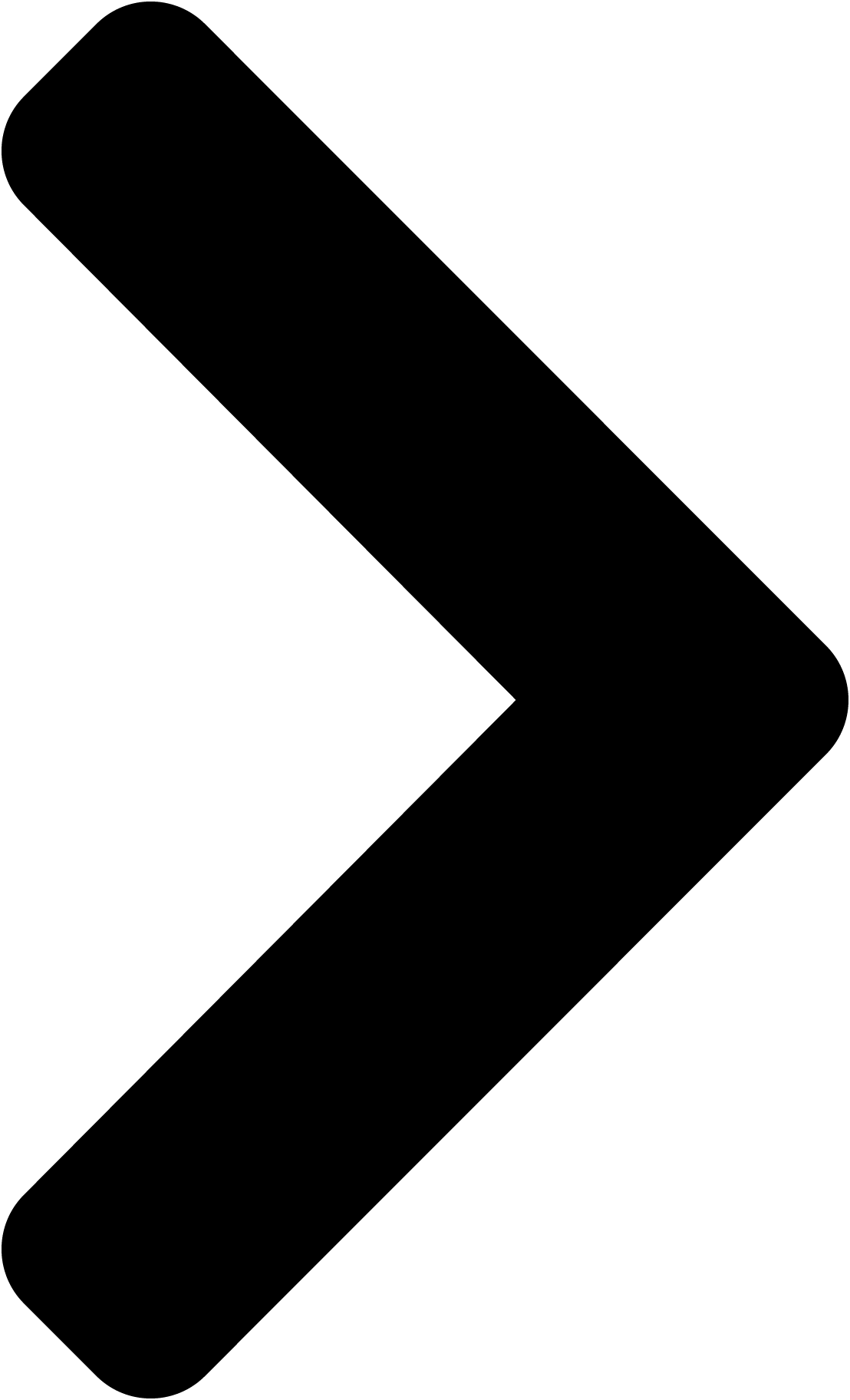
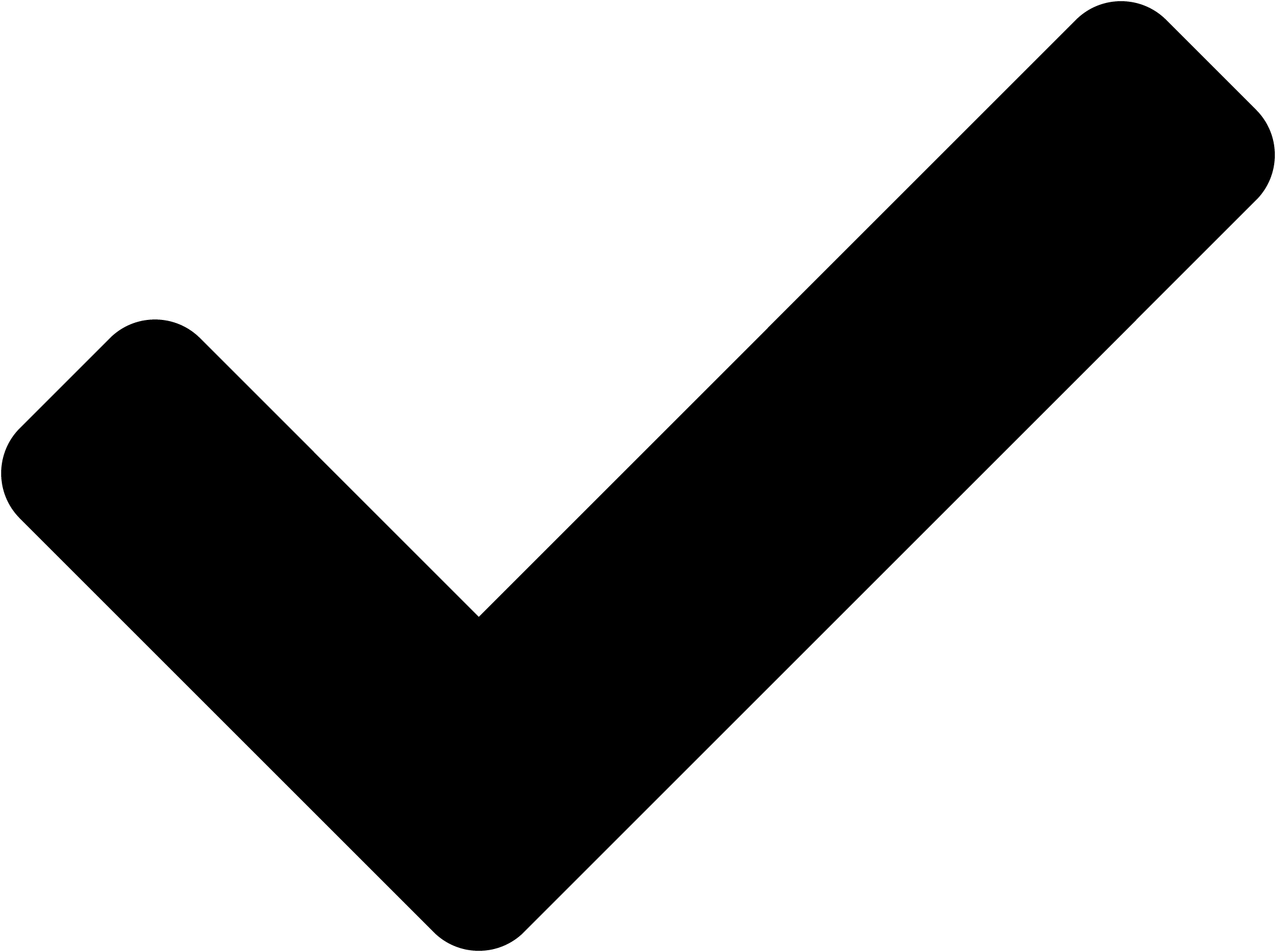
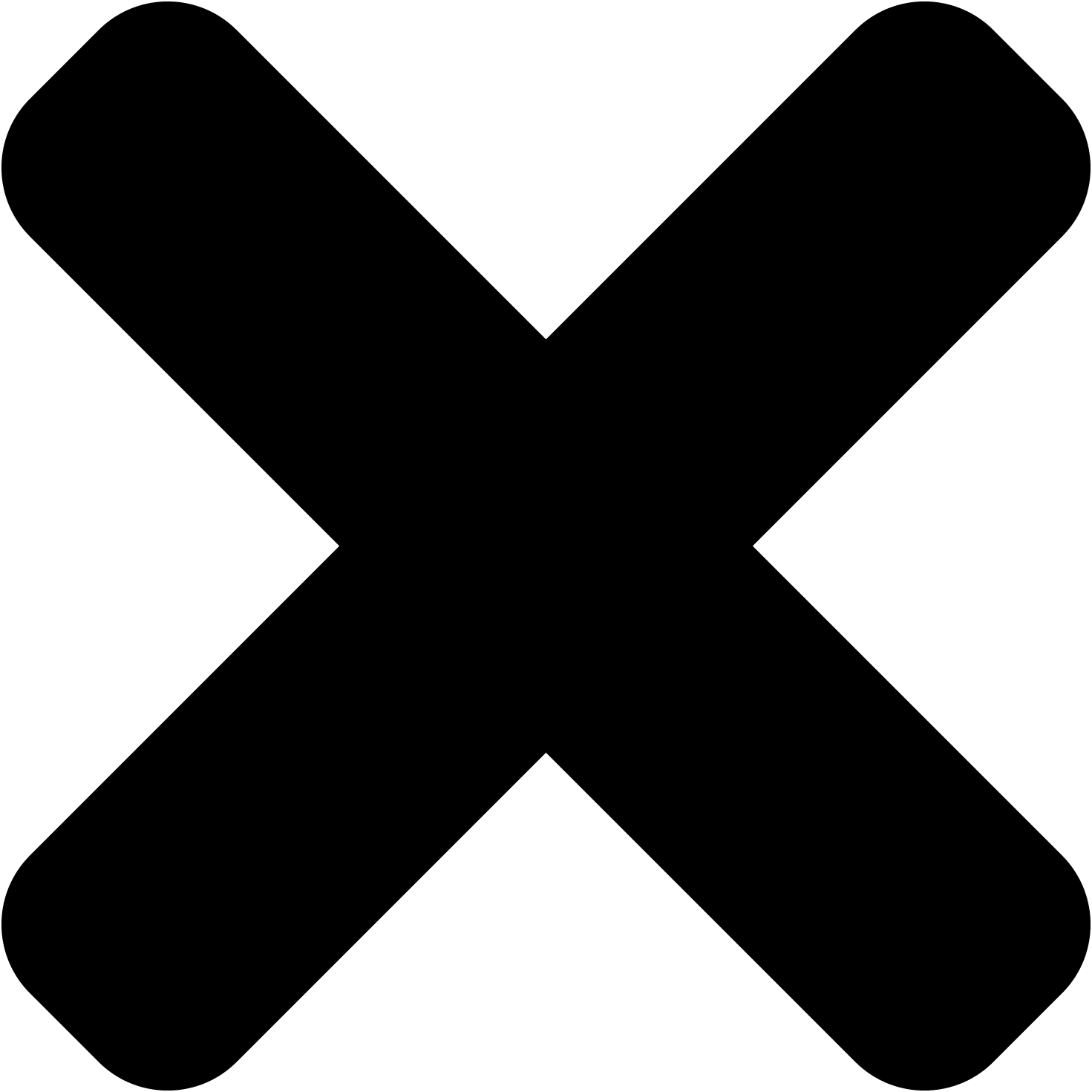
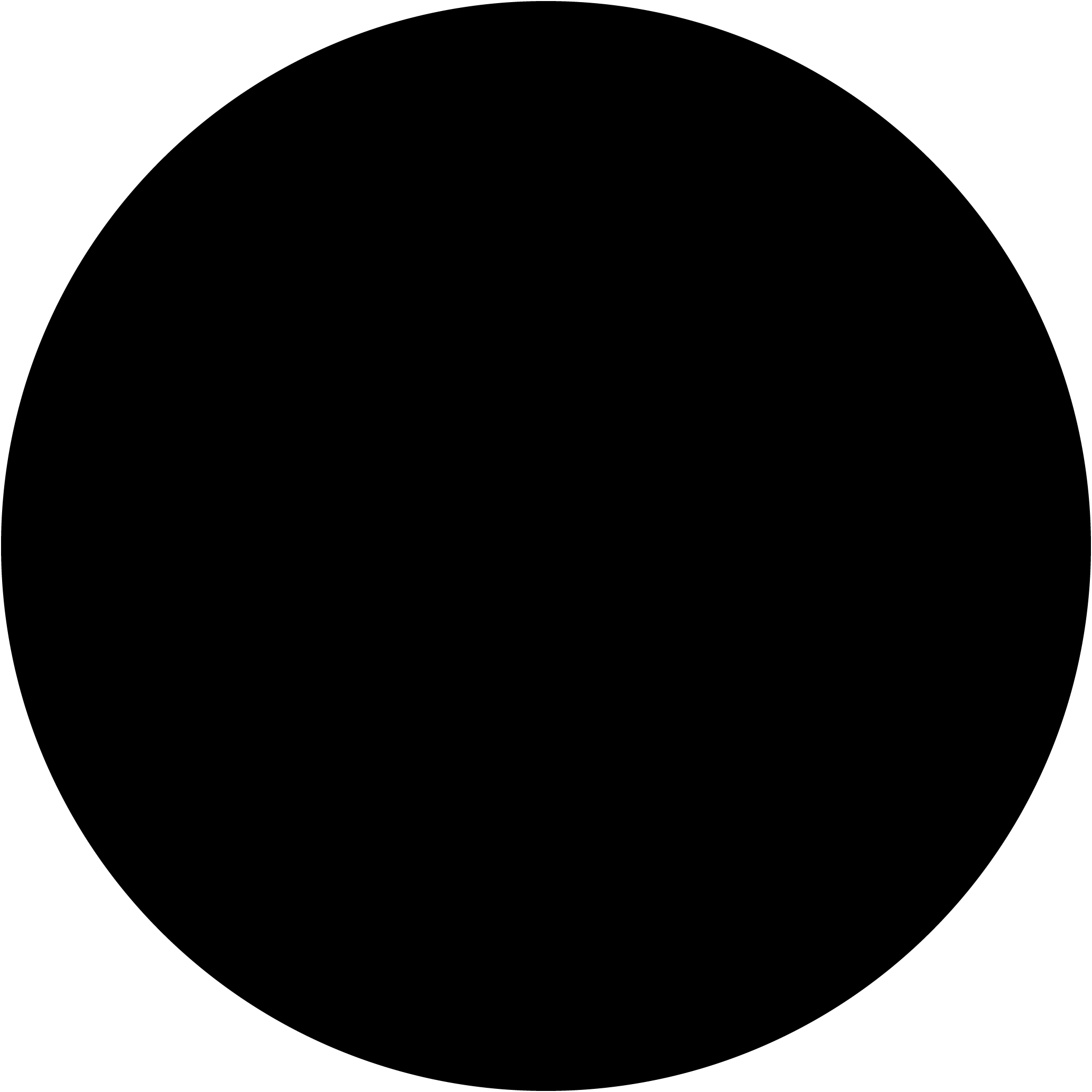
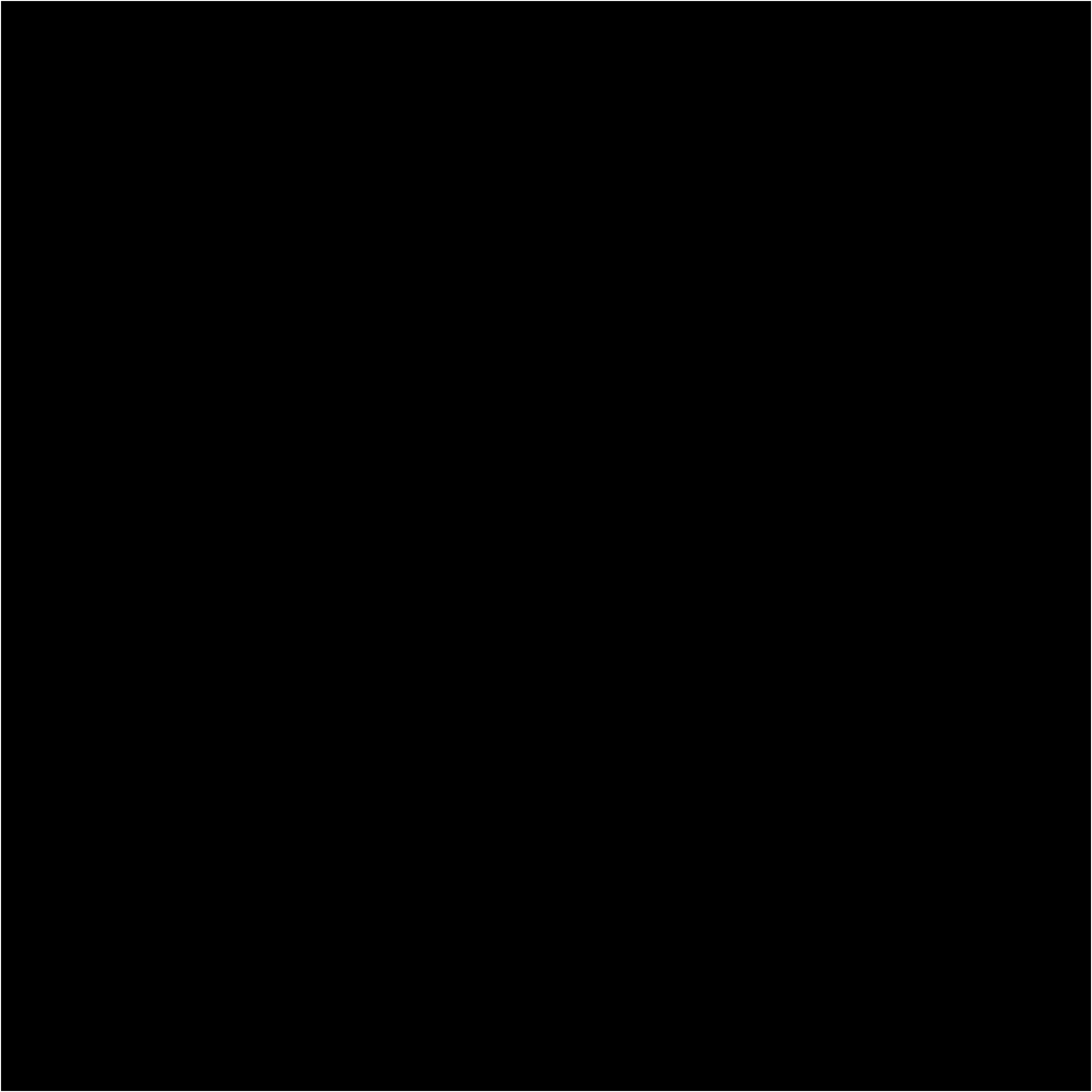
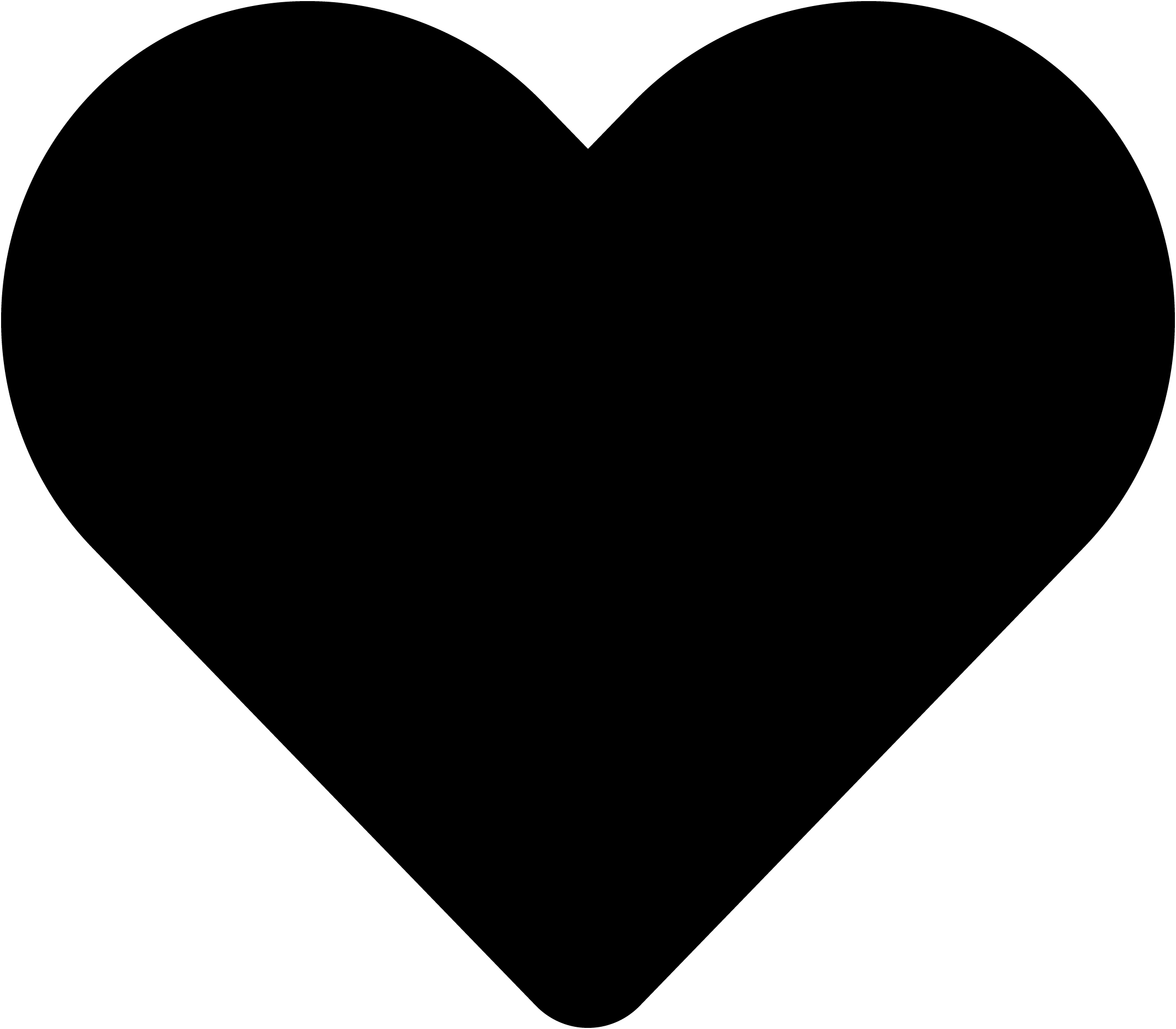
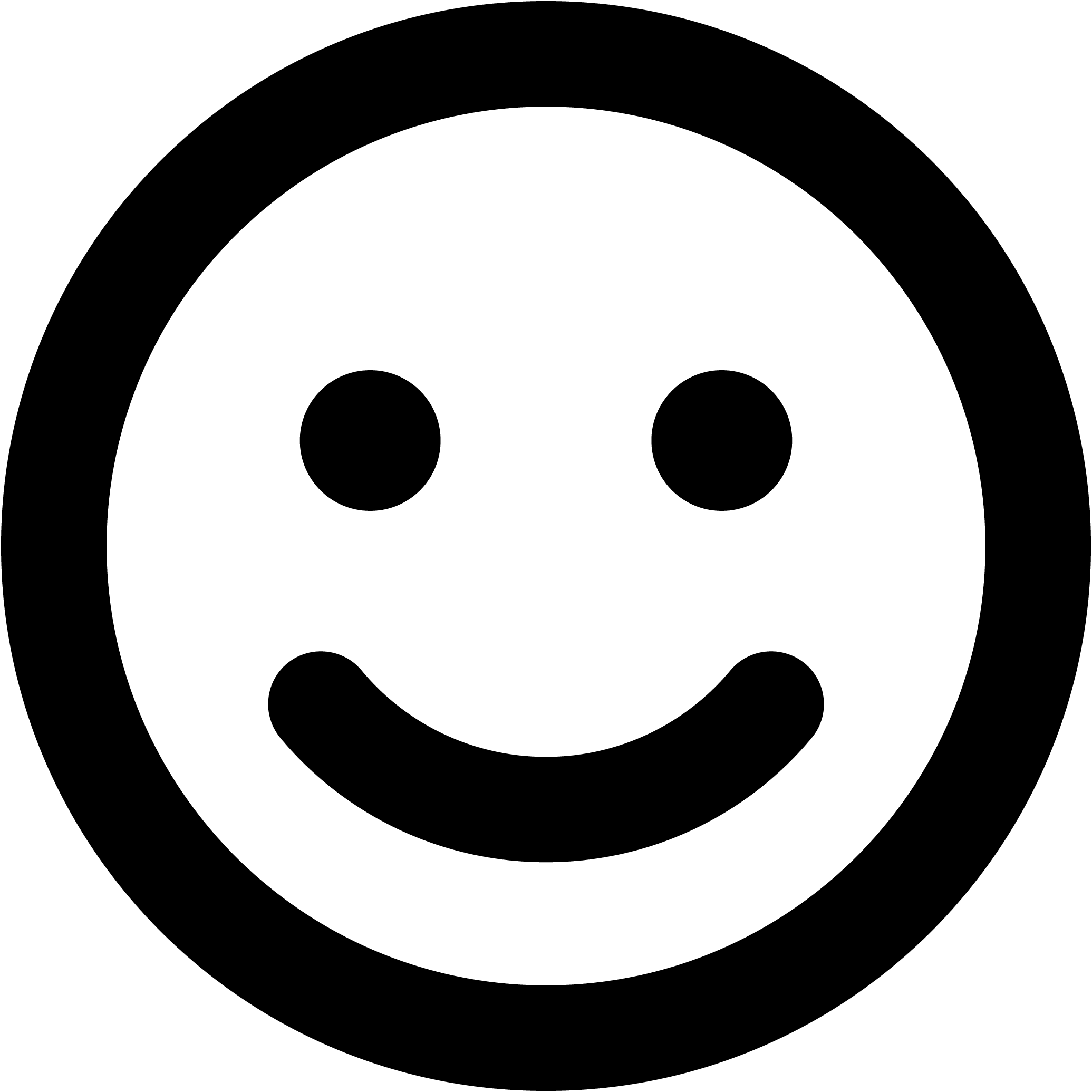
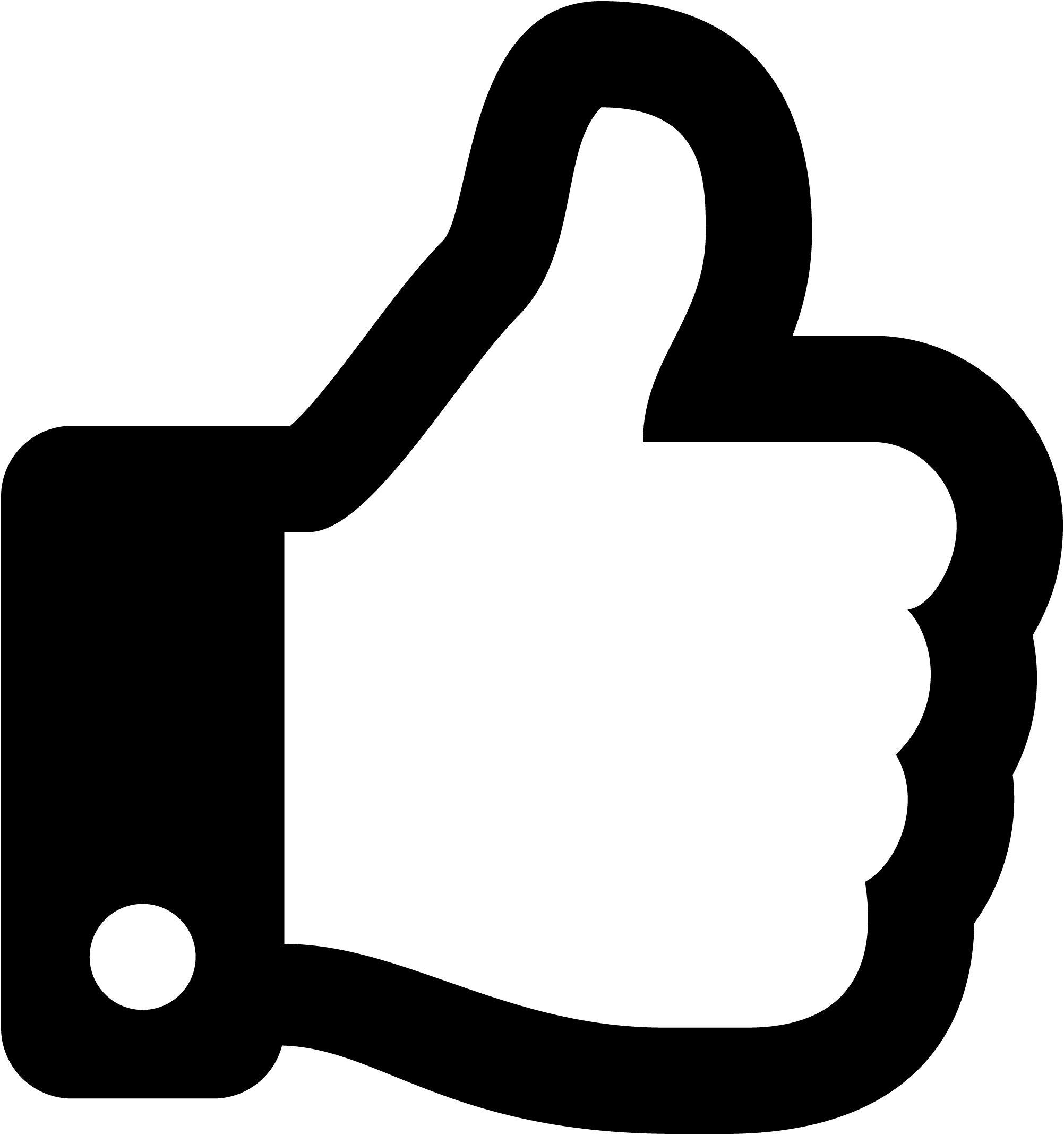
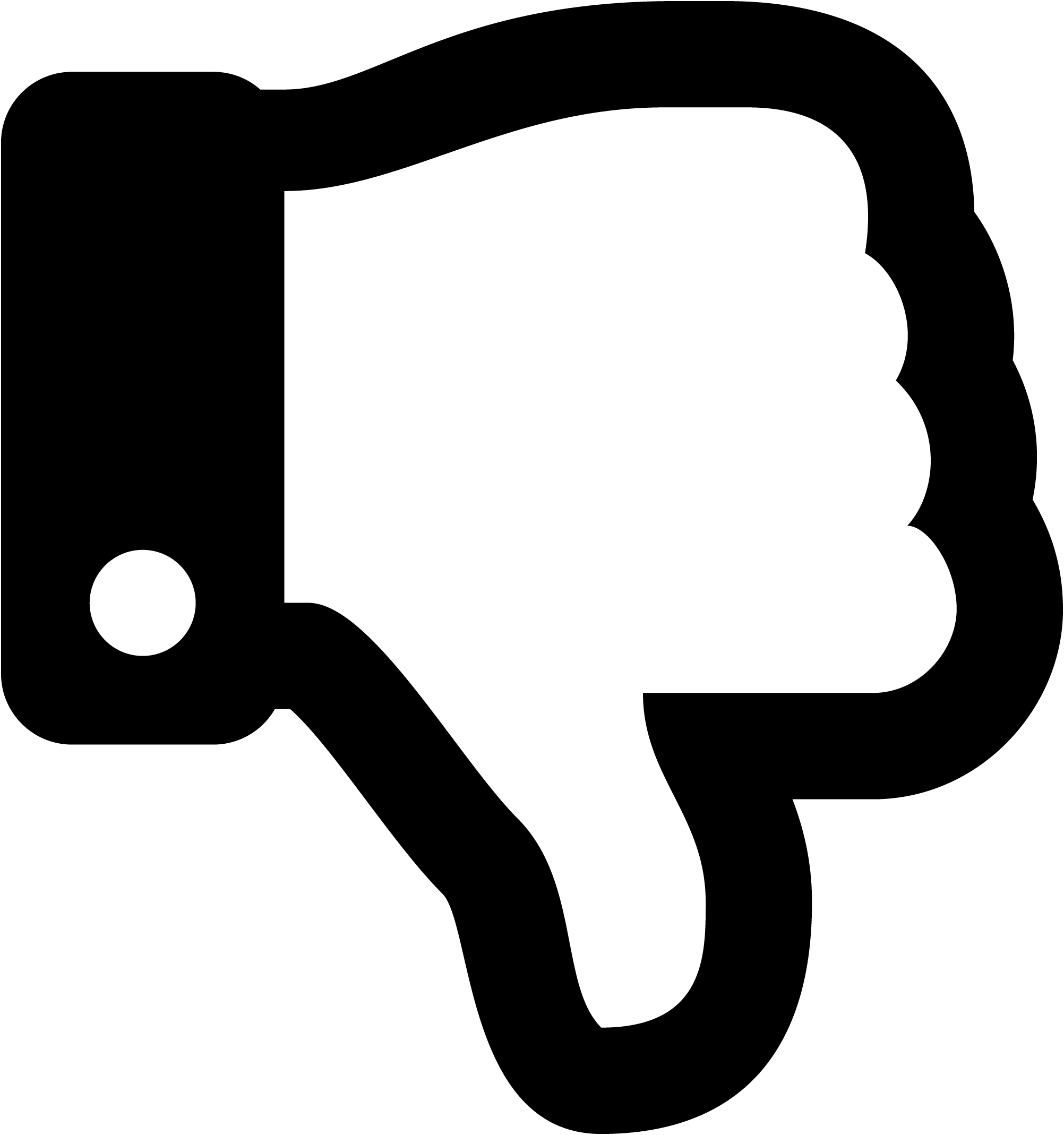
Border colour
Rotate
Skew (x-axis)
Skew (y-axis)
Image (max size: 2mb)
Or drag a symbol into the upload area
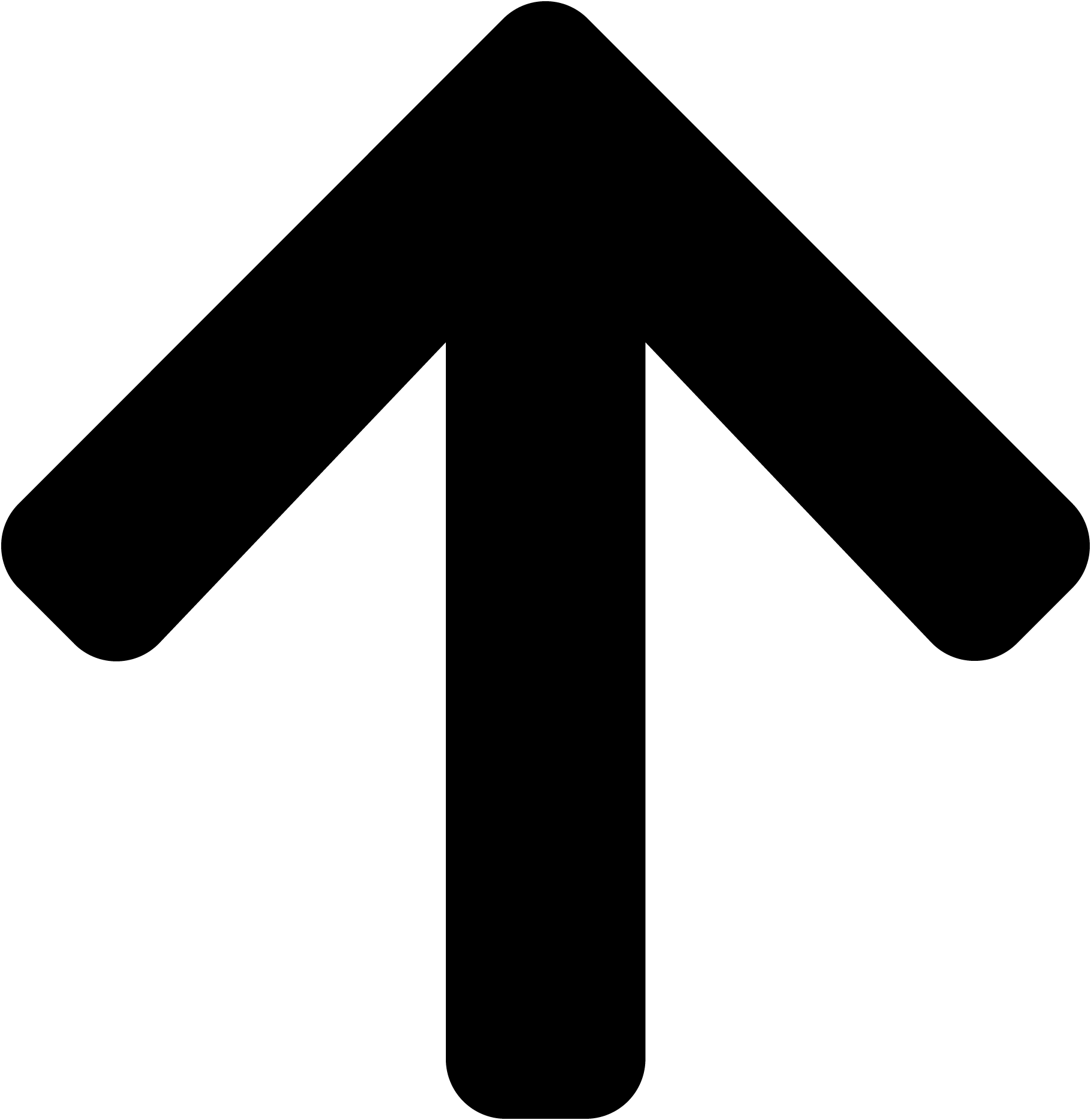
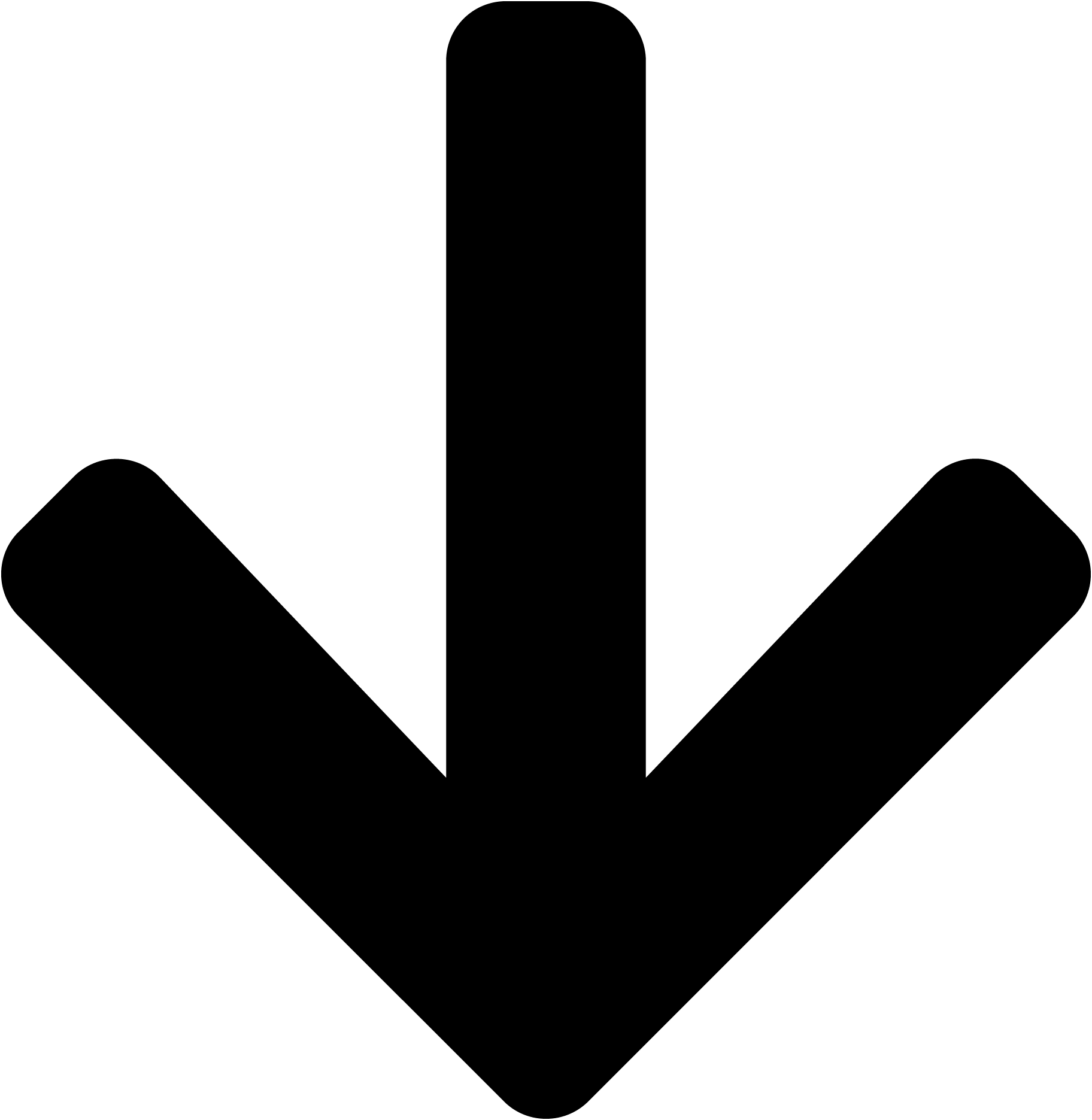
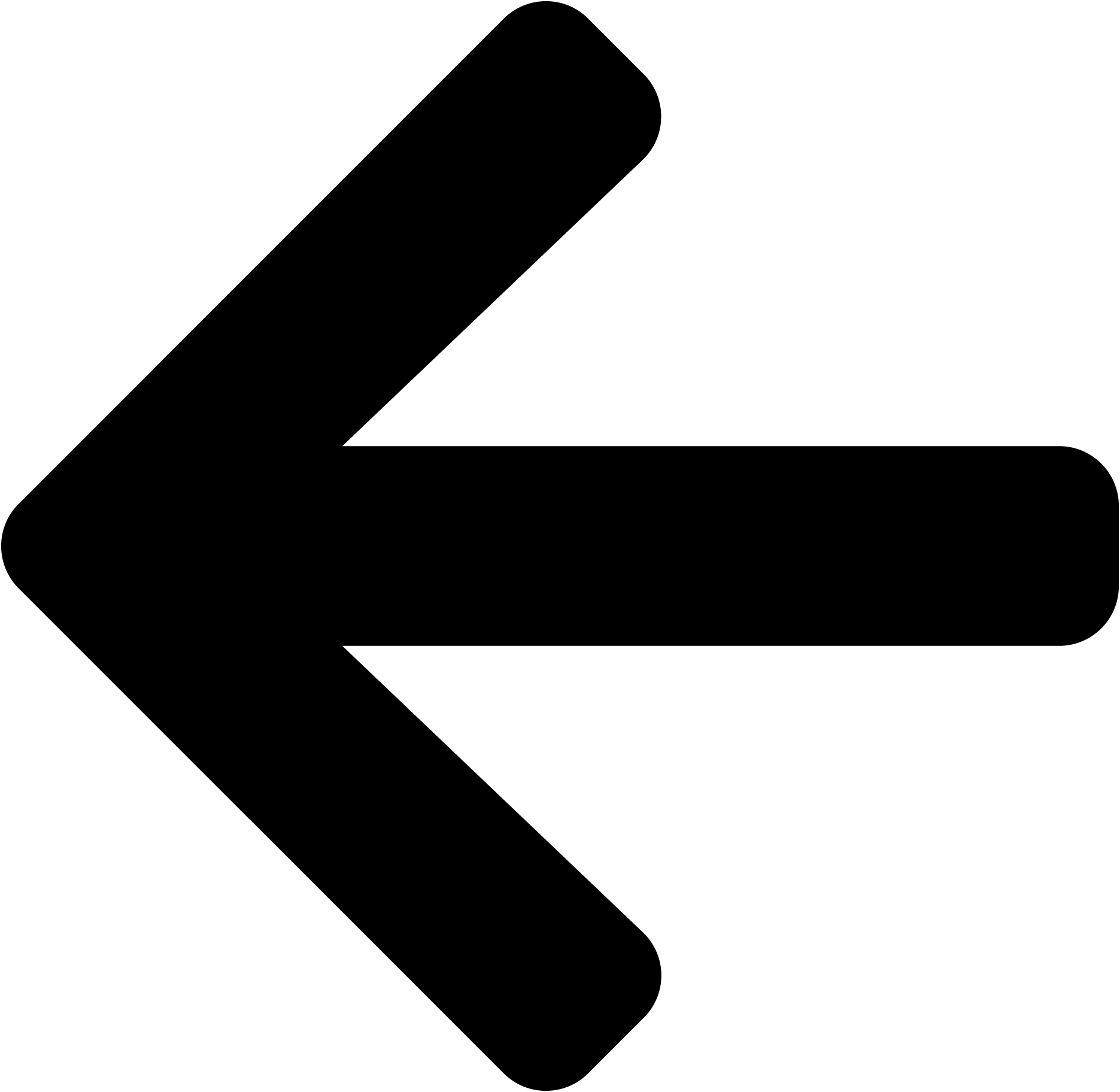
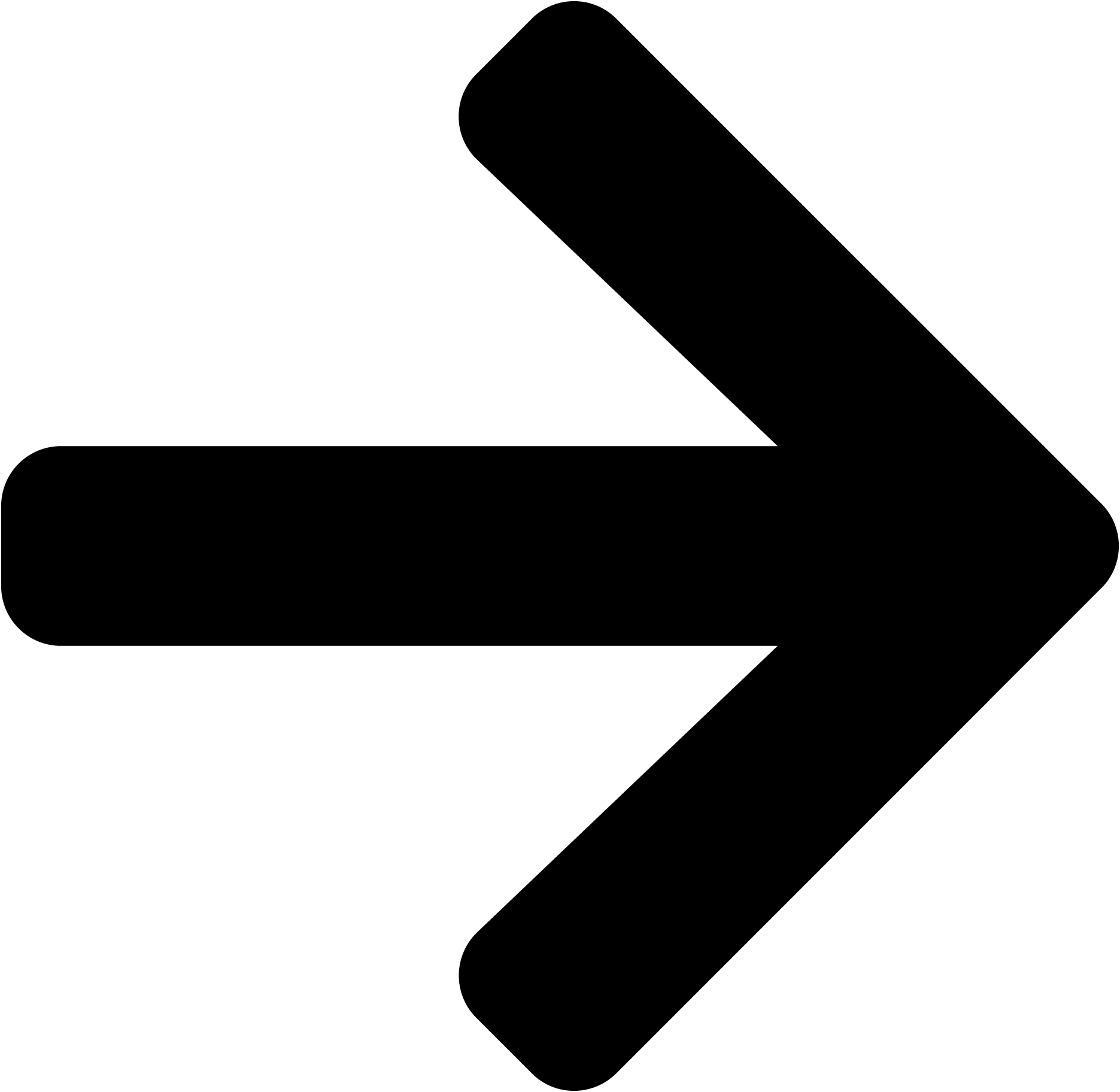
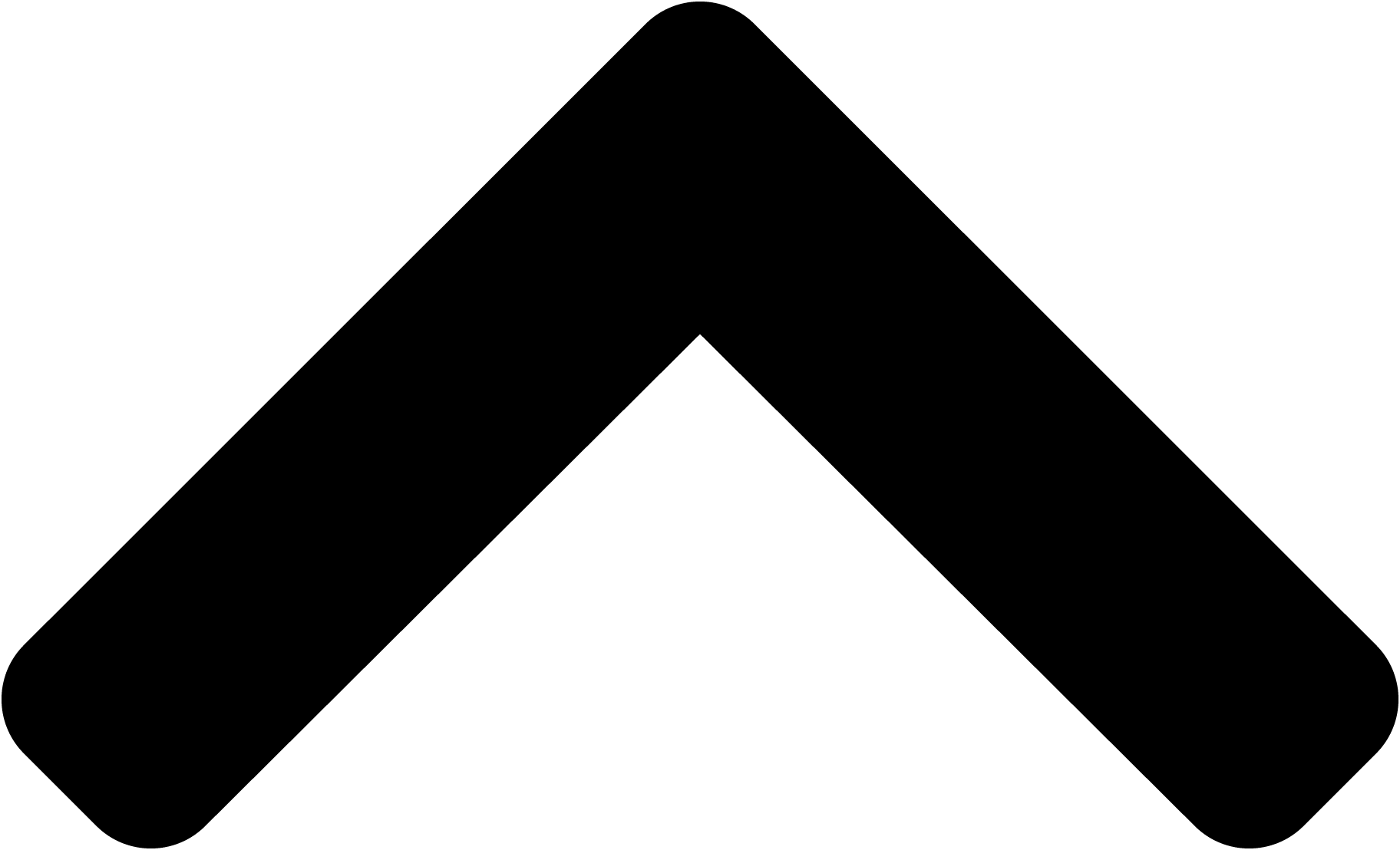
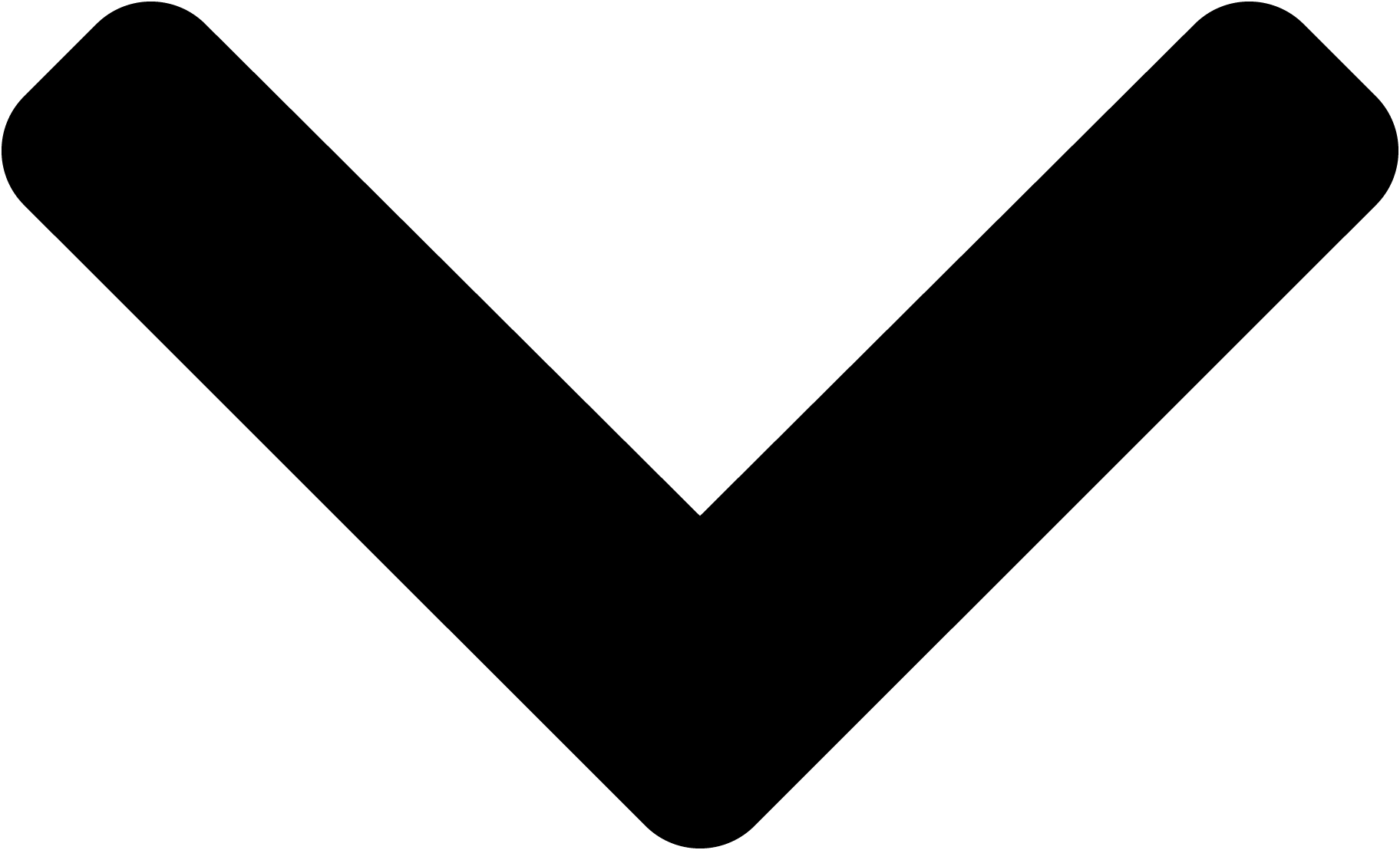
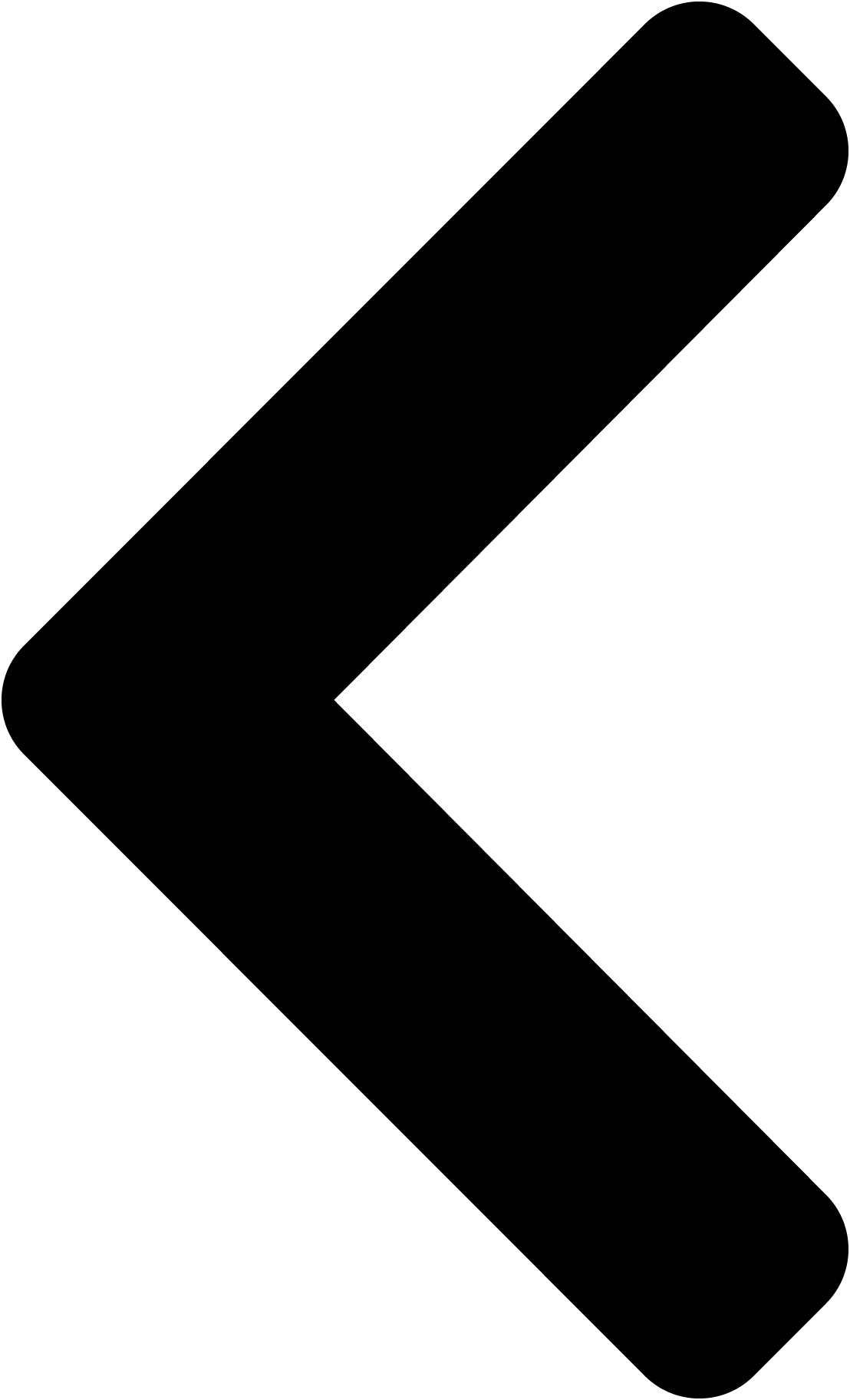
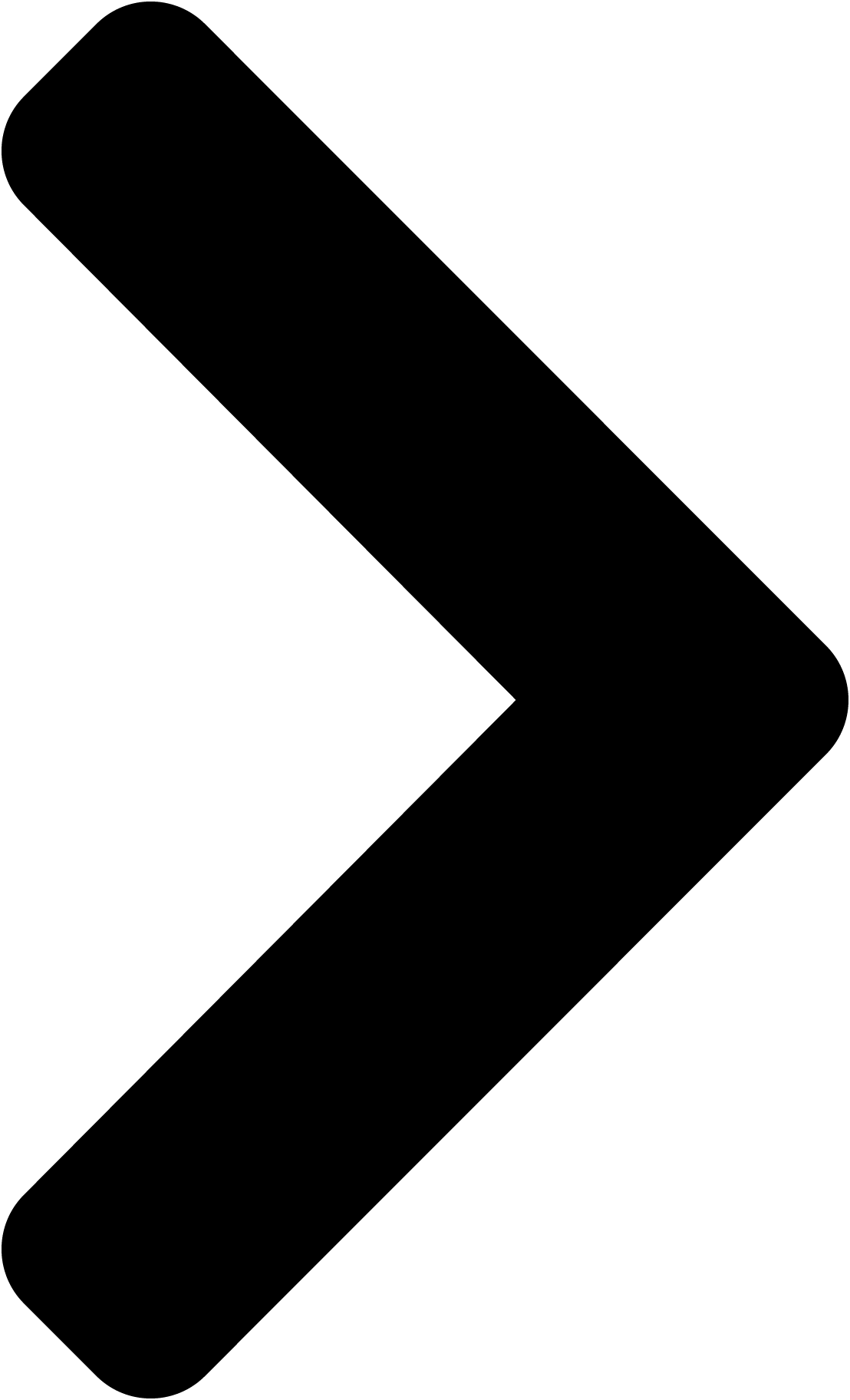
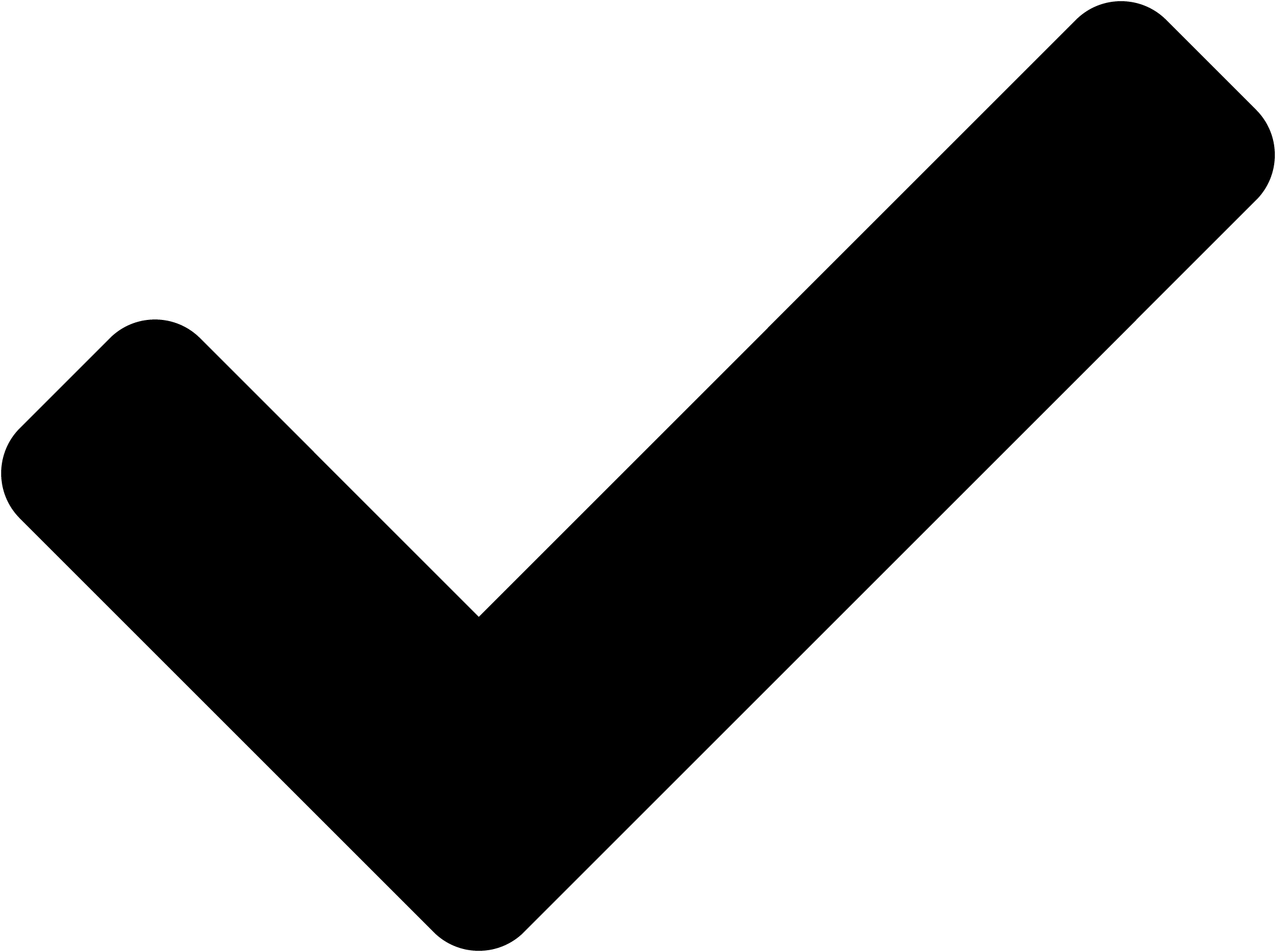
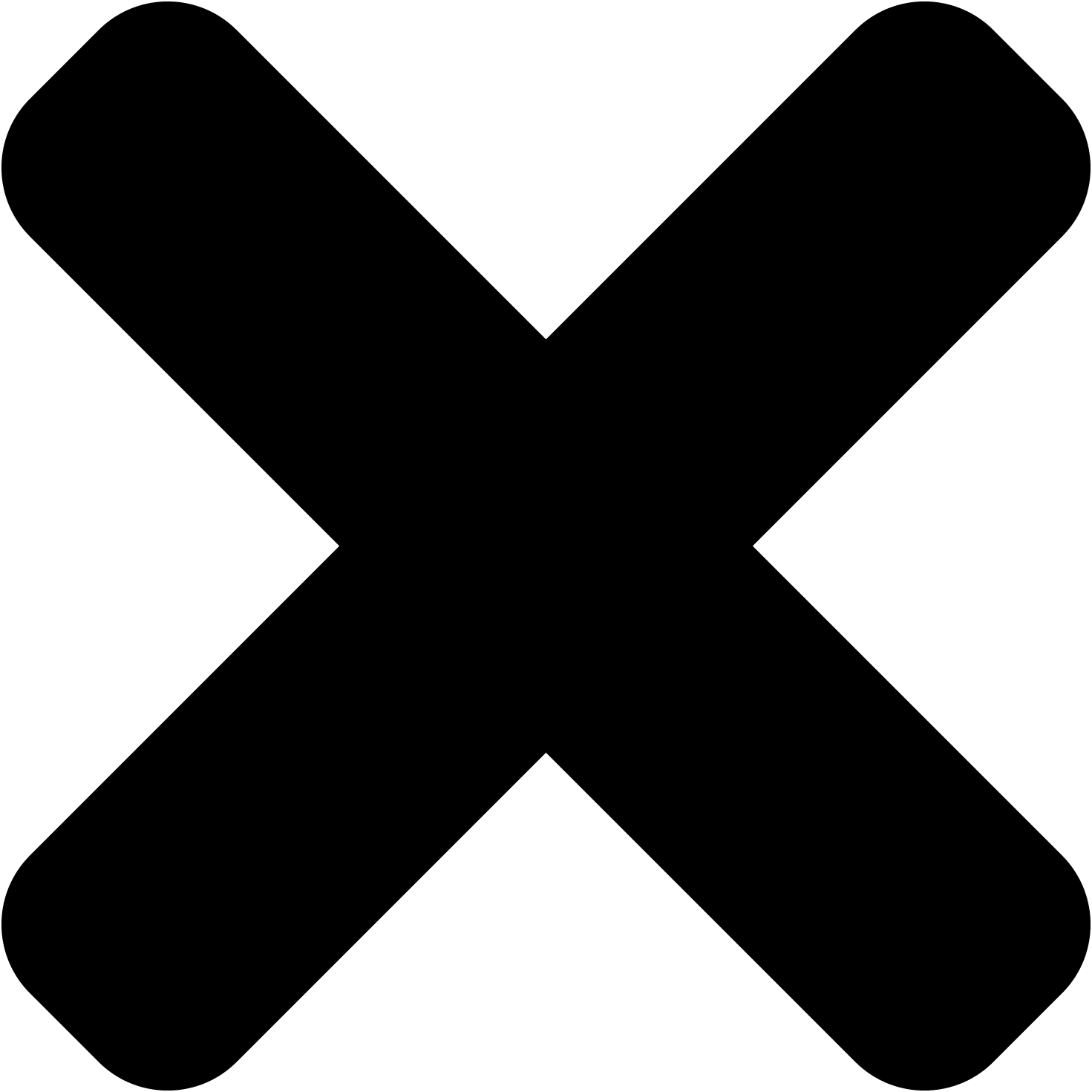
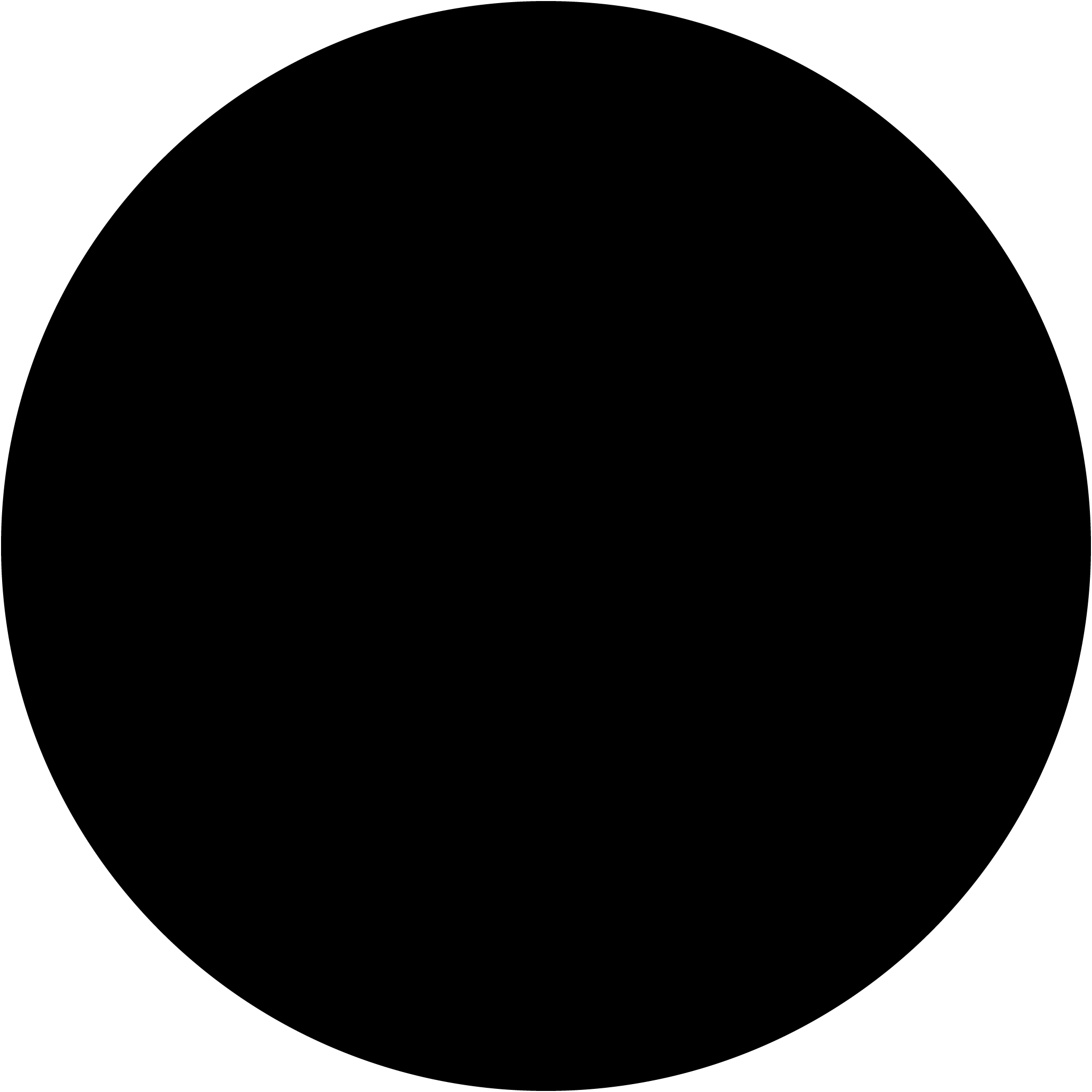
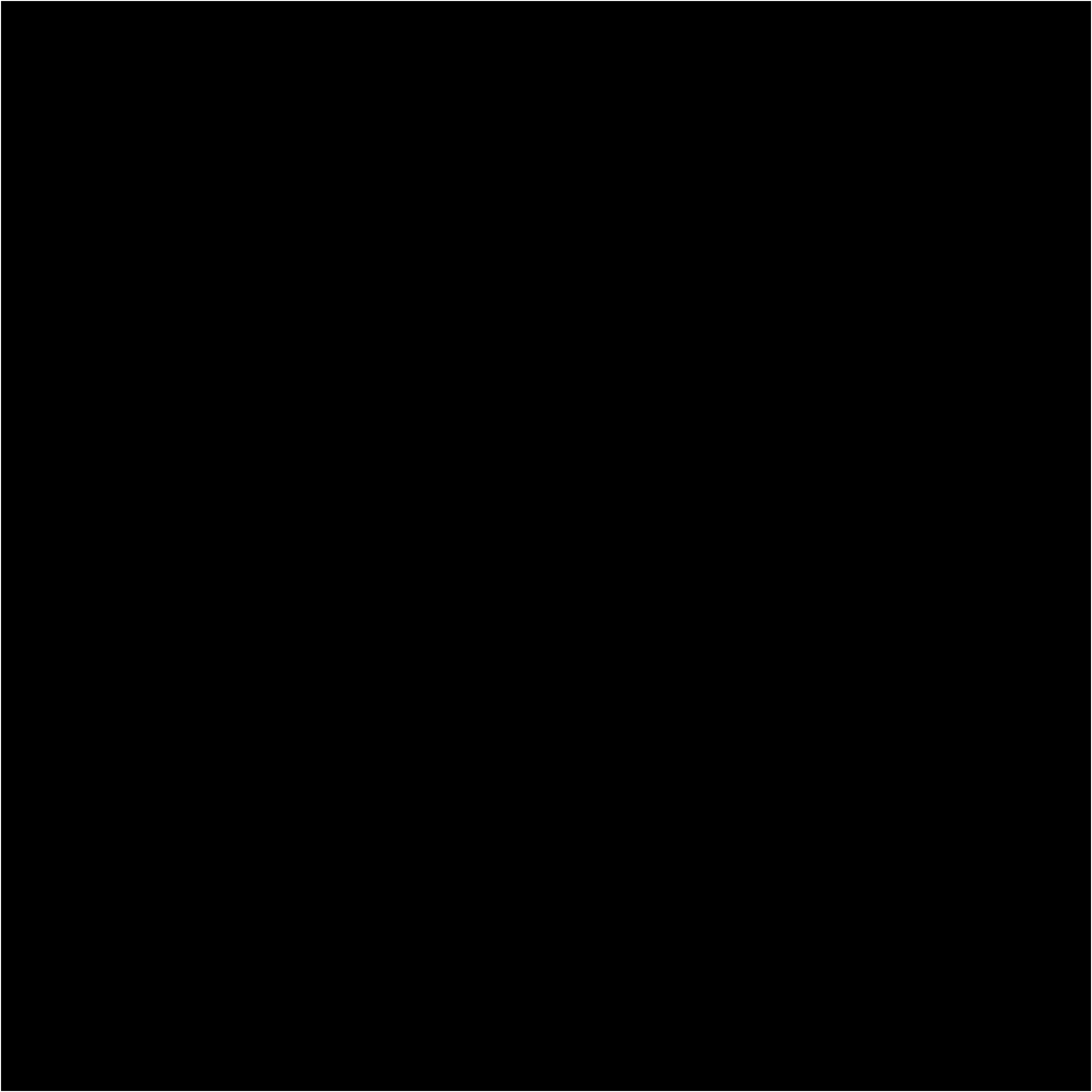
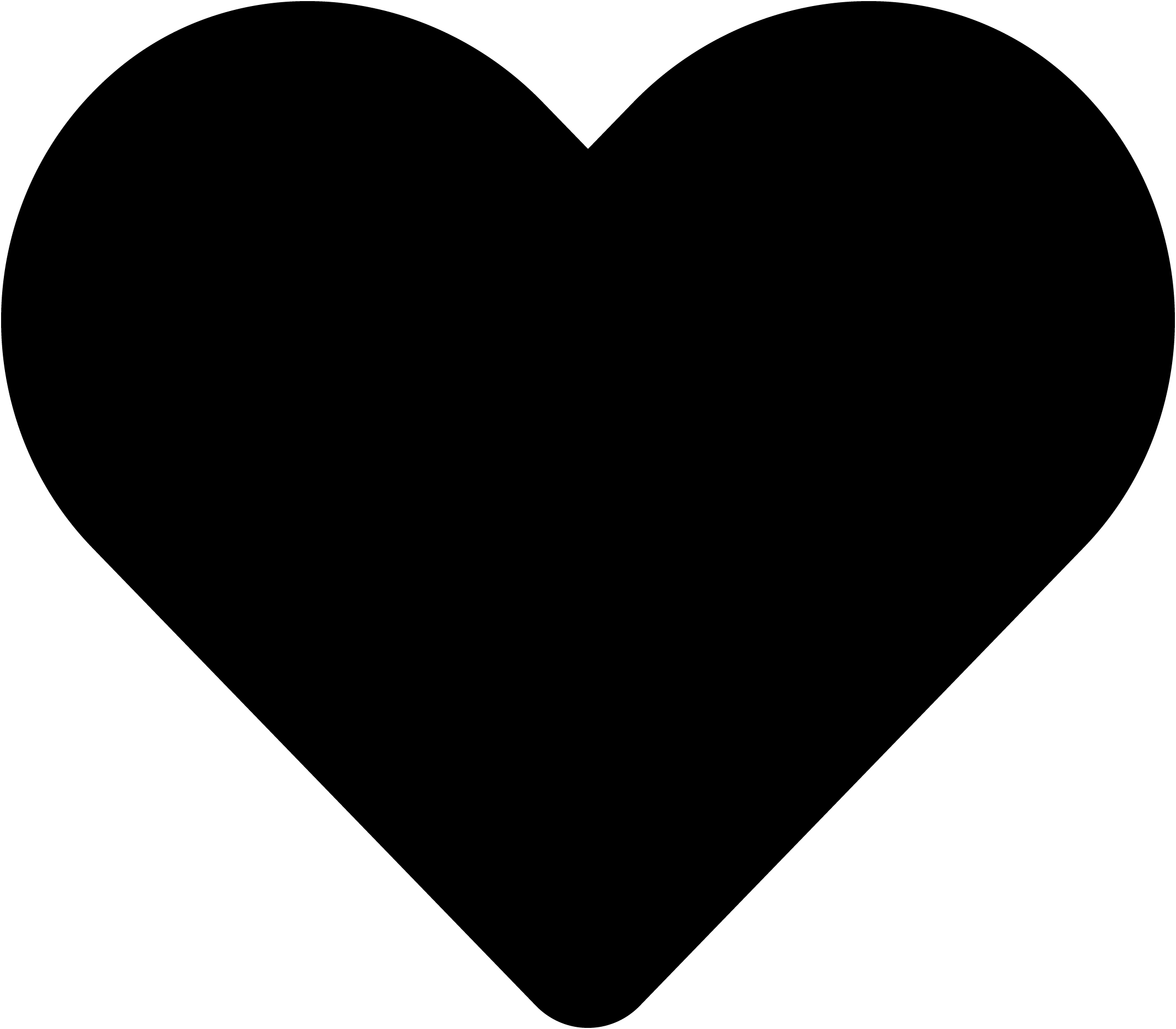
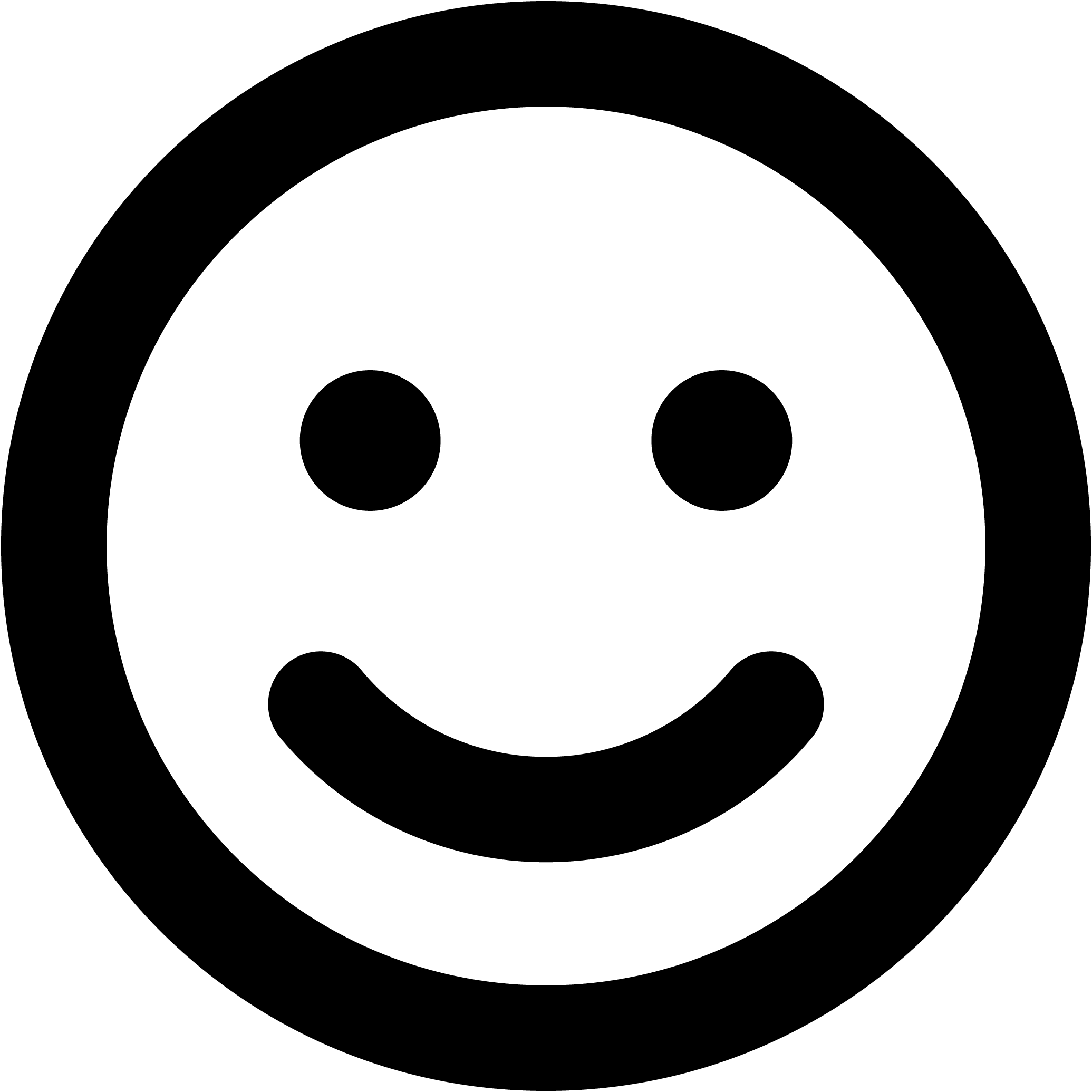
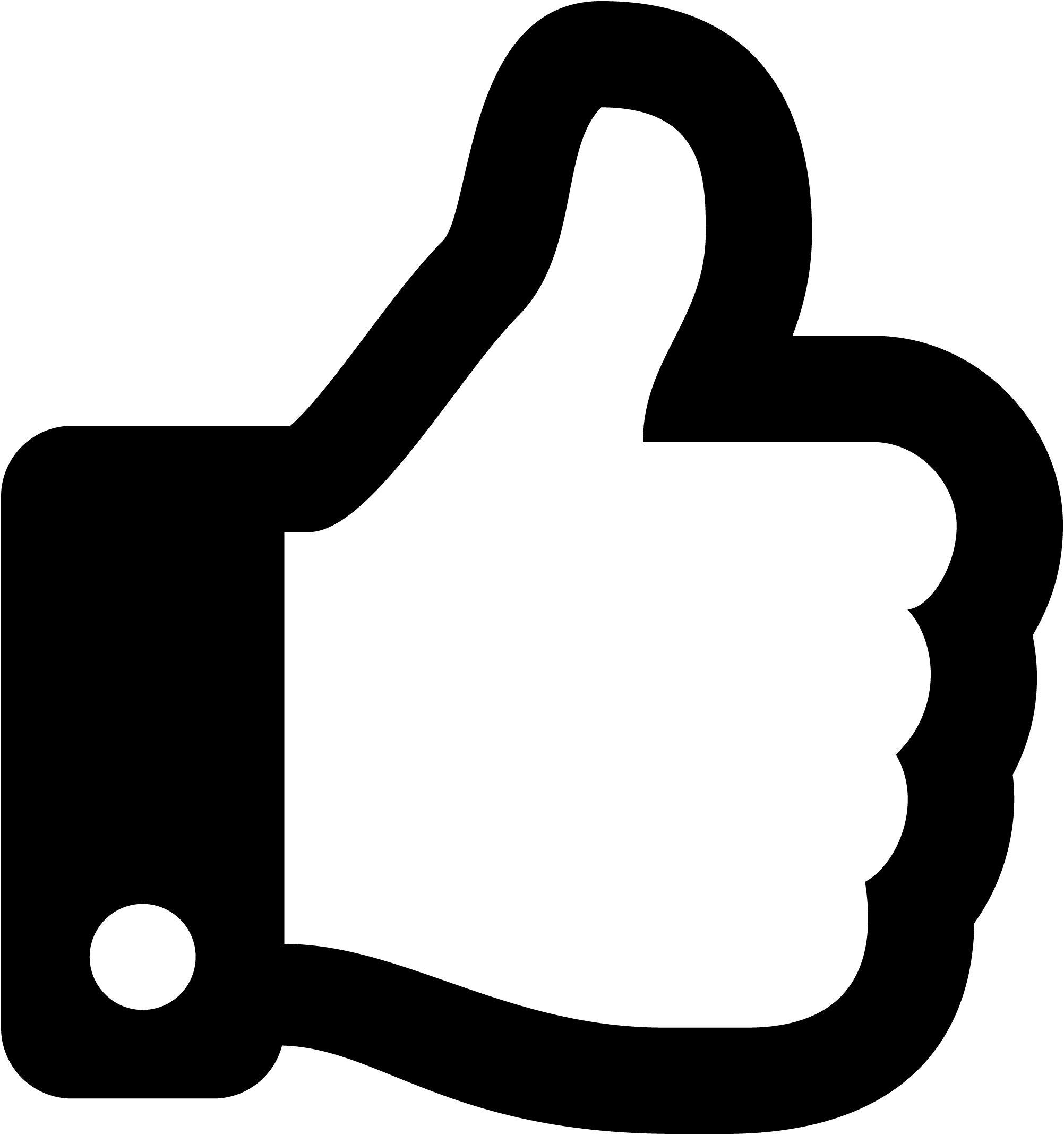
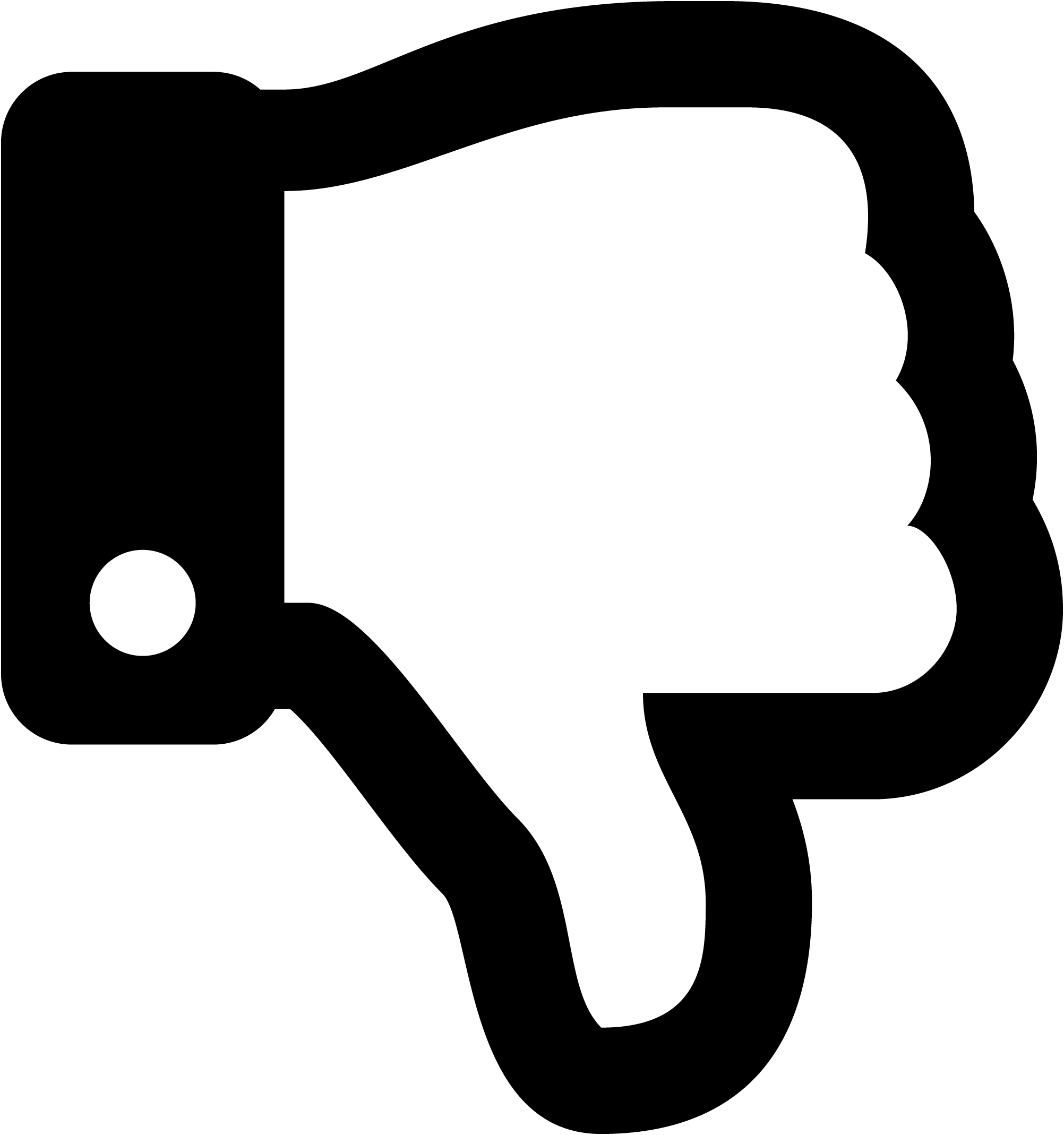
Image description/alt-tag
Image caption
Image link
Rollover Image (max size: 2mb)
Or drag a symbol into the upload area
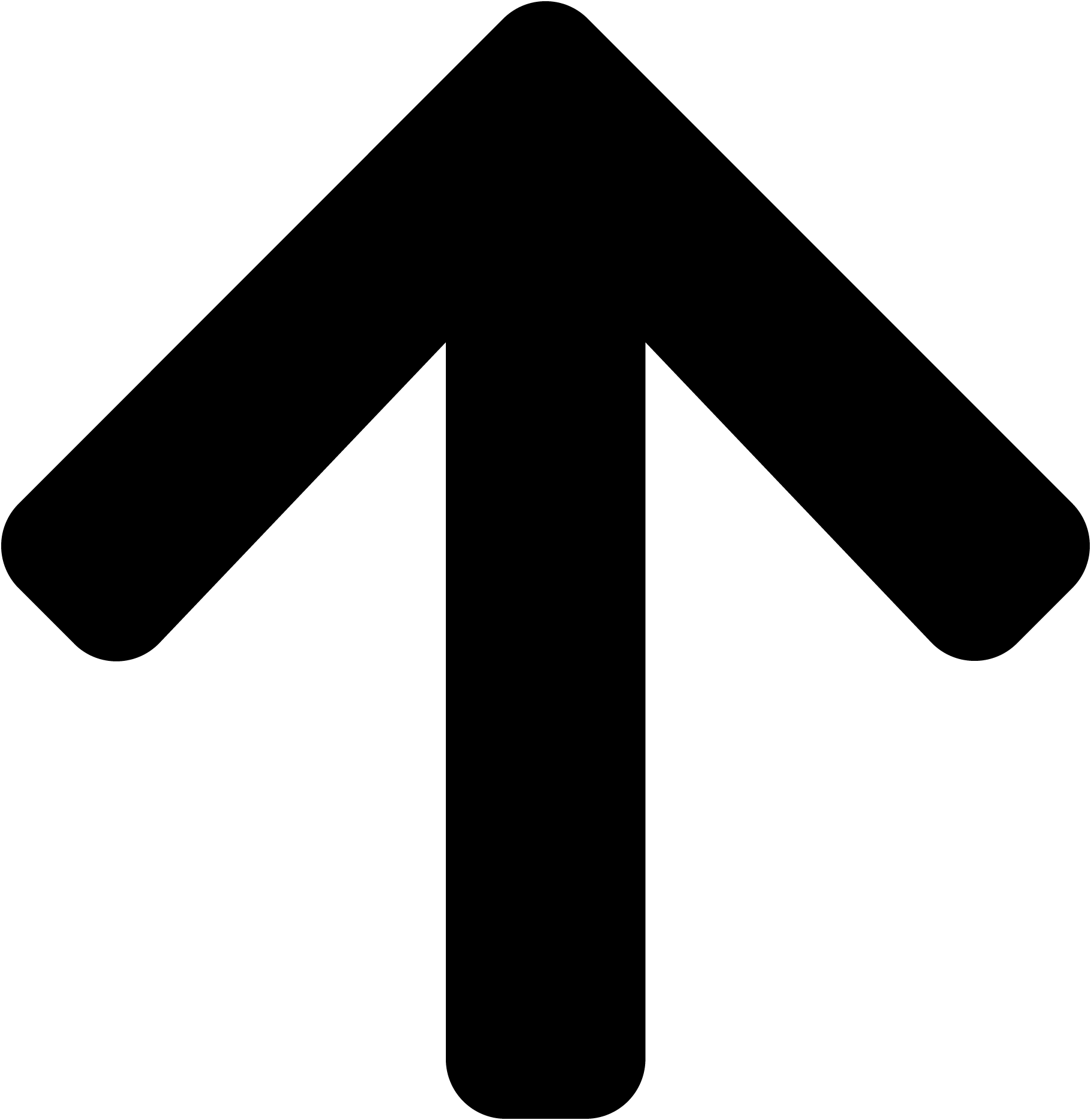
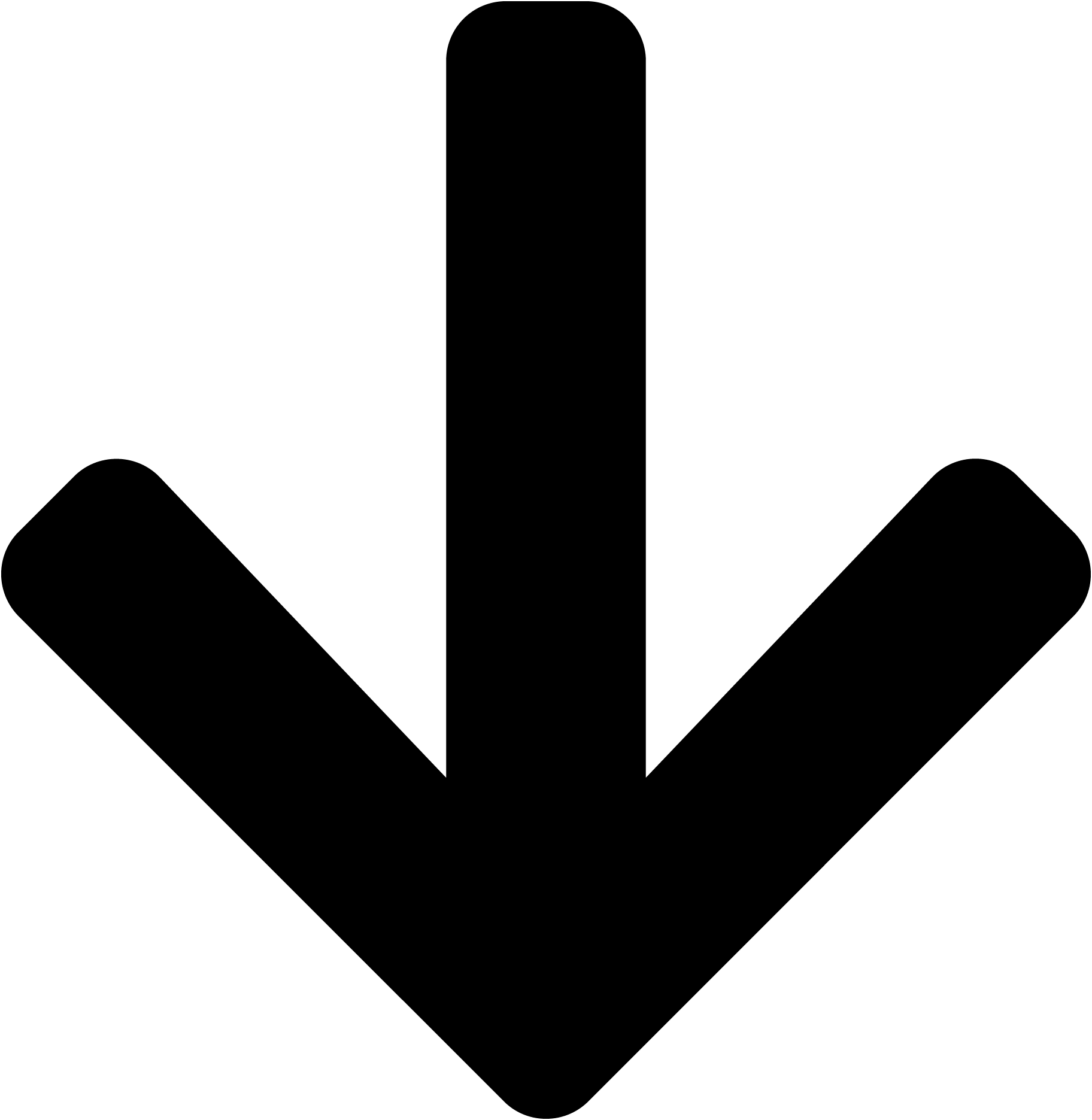
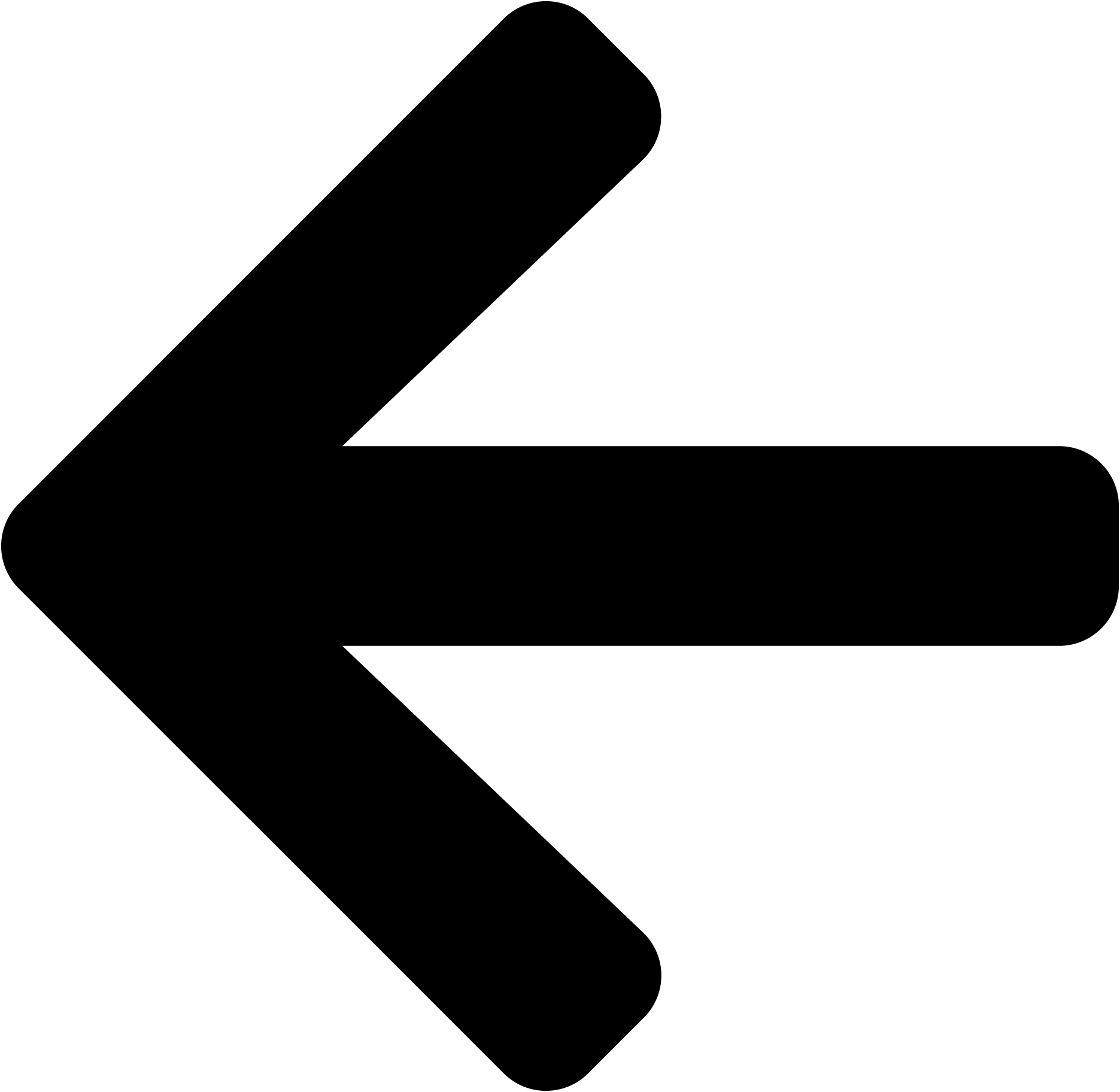
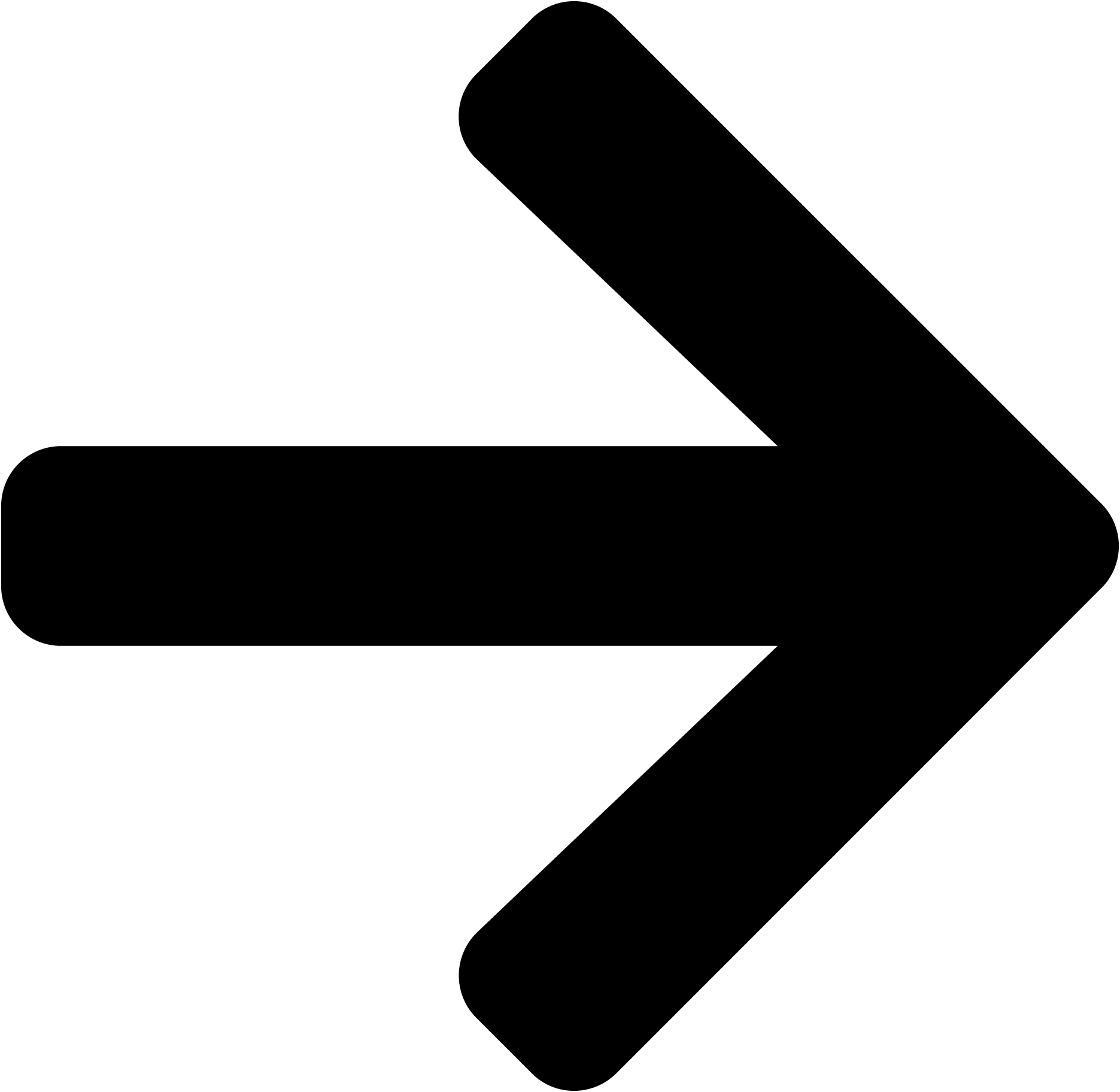
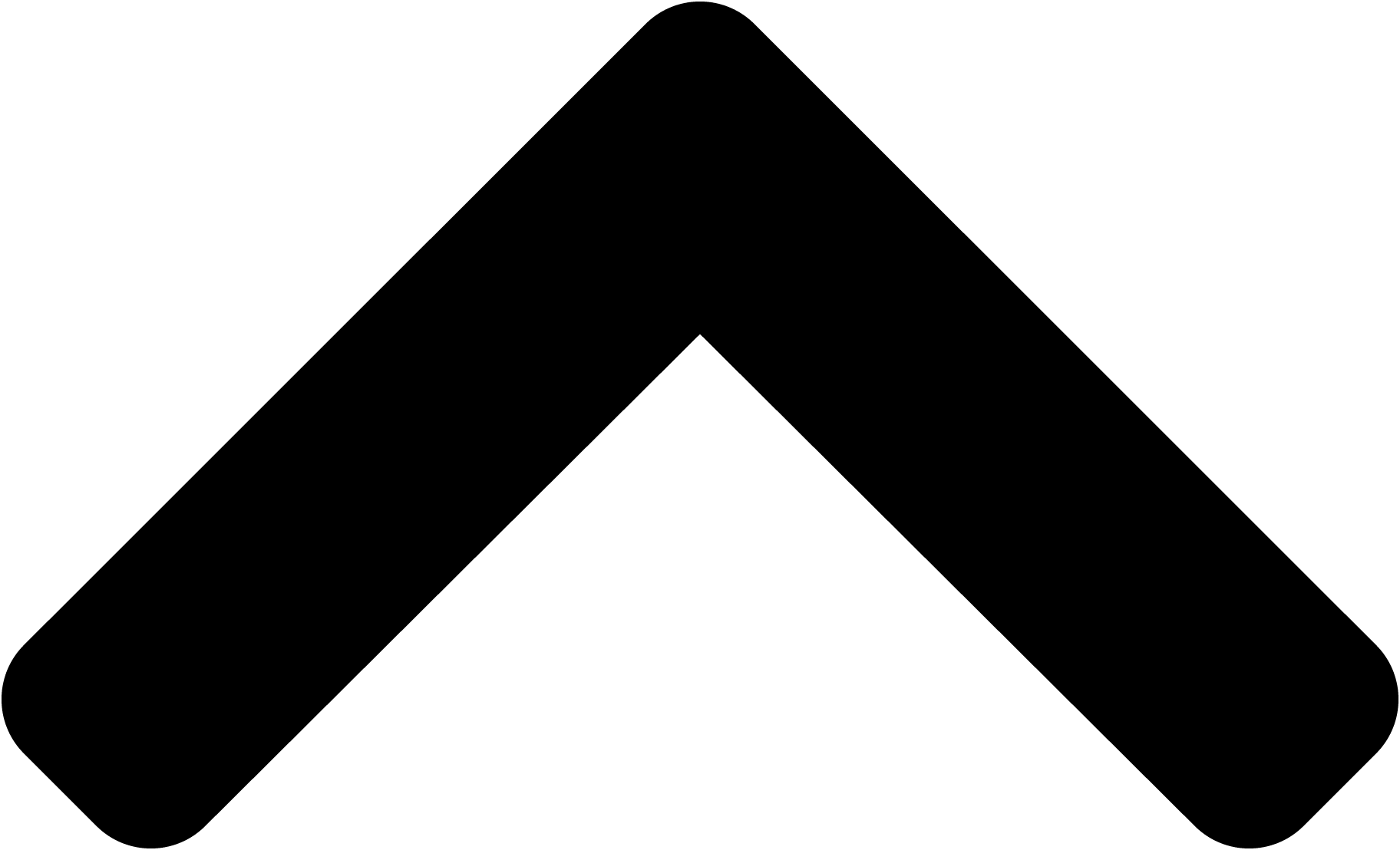
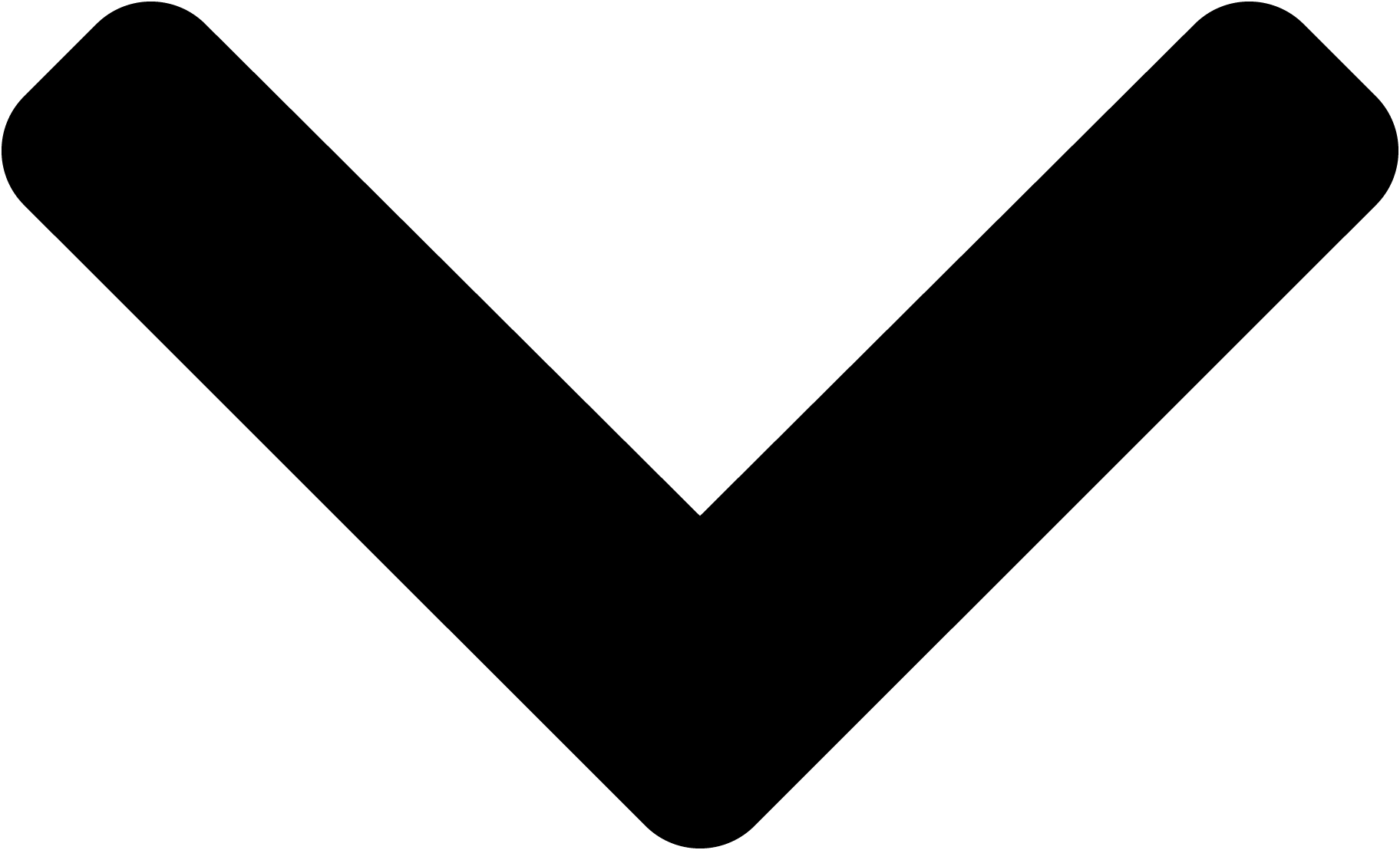
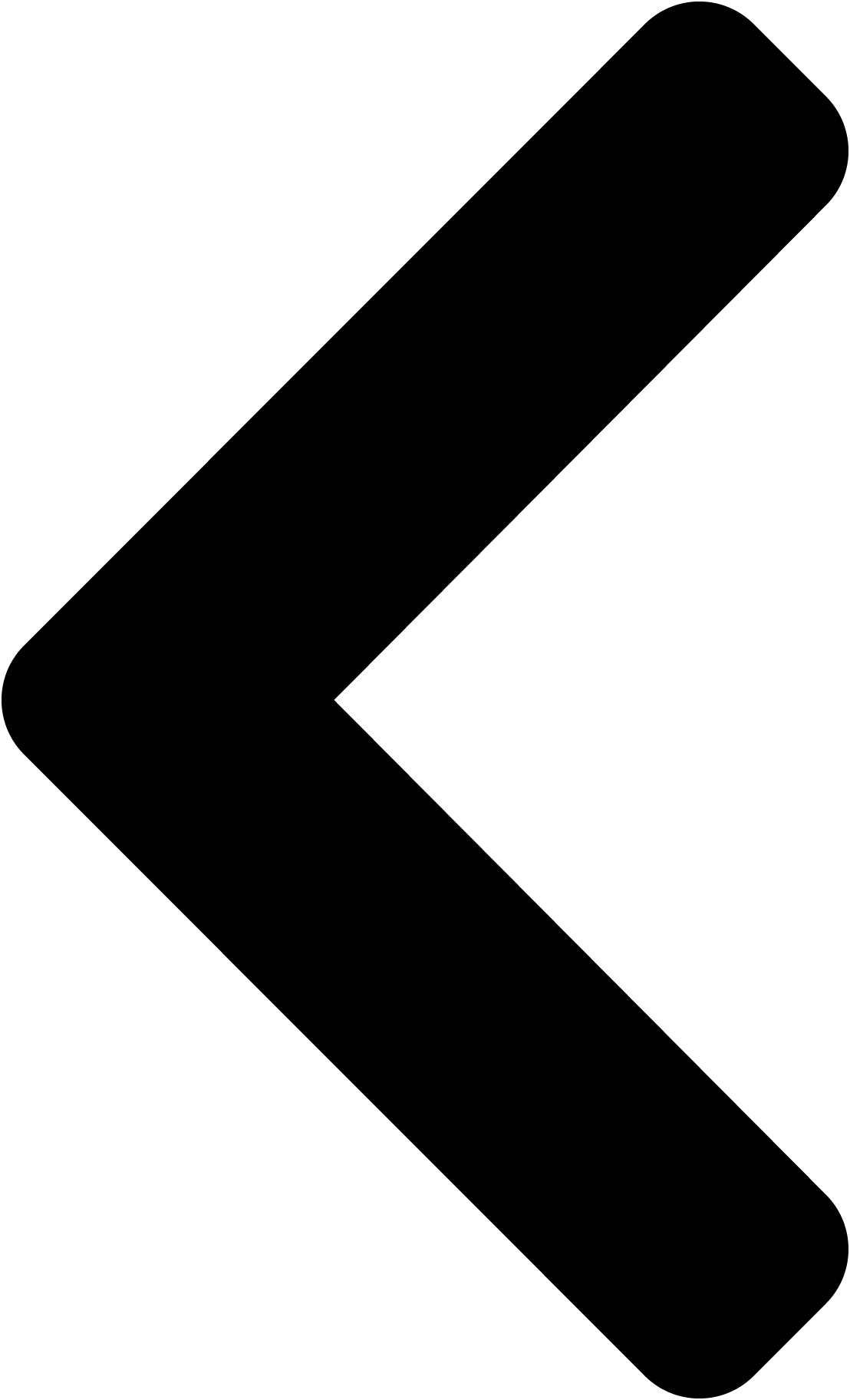
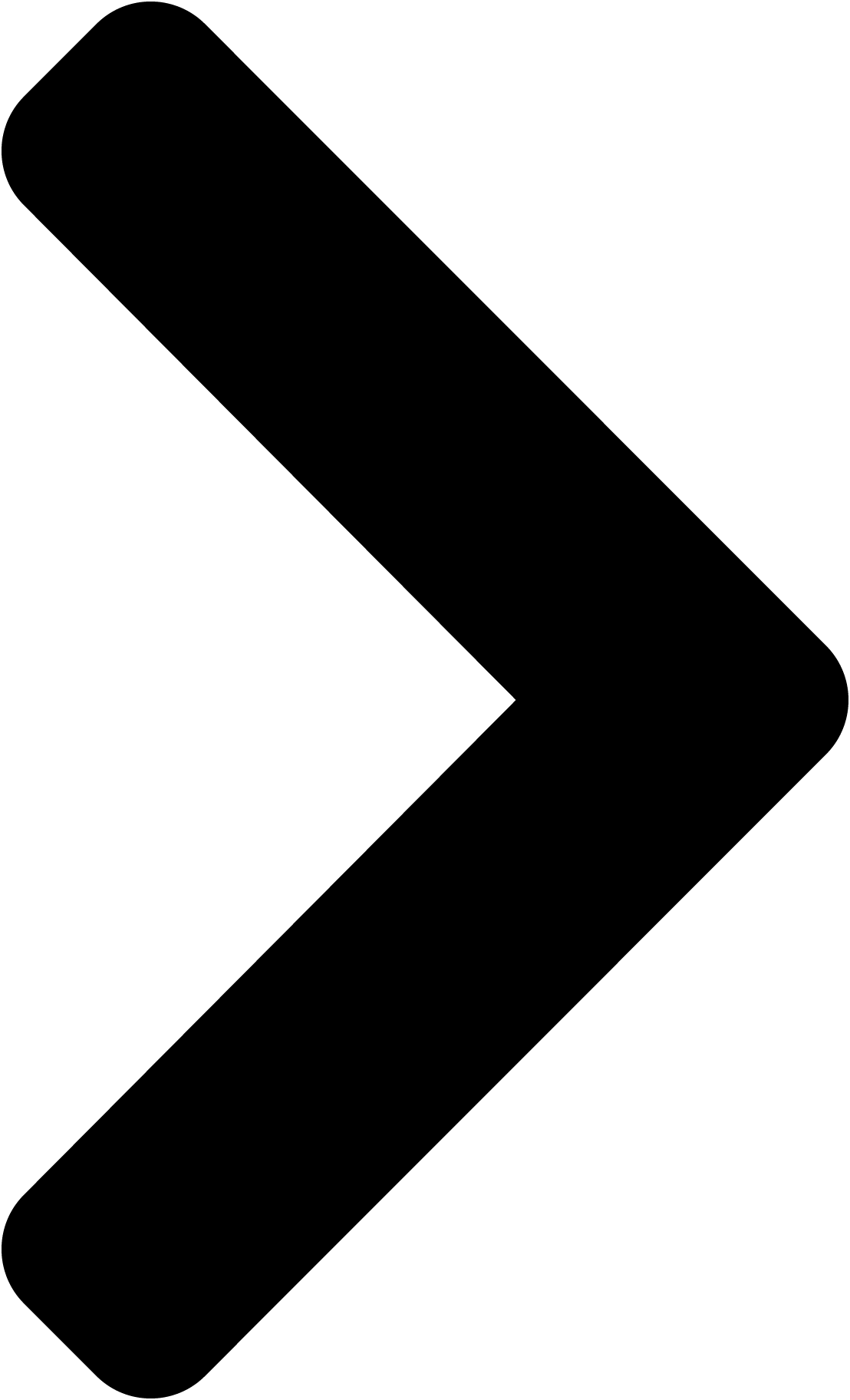
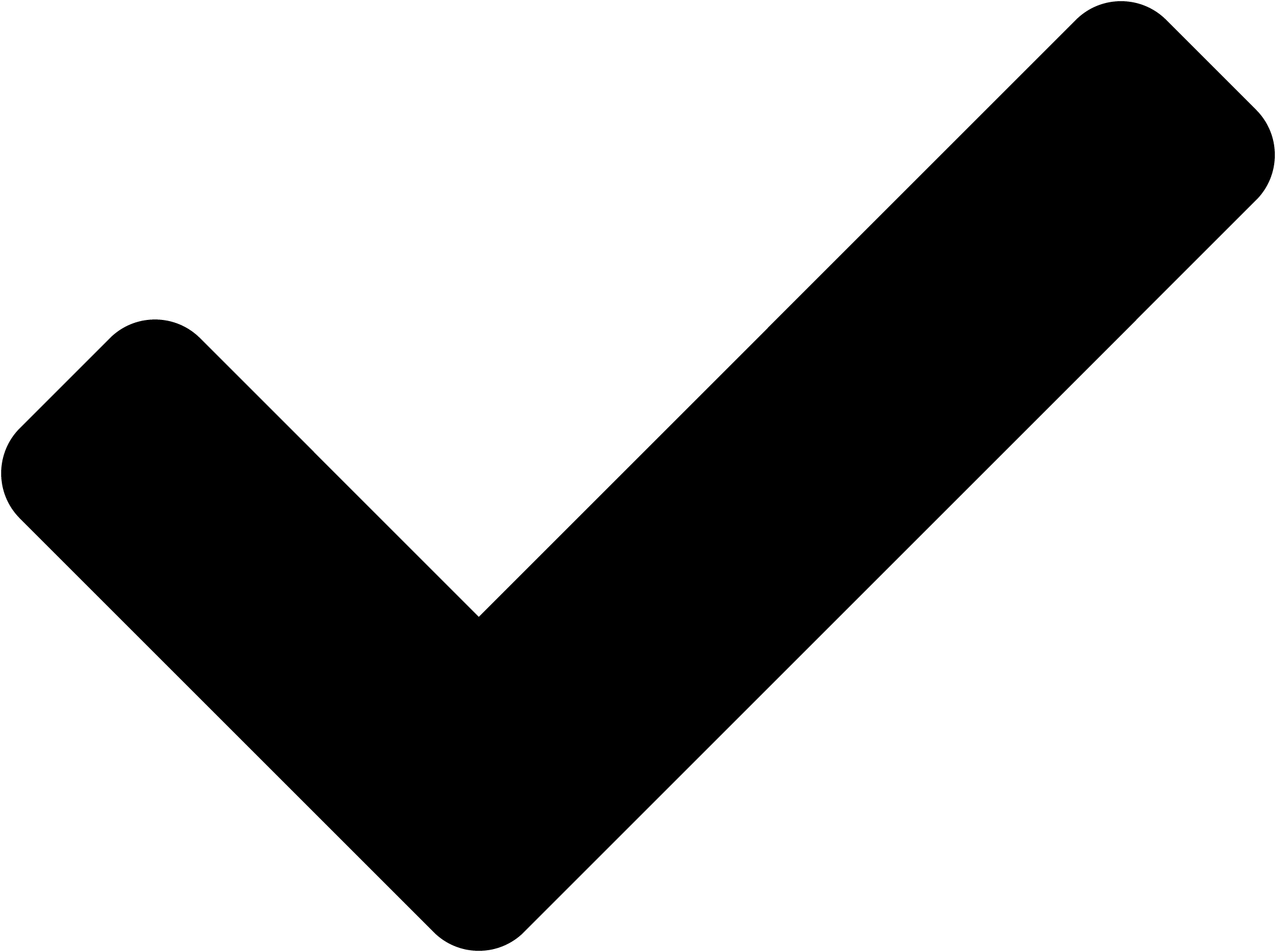
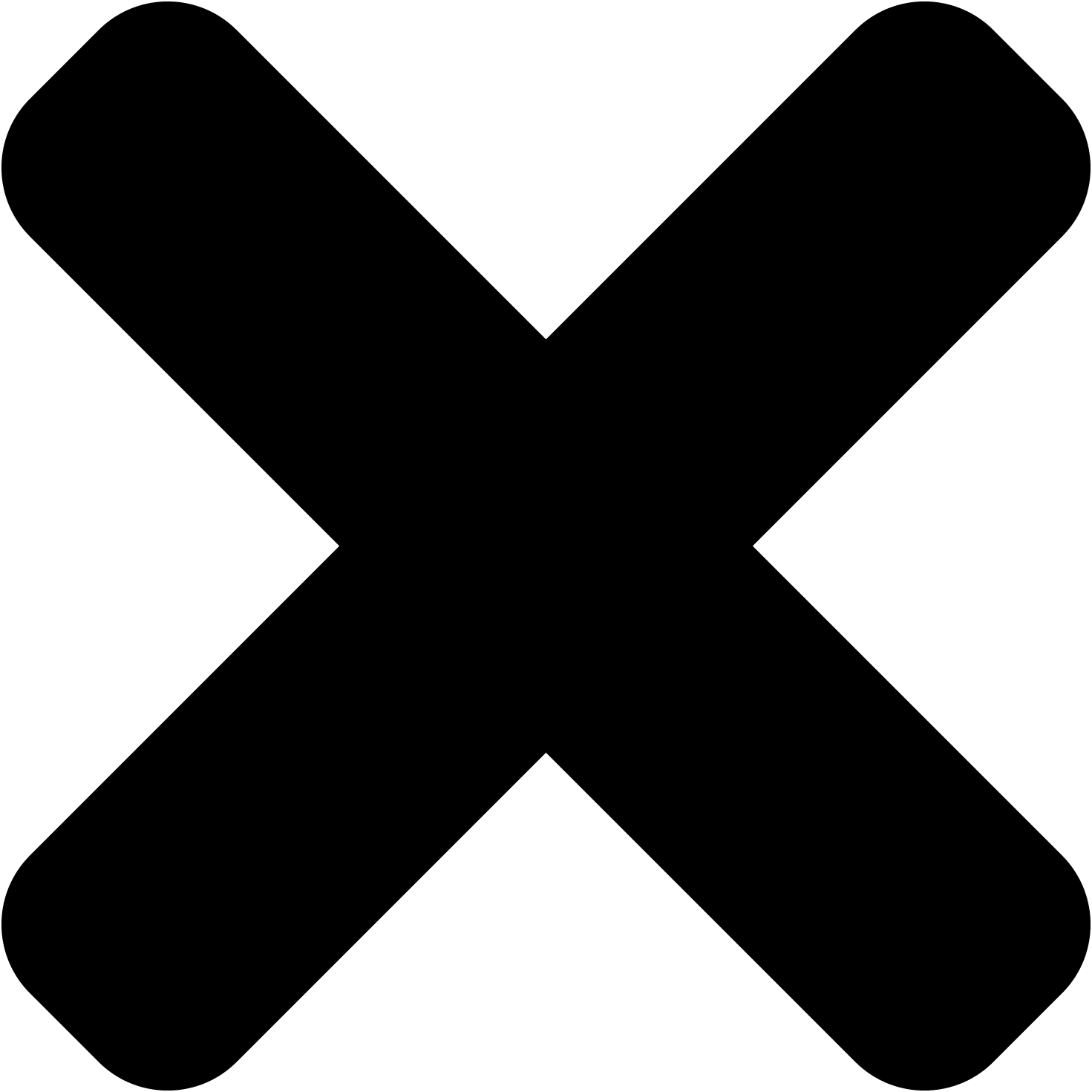
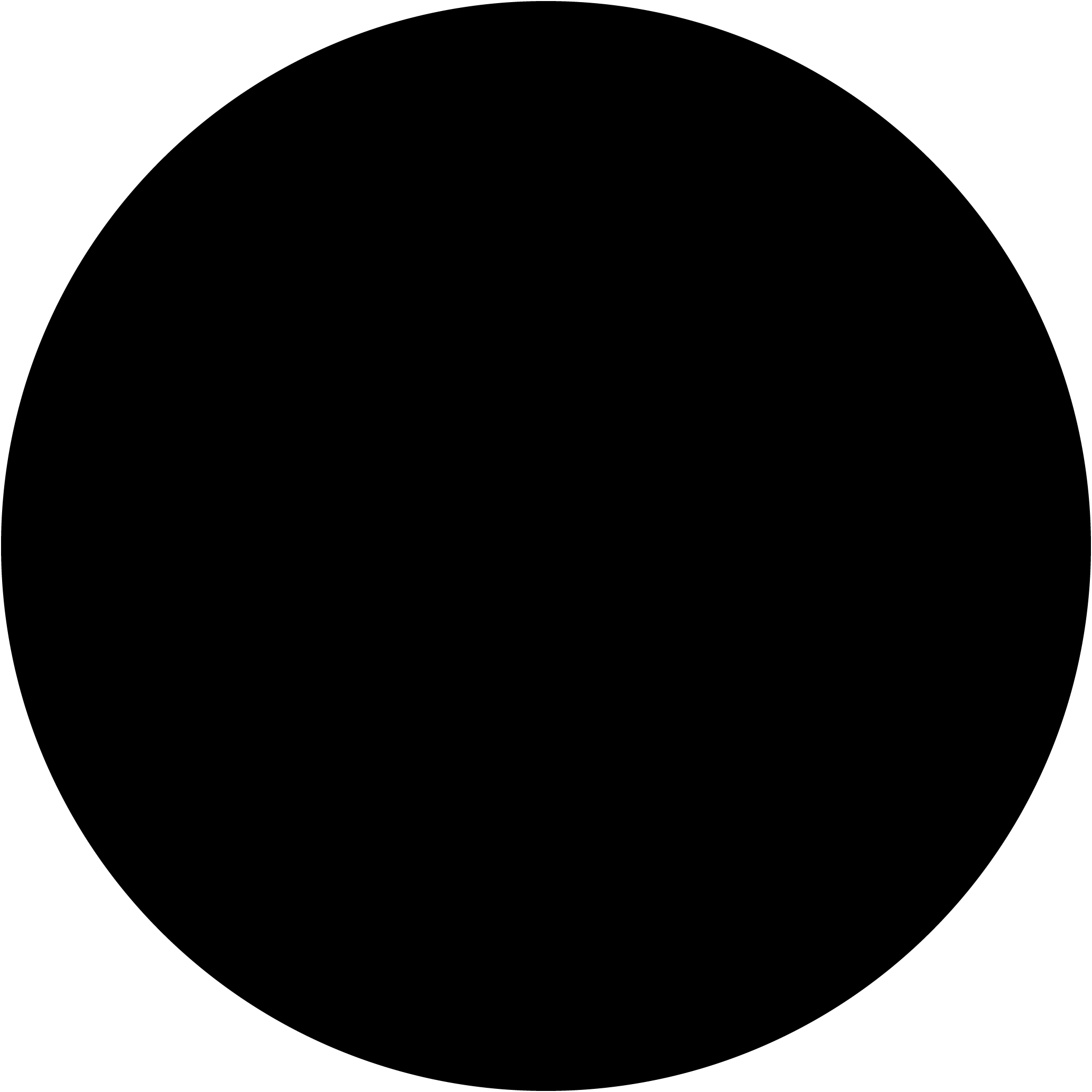
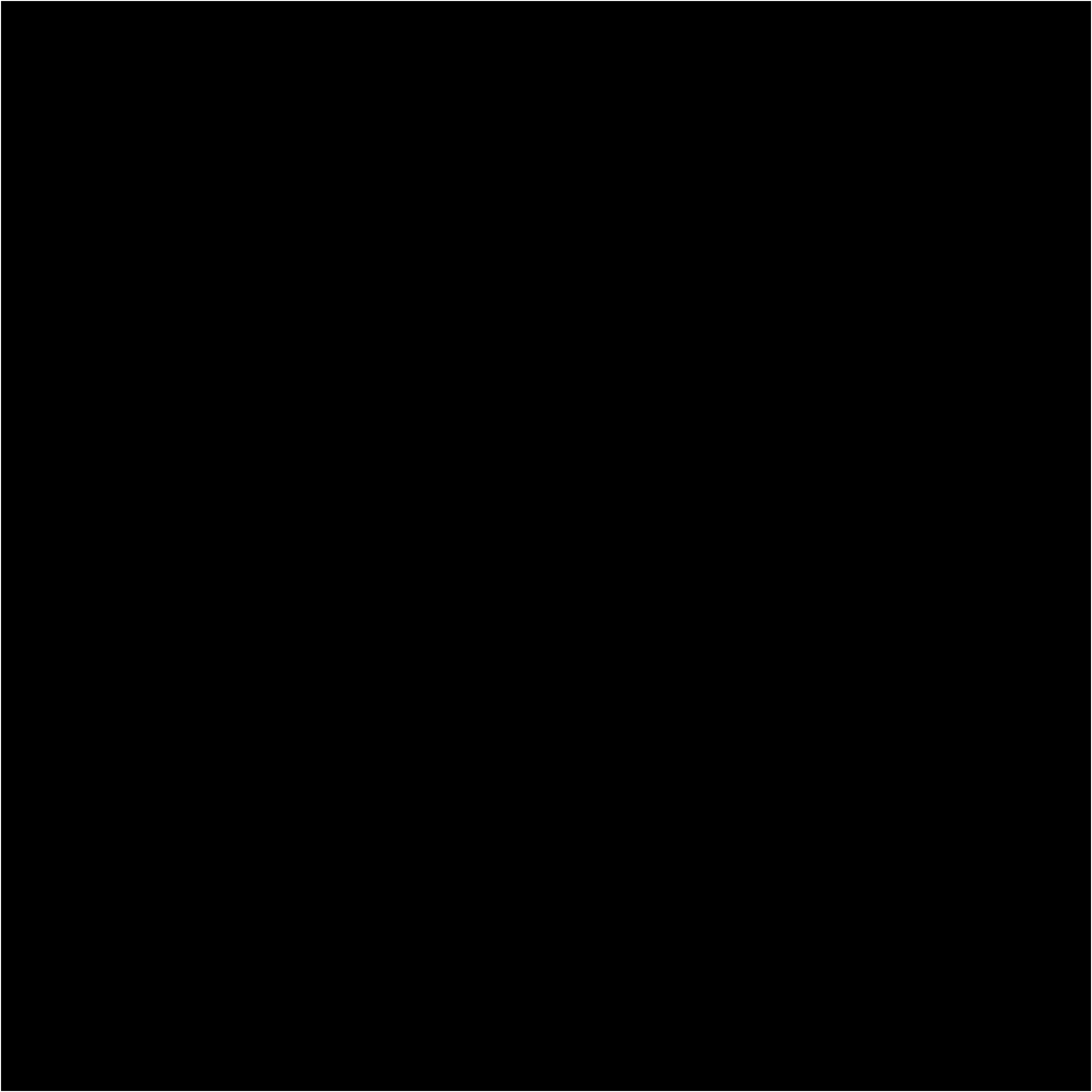
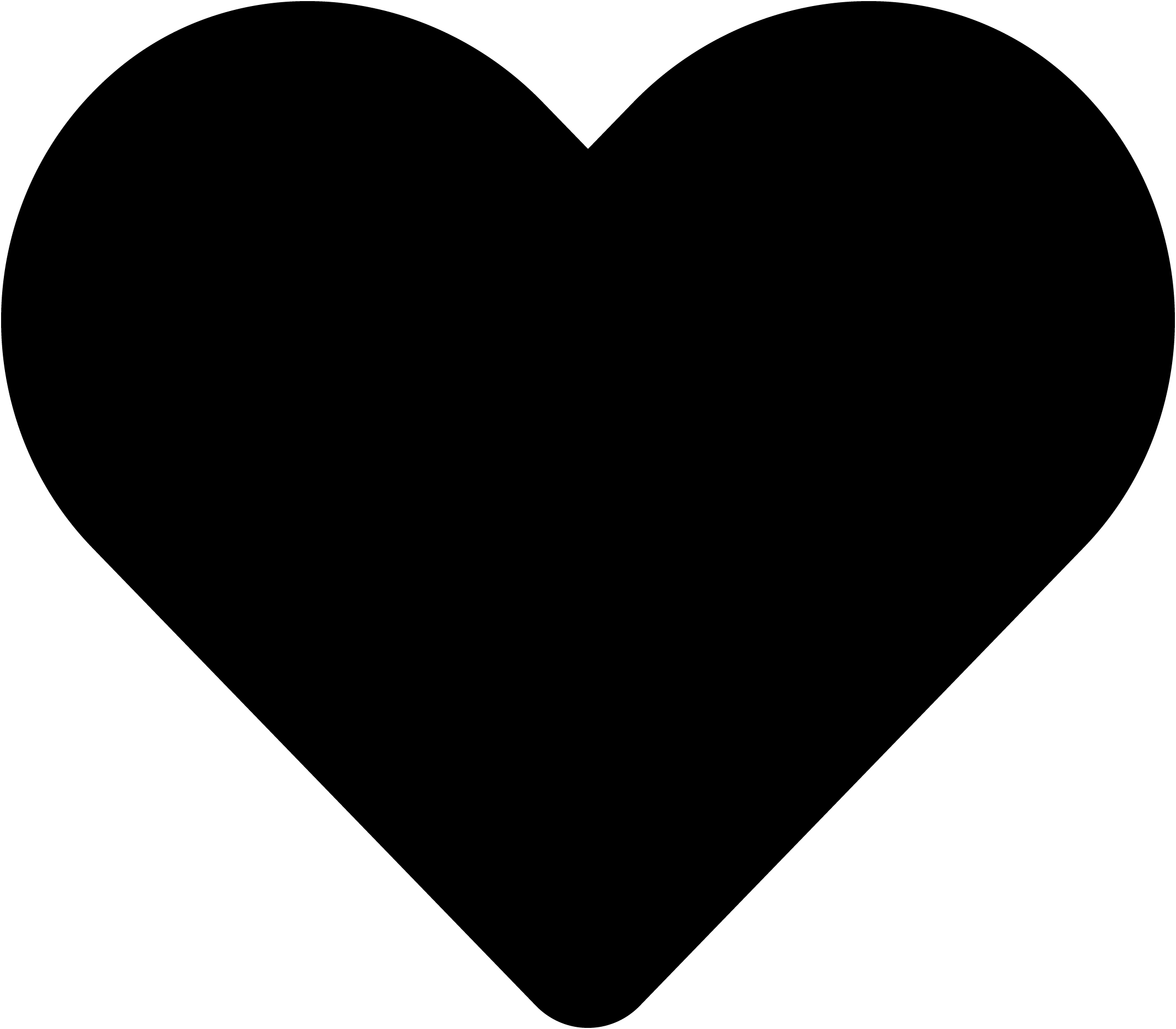
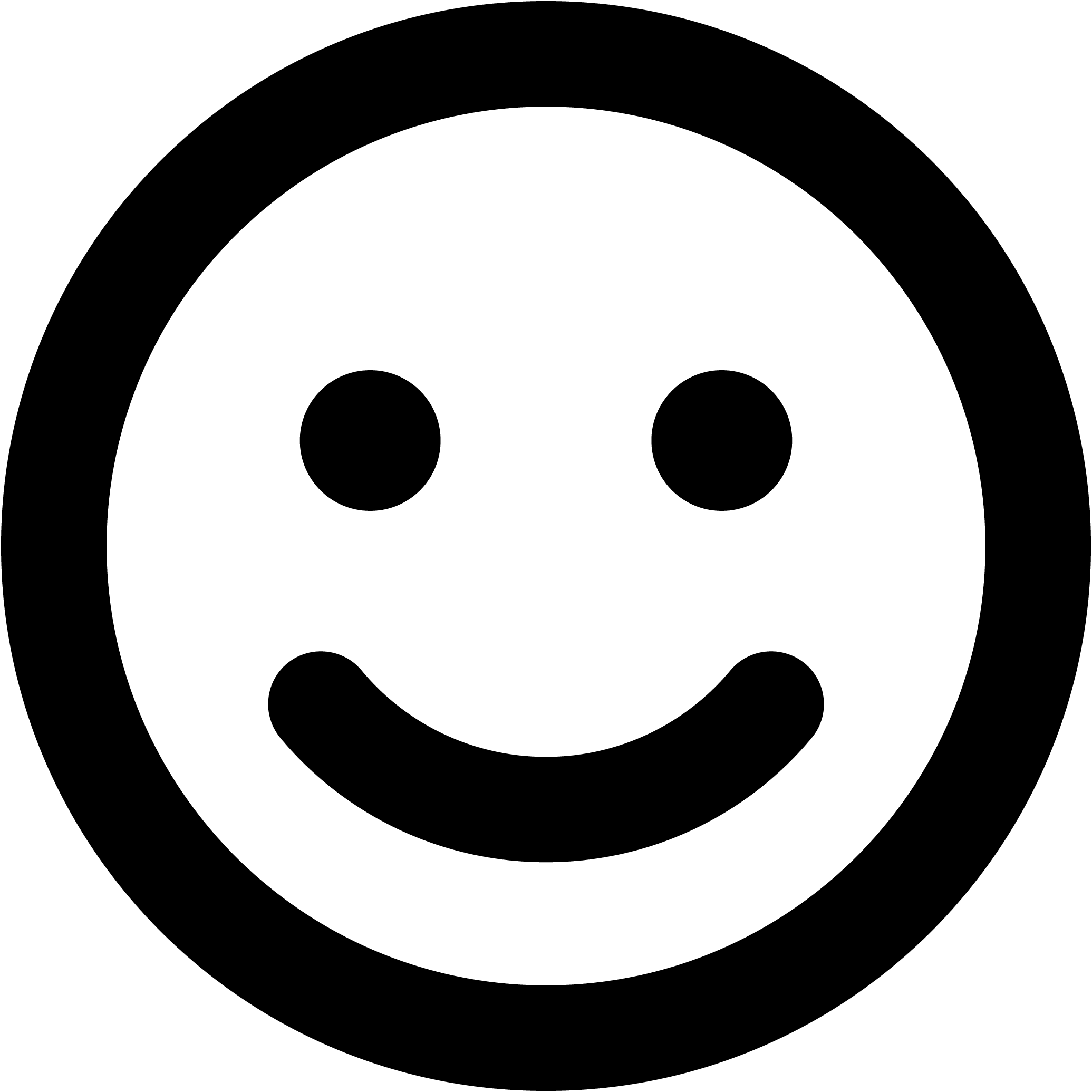
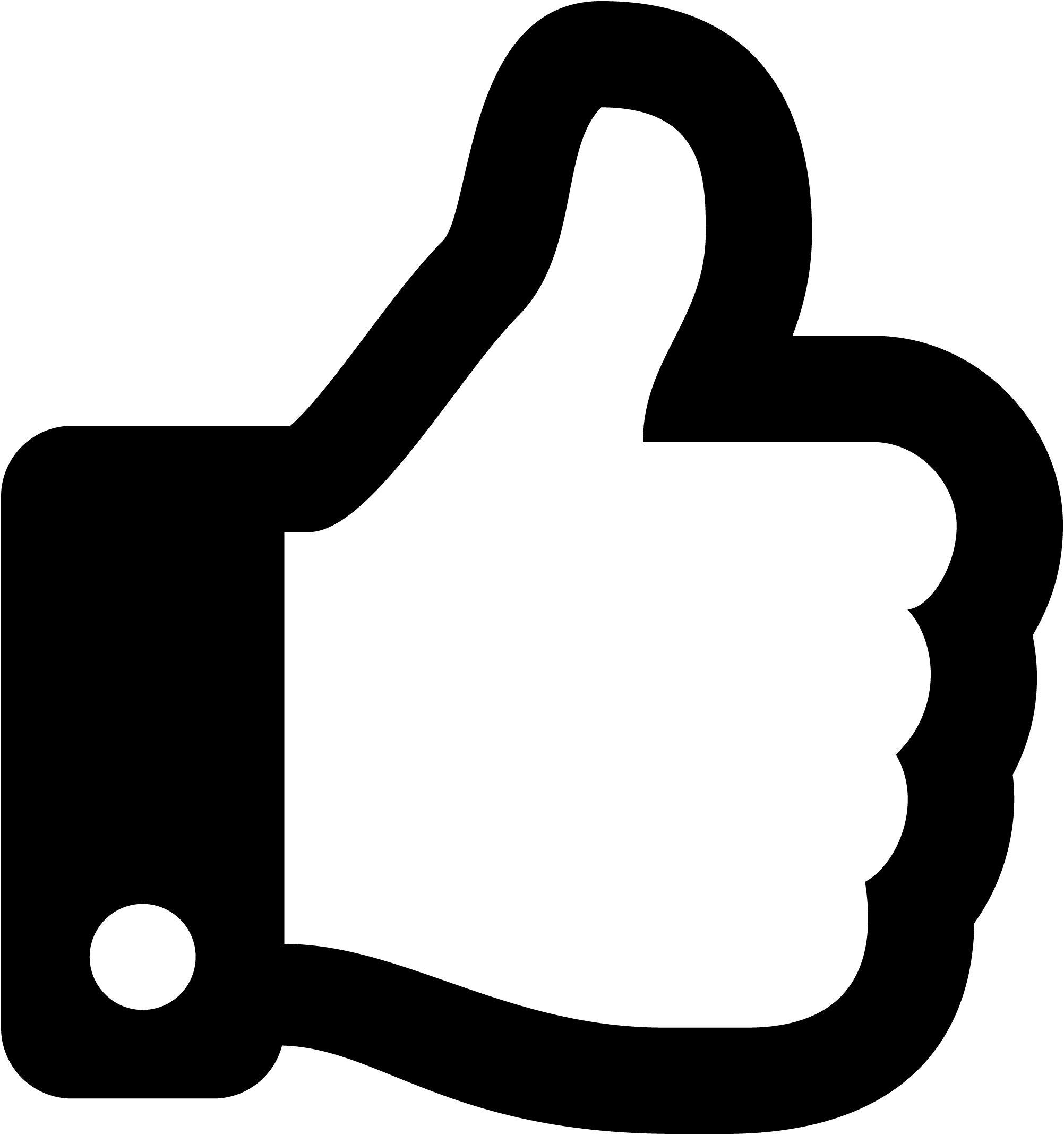
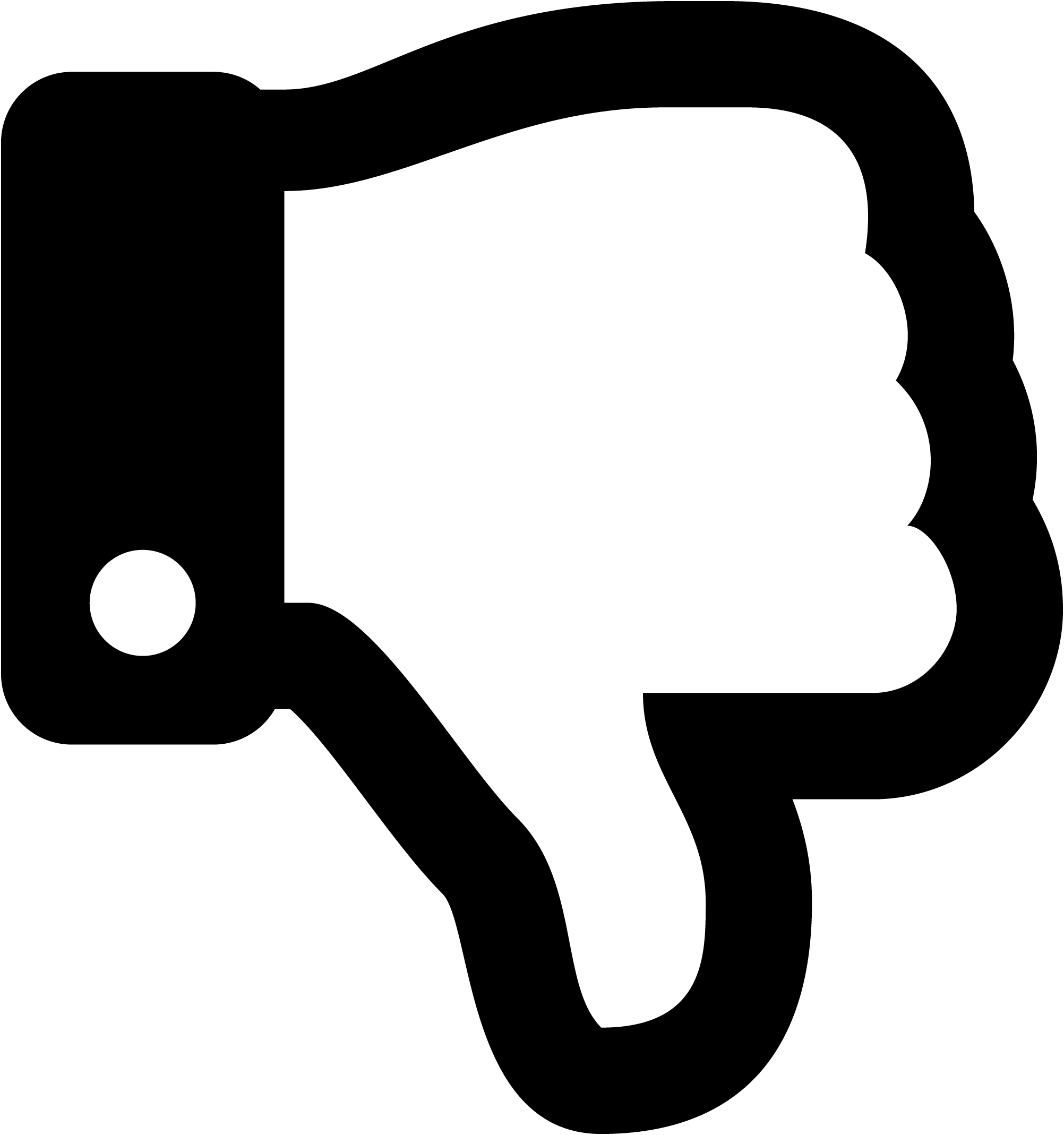
Border colour
Rotate
Skew (x-axis)
Skew (y-axis)
Image (max size: 2mb)
Or drag a symbol into the upload area
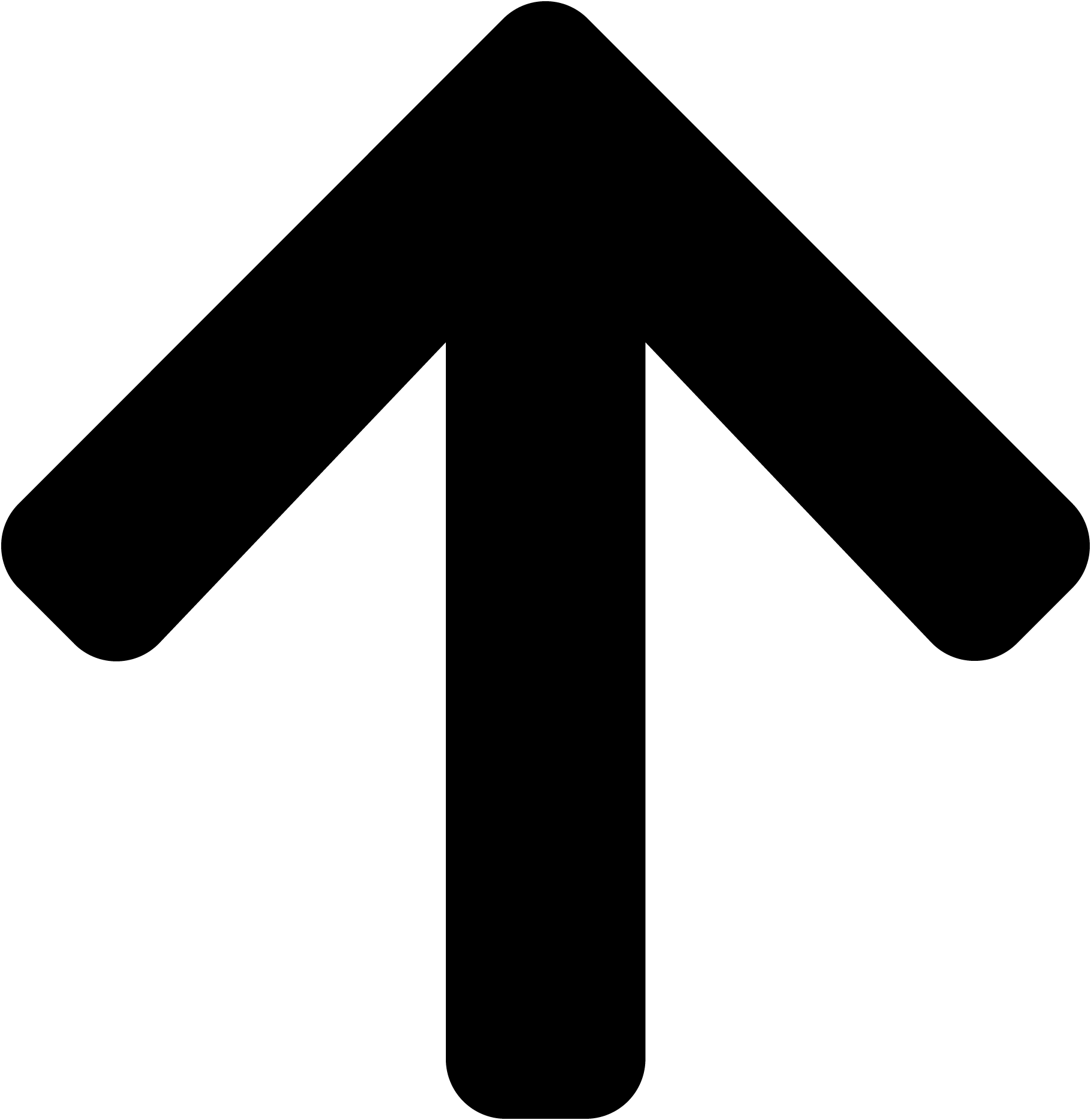
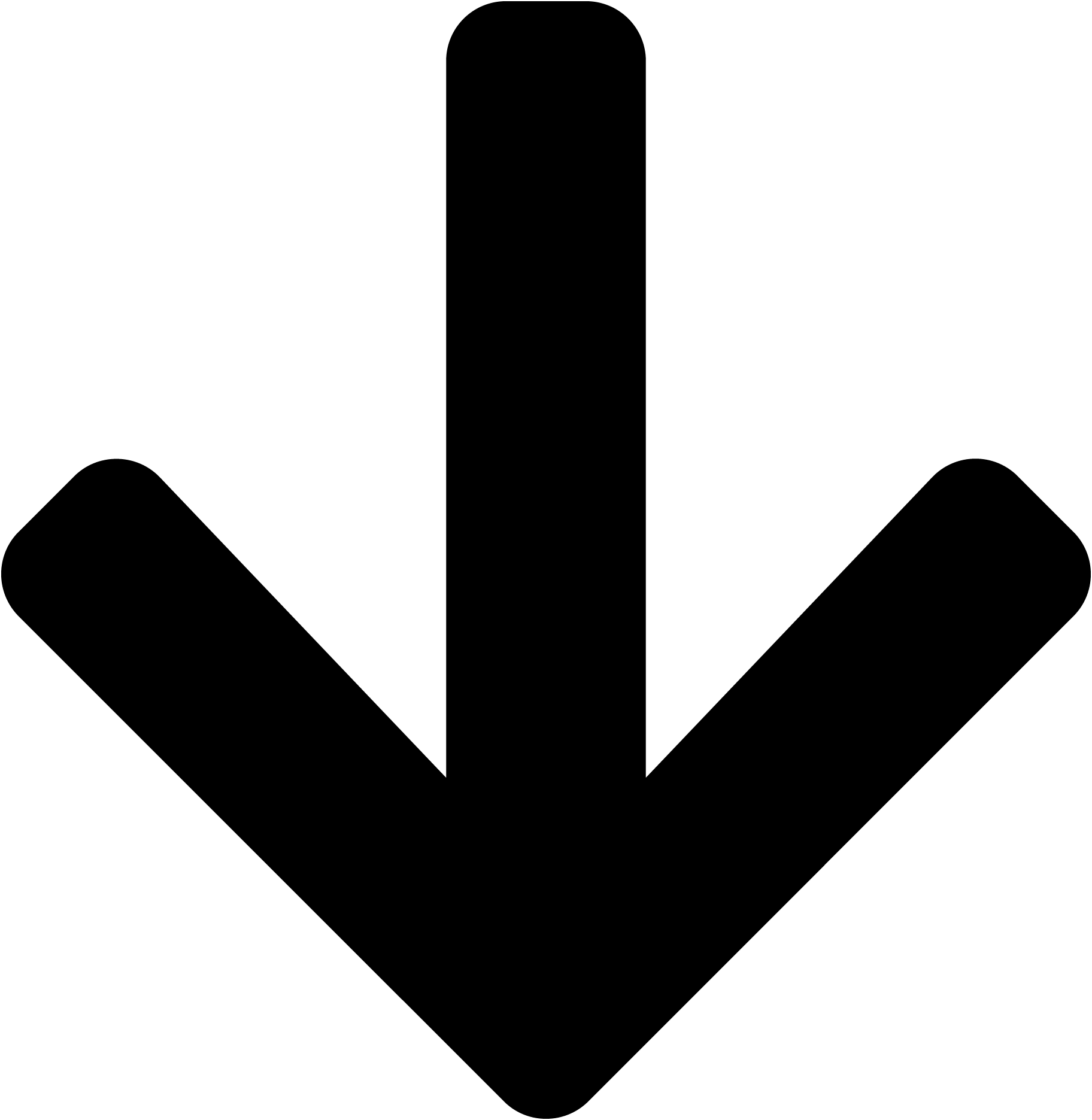
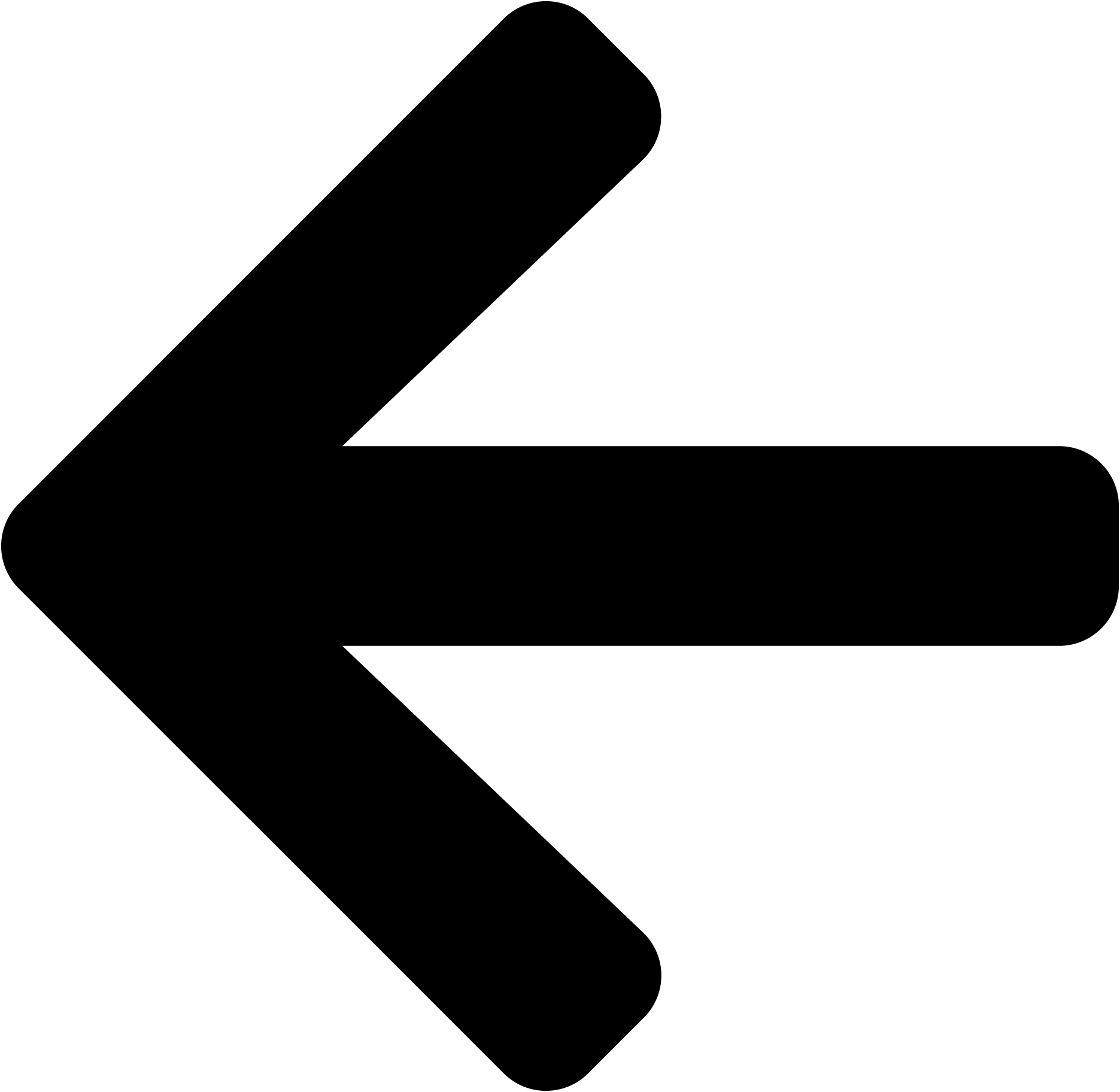
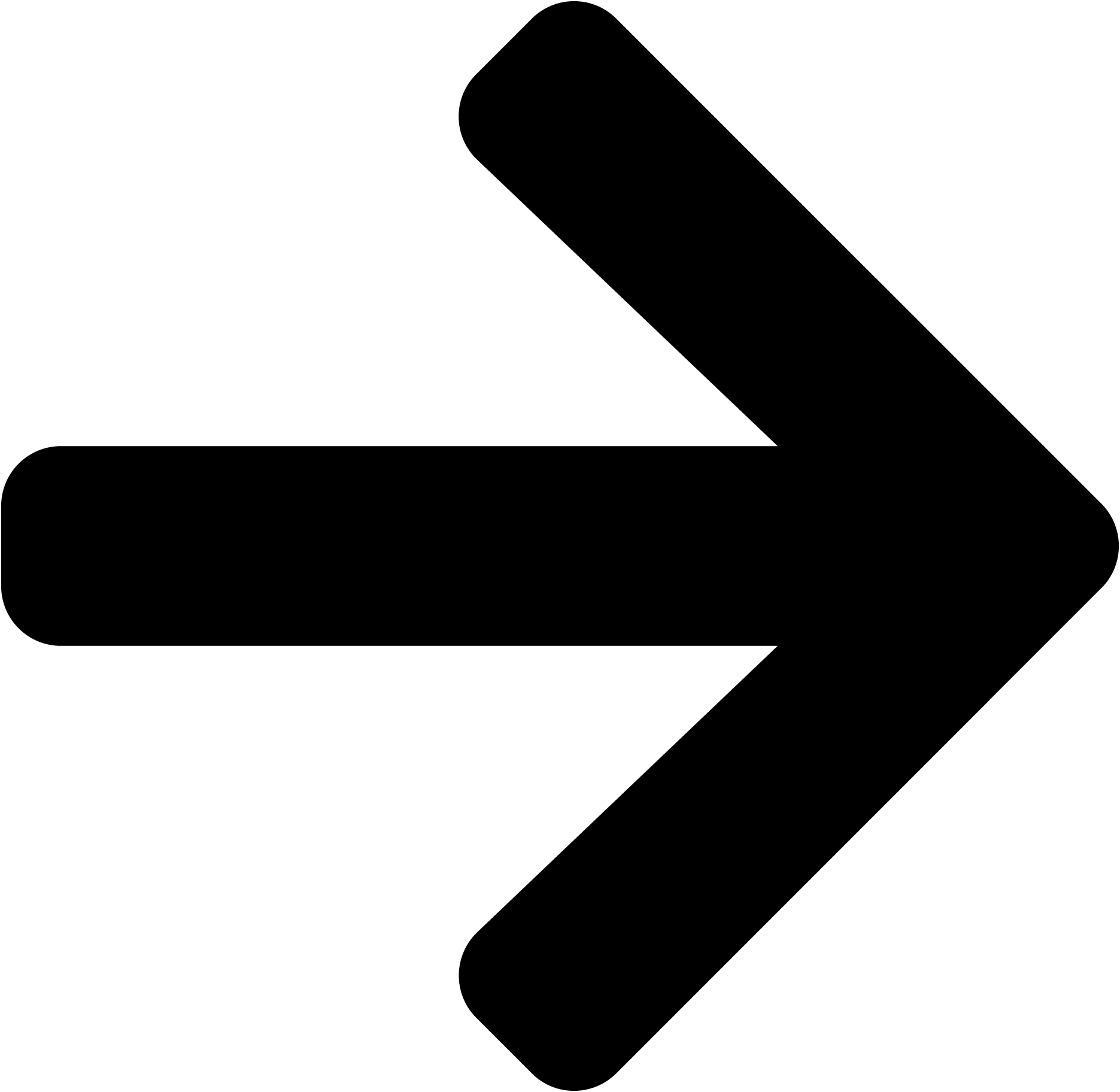
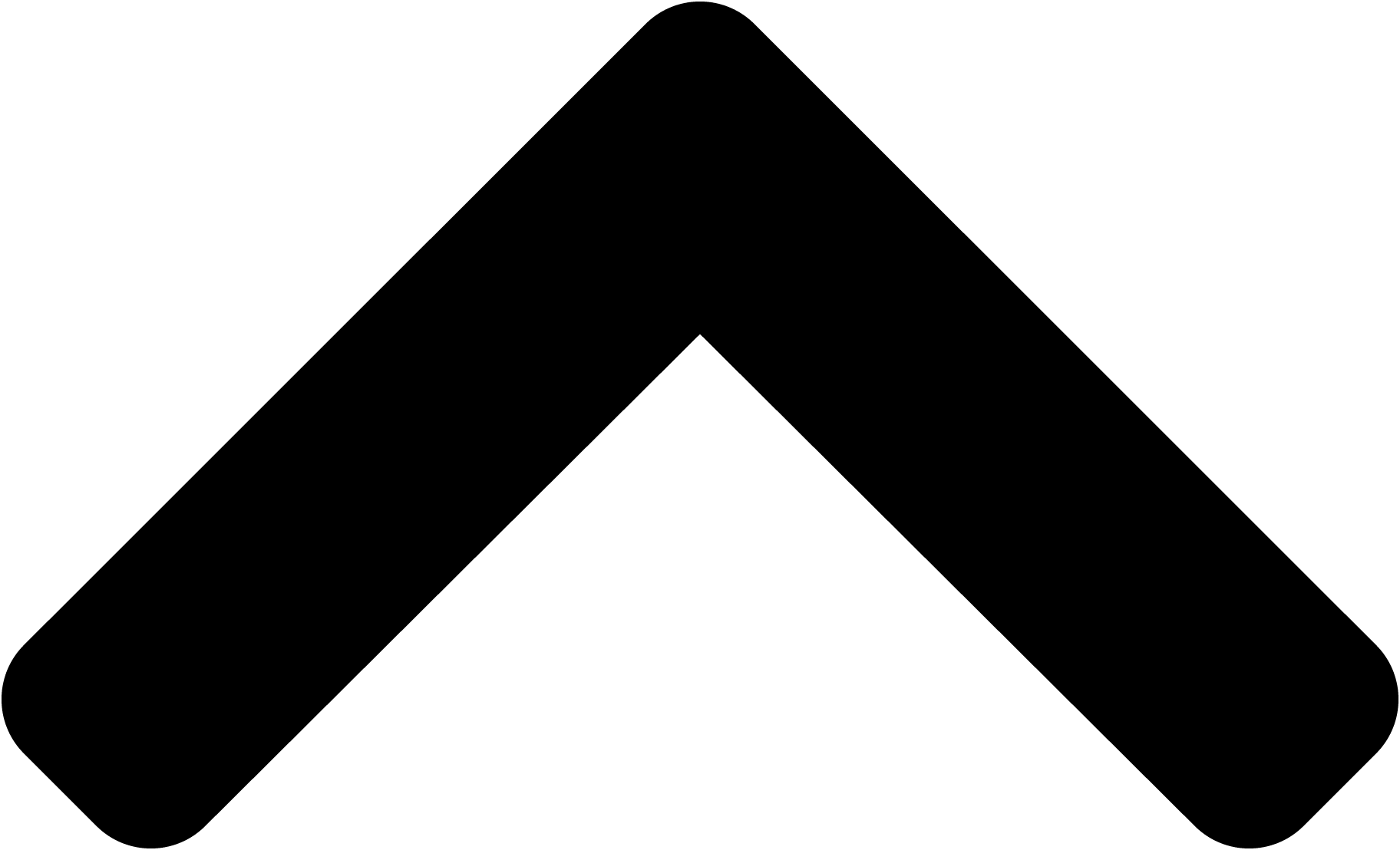
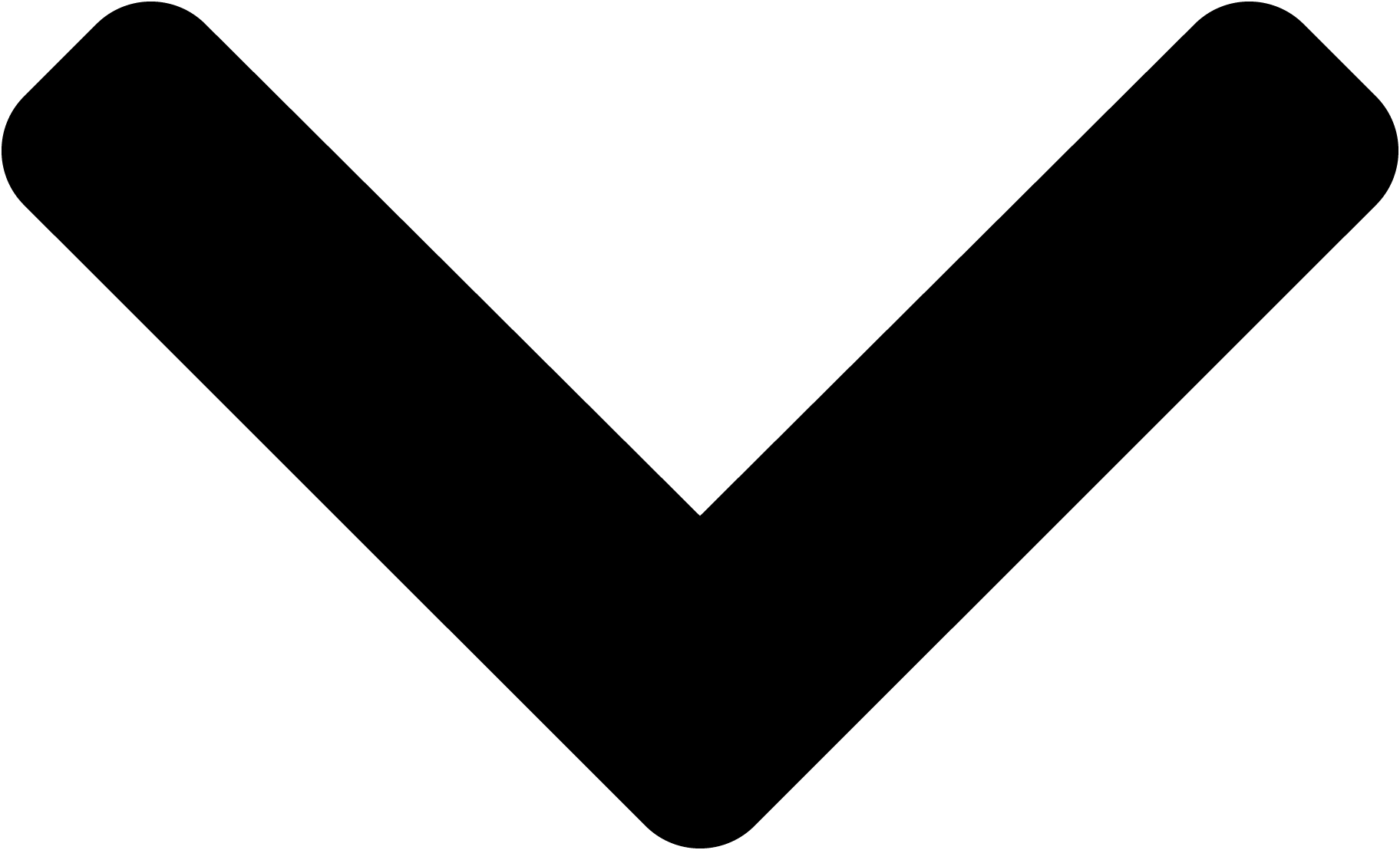
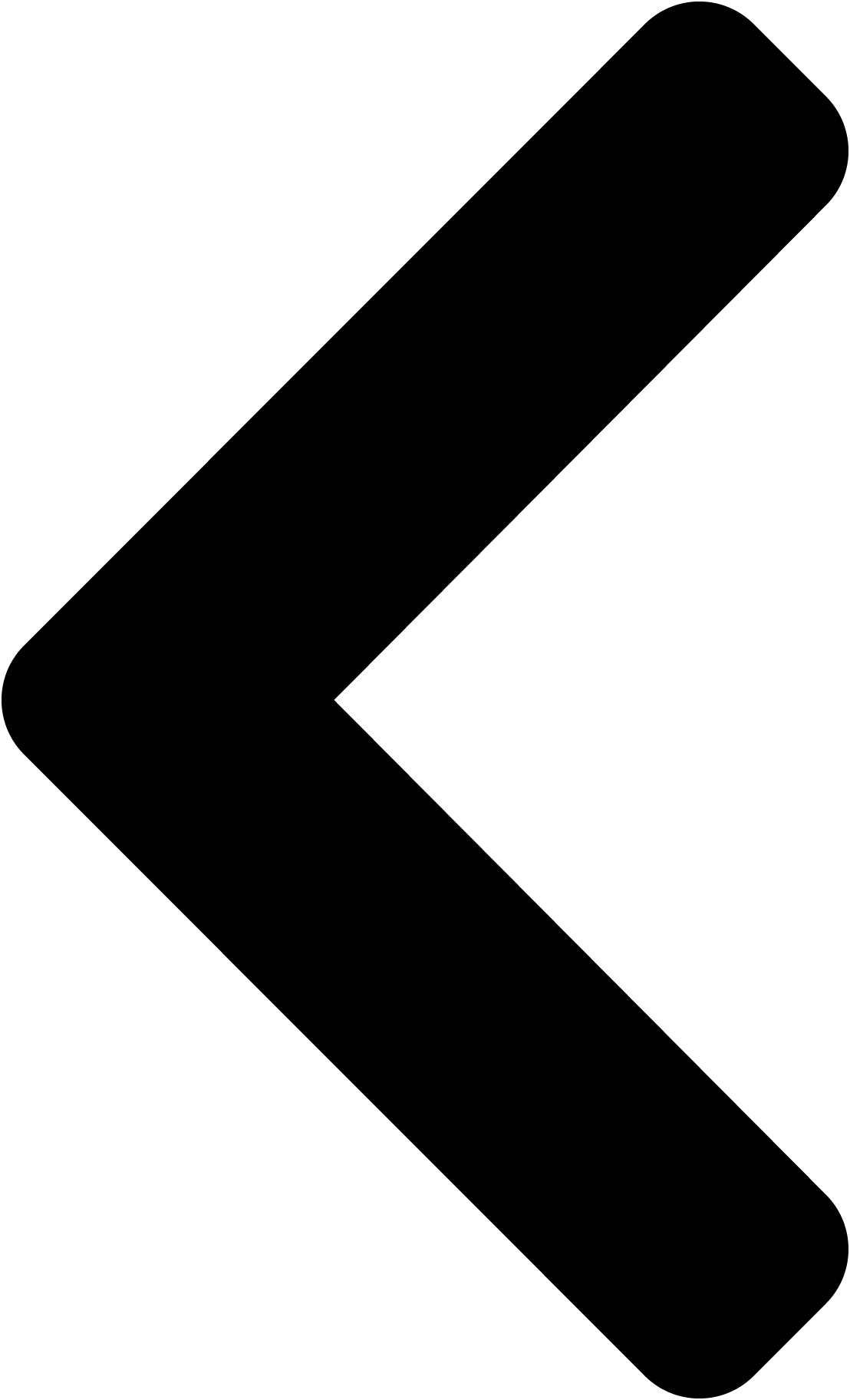
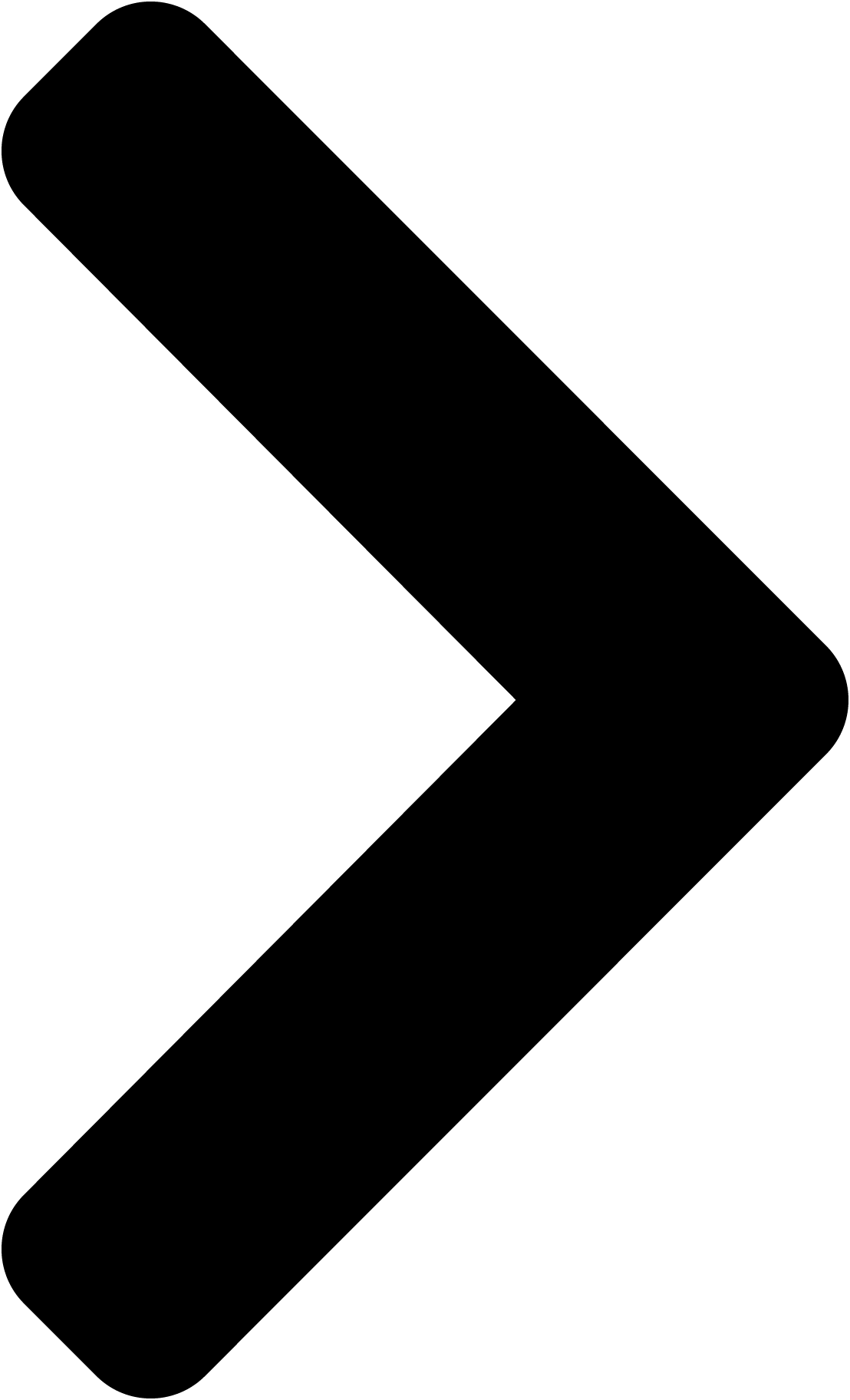
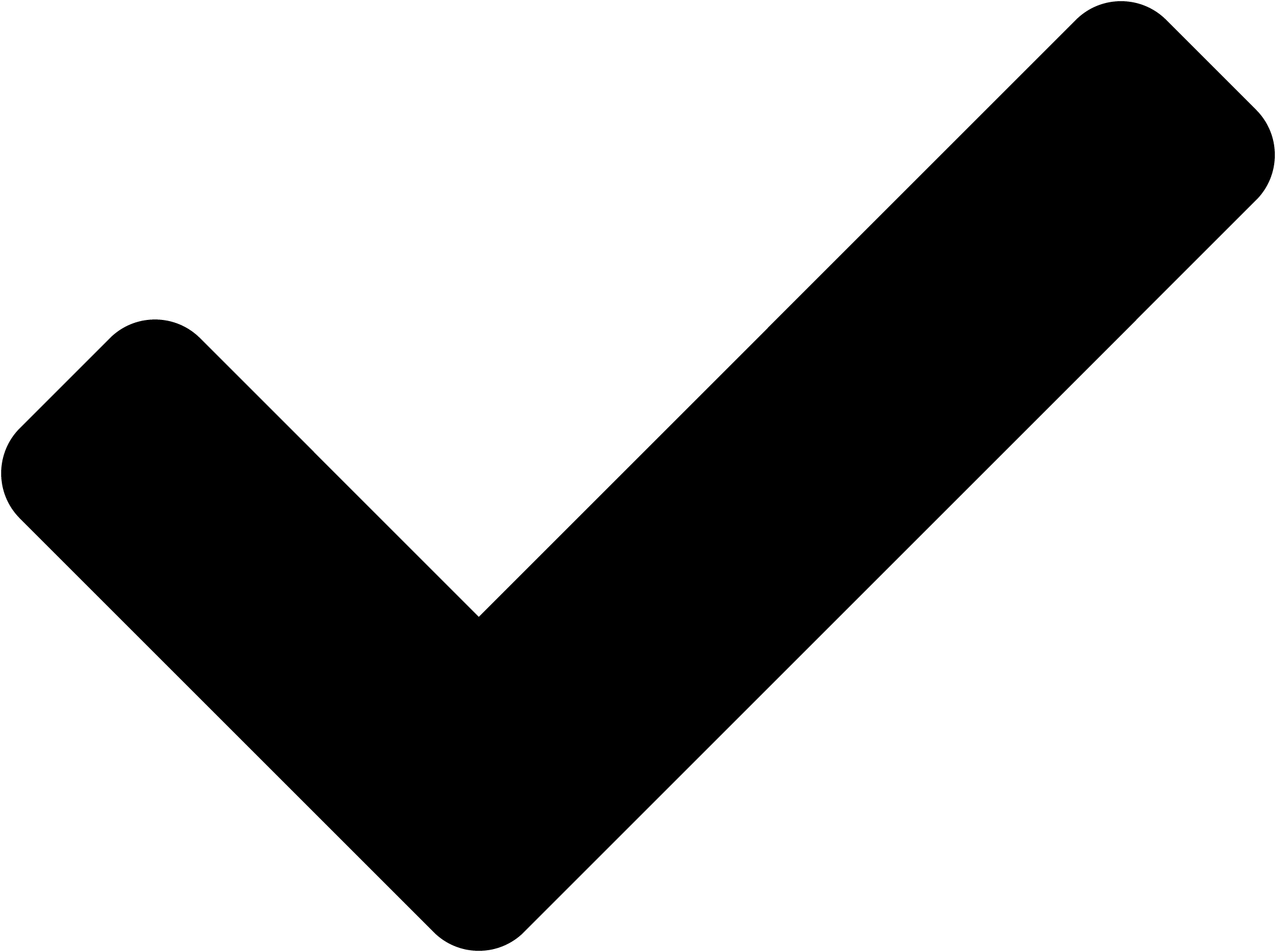
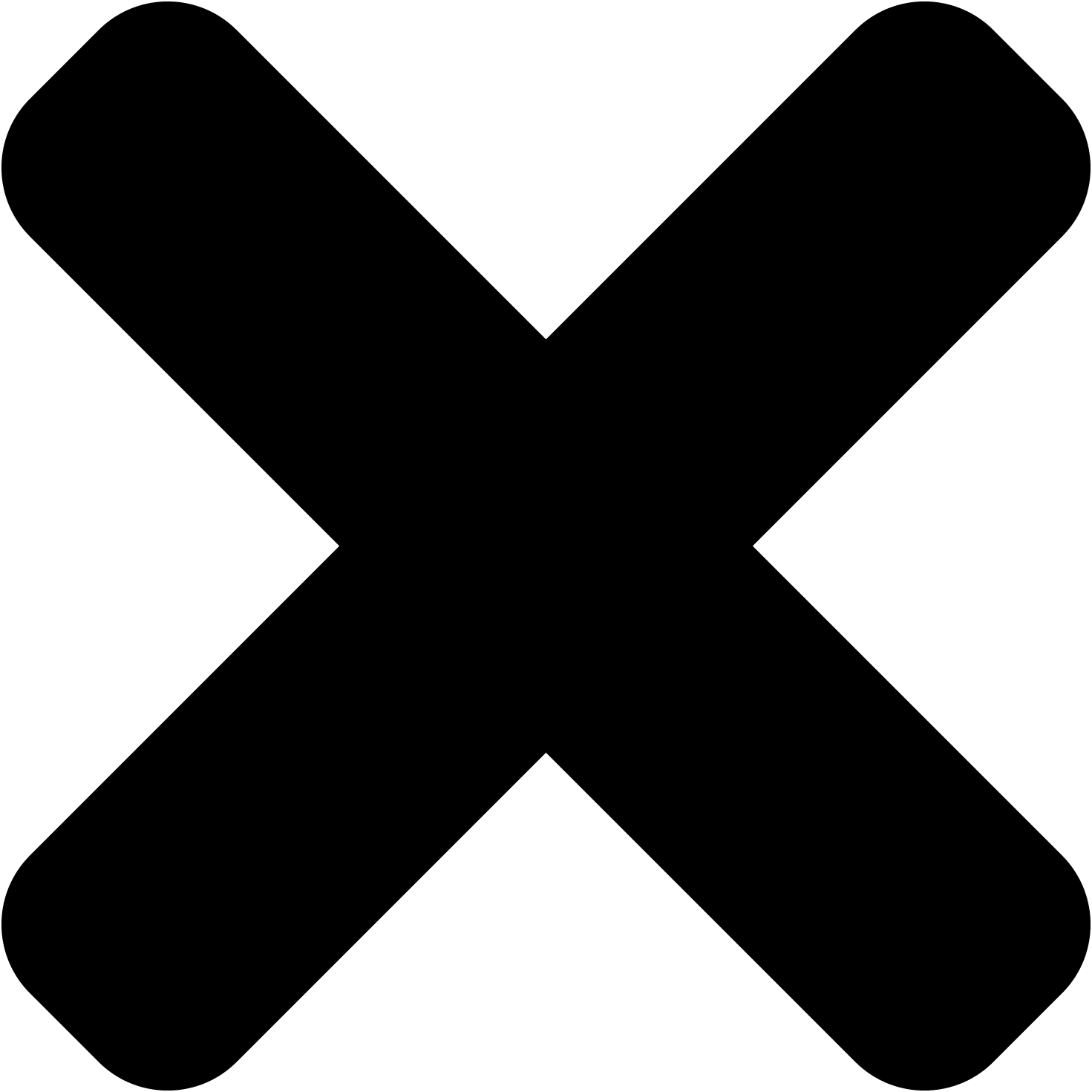
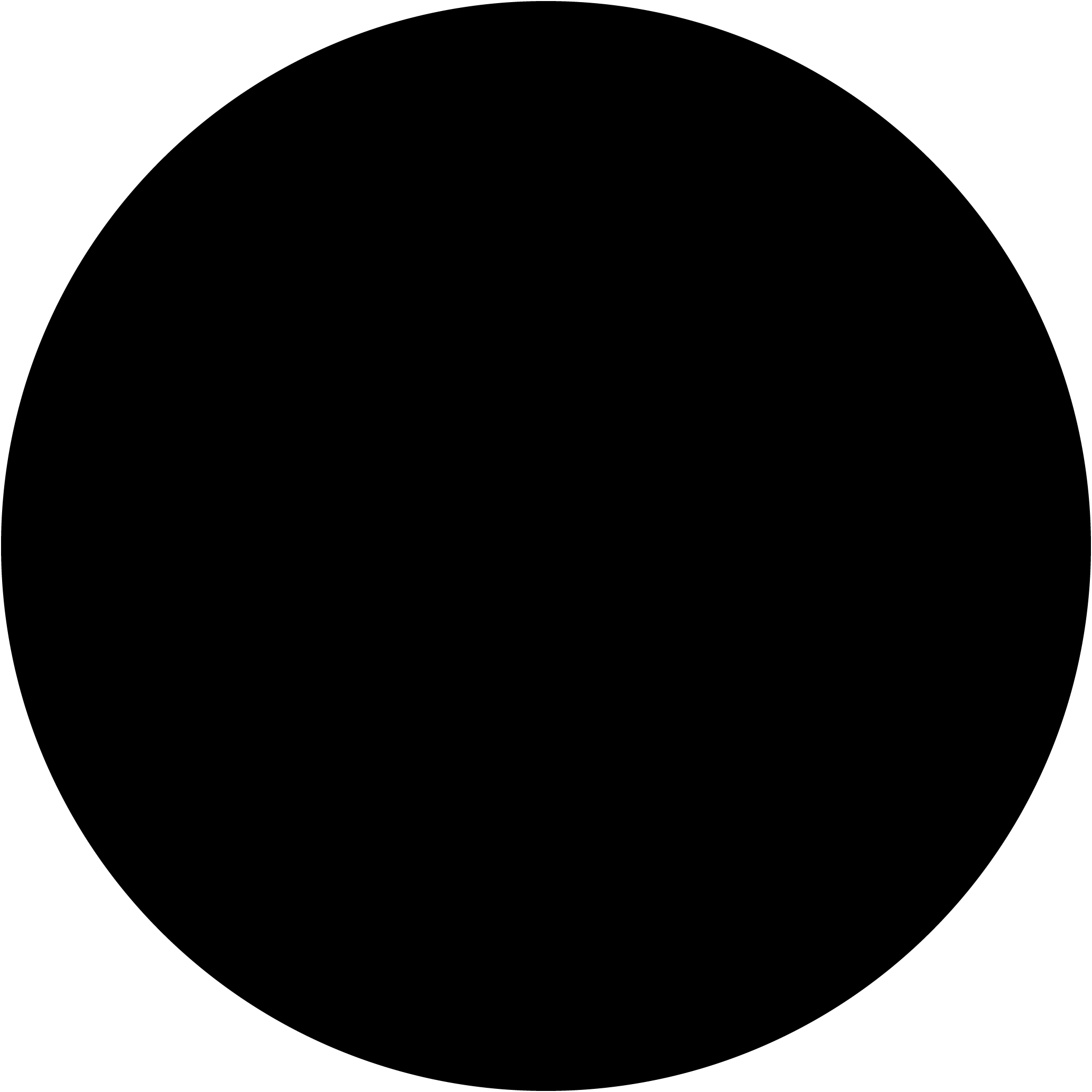
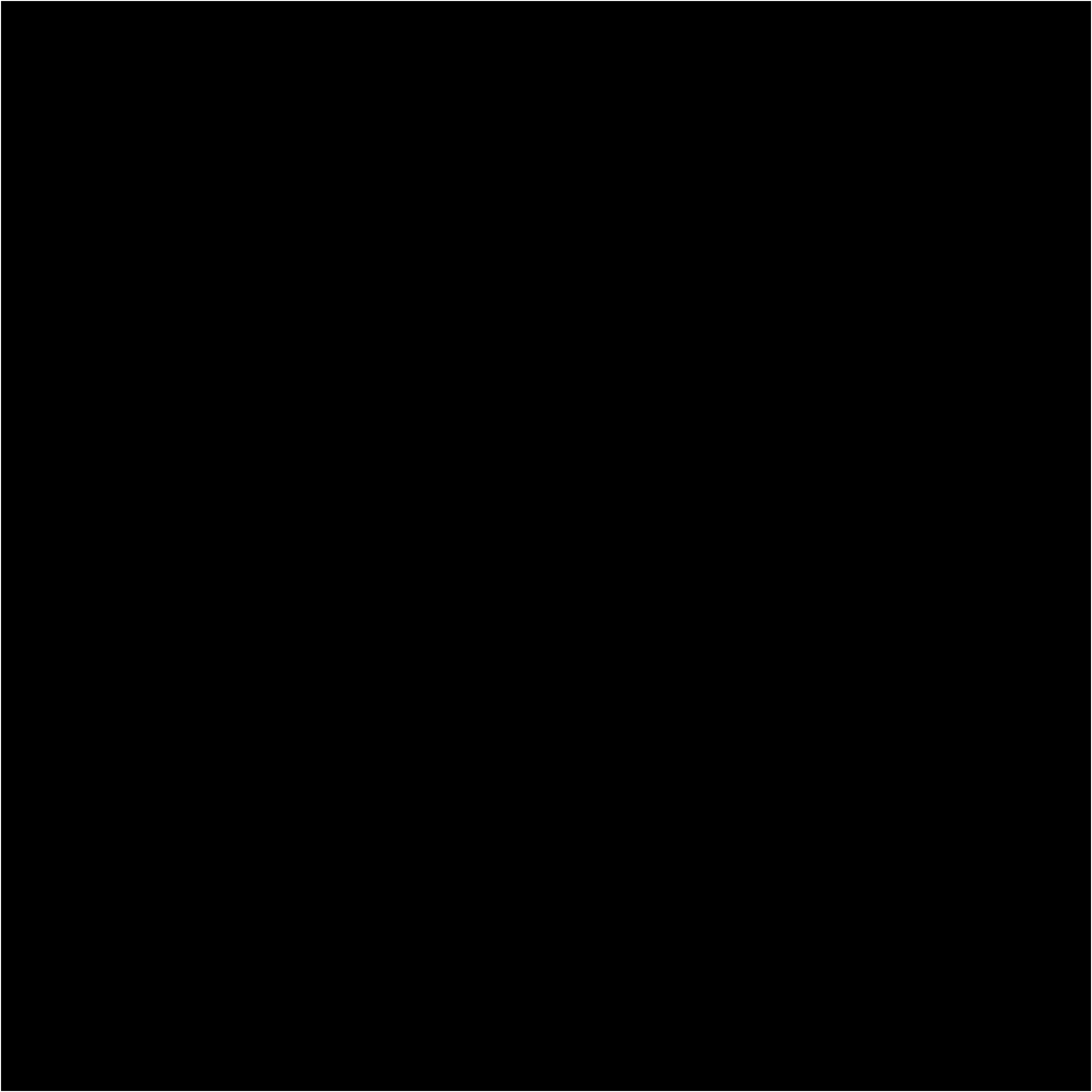
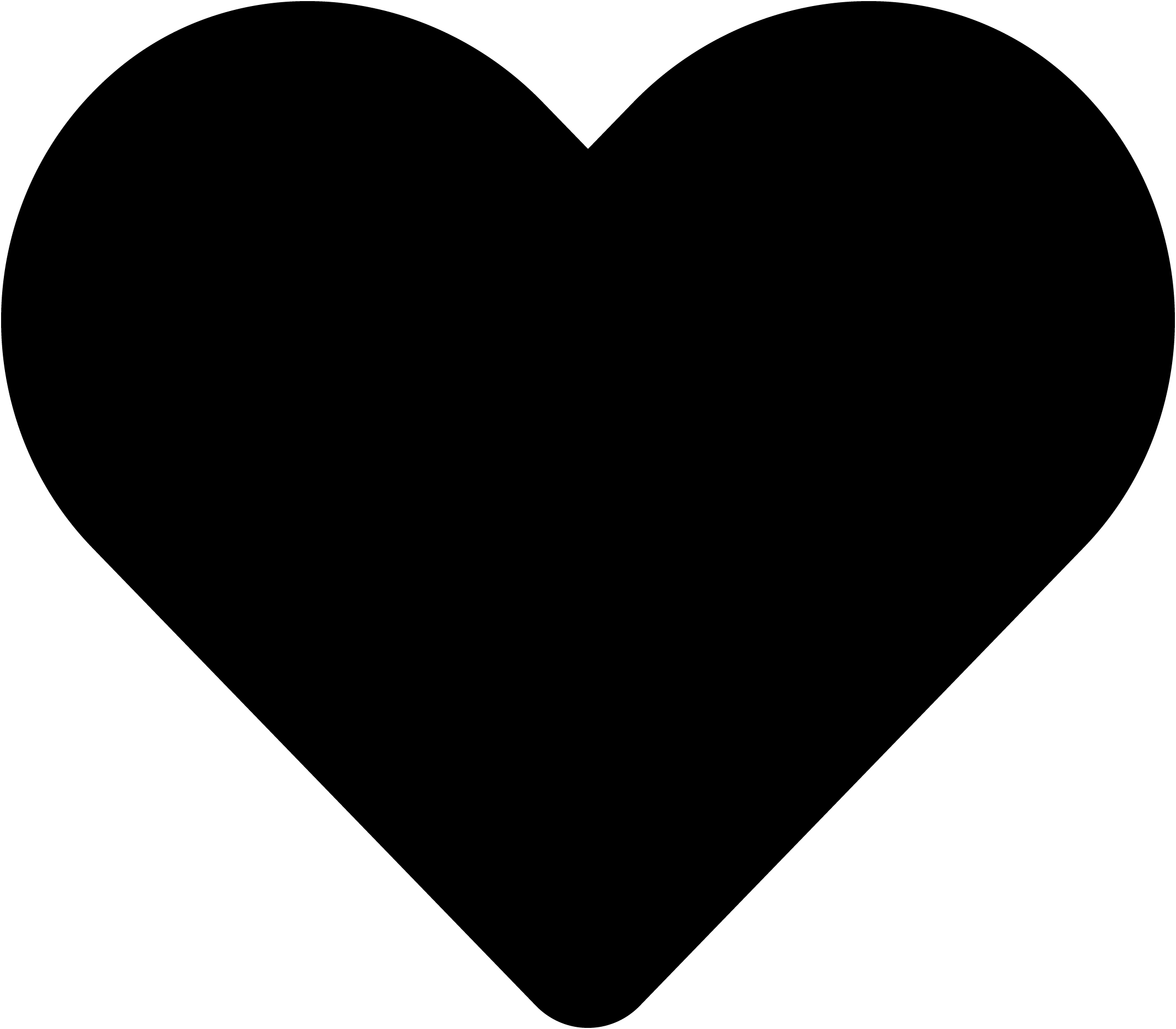
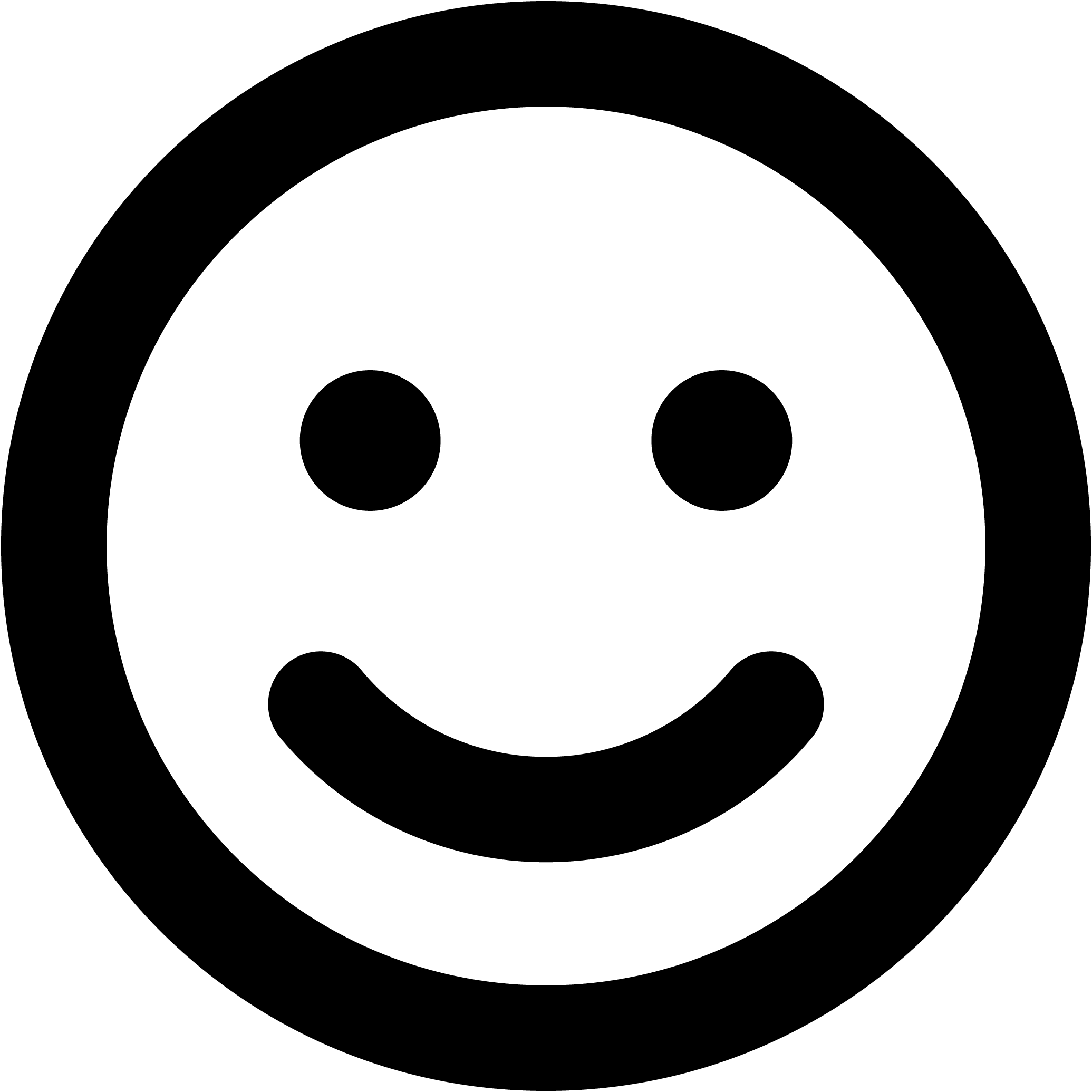
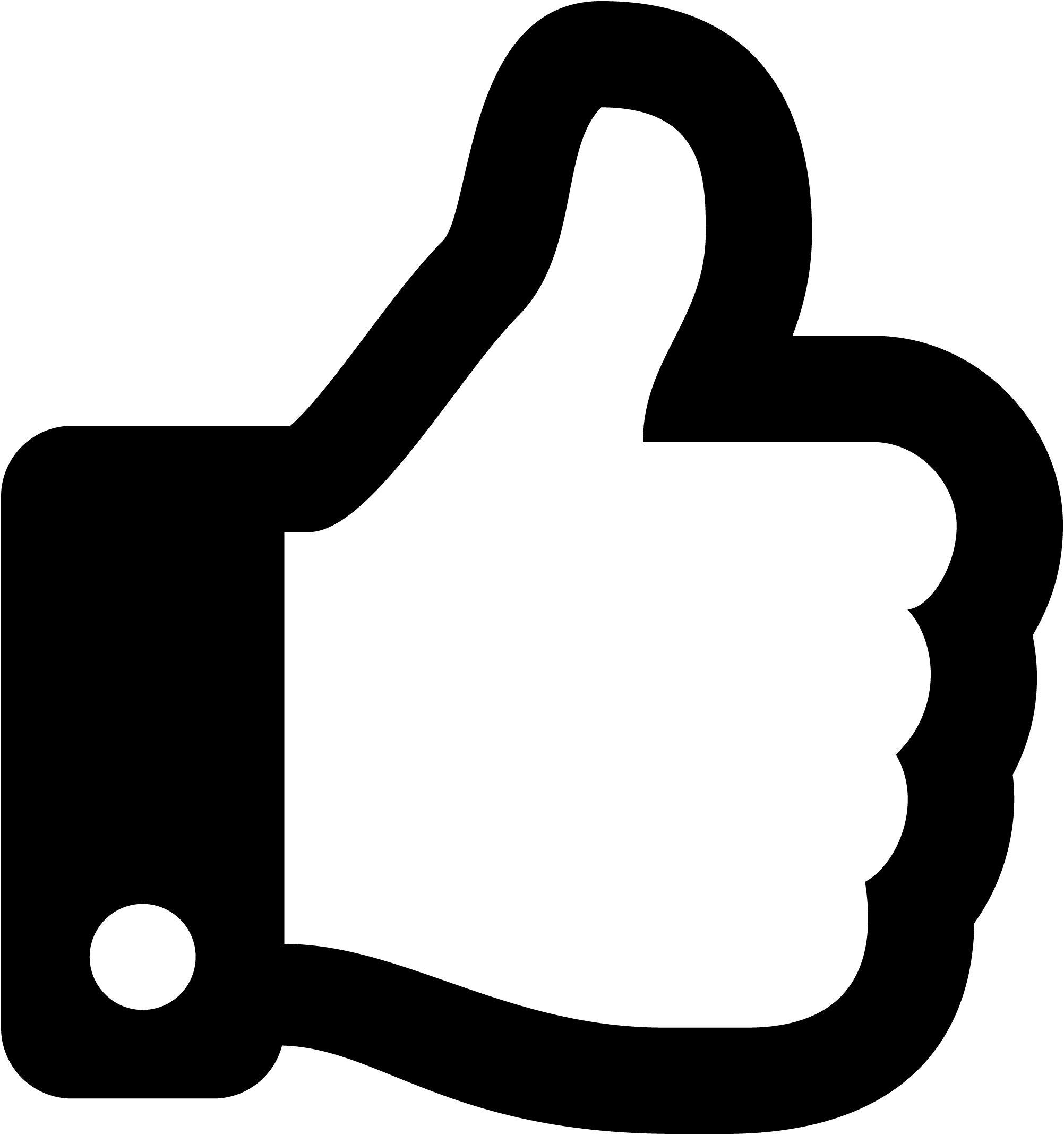
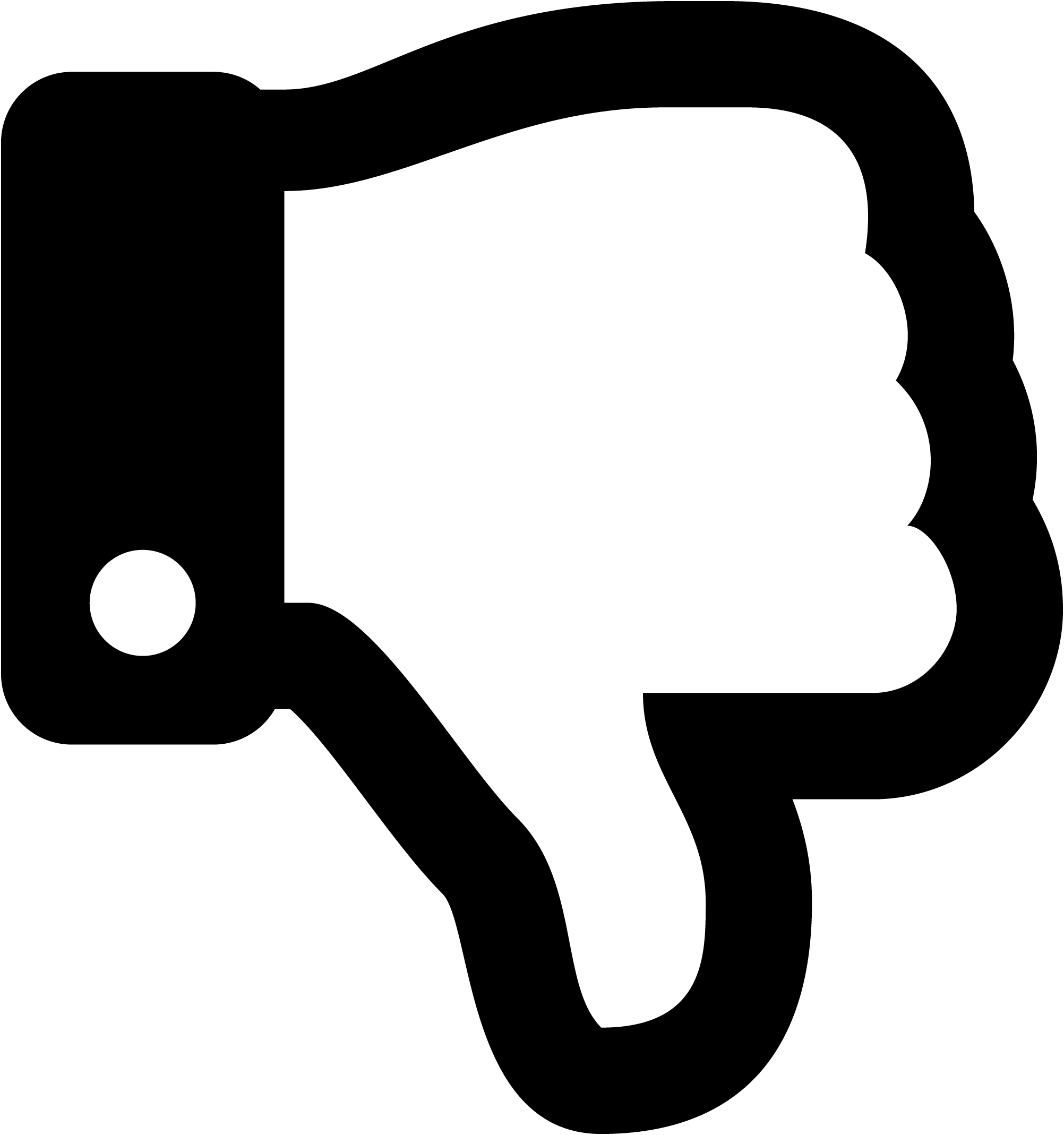
Image description/alt-tag
Image caption
Image link
Rollover Image (max size: 2mb)
Or drag a symbol into the upload area
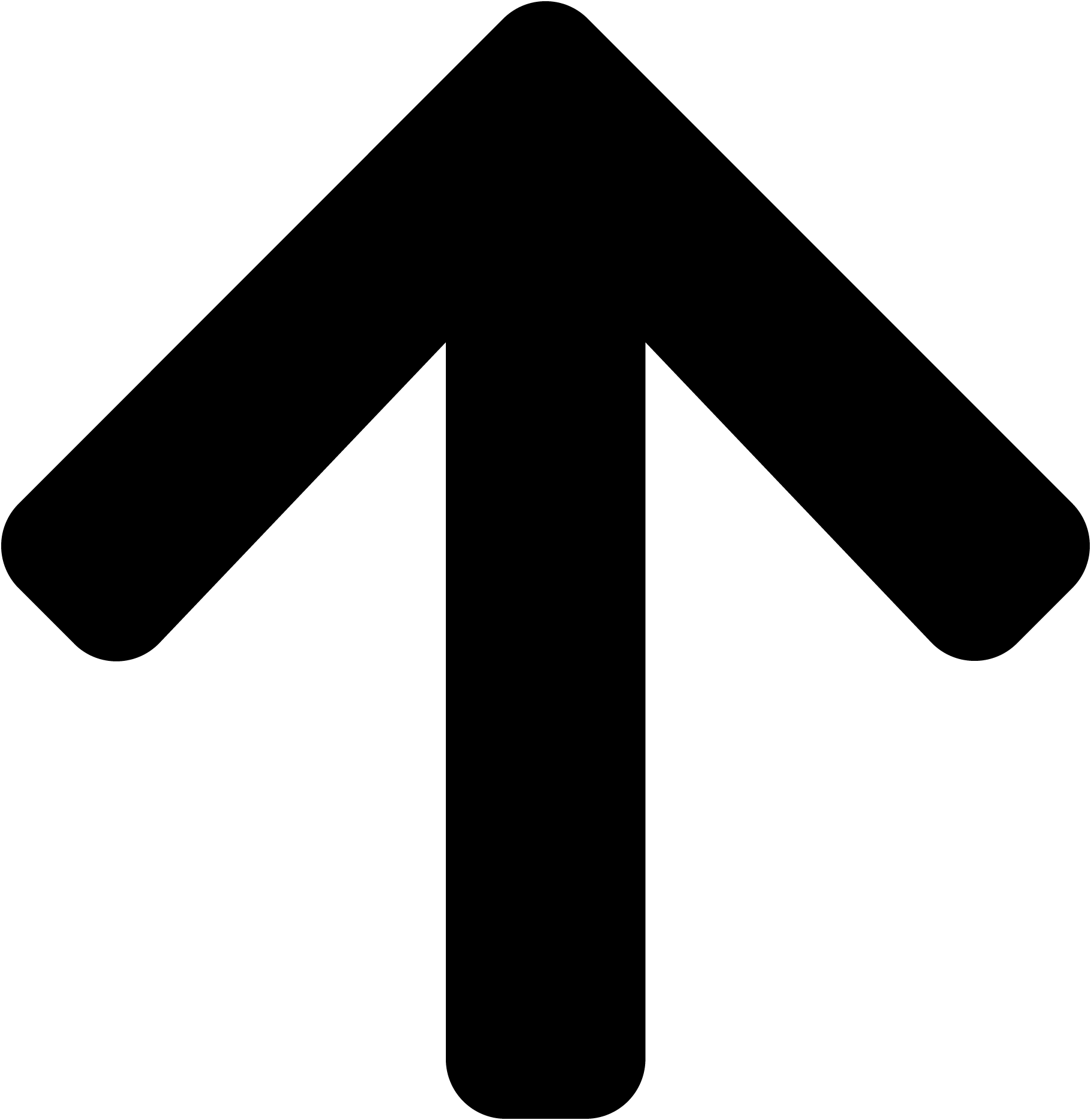
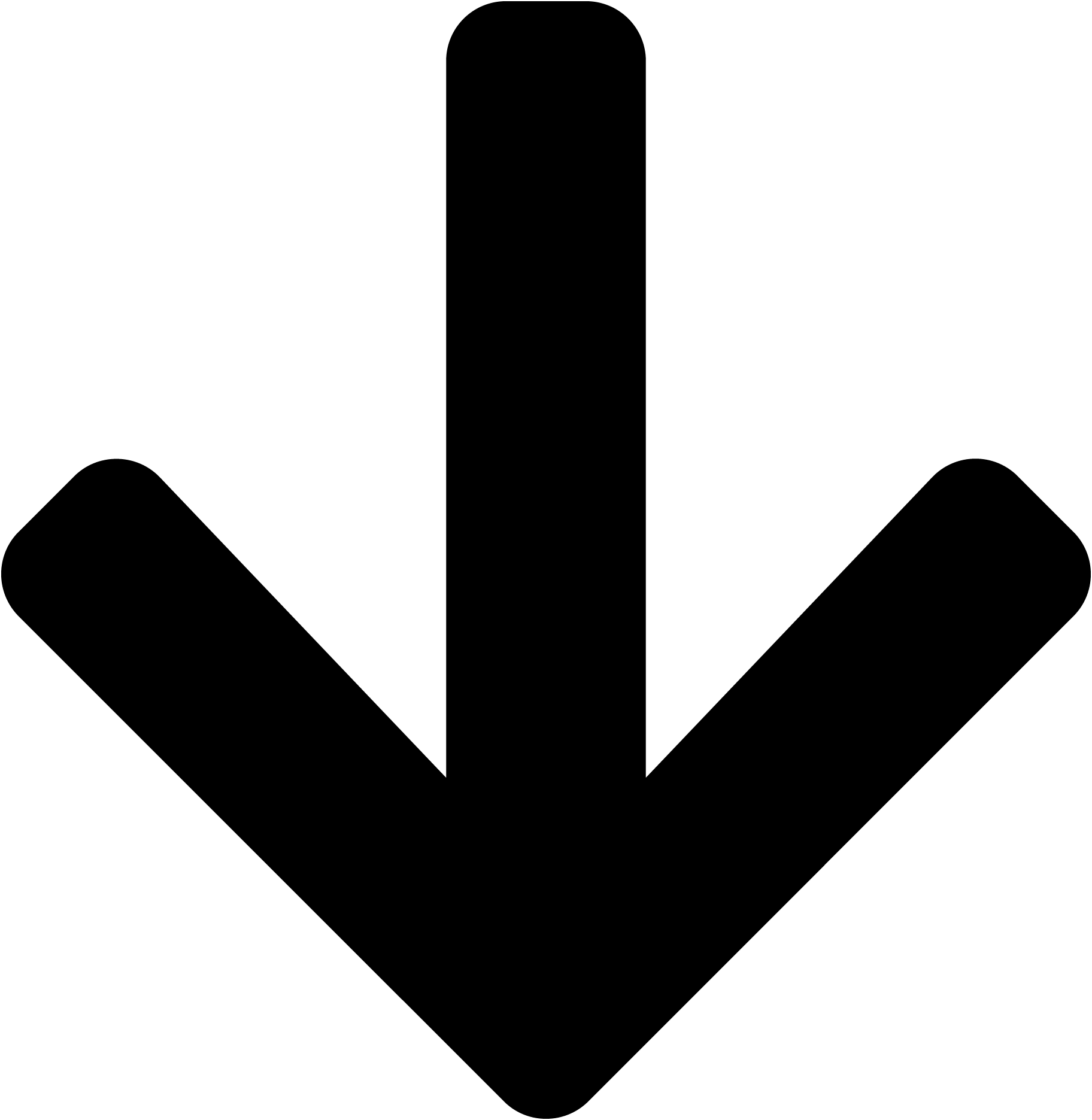
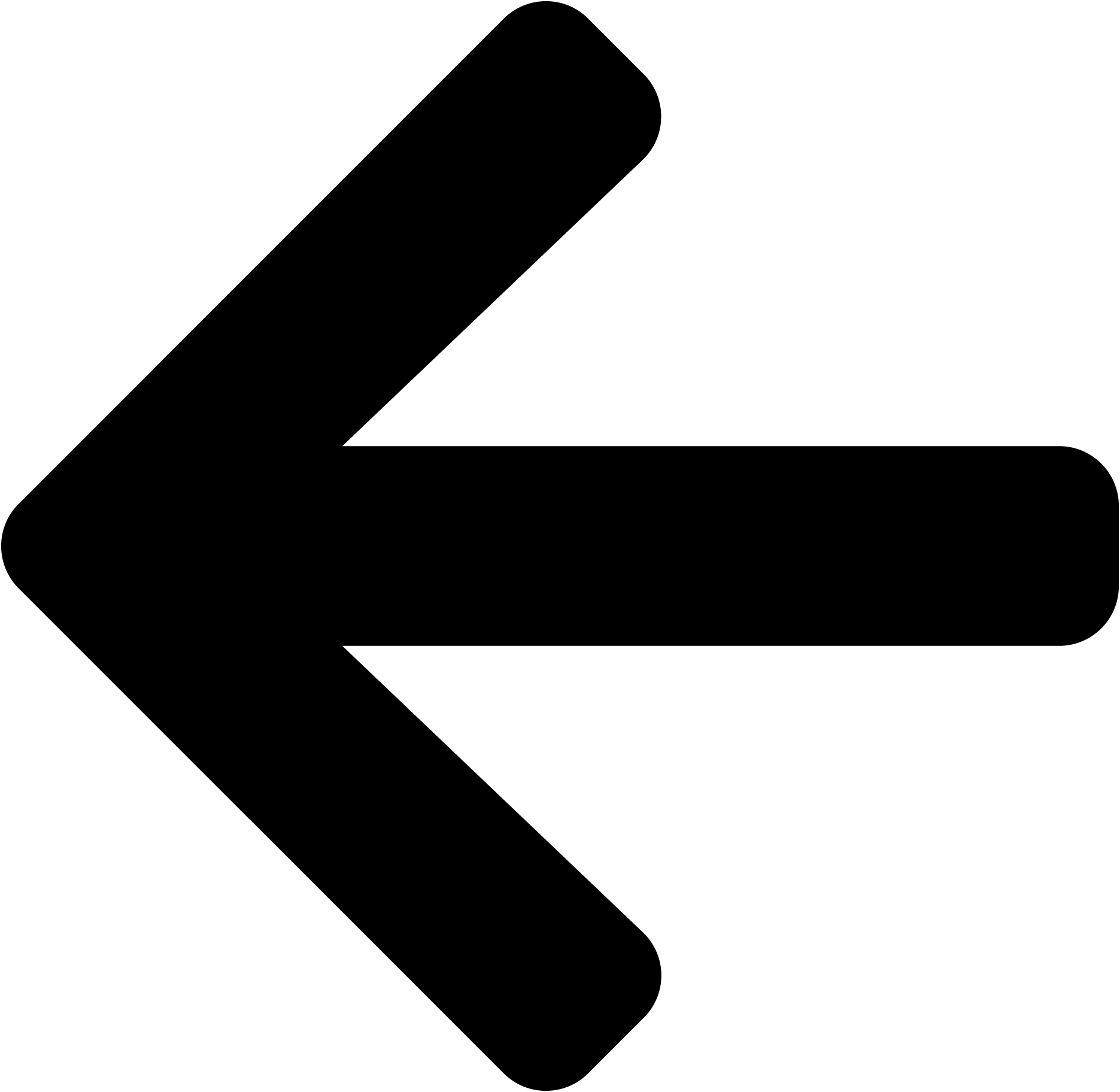
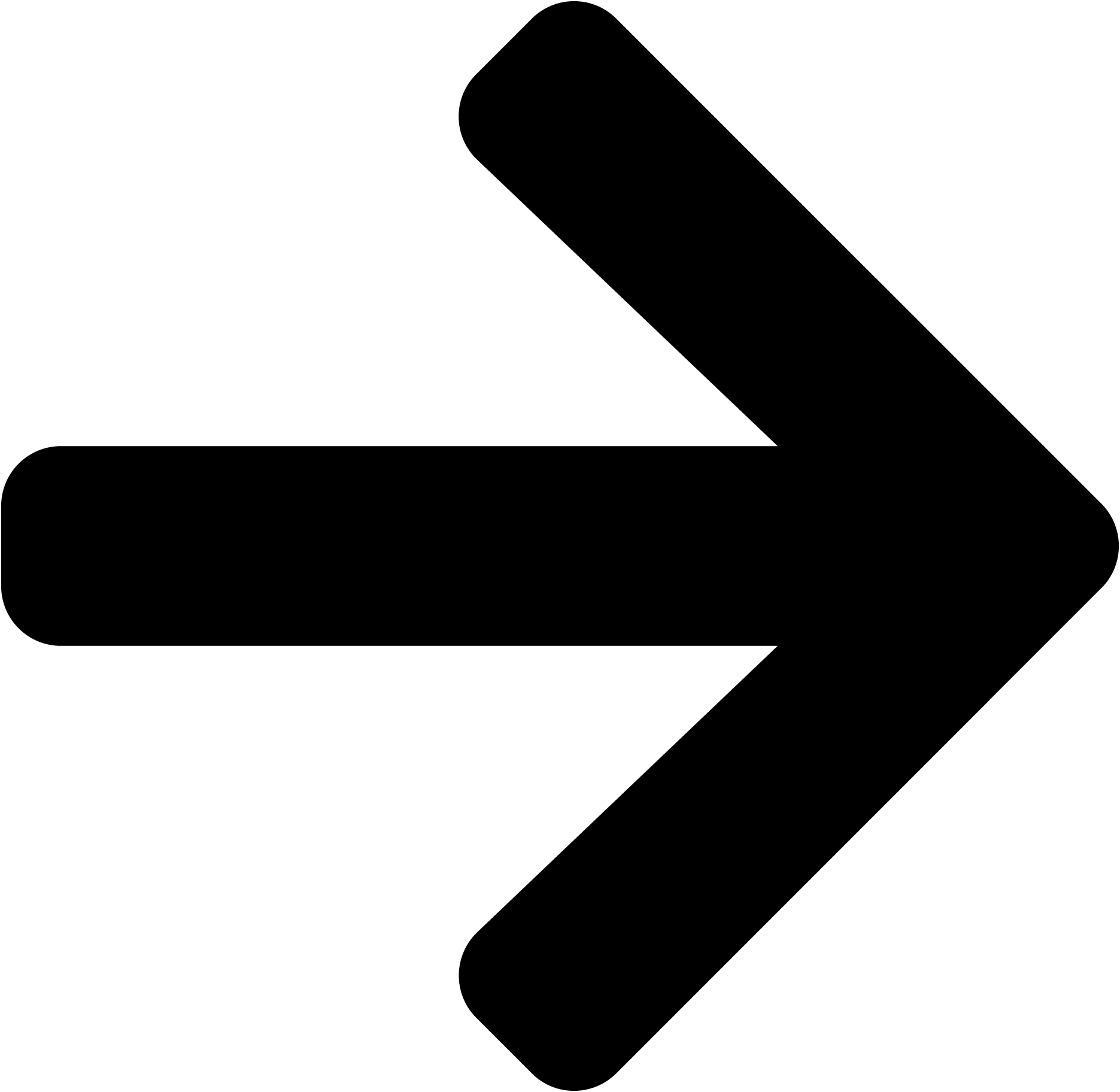
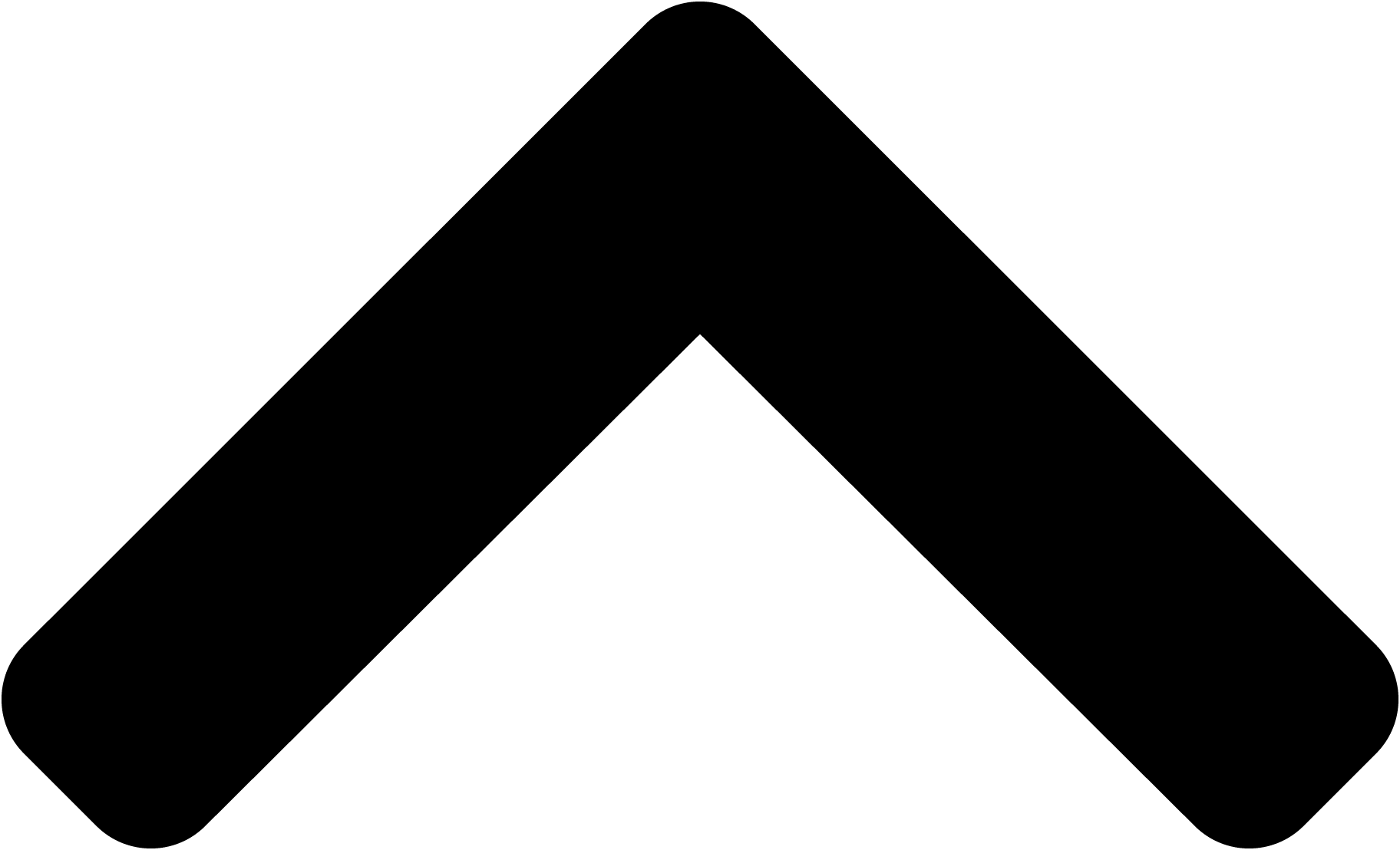
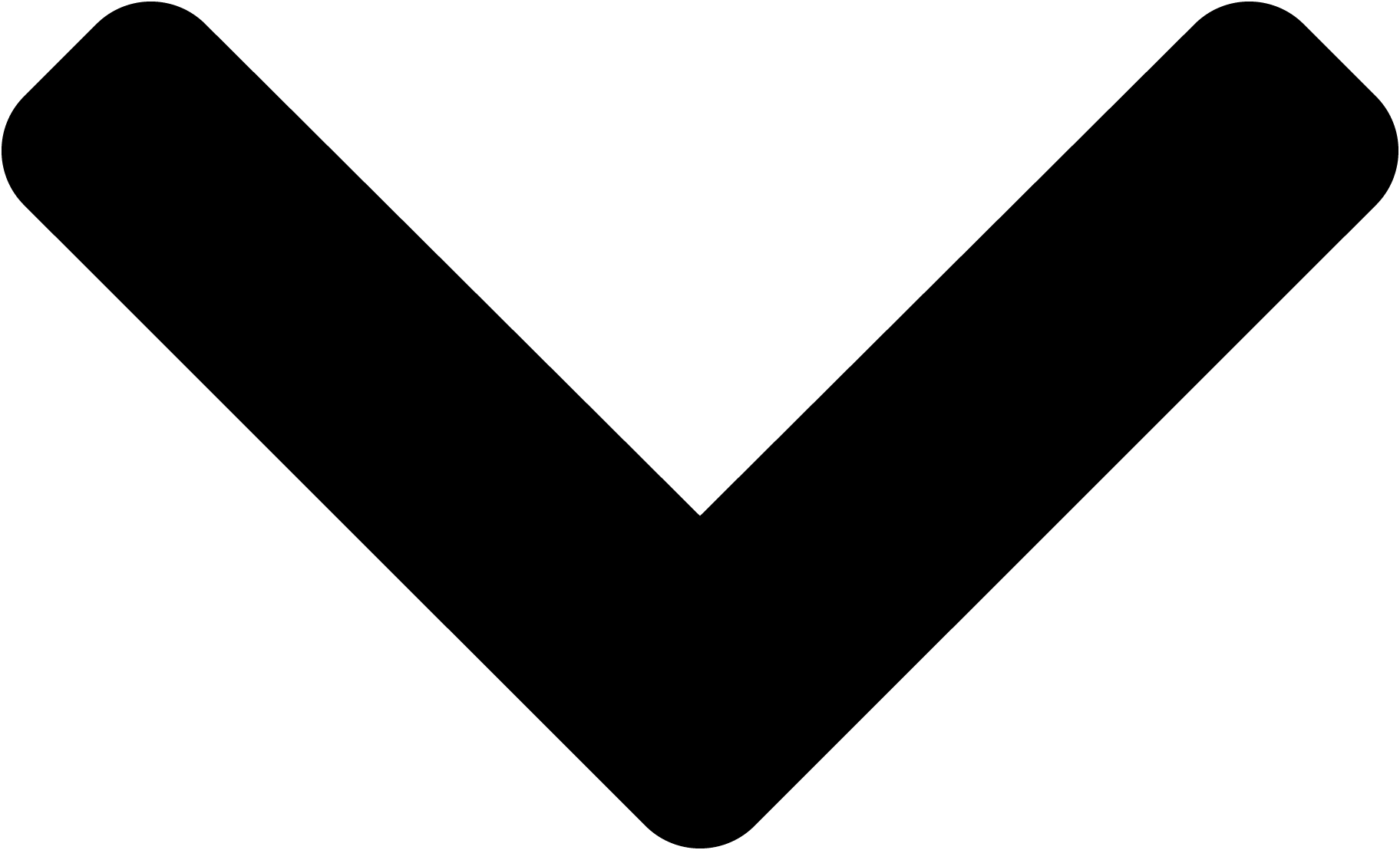
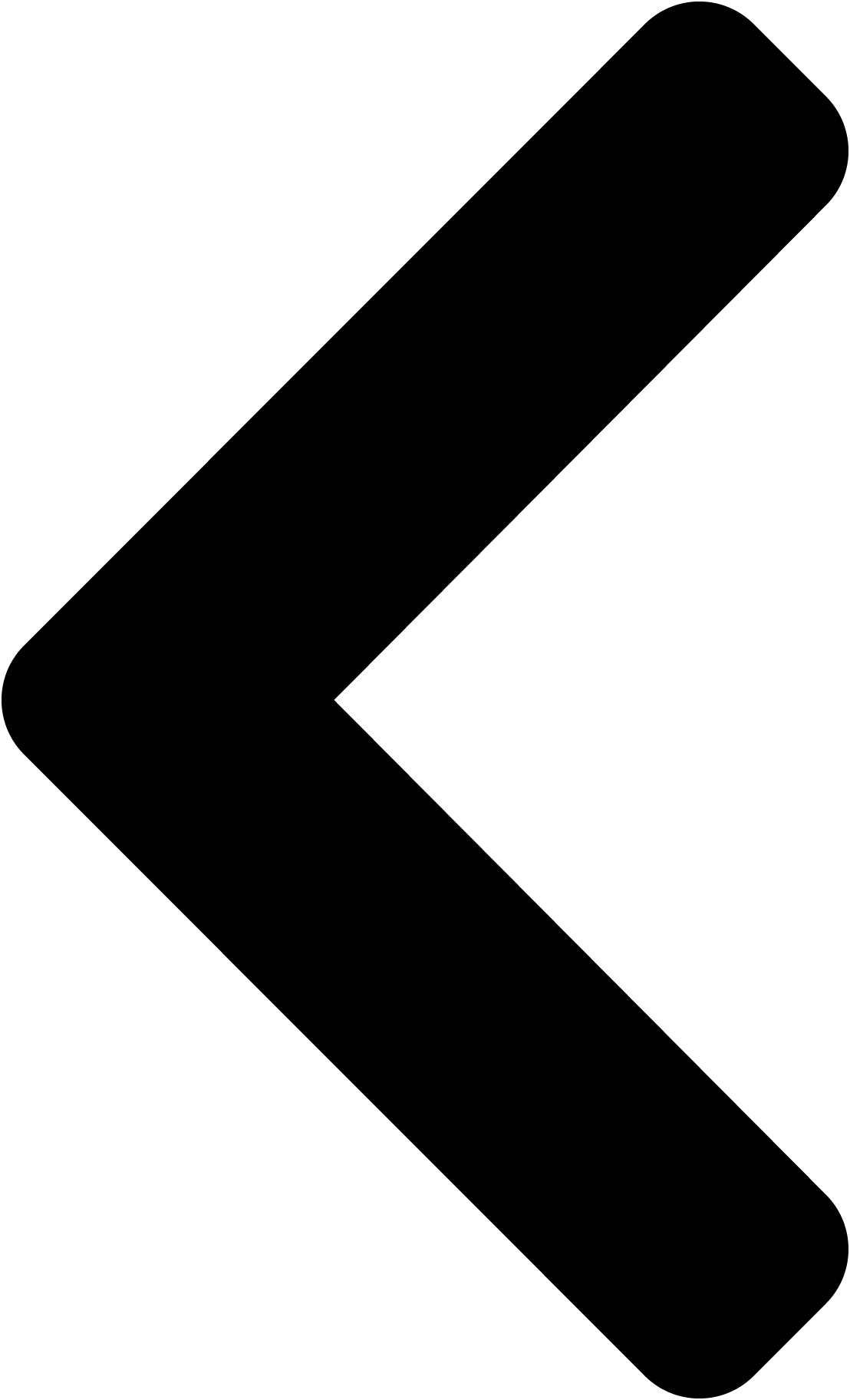
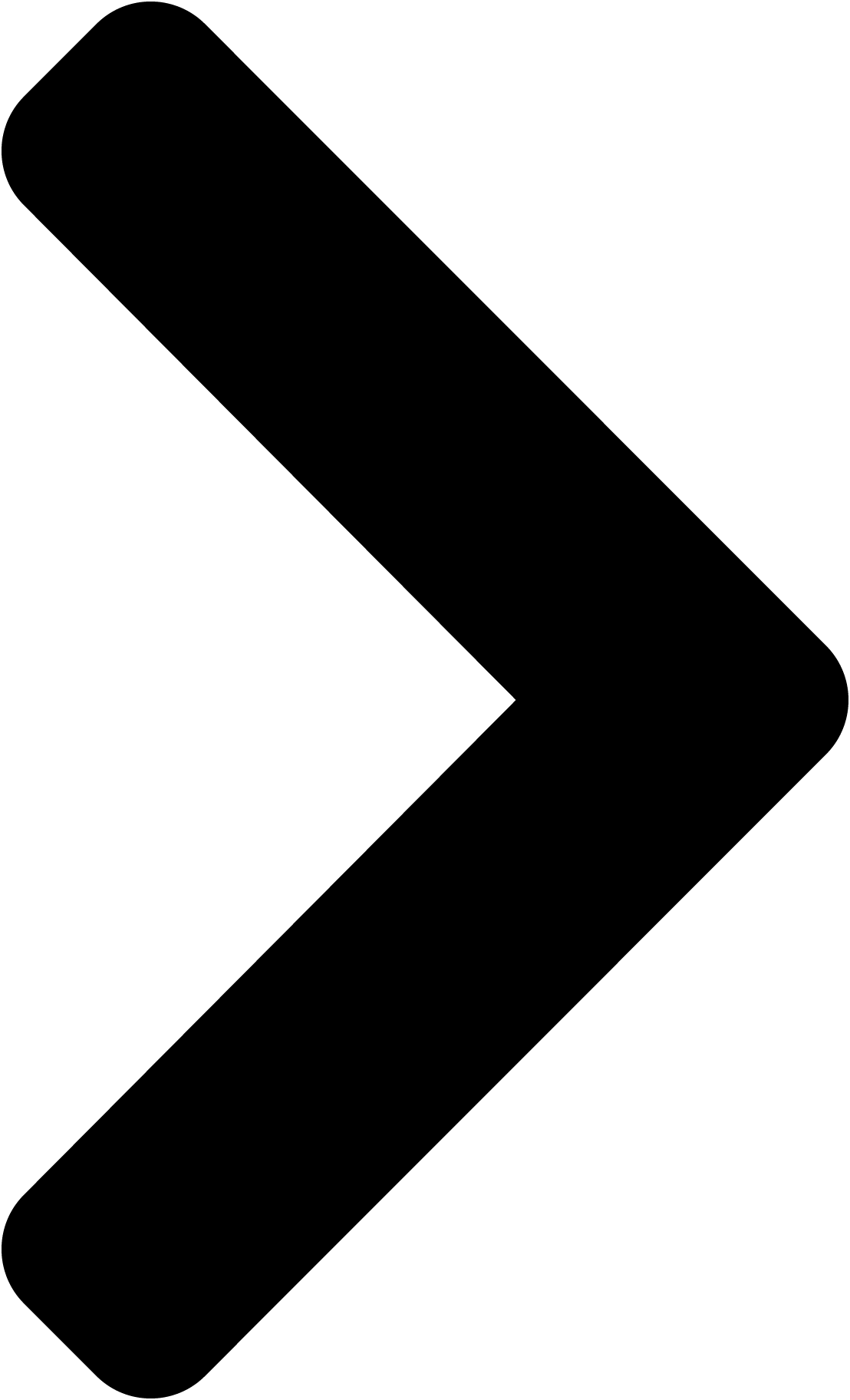
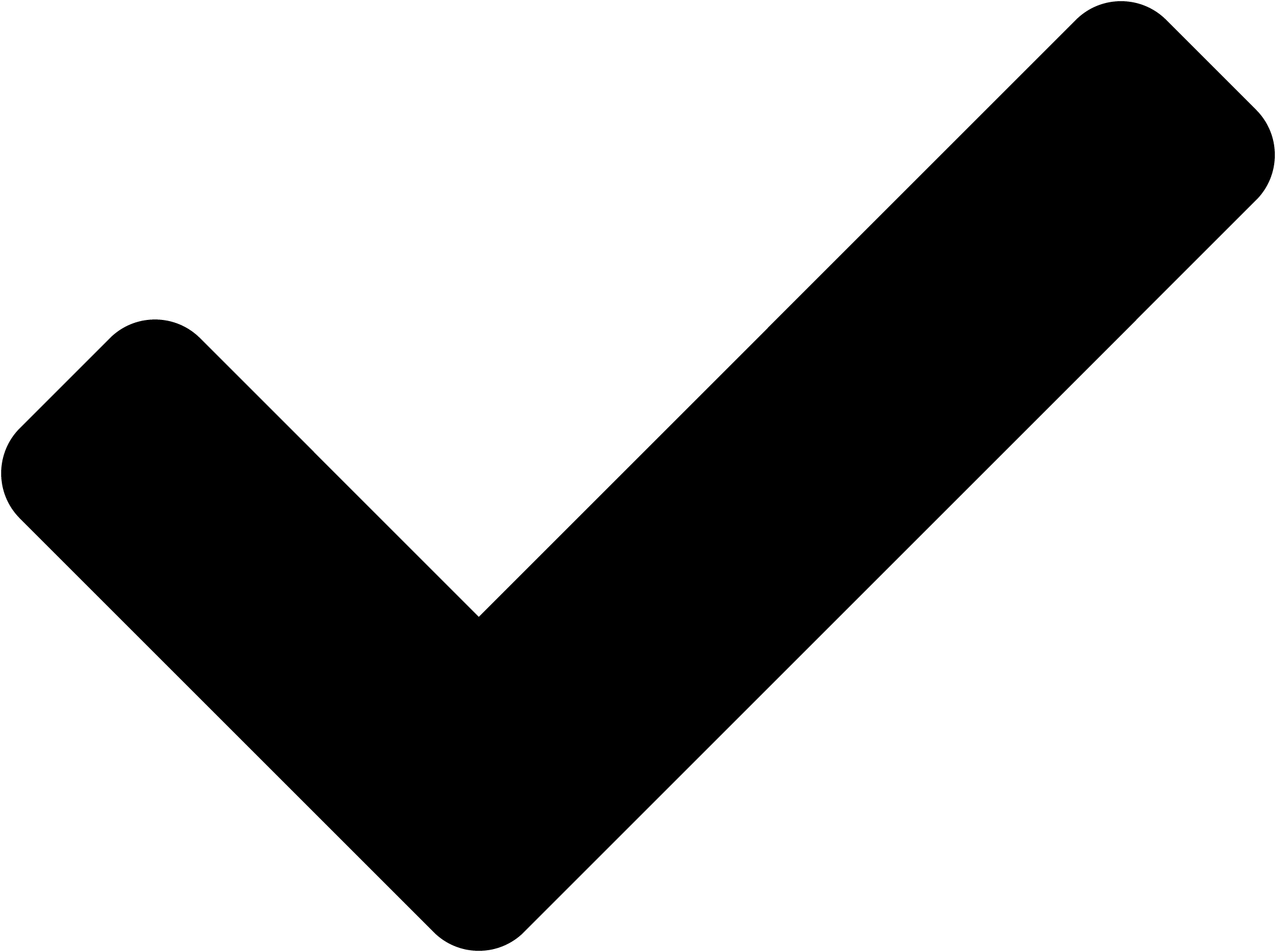
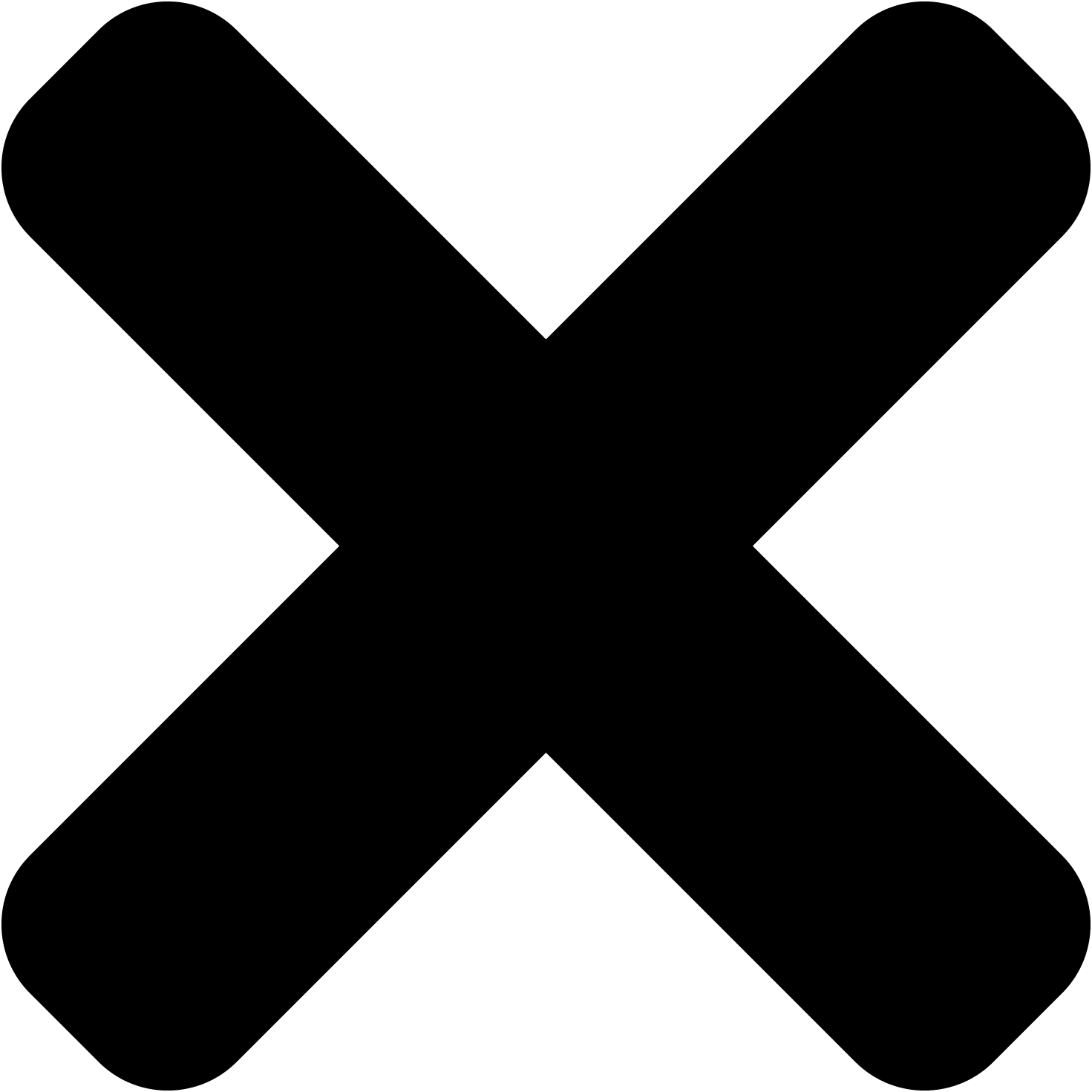
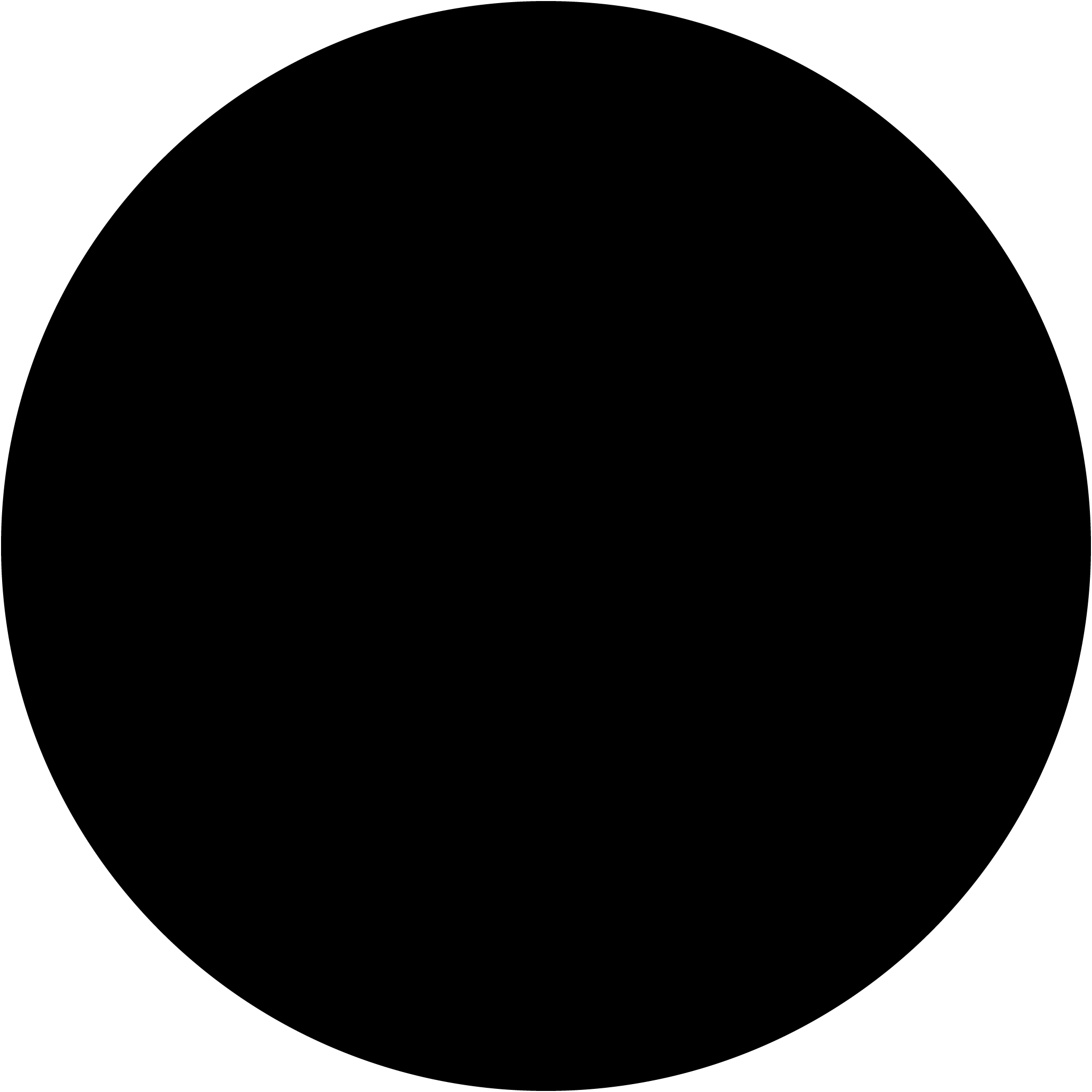
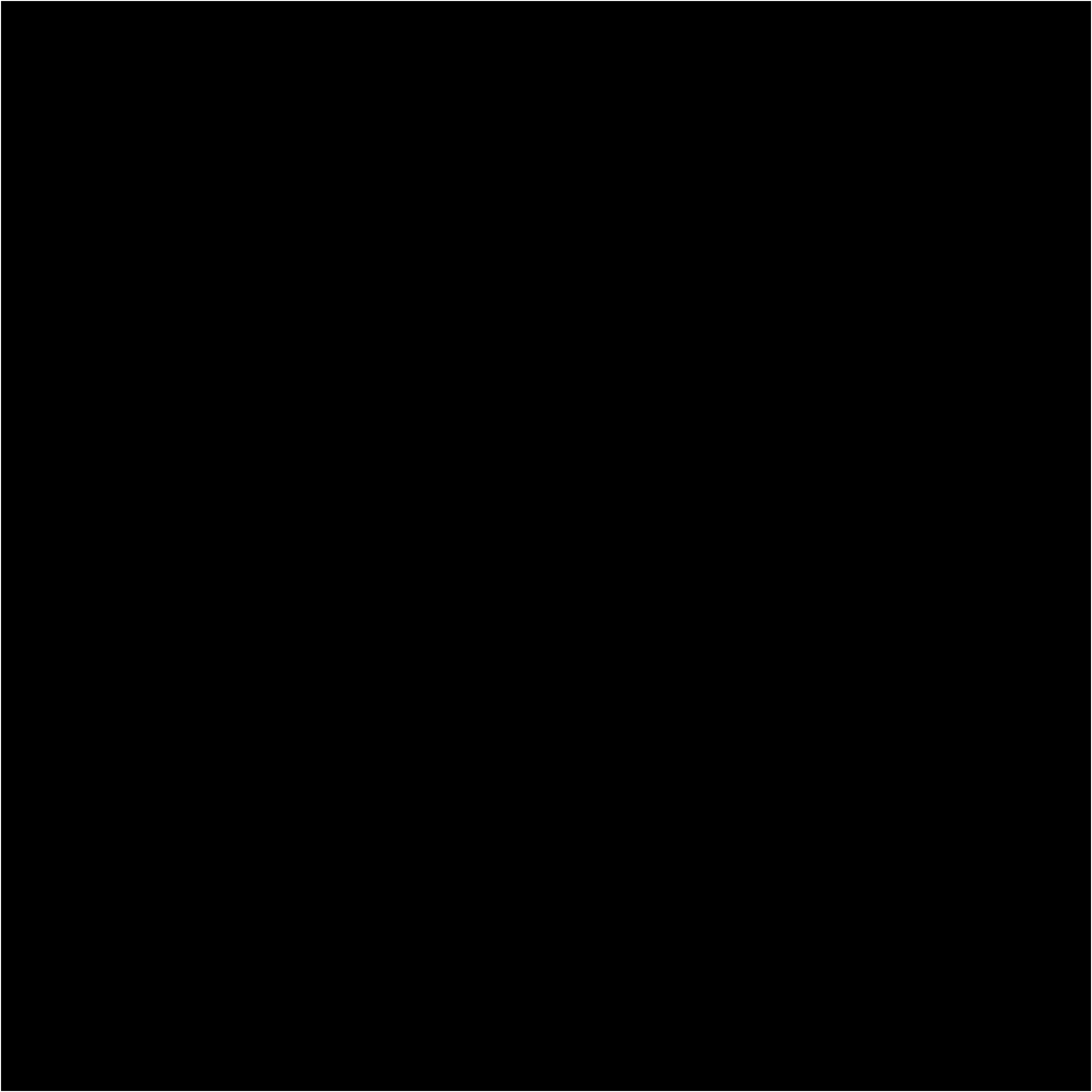
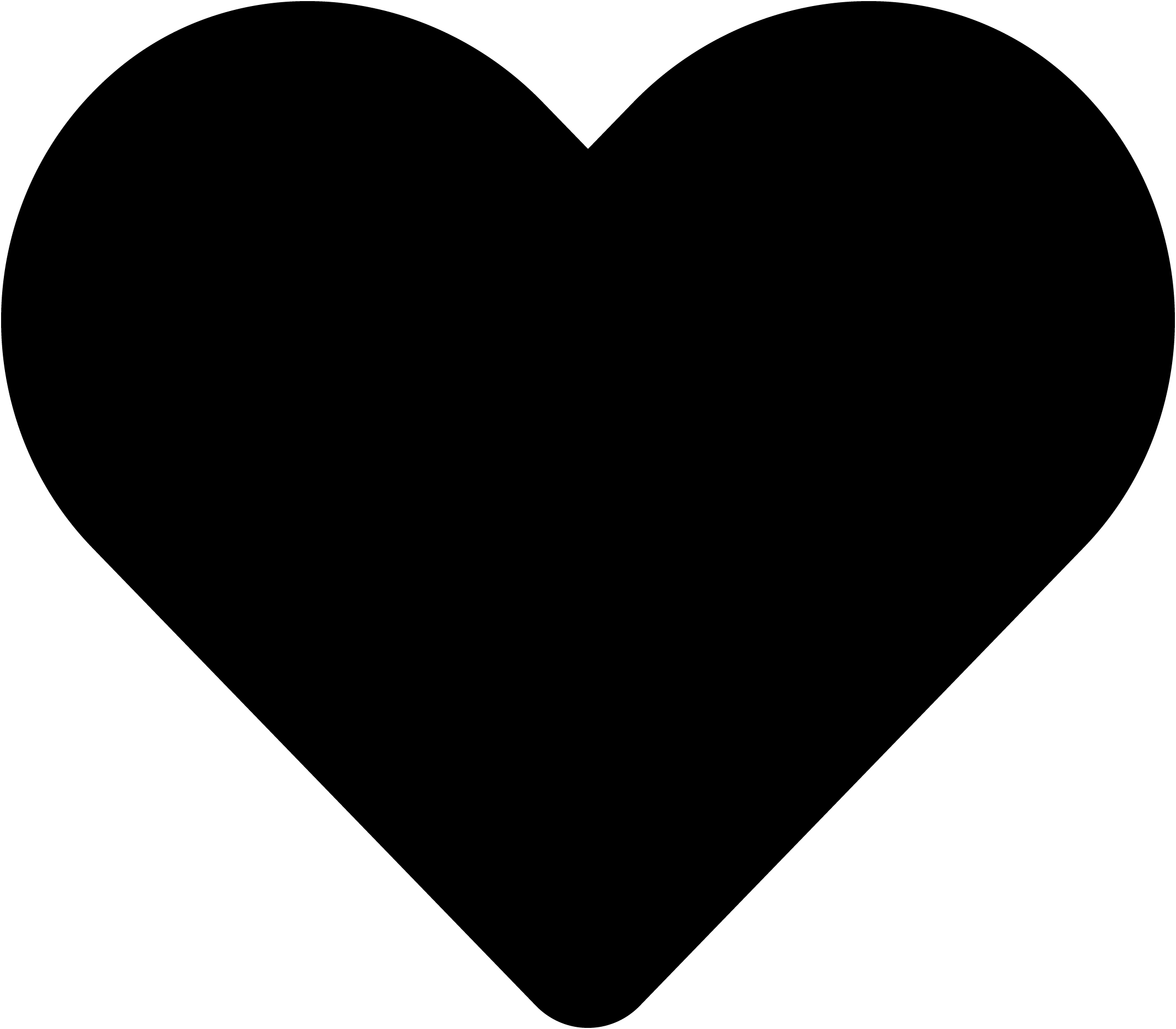
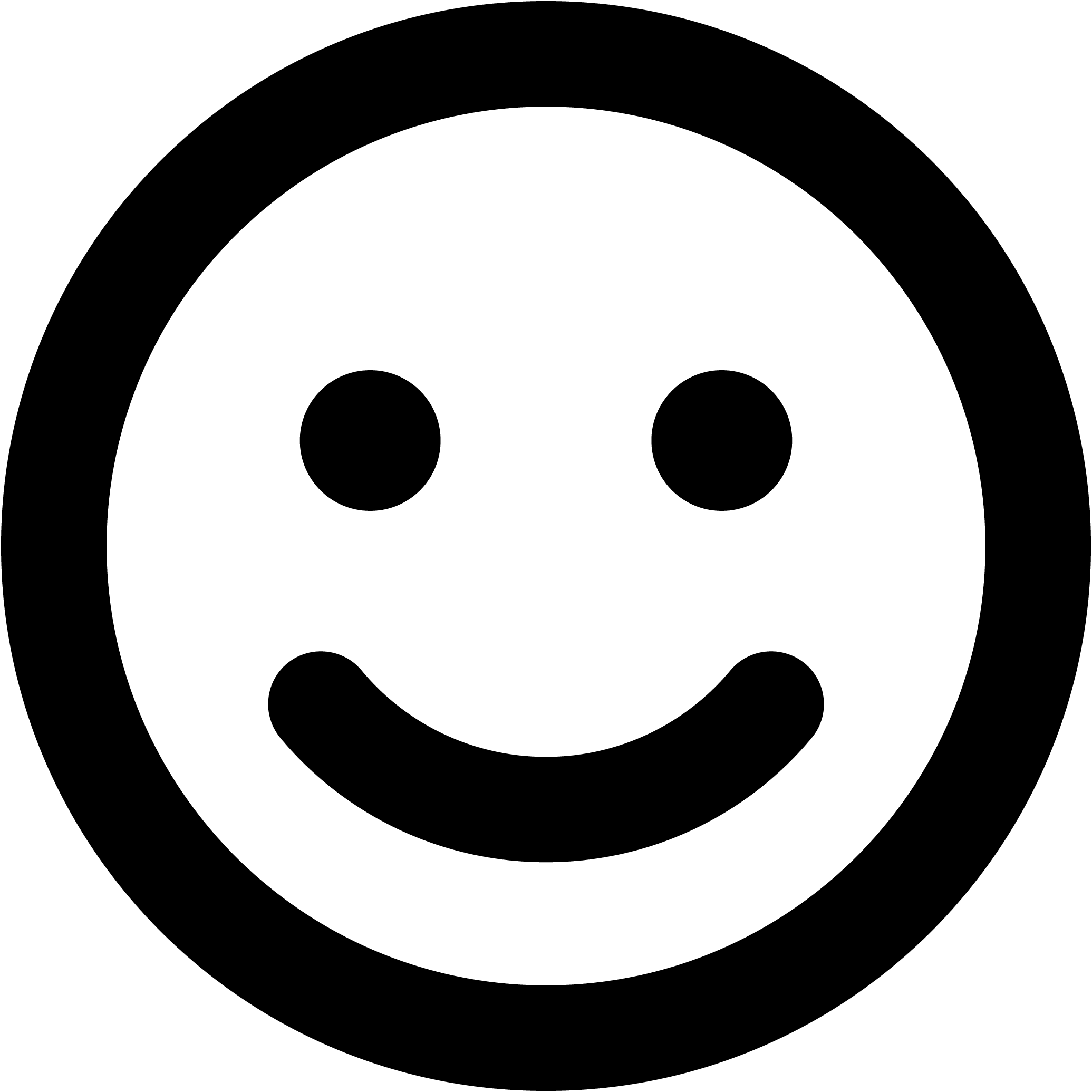
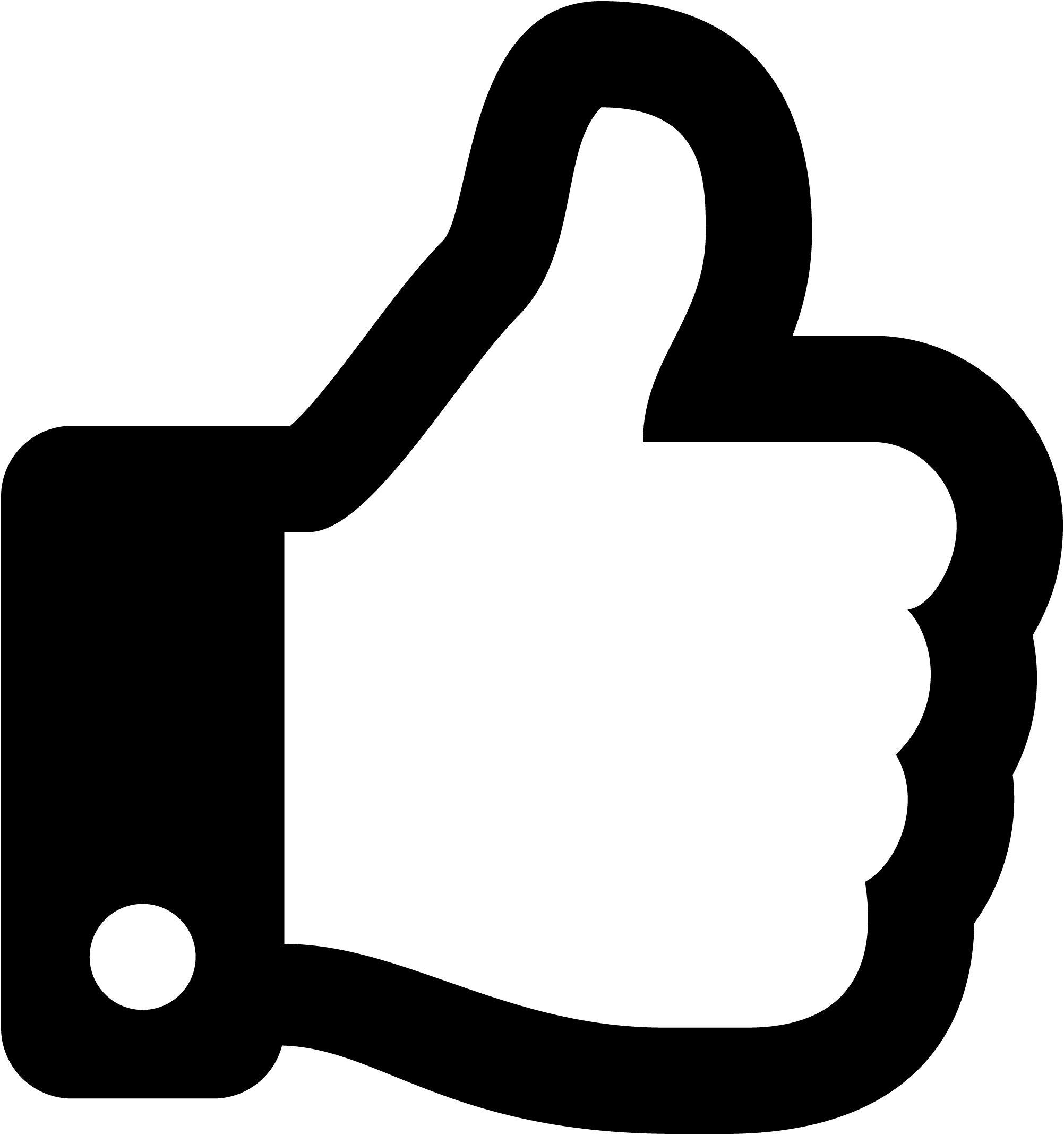
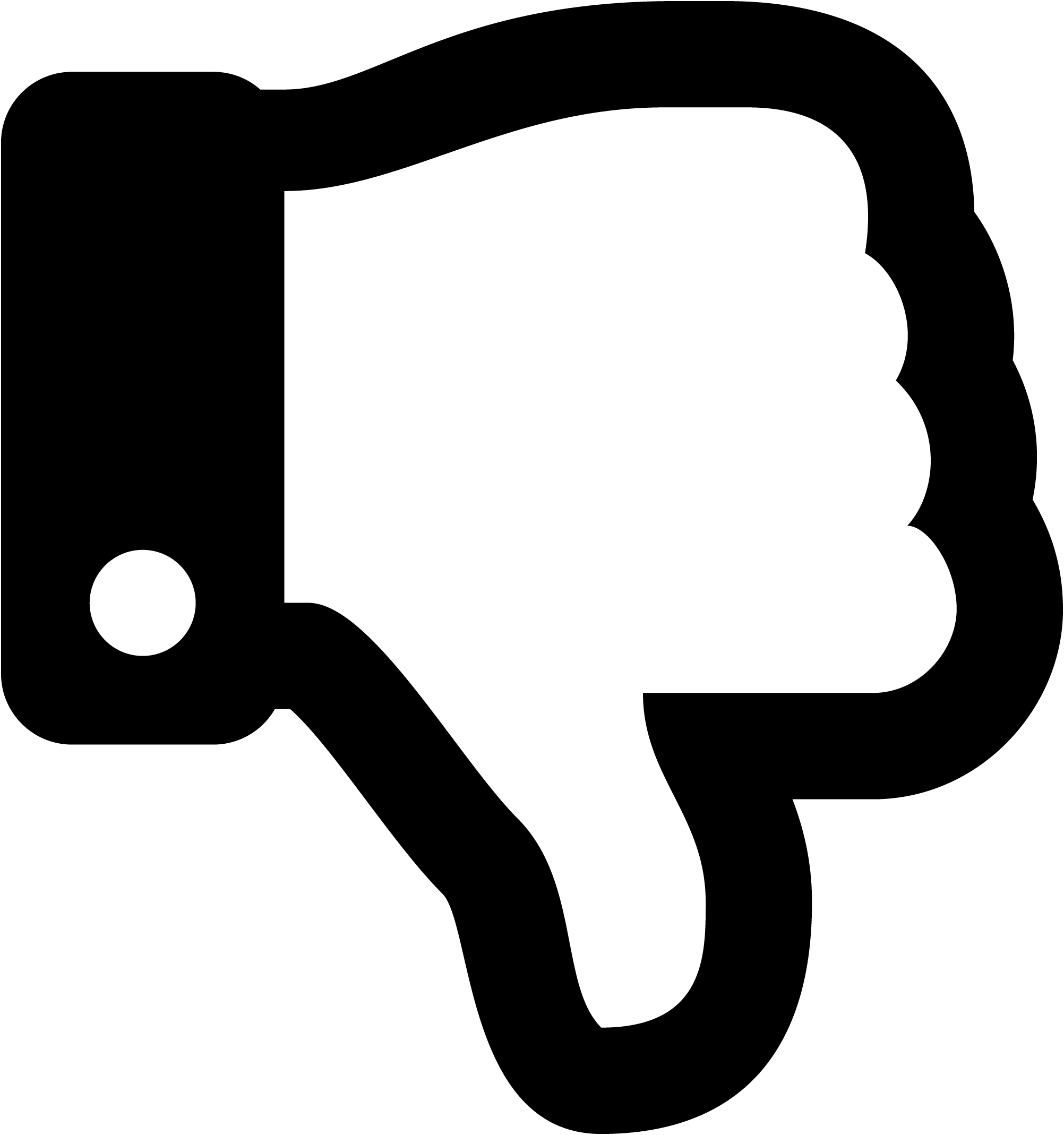
Border colour
Rotate
Skew (x-axis)
Skew (y-axis)
Image (max size: 2mb)
Or drag a symbol into the upload area
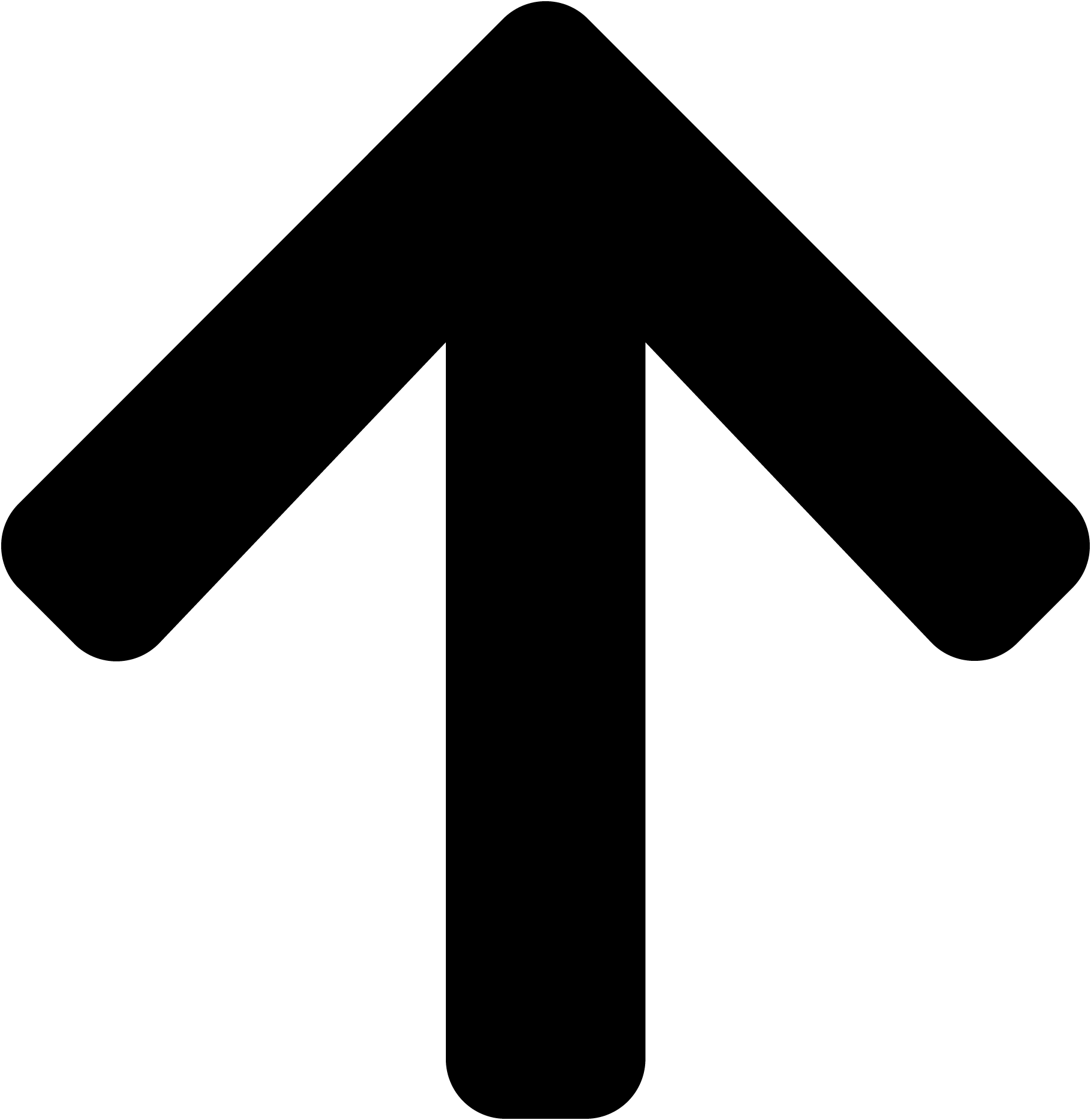
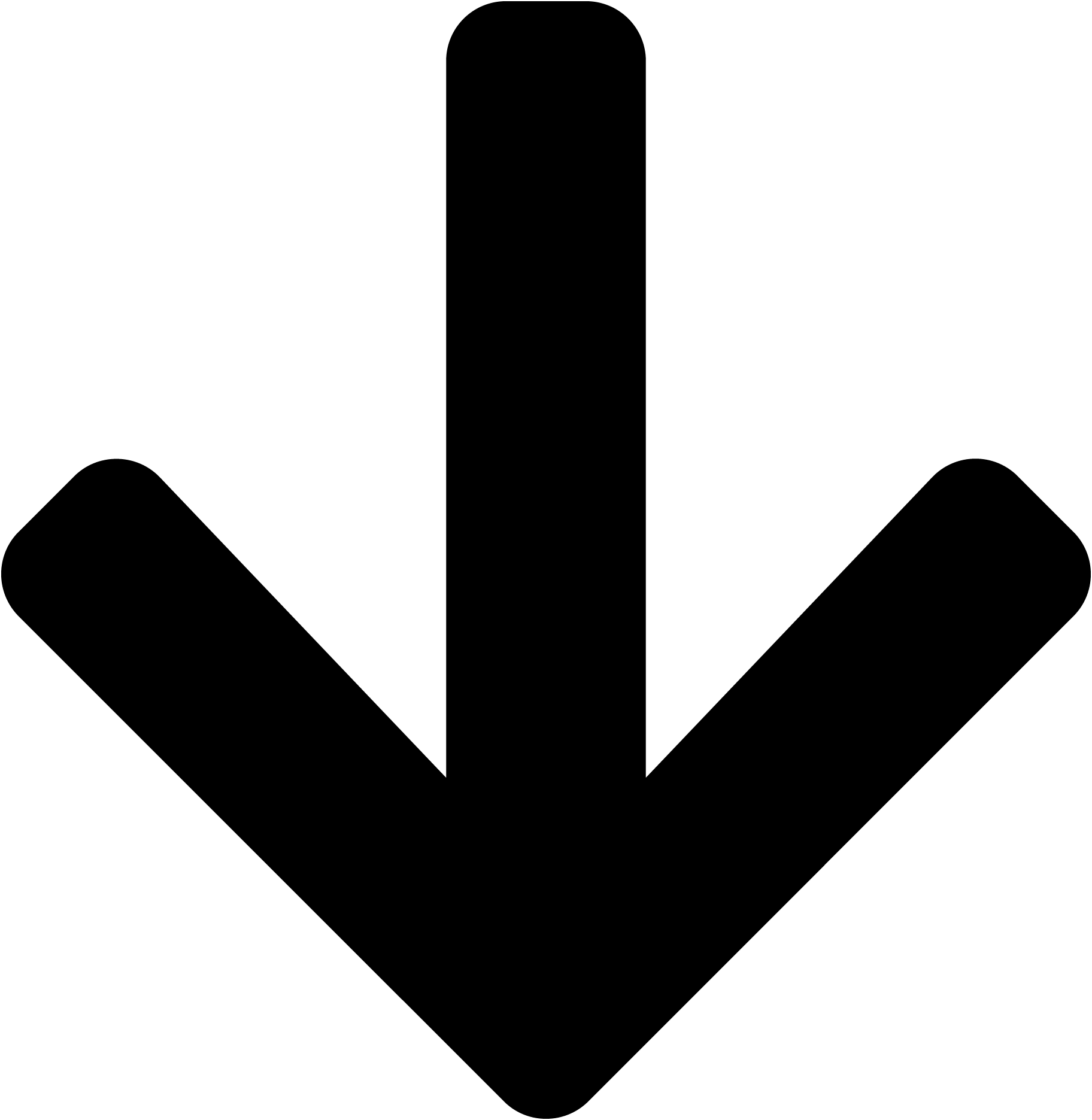
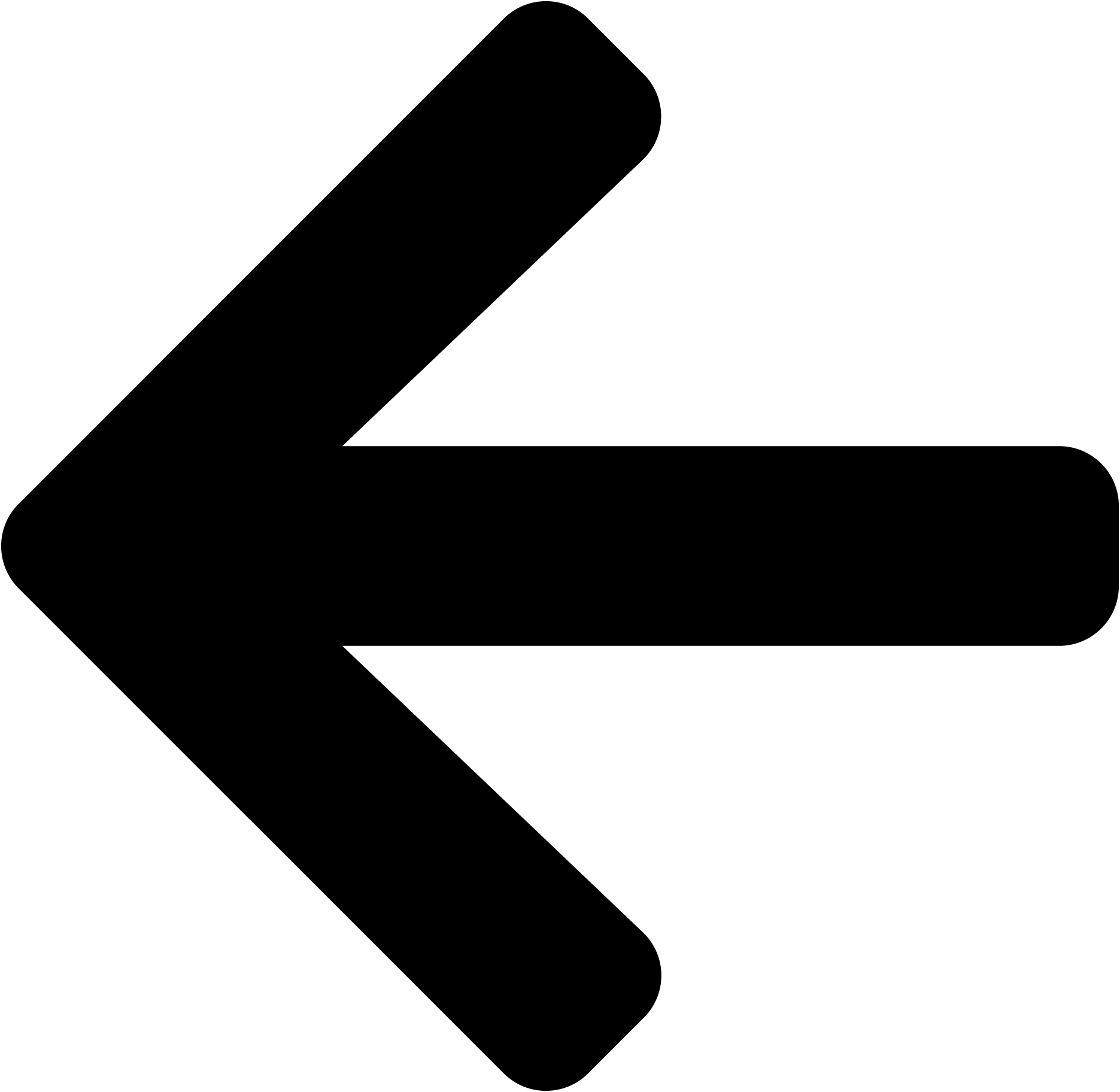
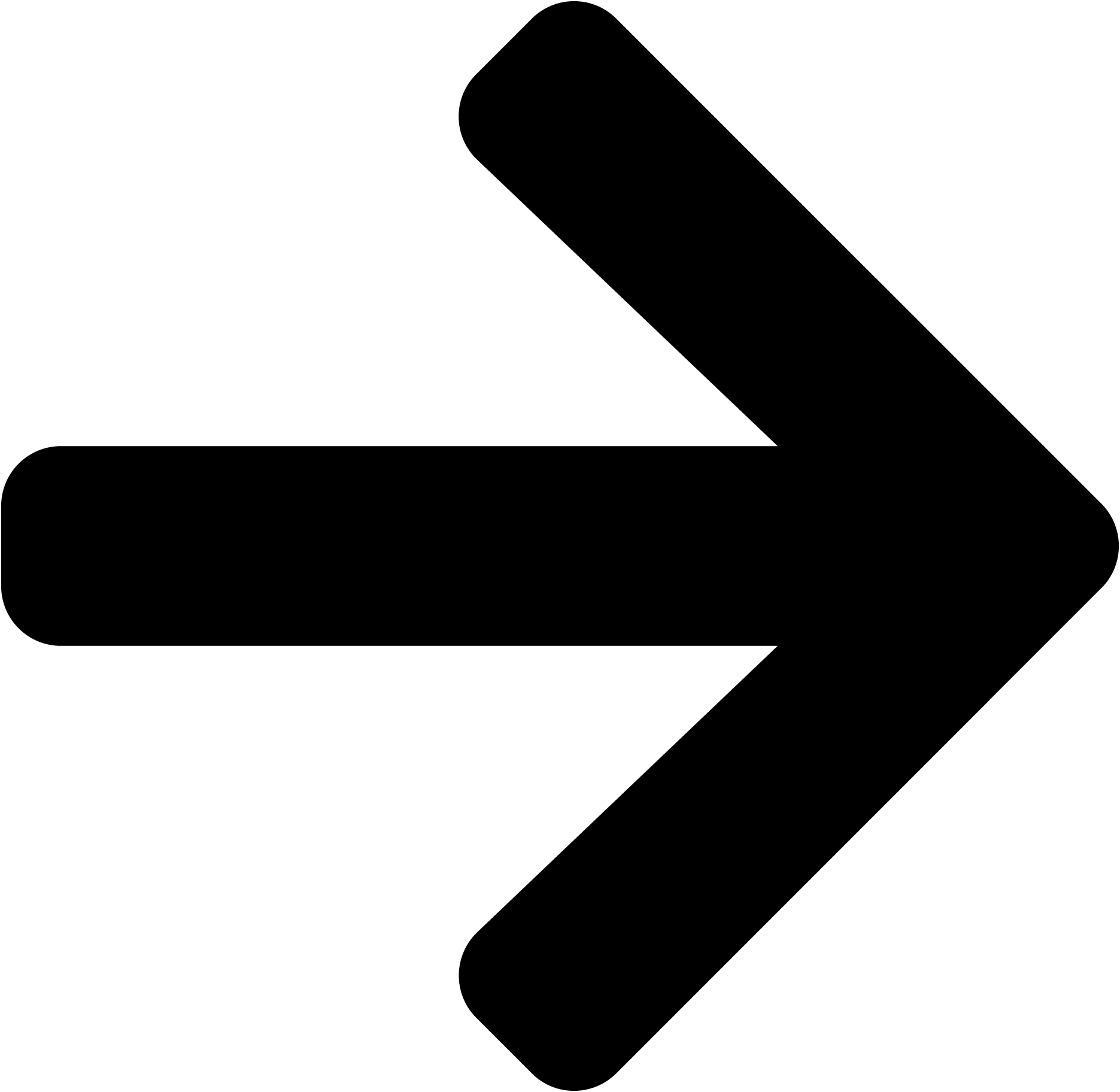
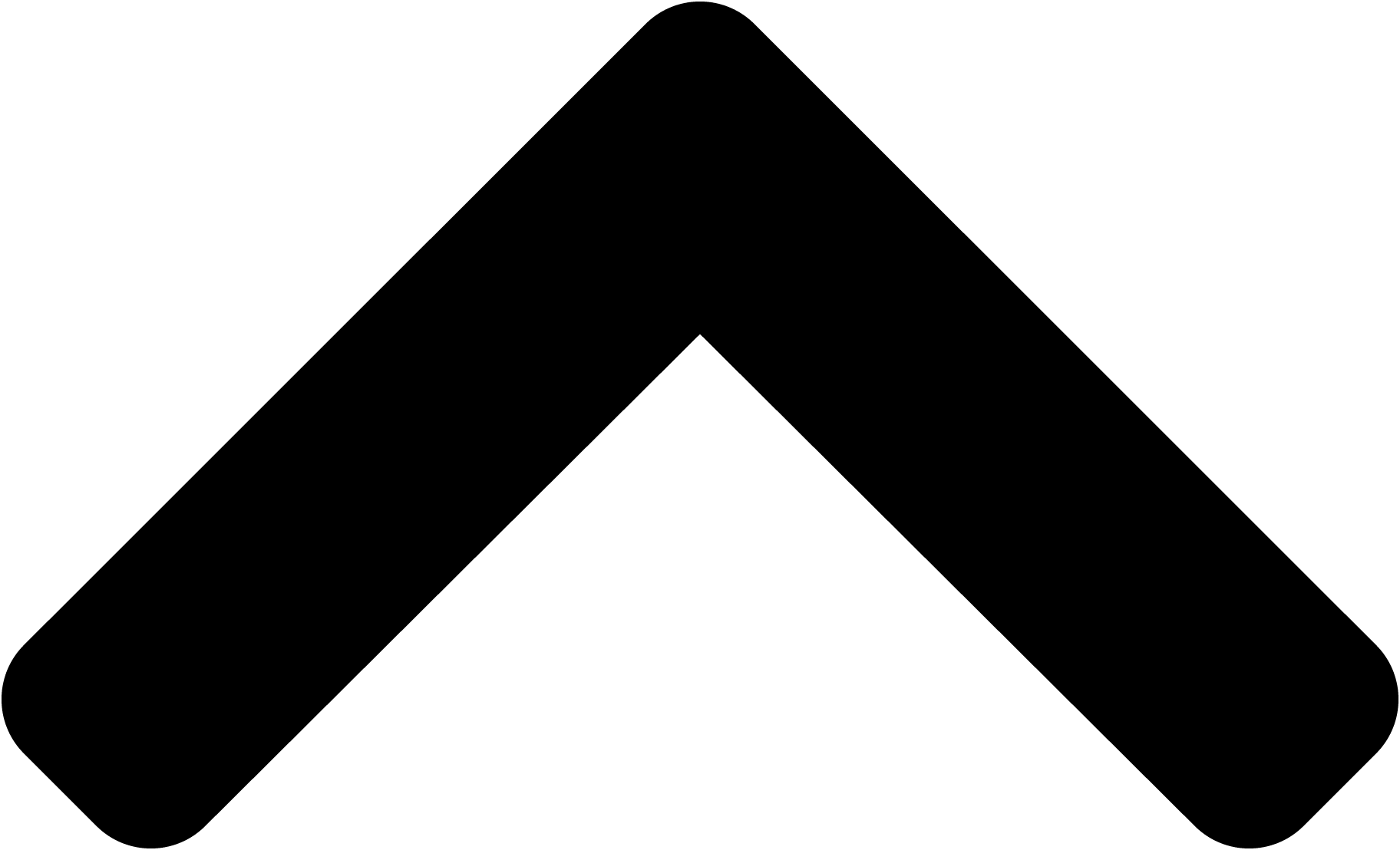
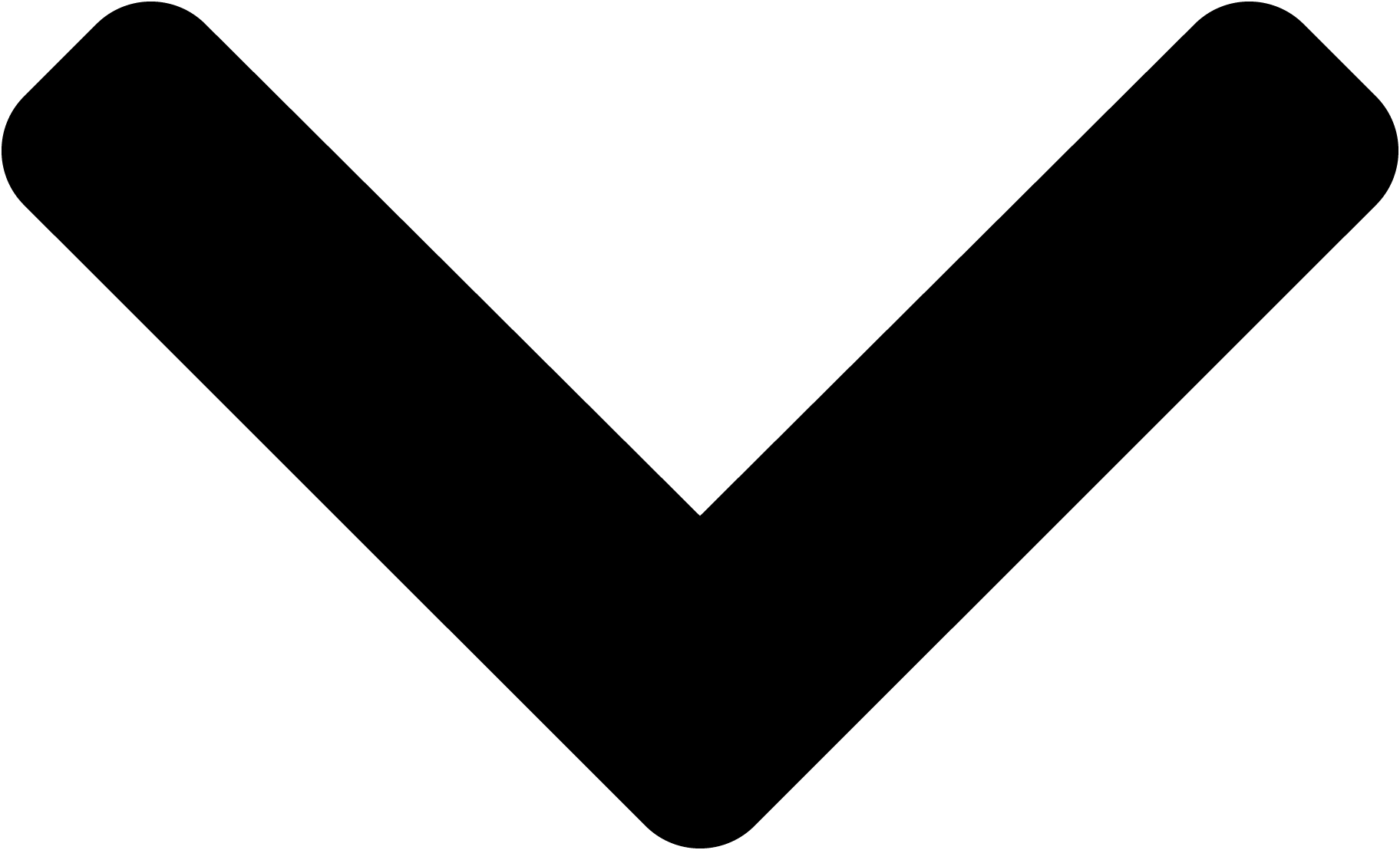
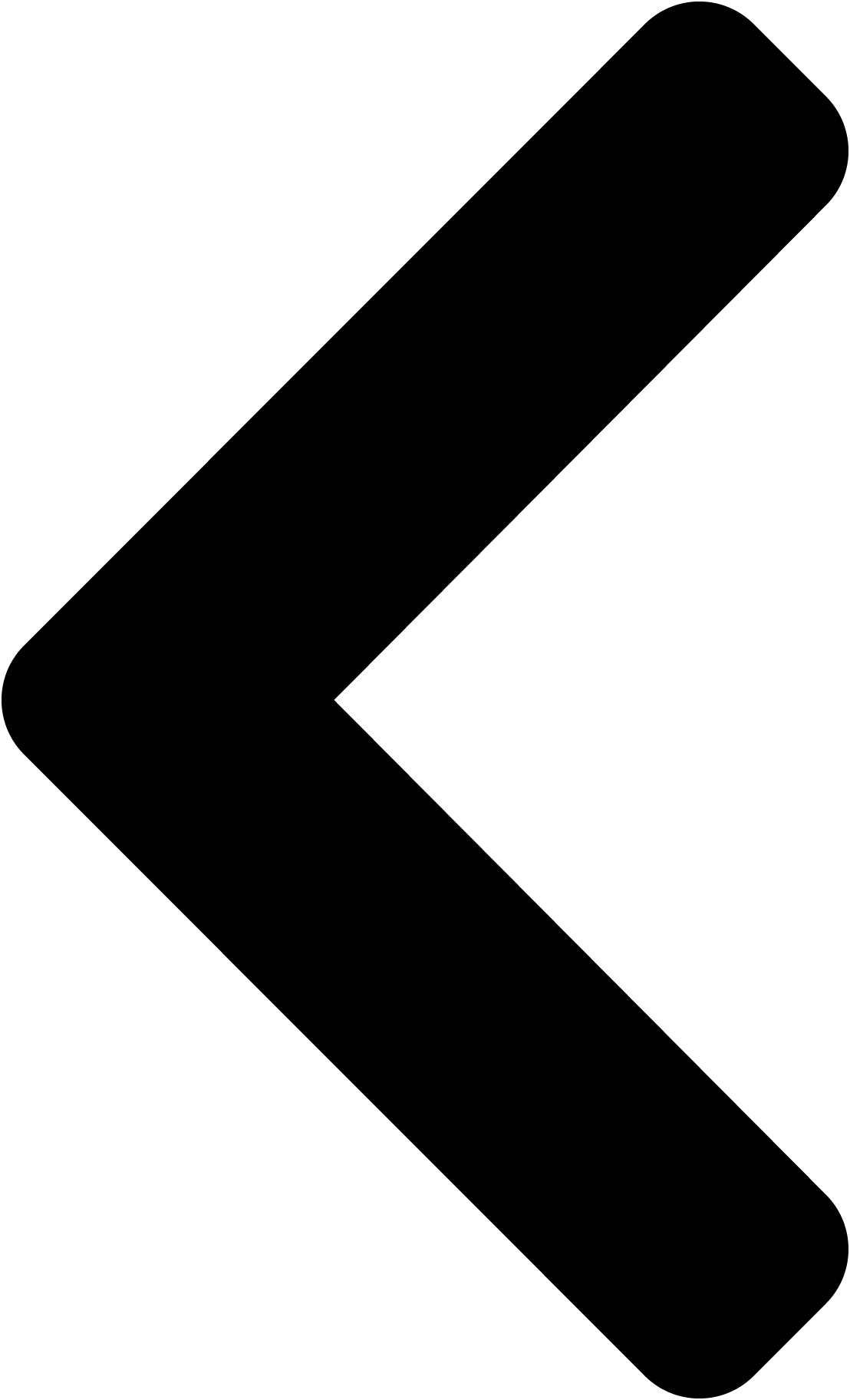
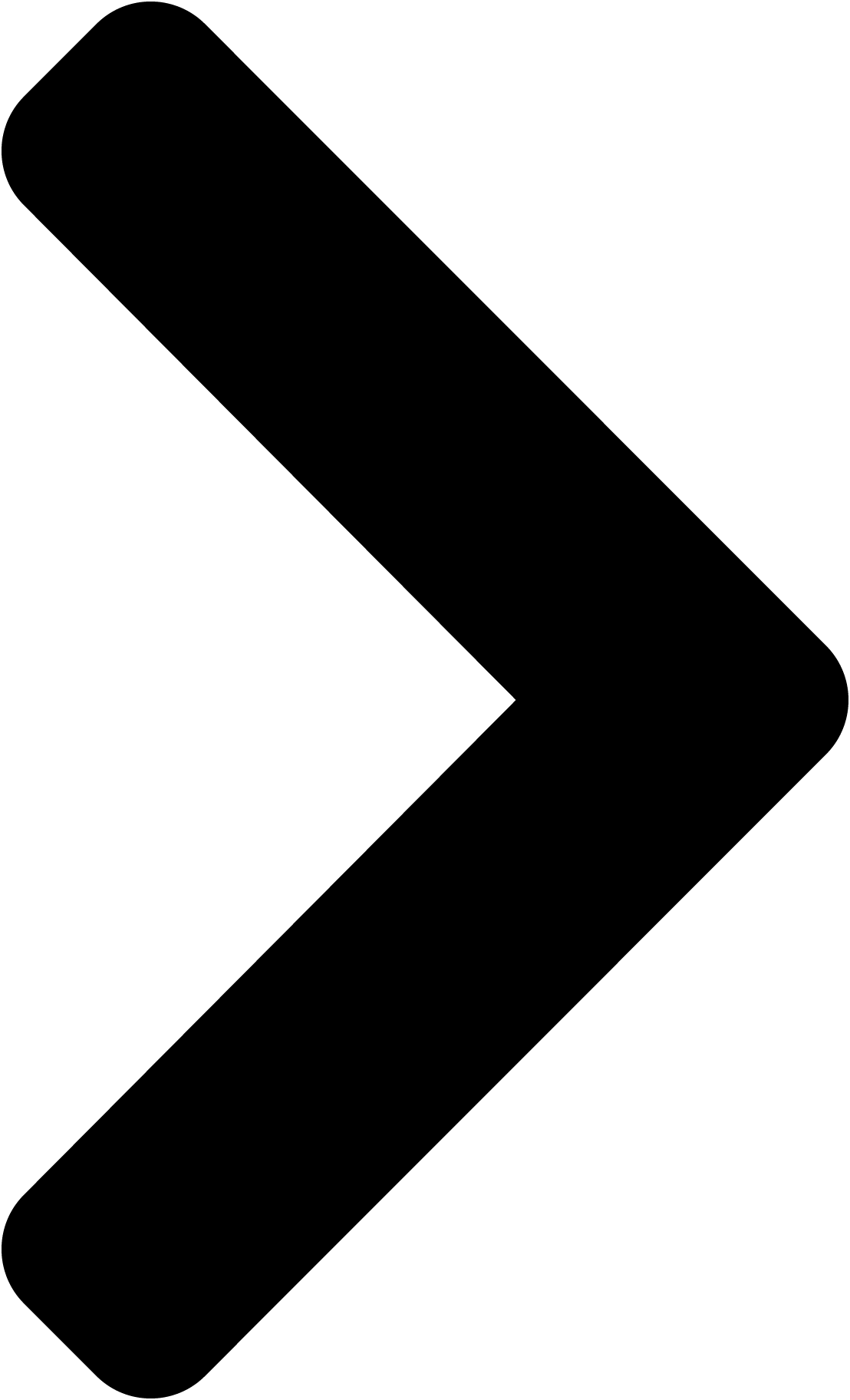
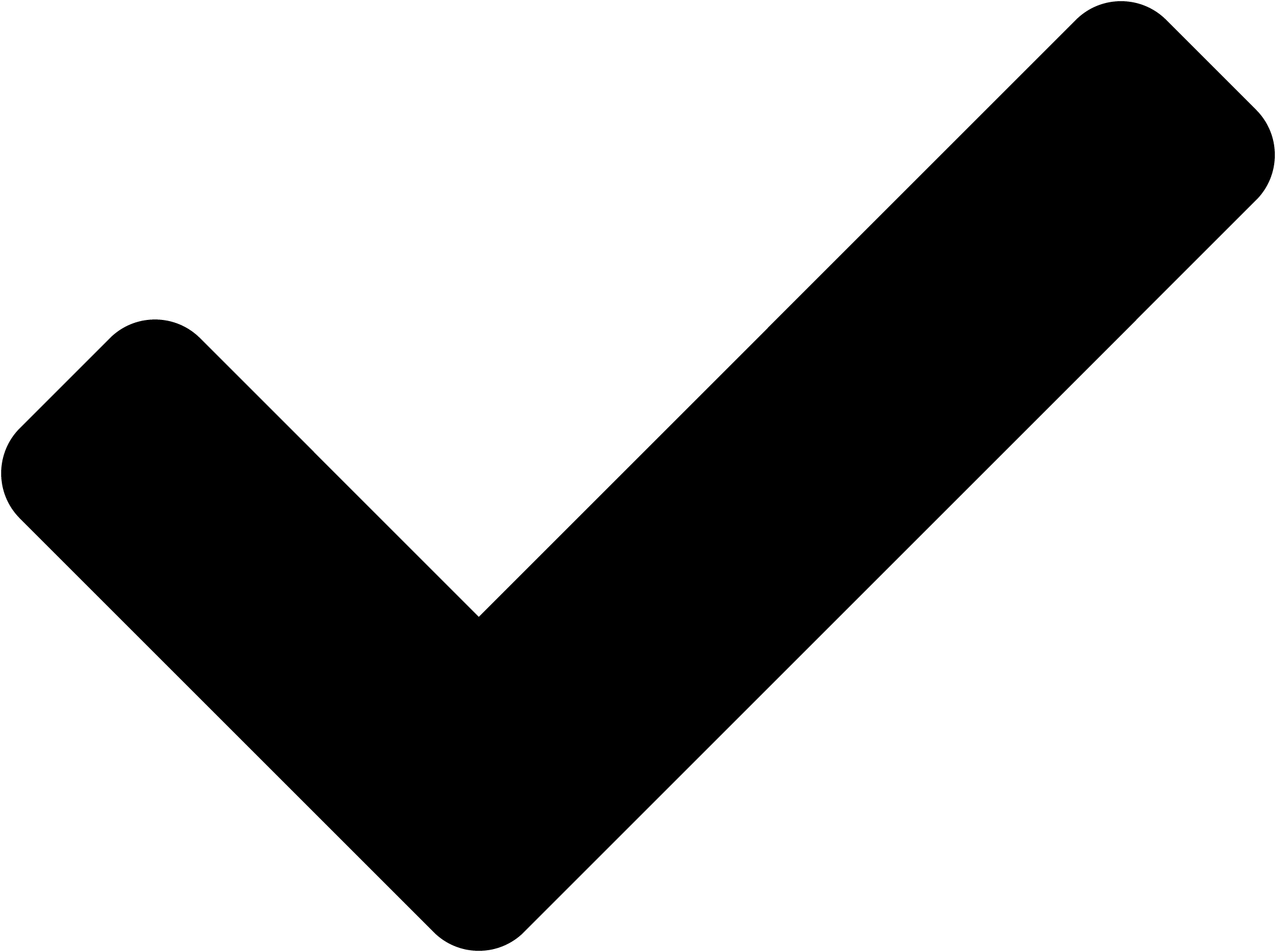
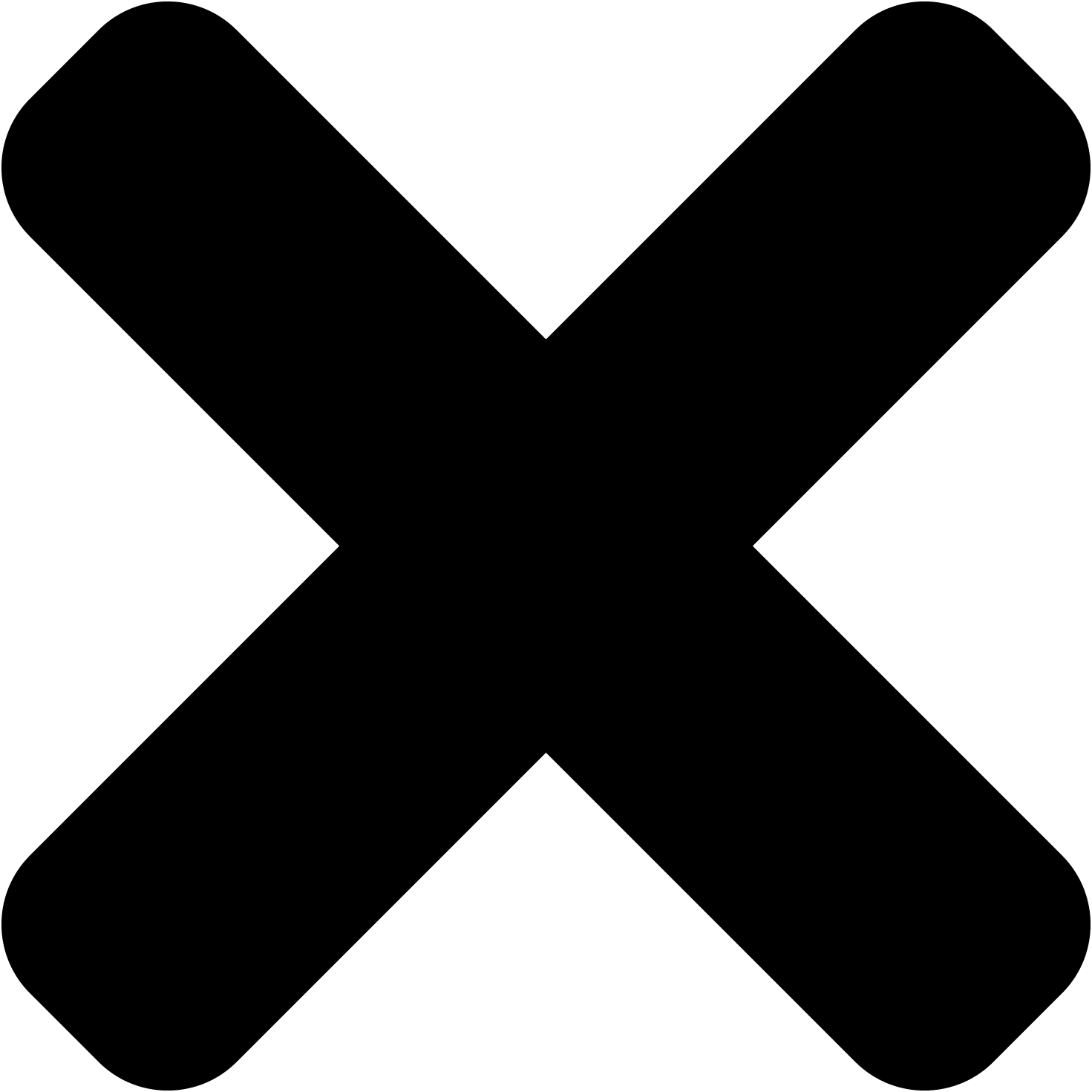
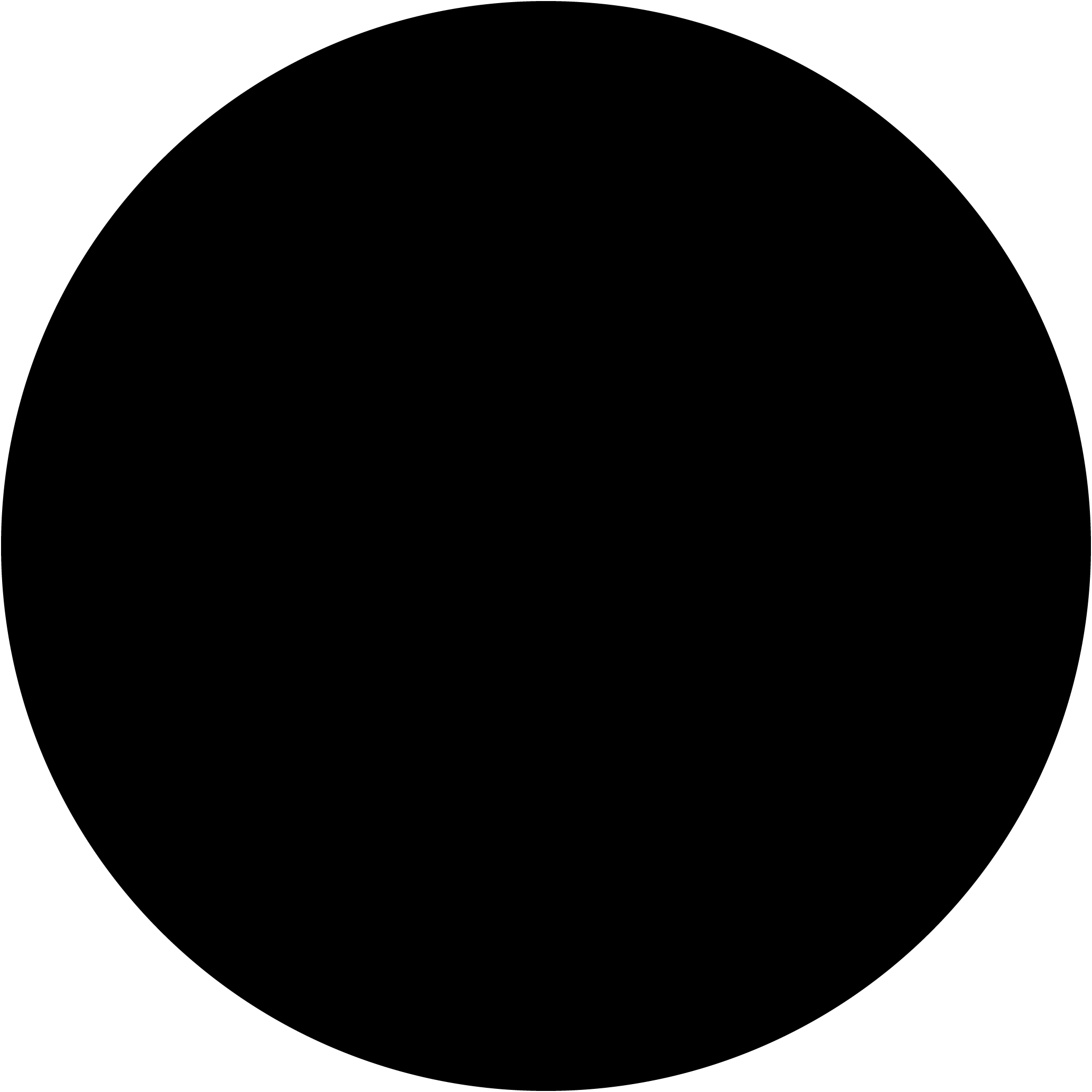
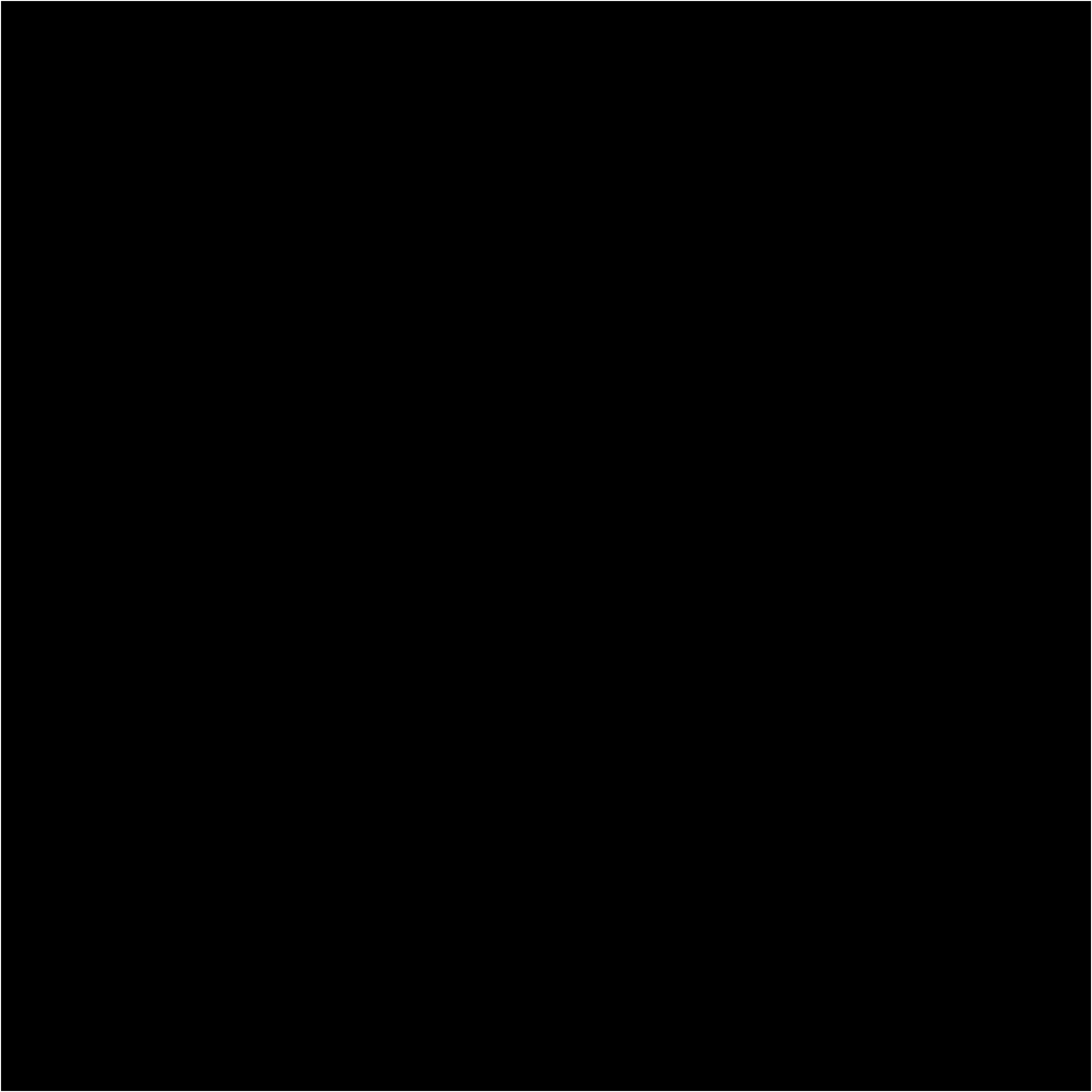
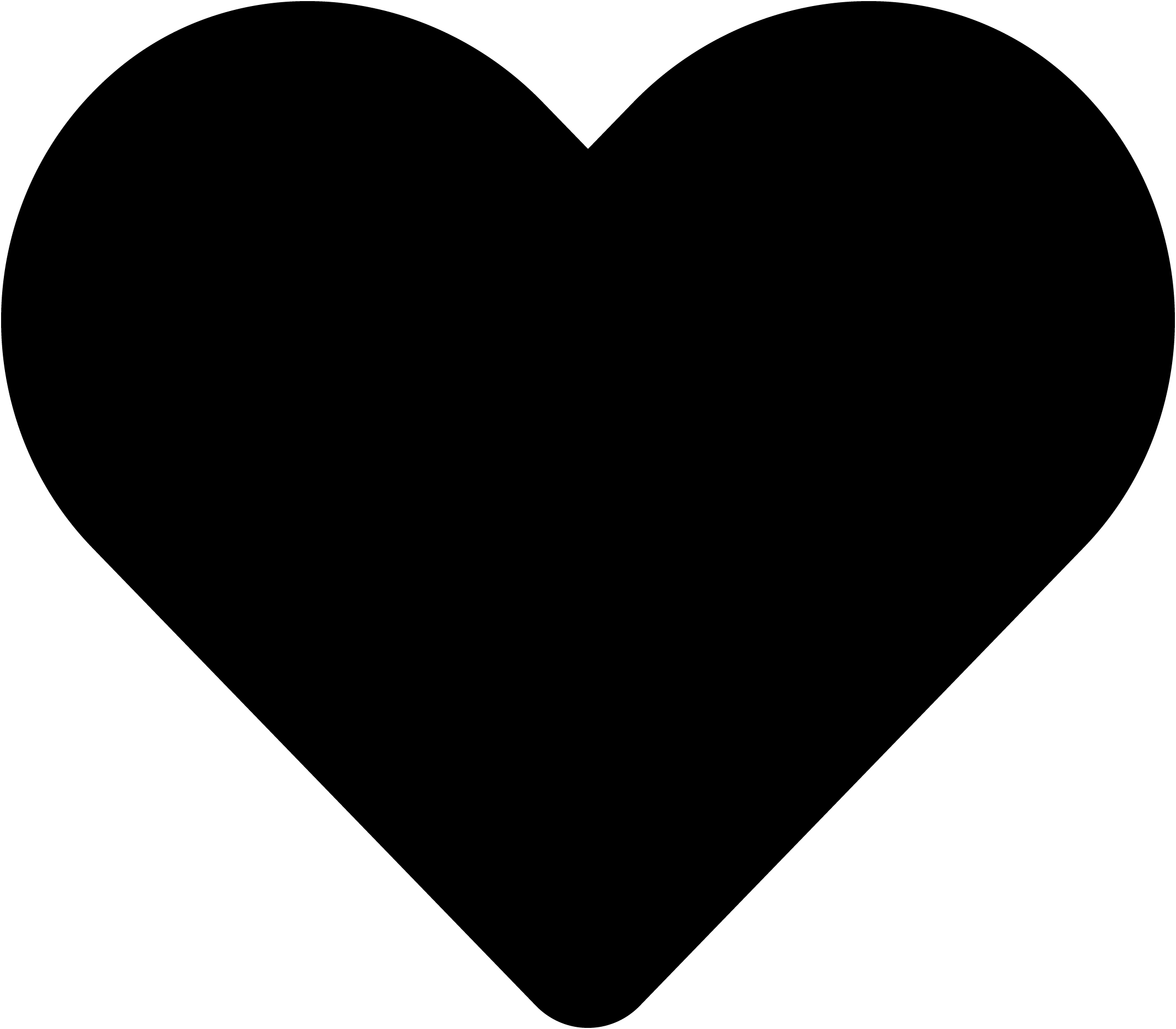
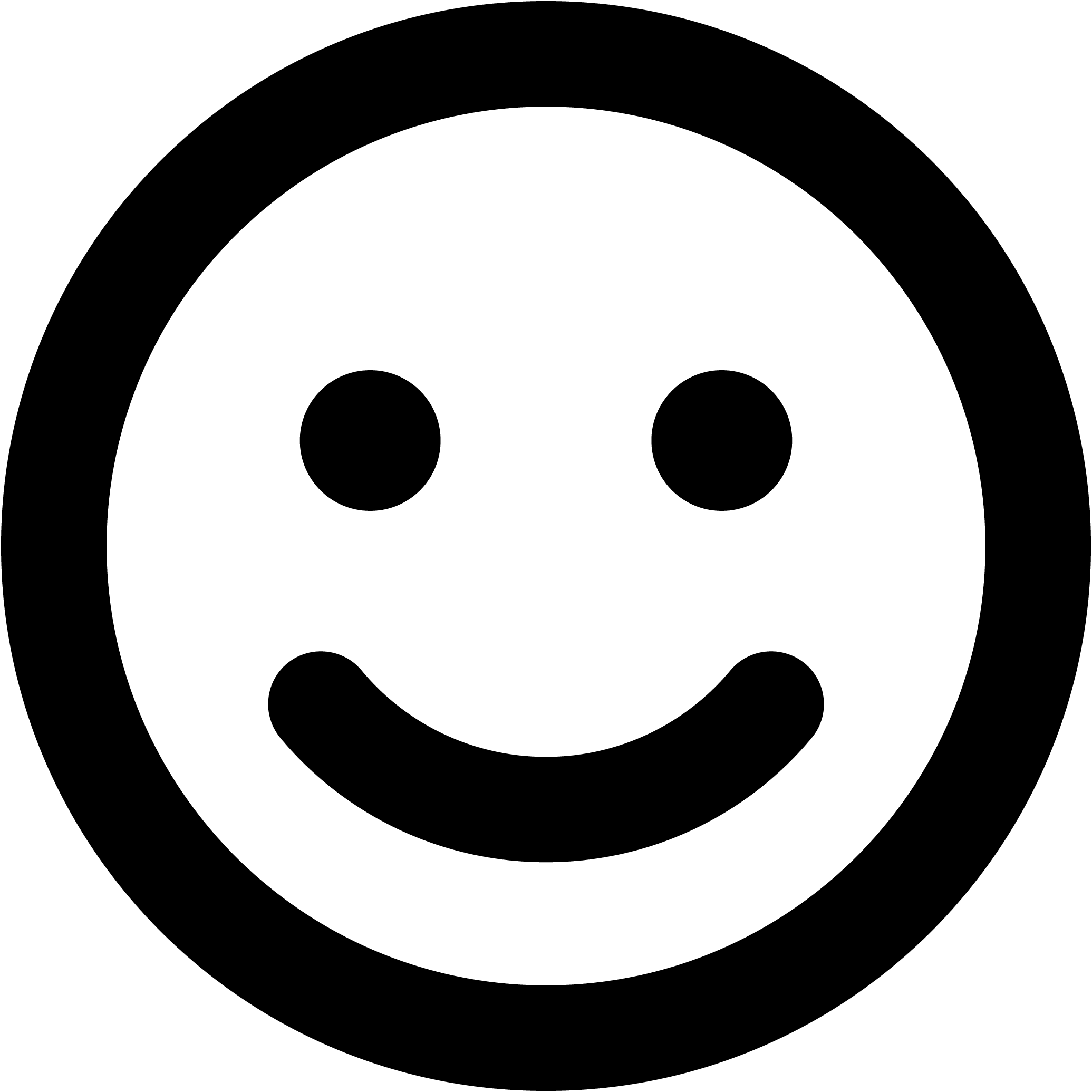
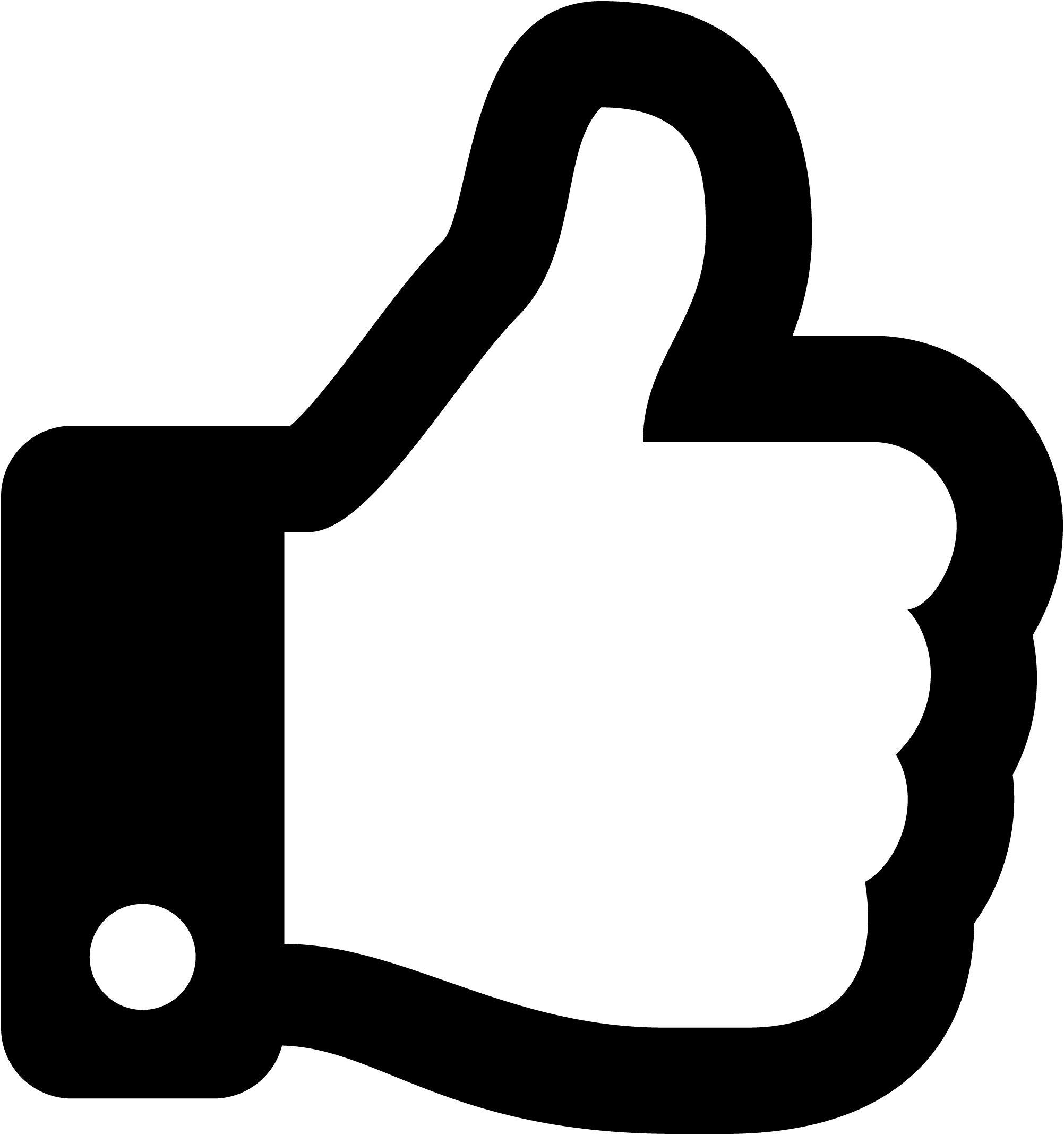
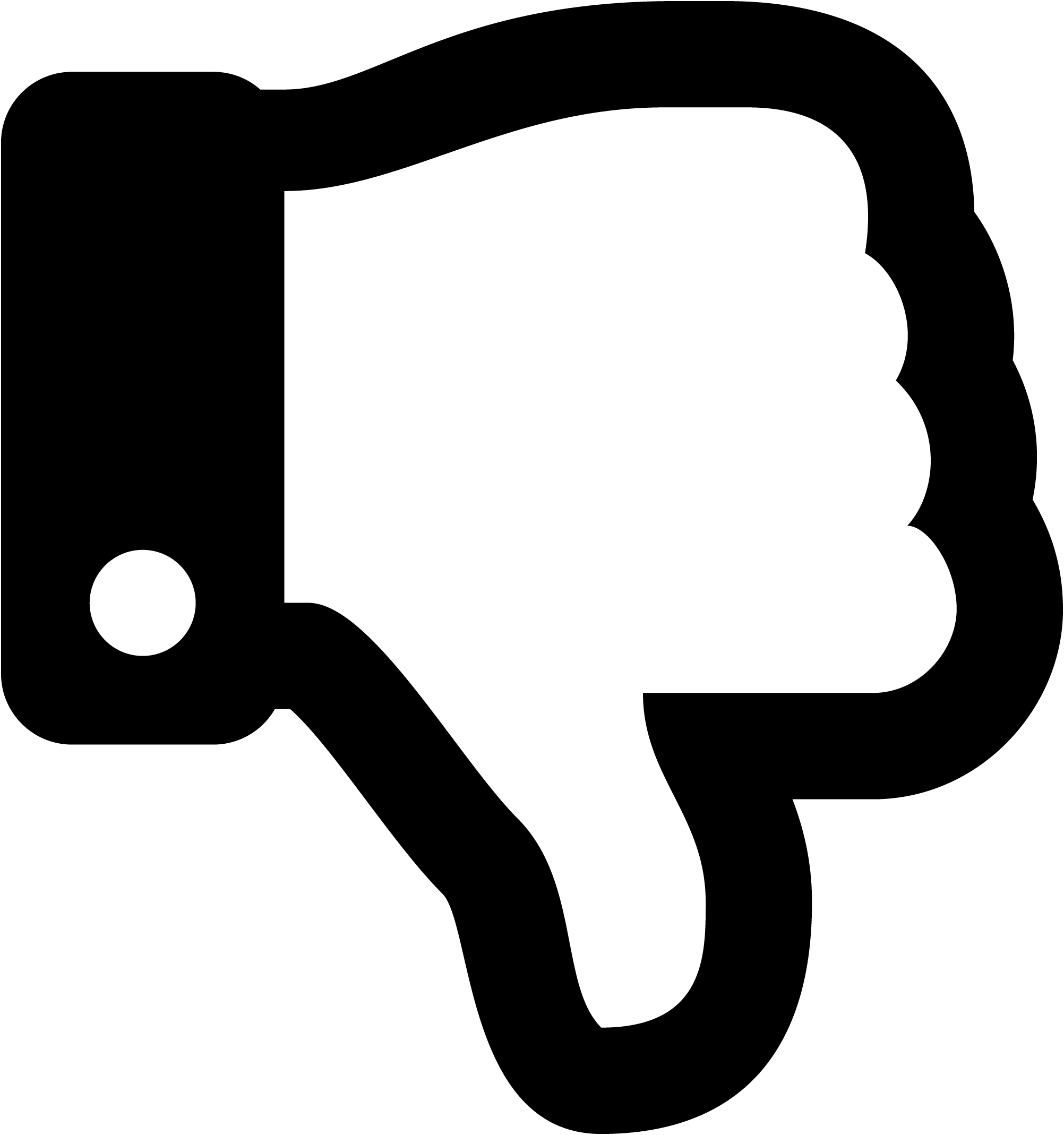
Image description/alt-tag
Image caption
Image link
Rollover Image (max size: 2mb)
Or drag a symbol into the upload area
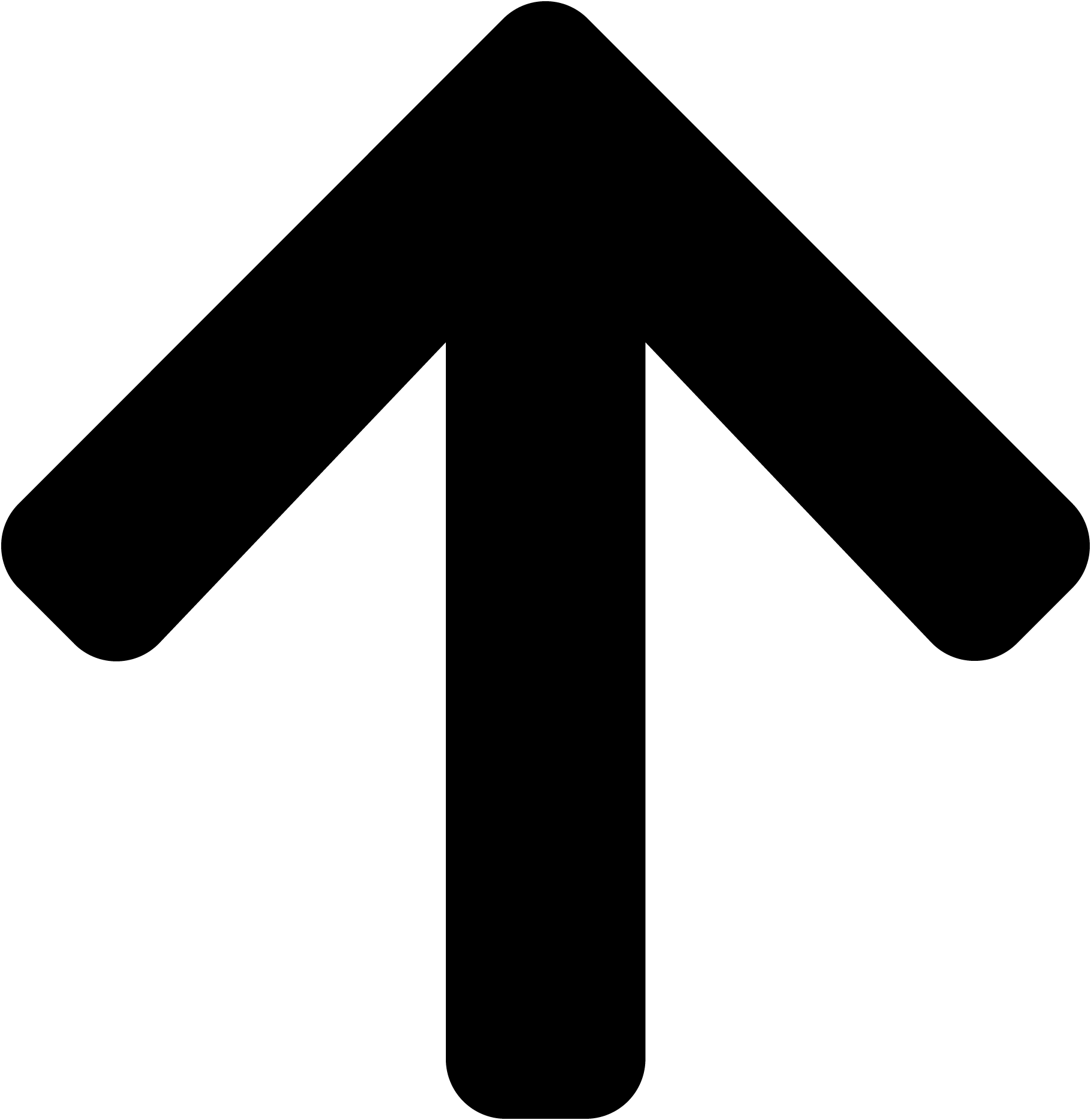
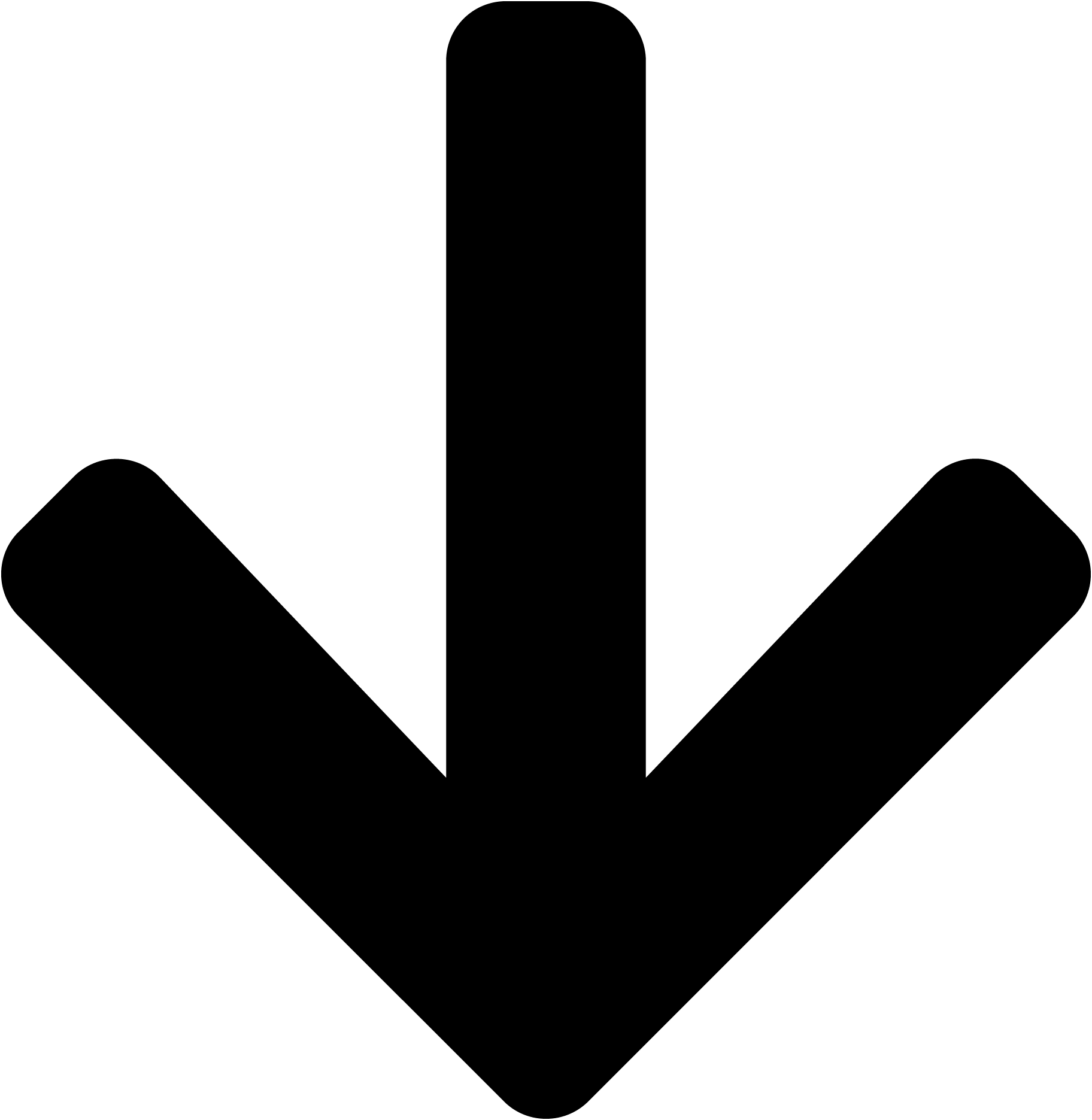
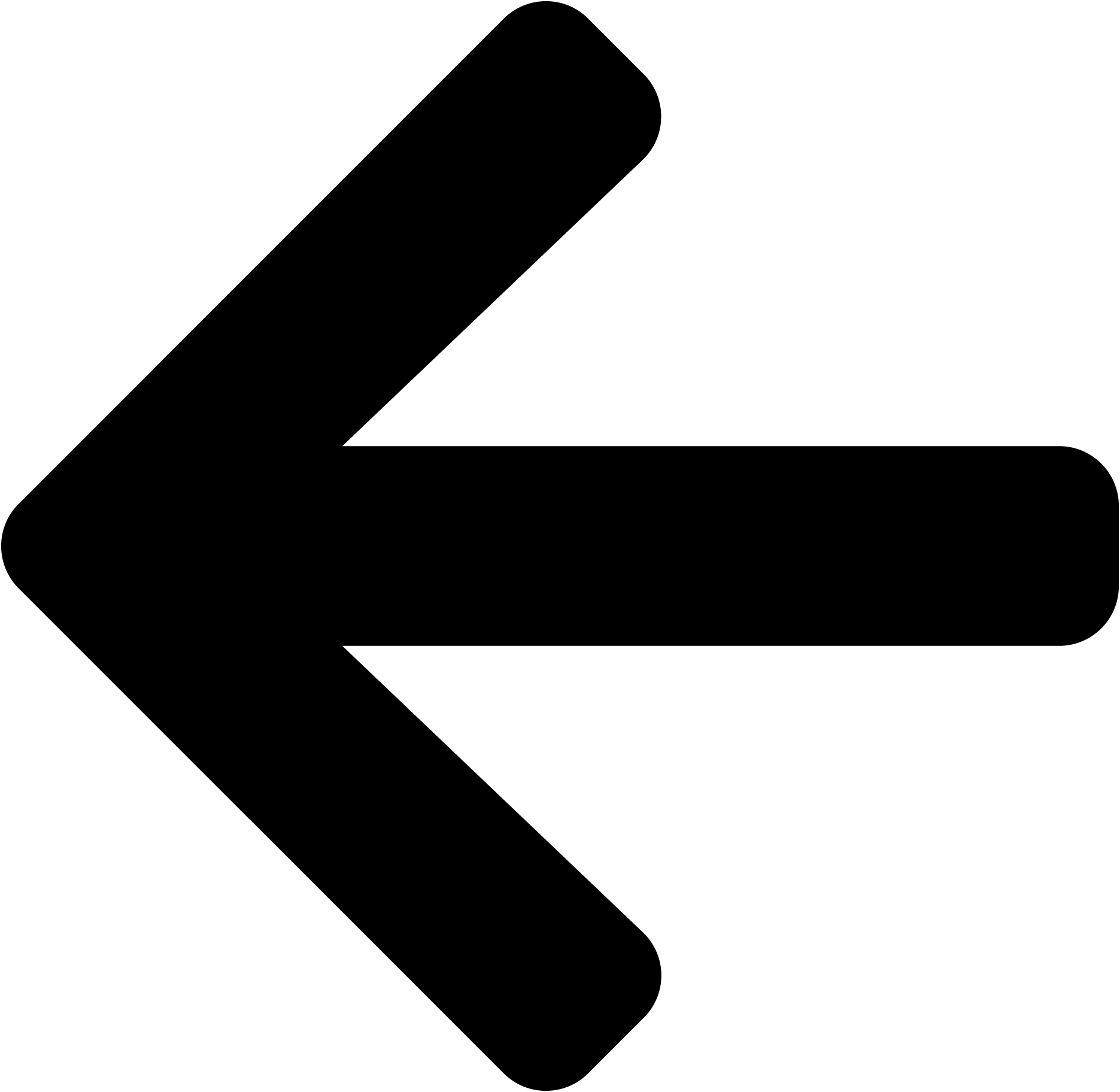
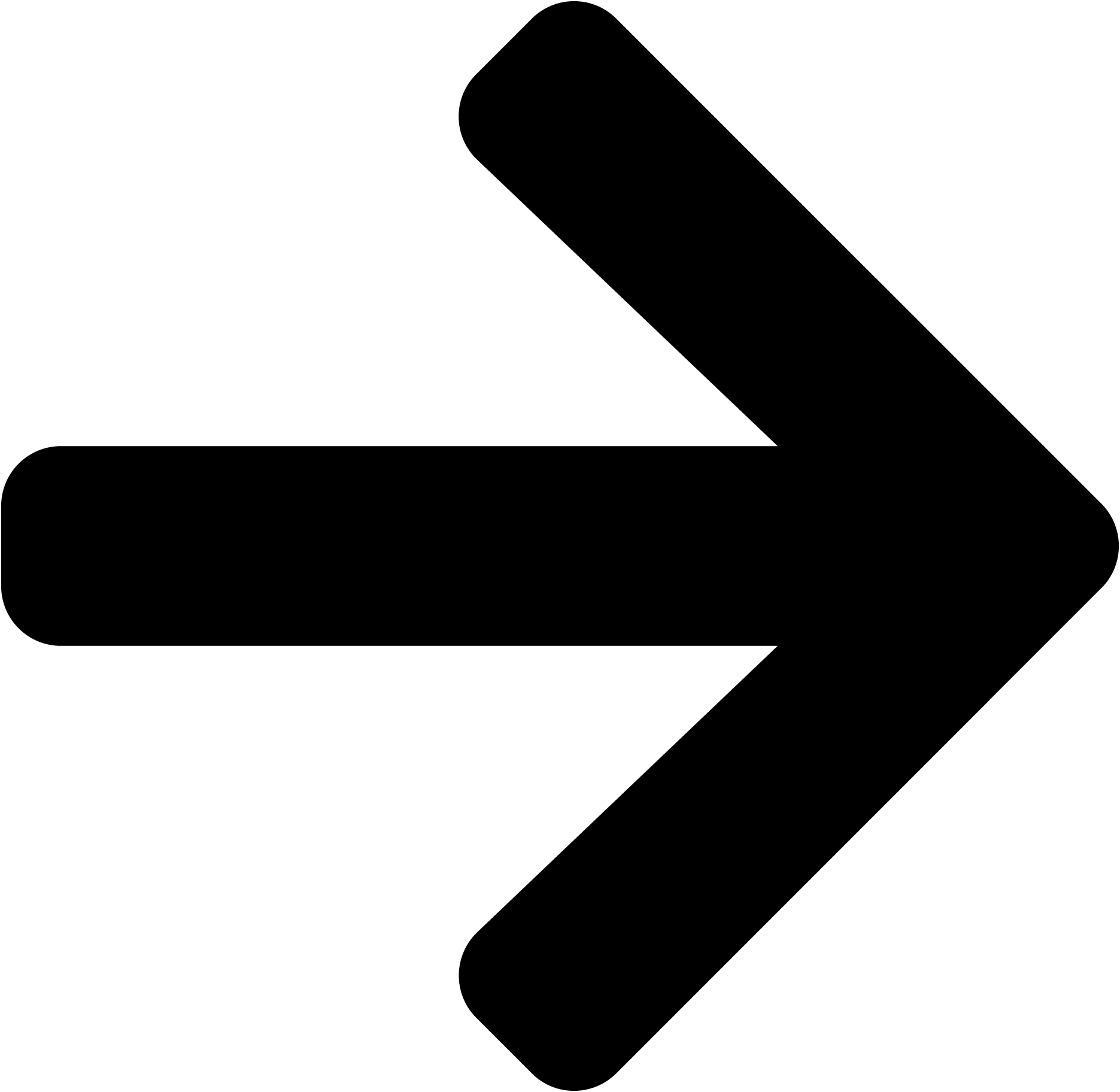
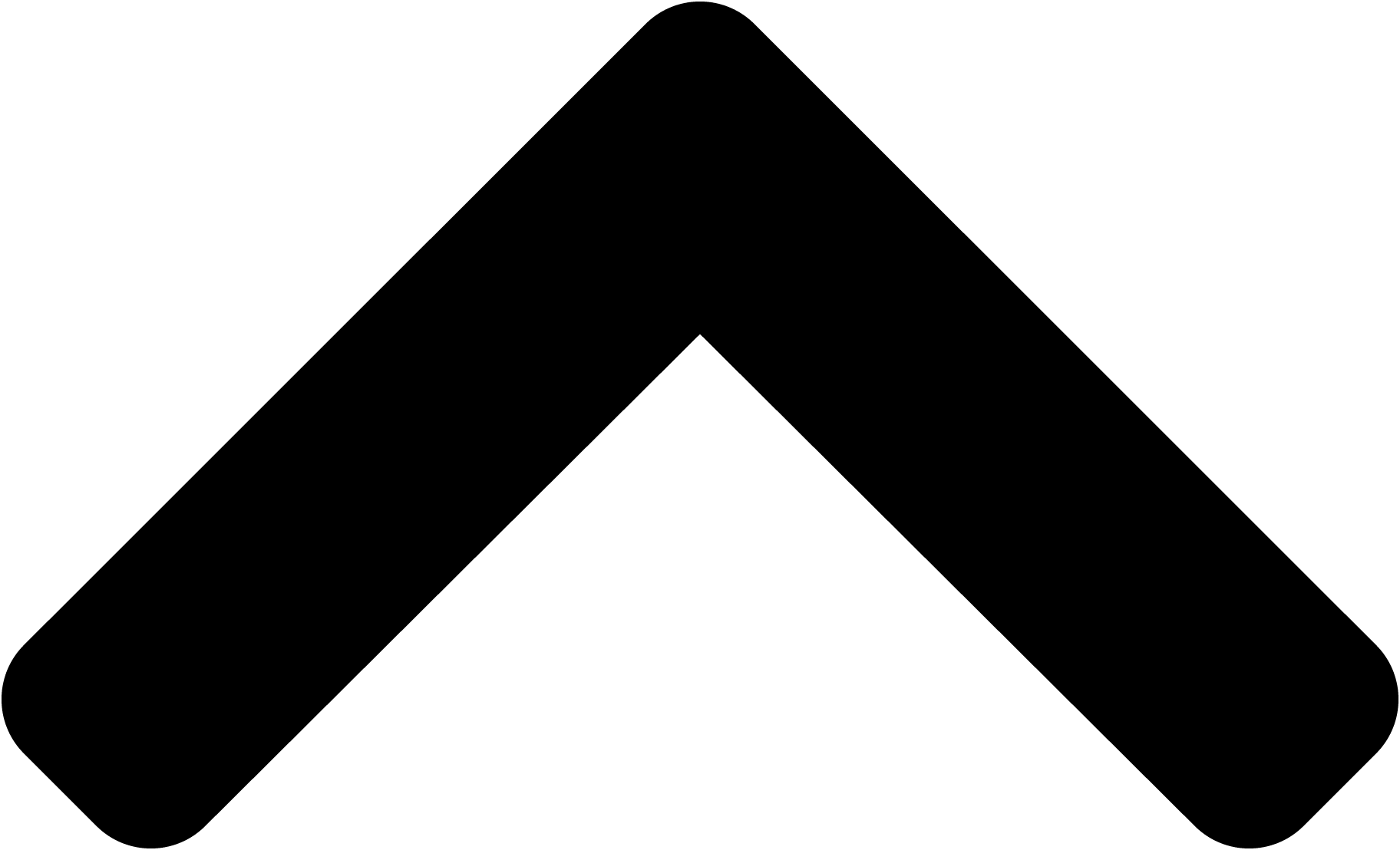
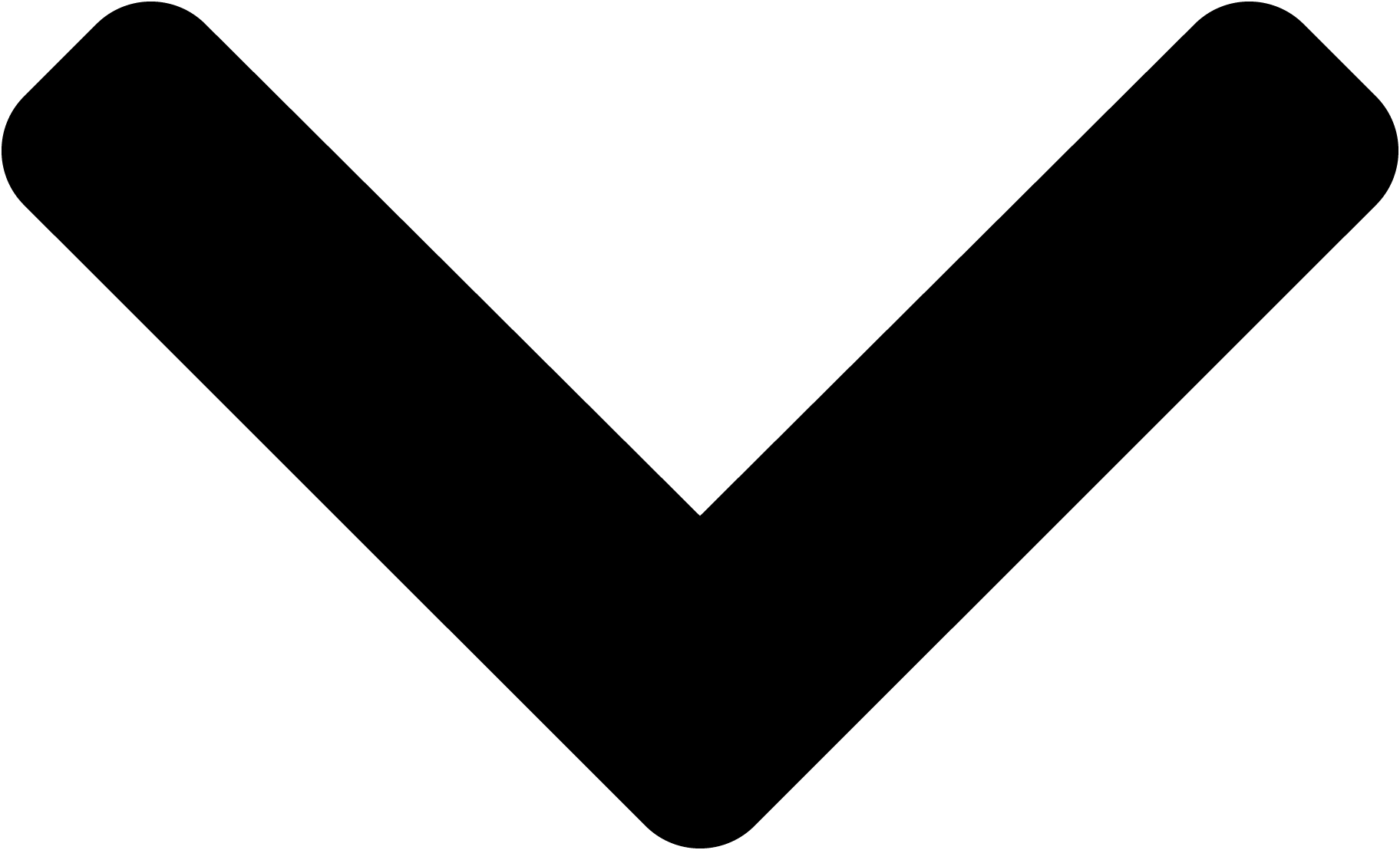
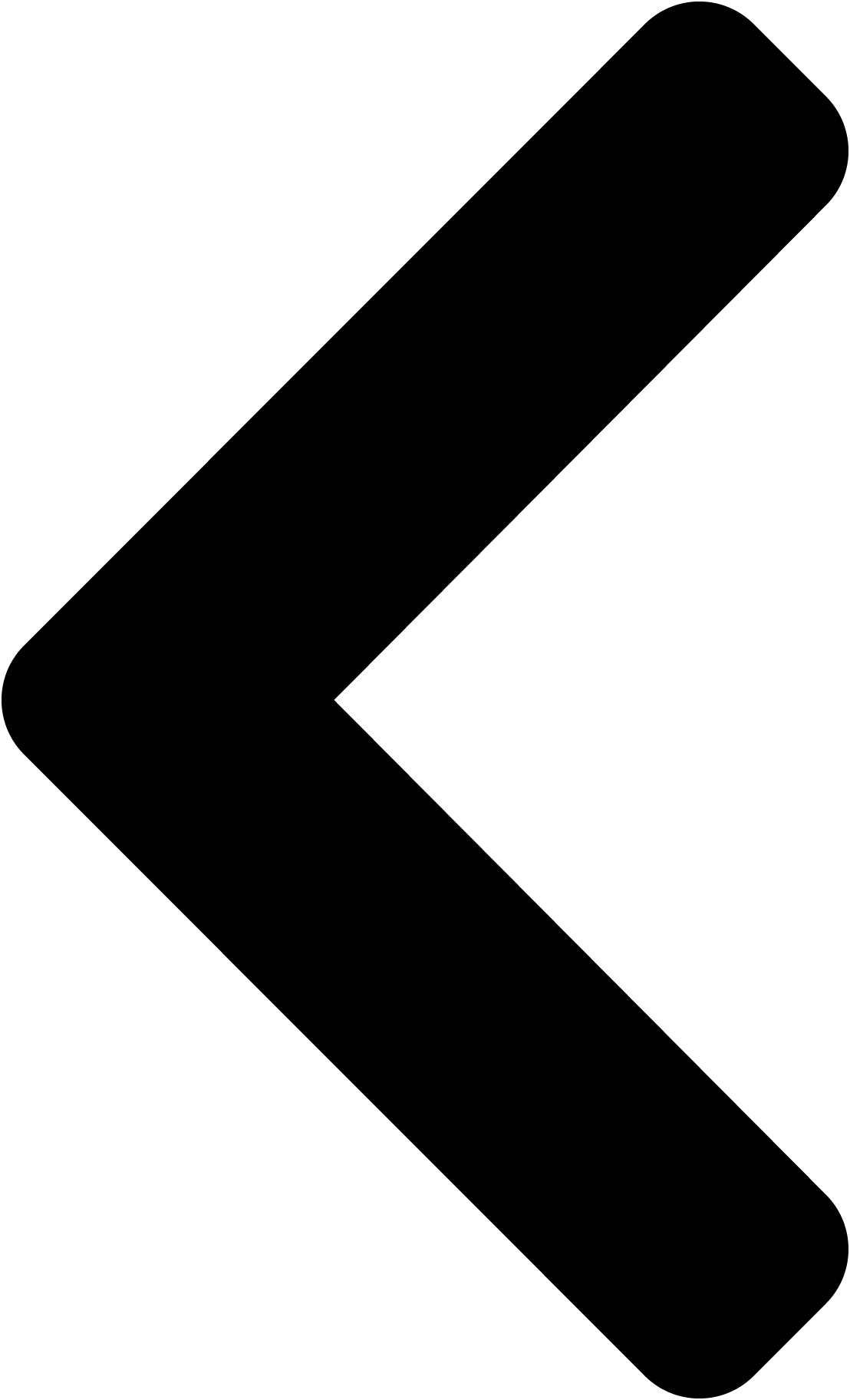
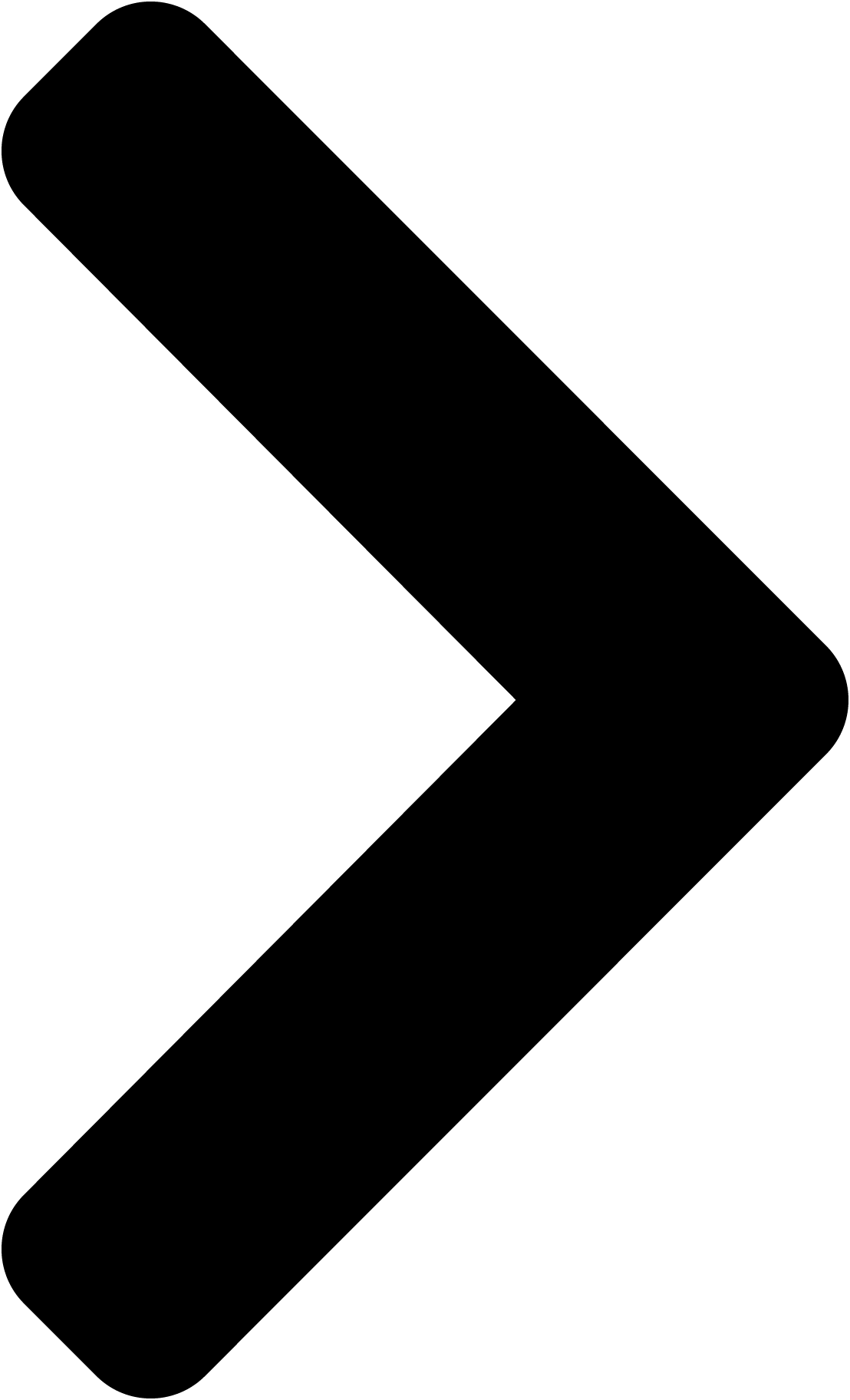
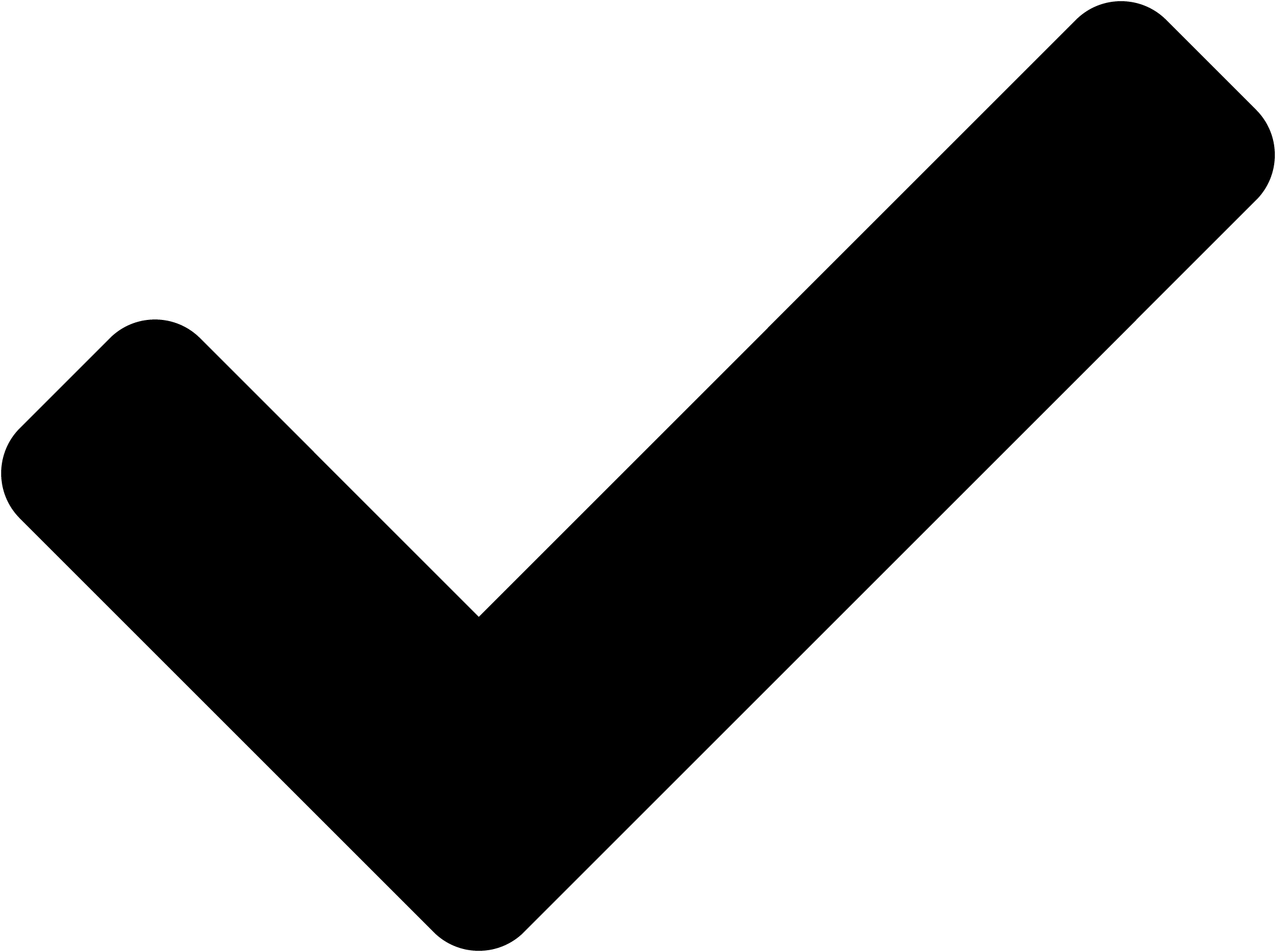
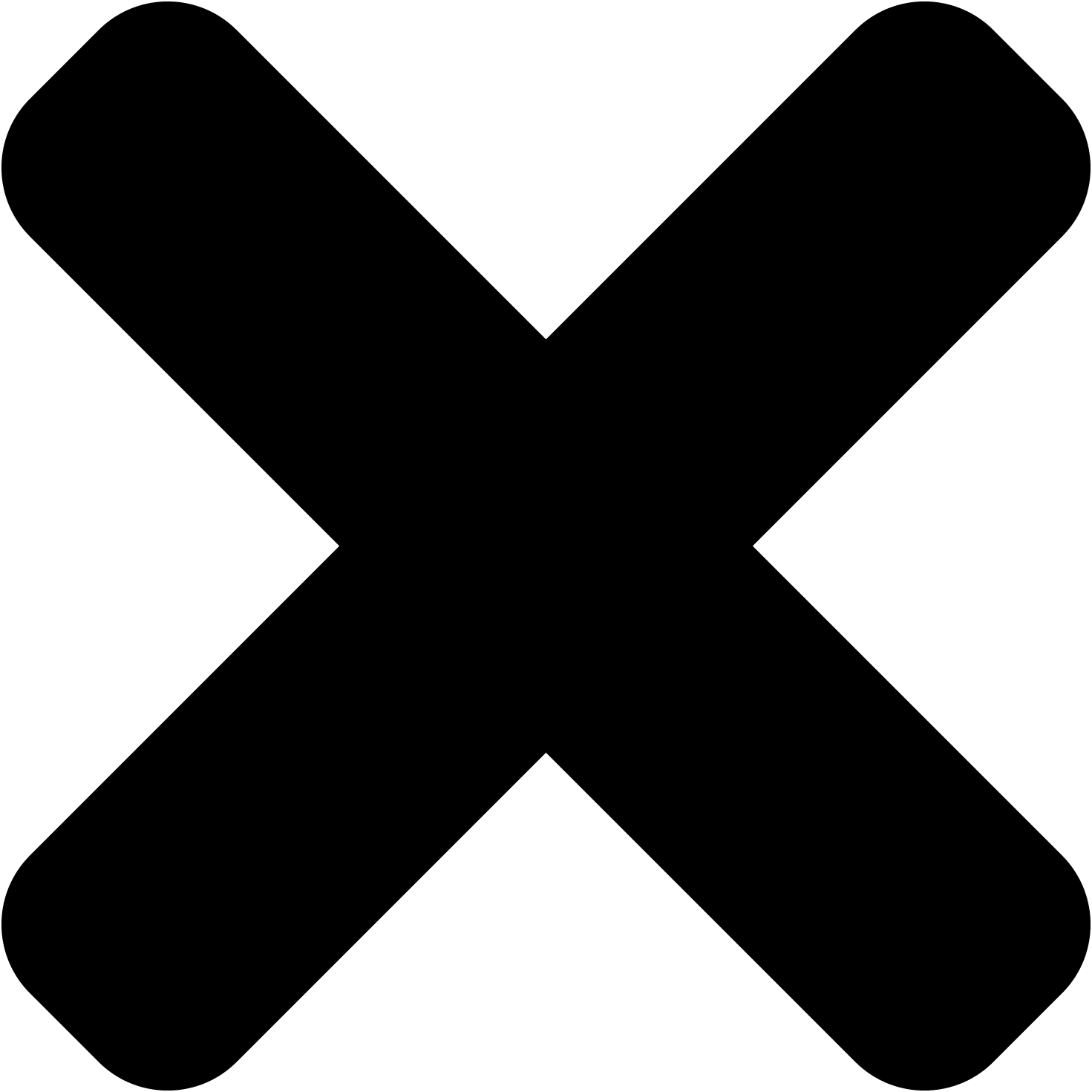
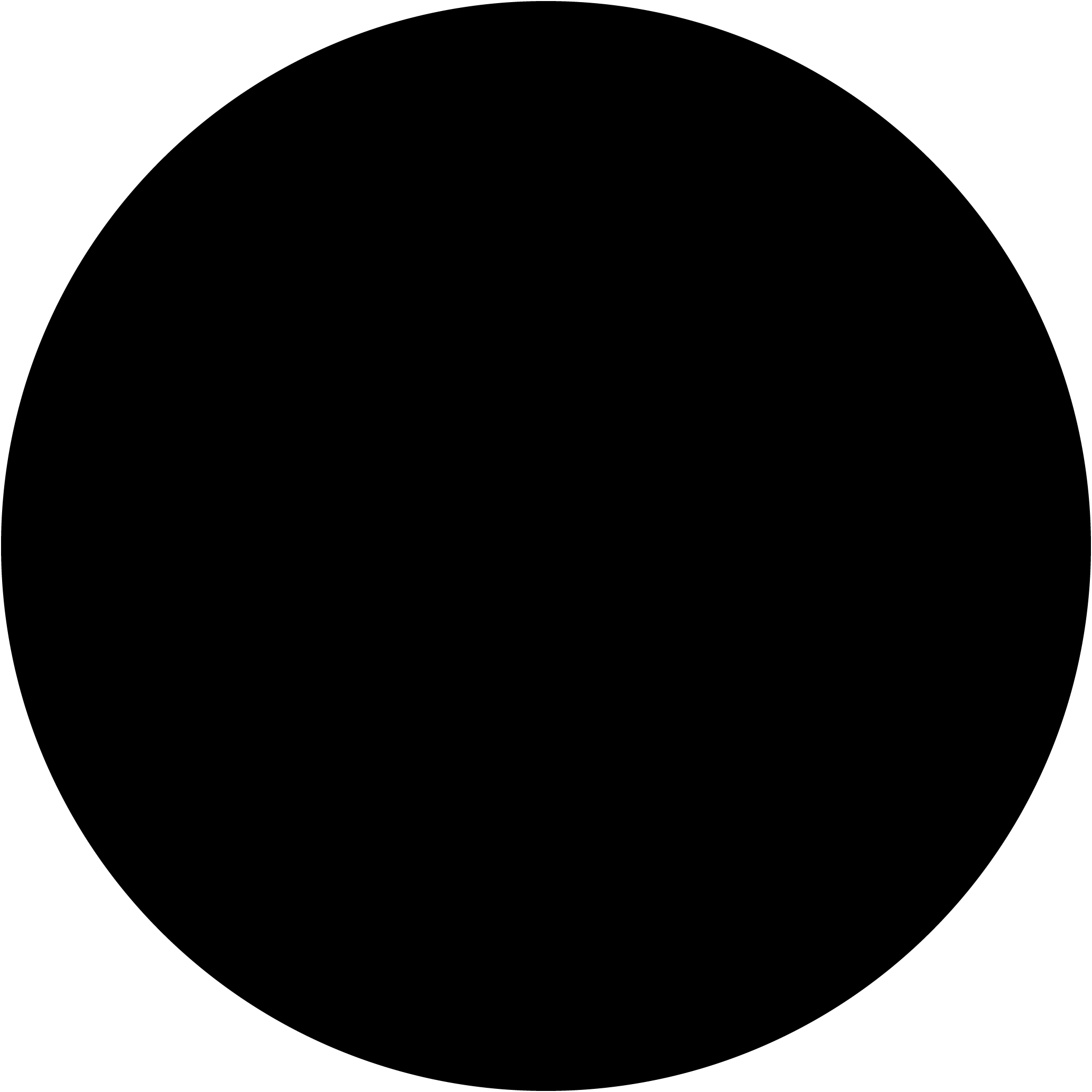
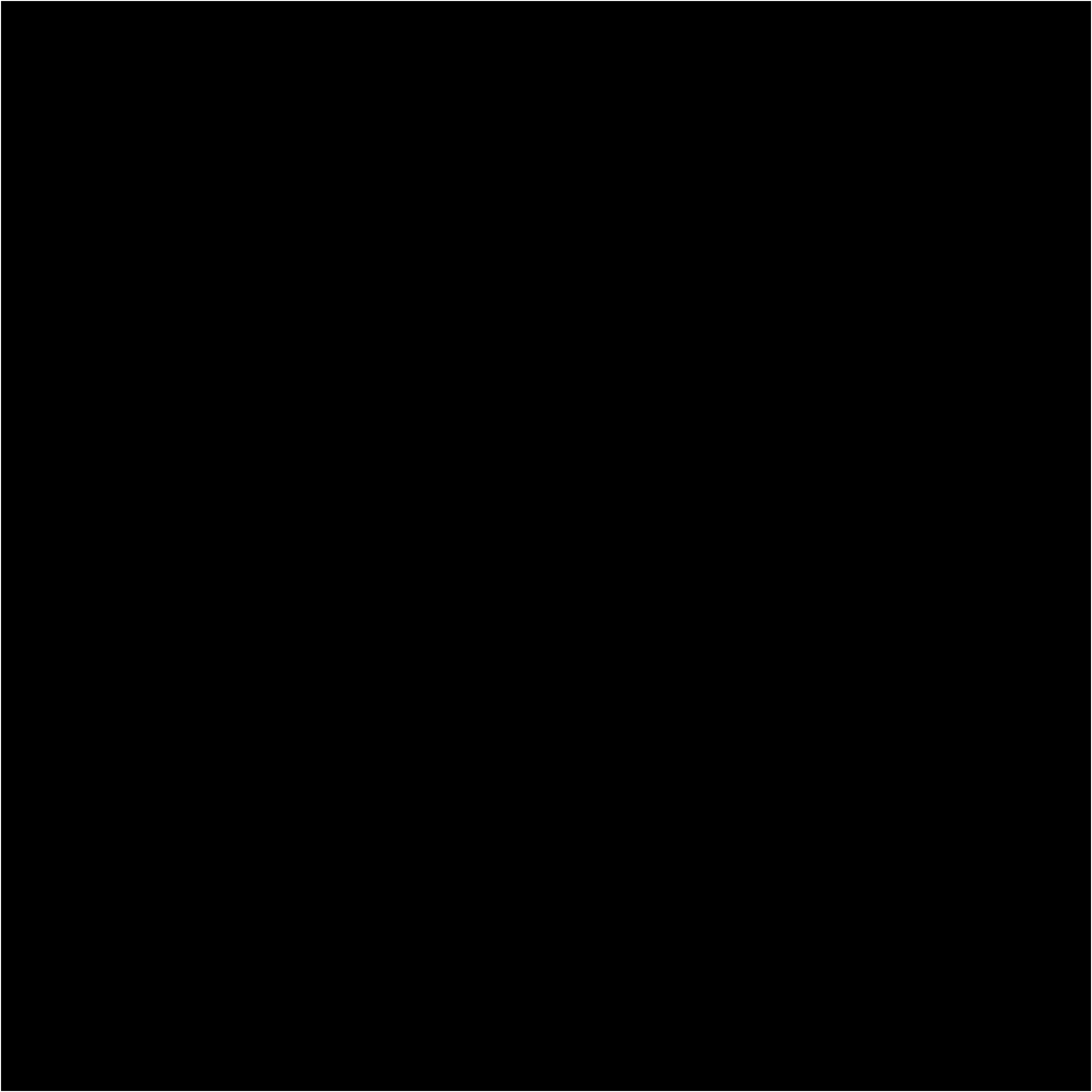
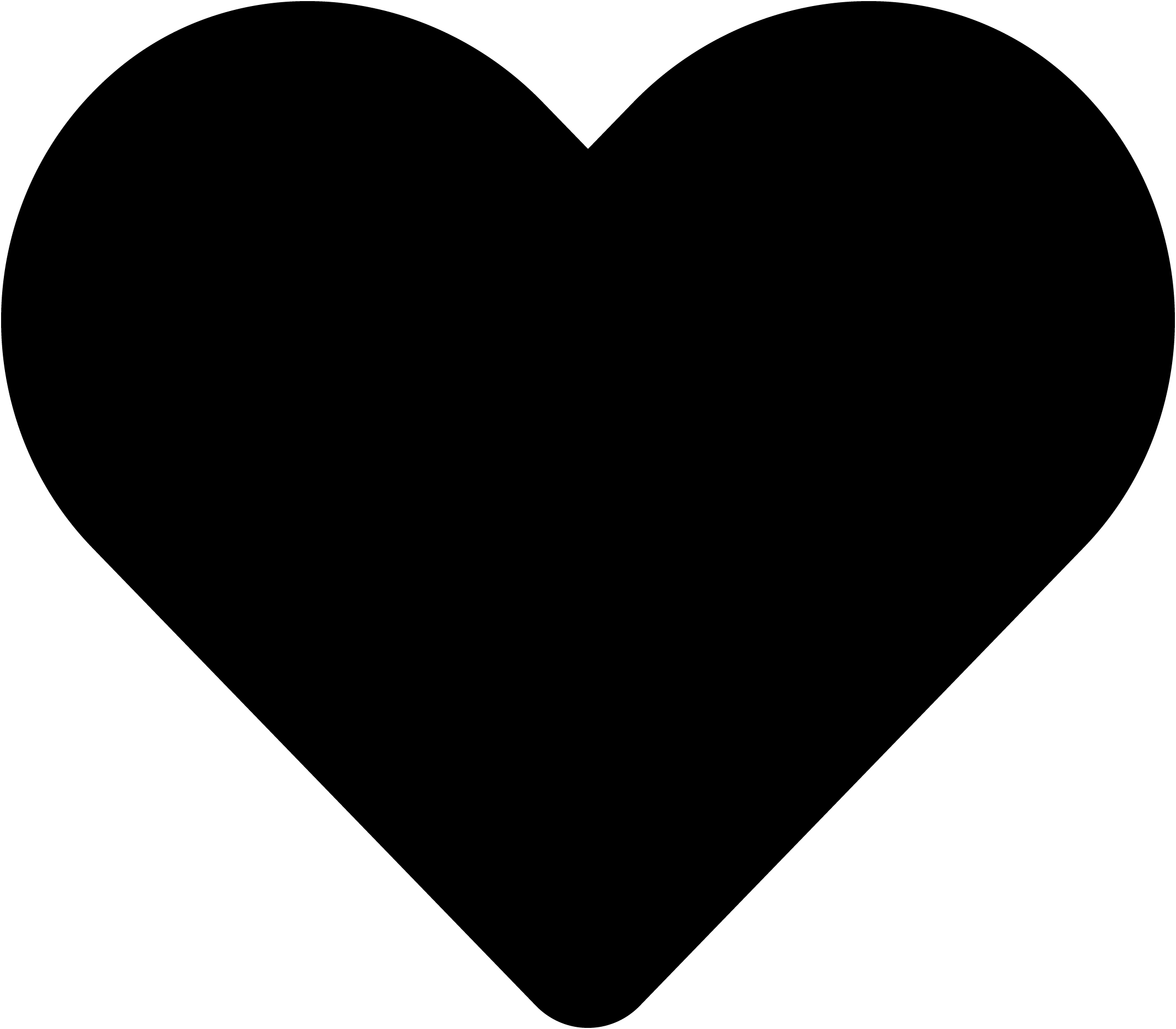
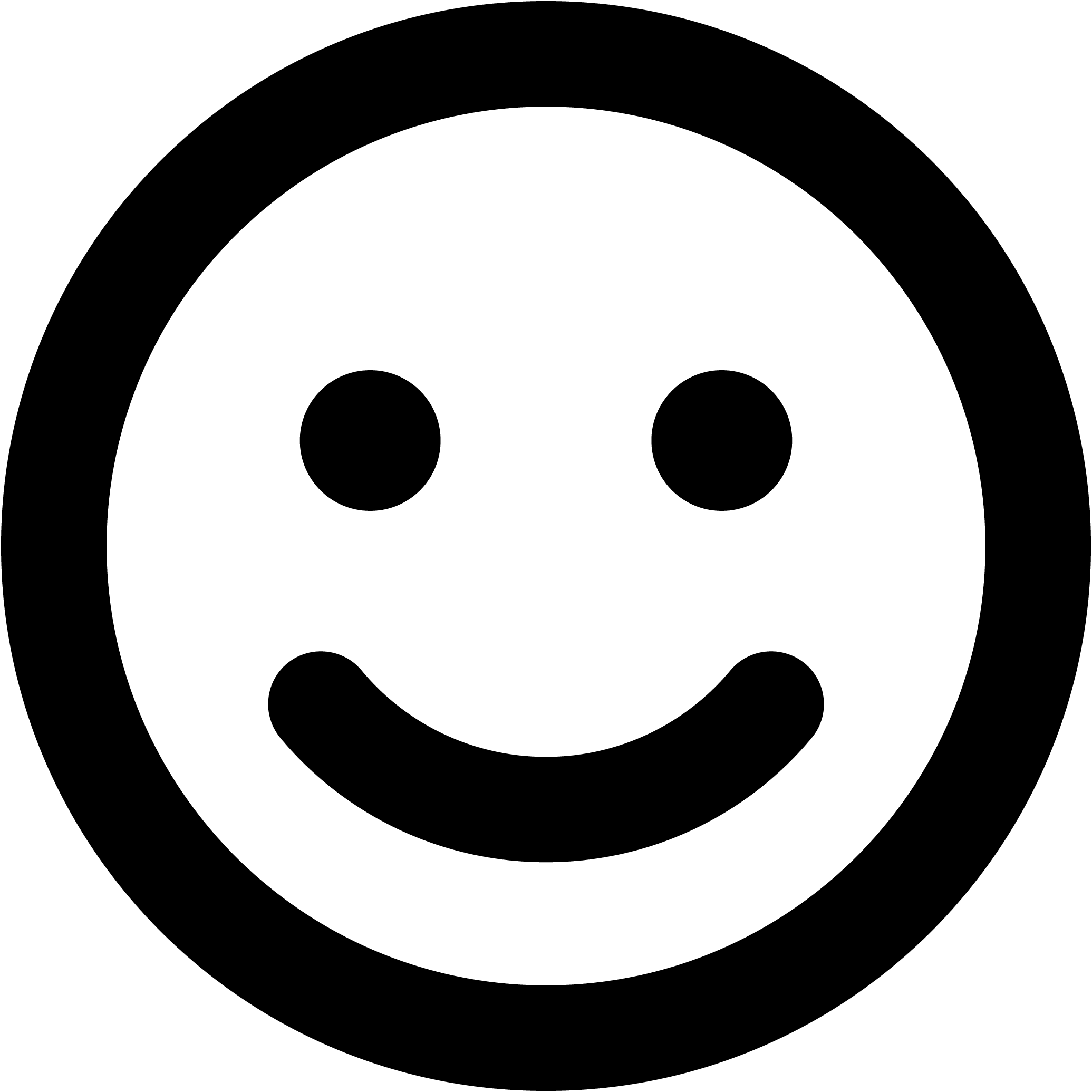
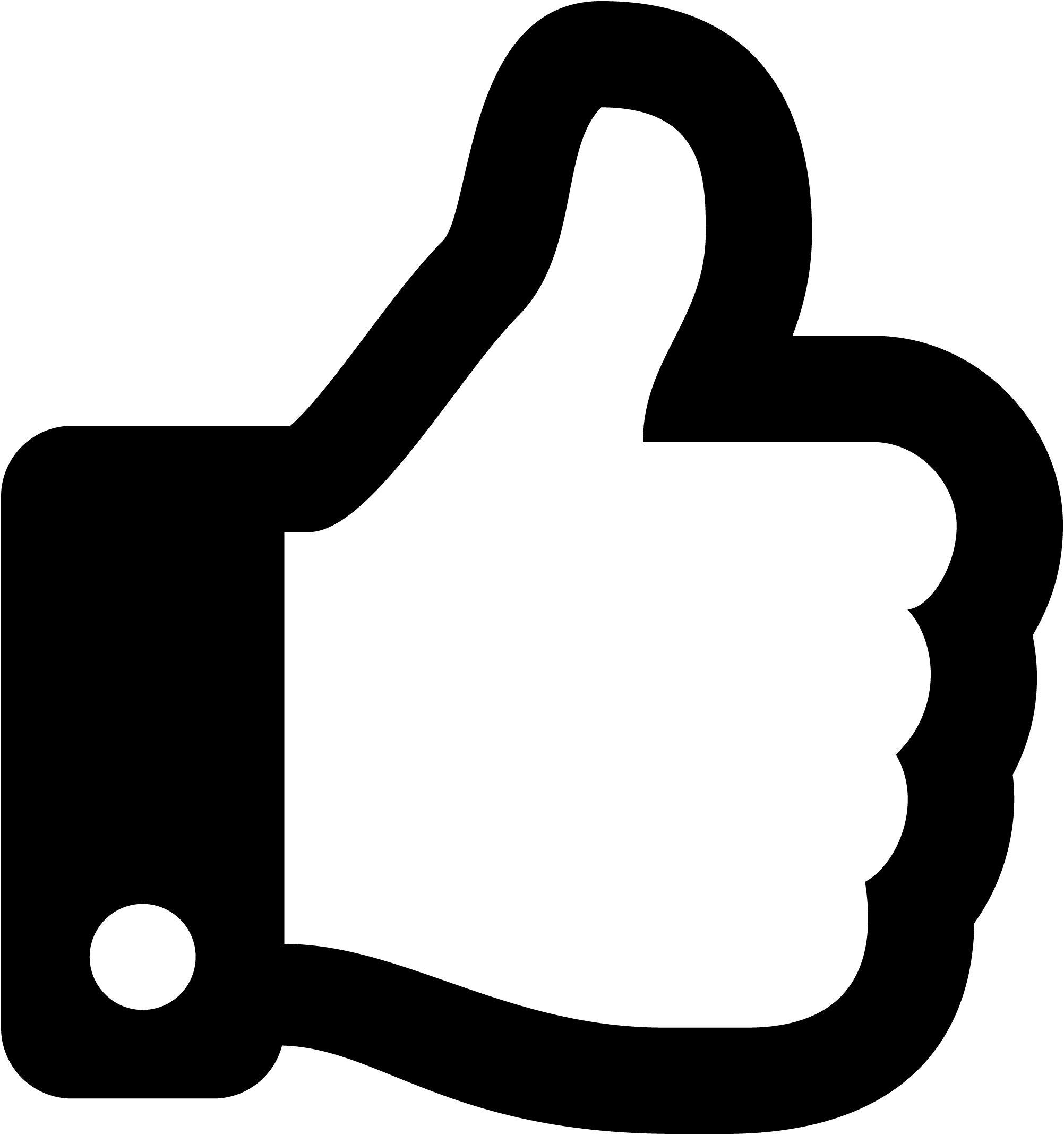
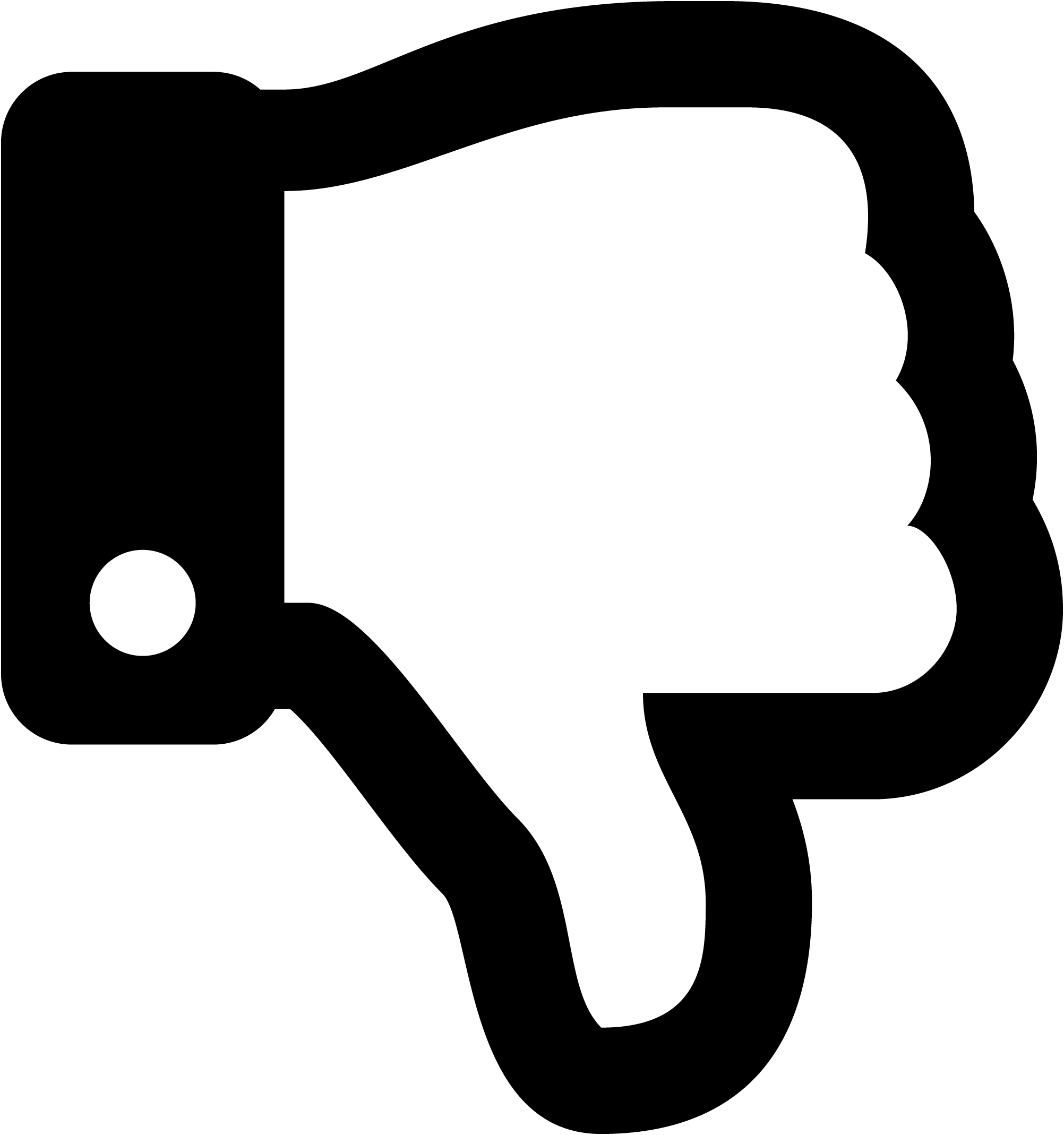
Border colour
Rotate
Skew (x-axis)
Skew (y-axis)
Image (max size: 2mb)
Or drag a symbol into the upload area
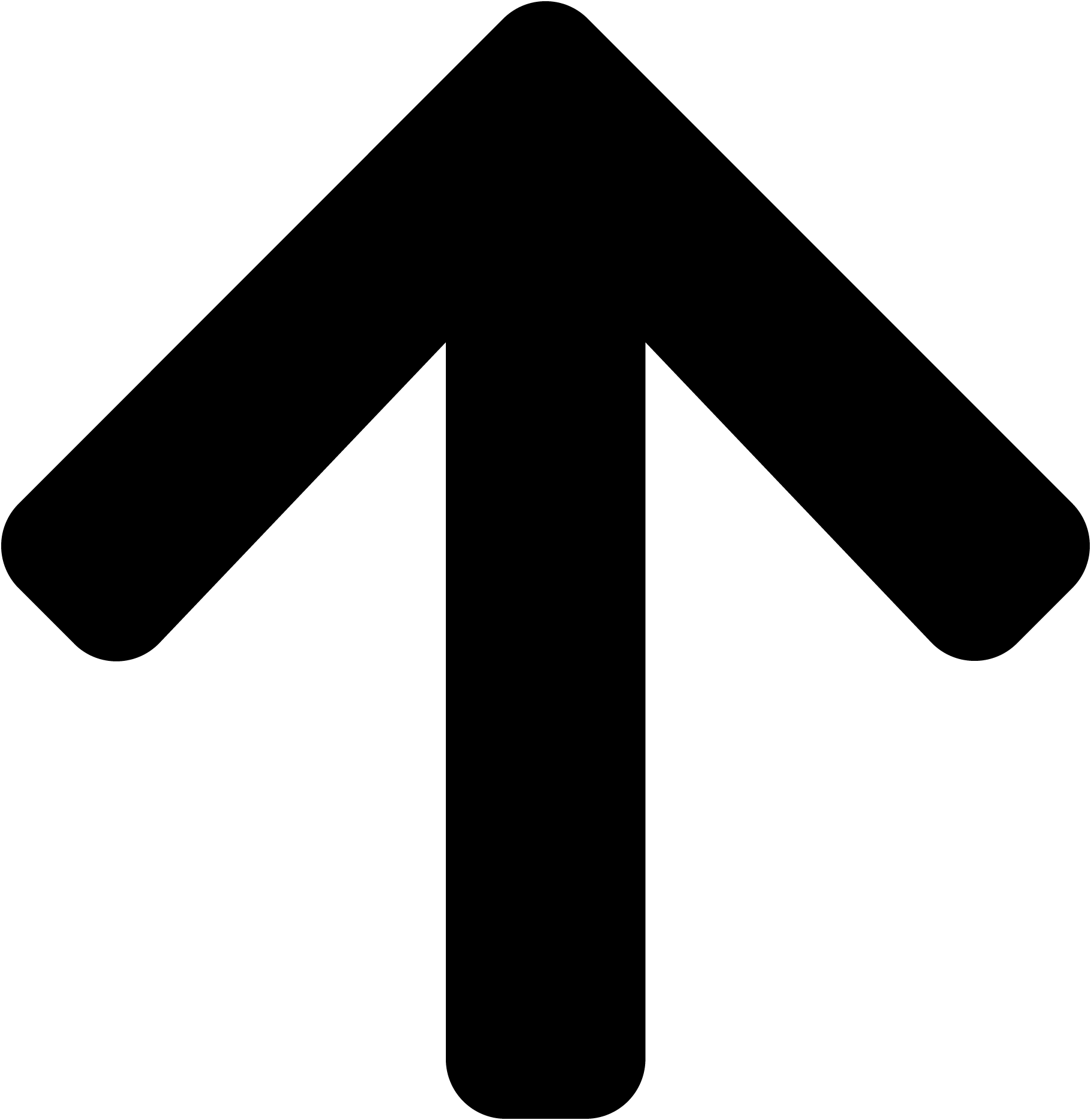
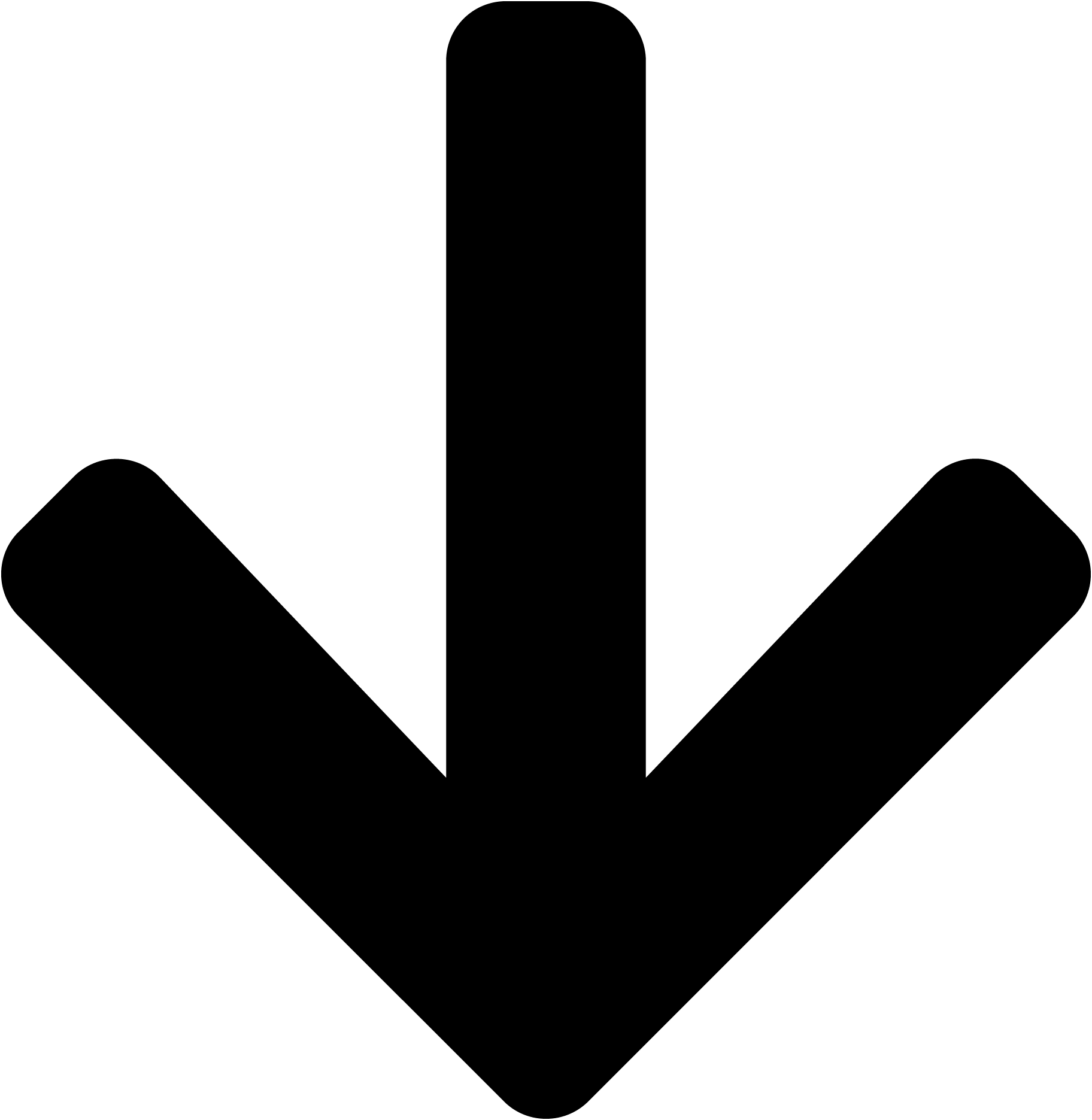
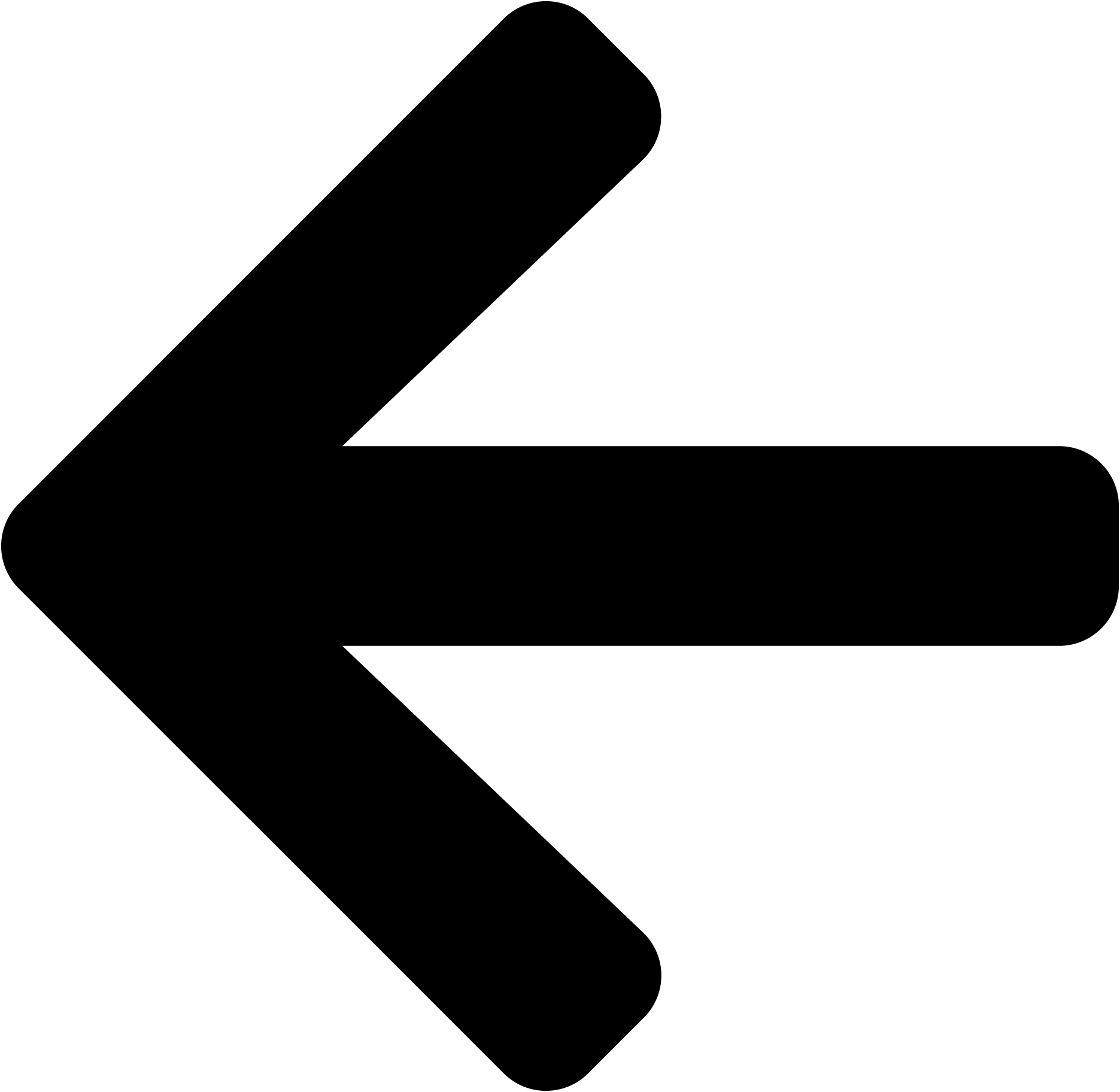
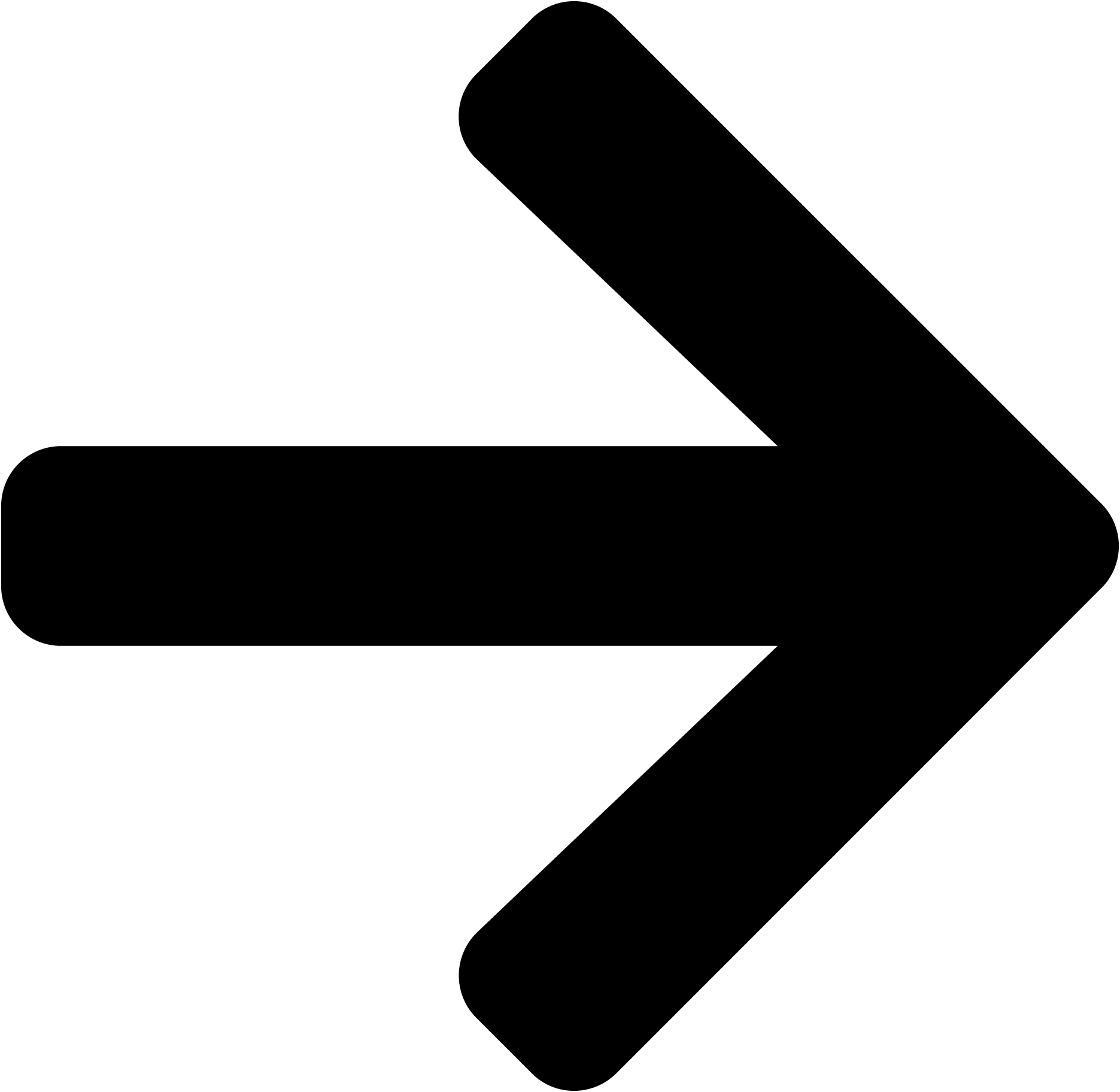
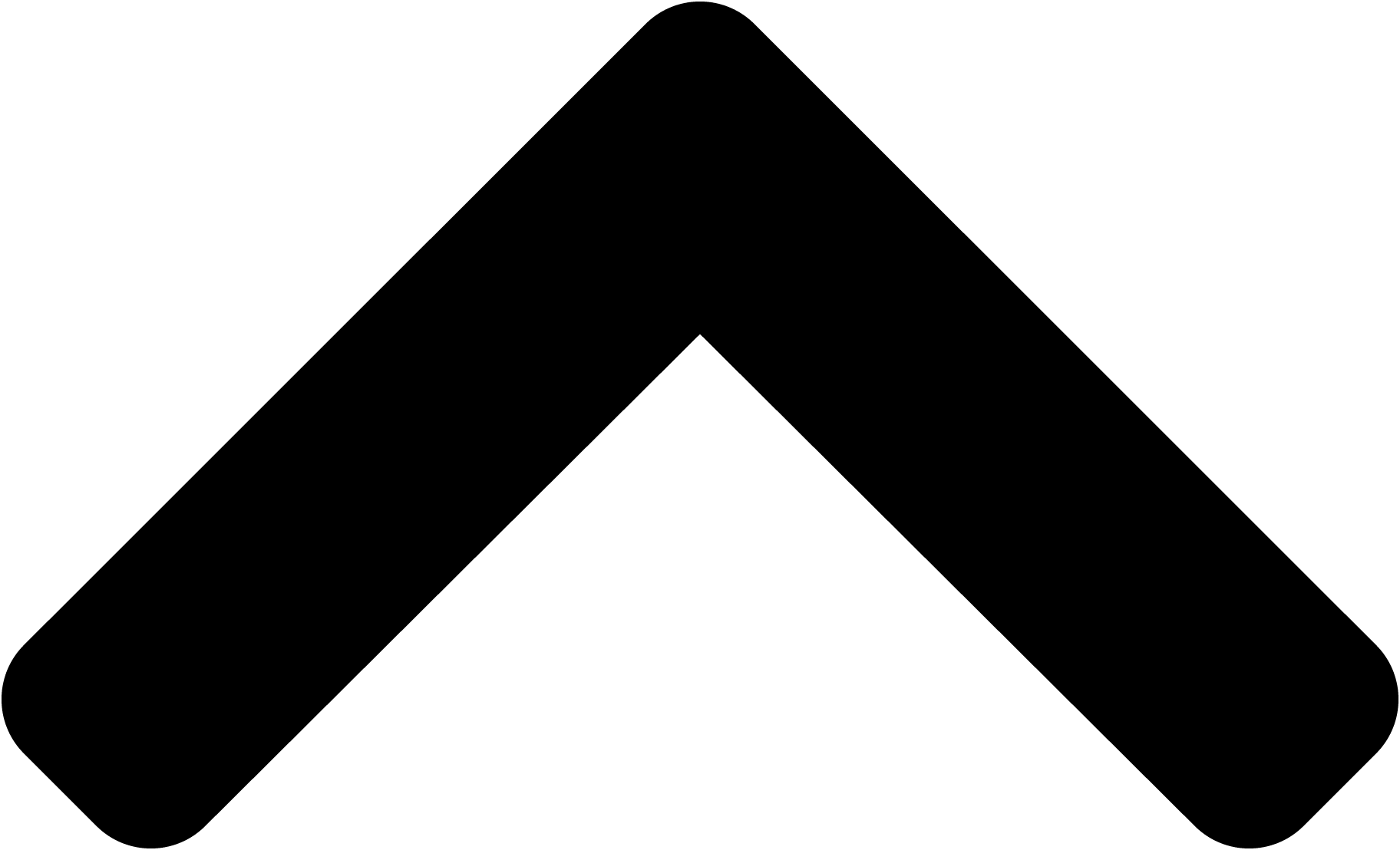
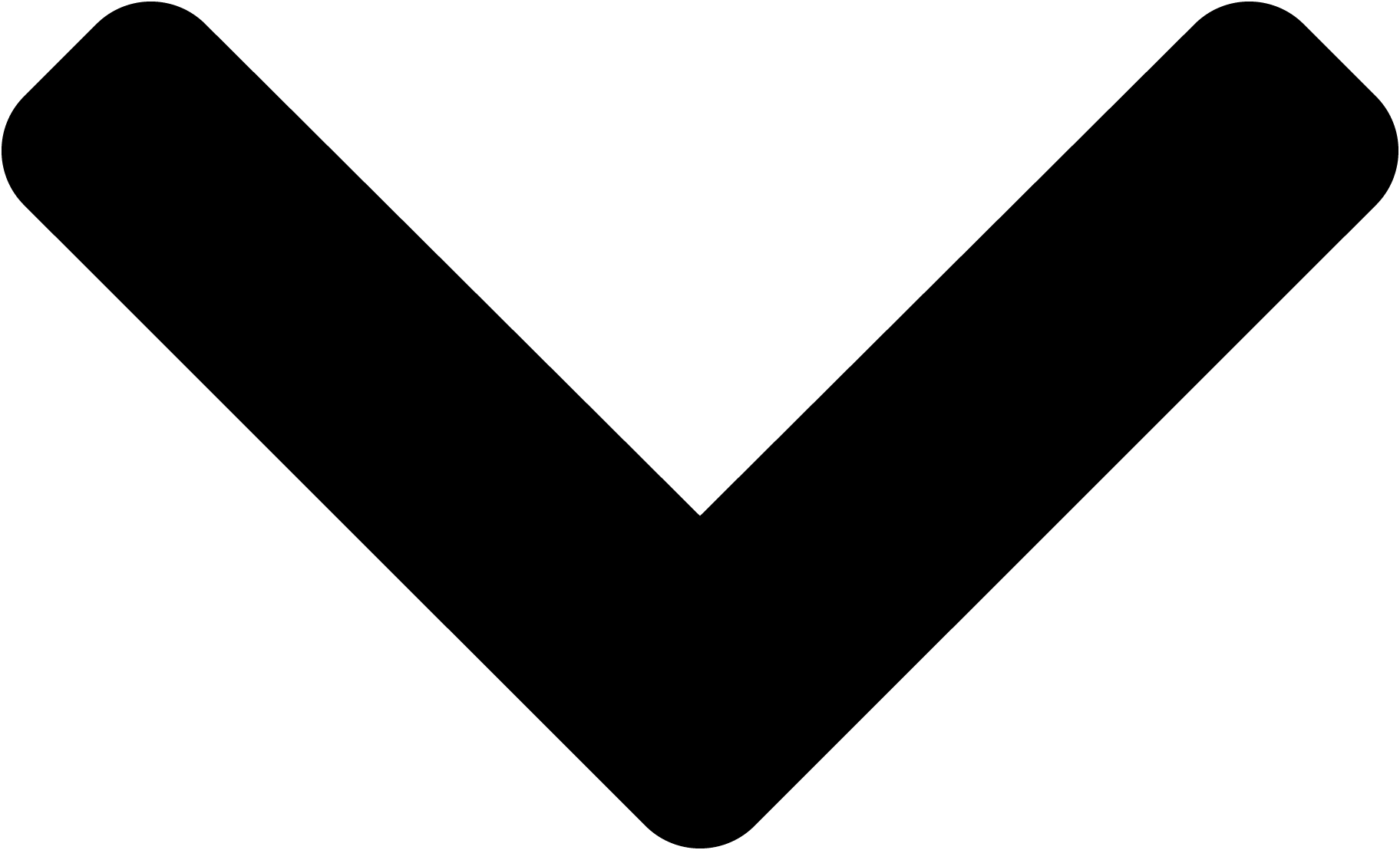
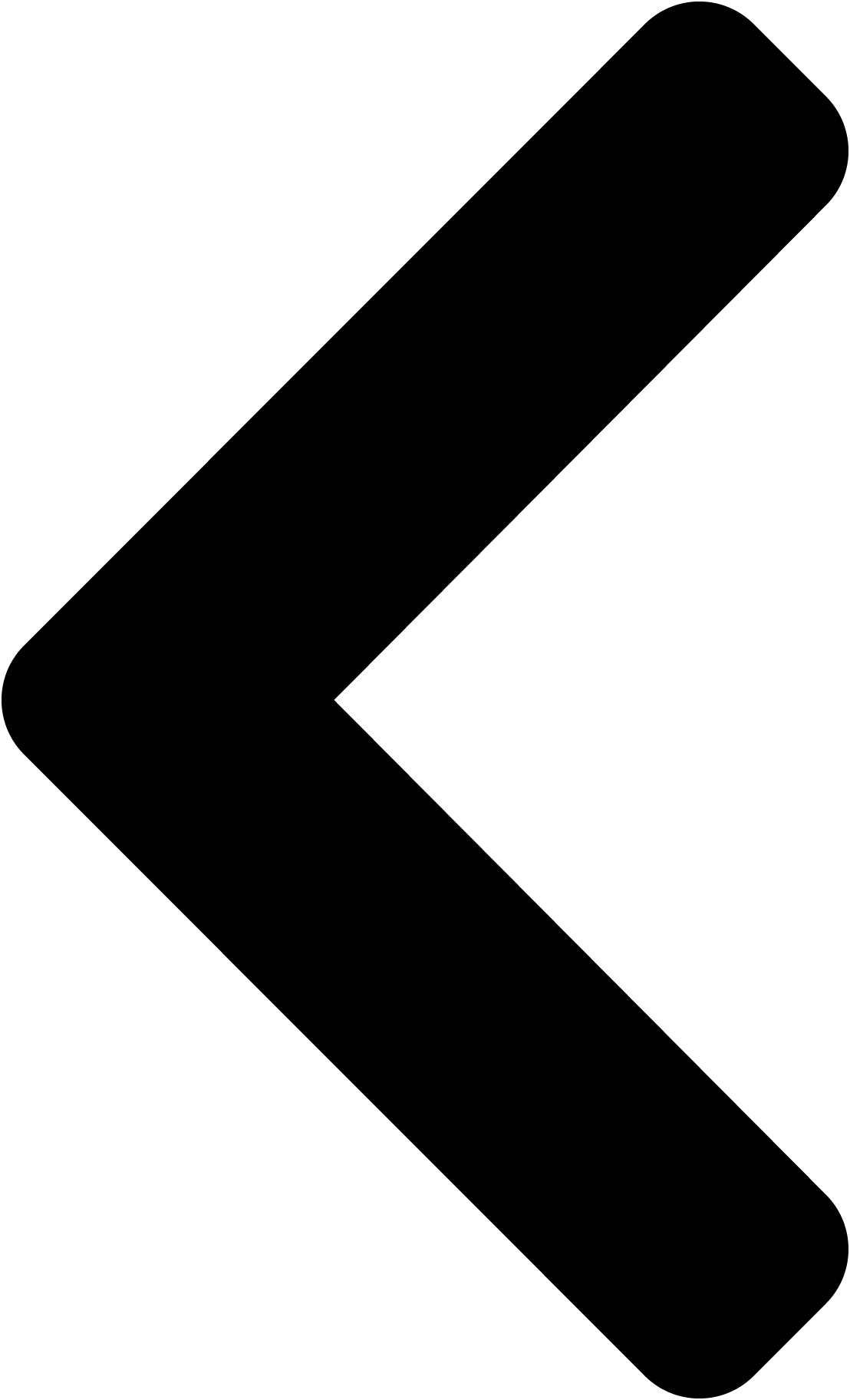
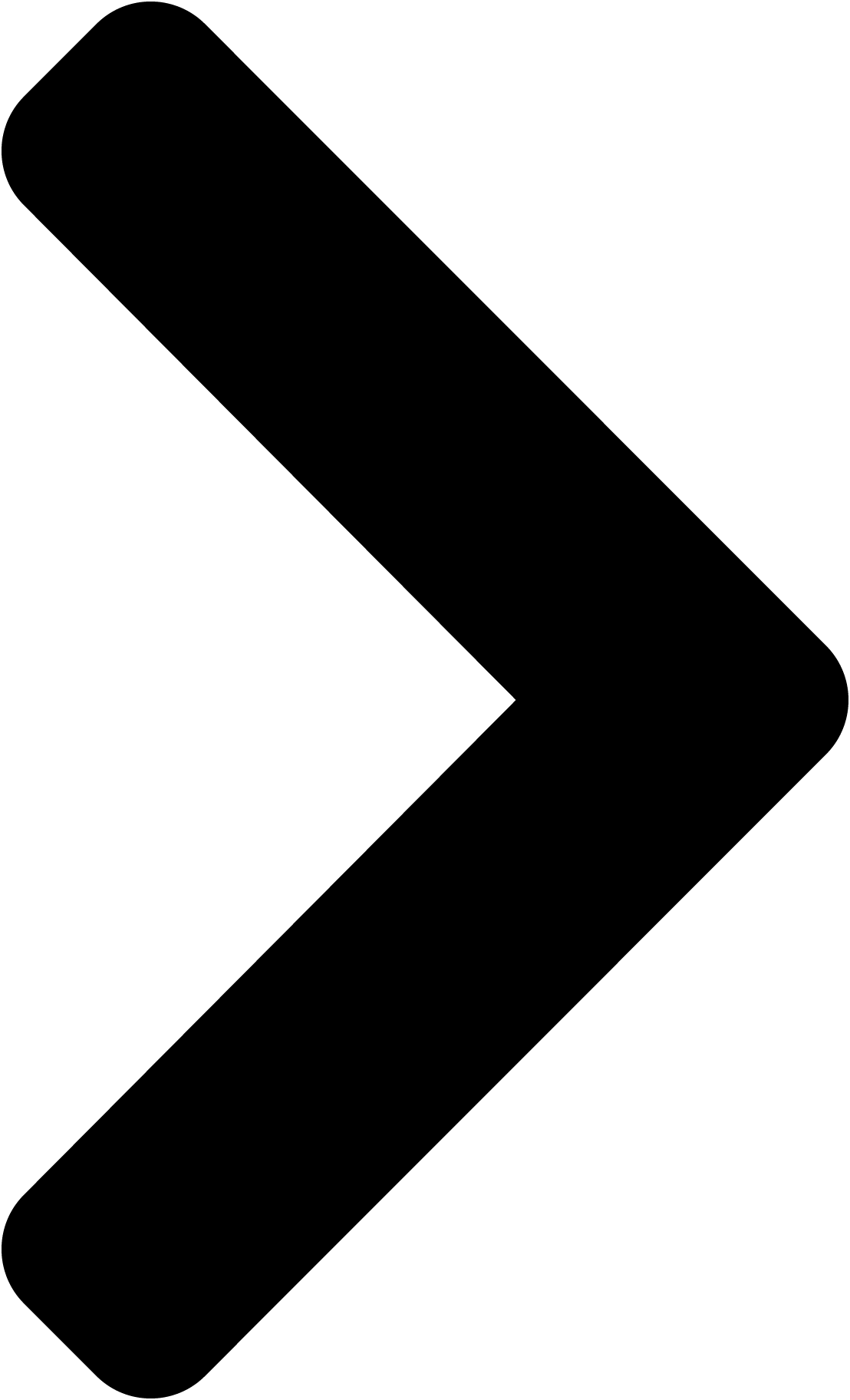
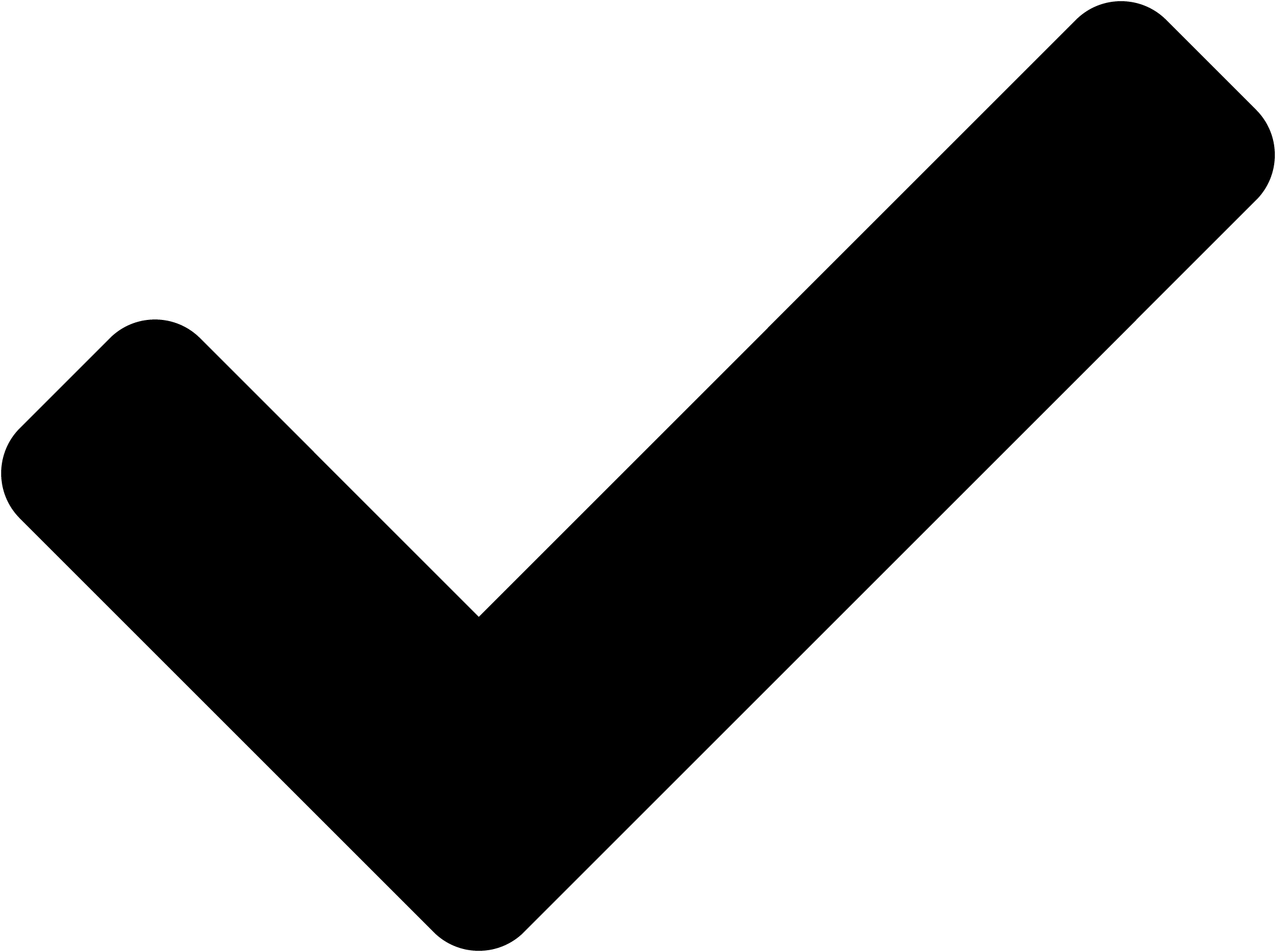
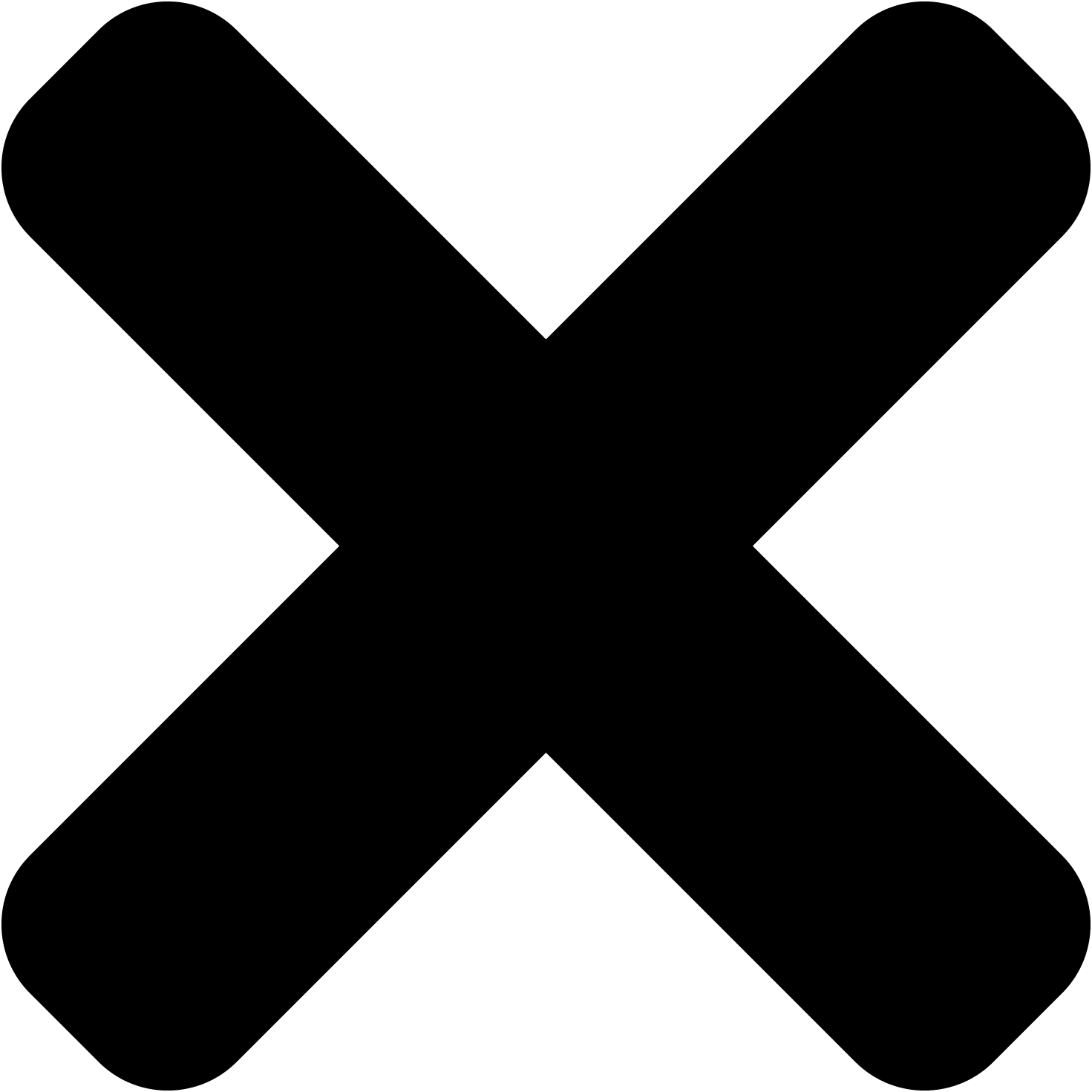
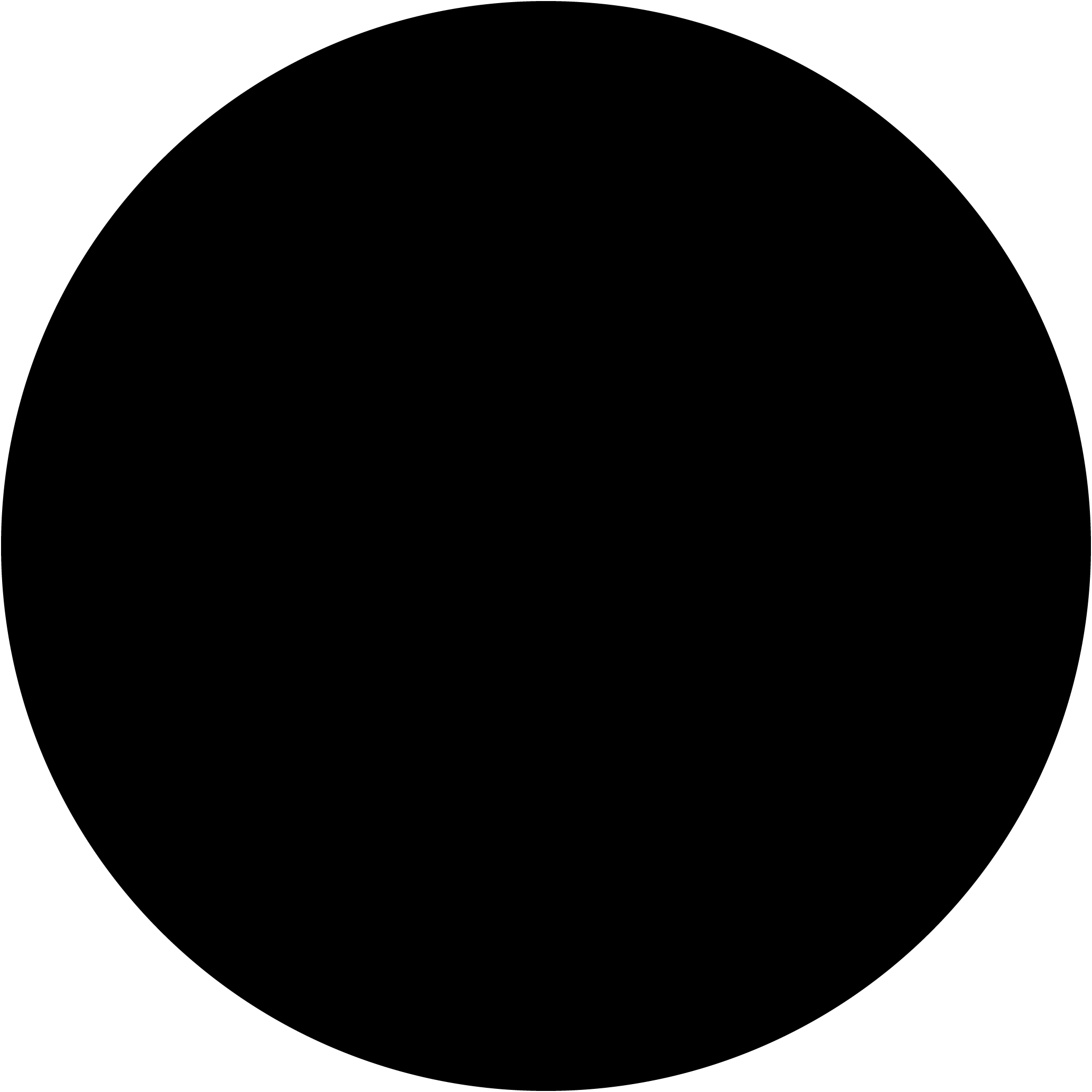
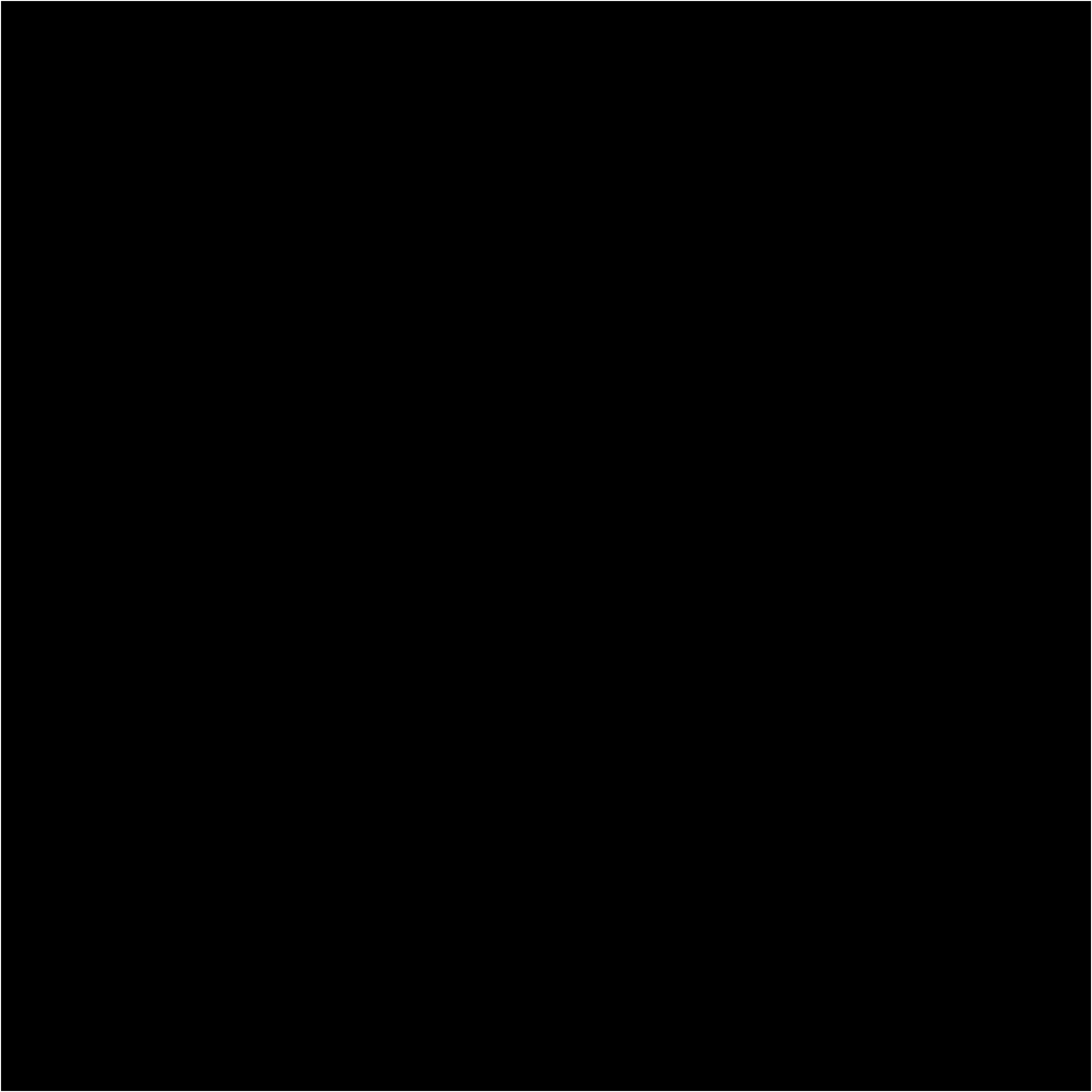
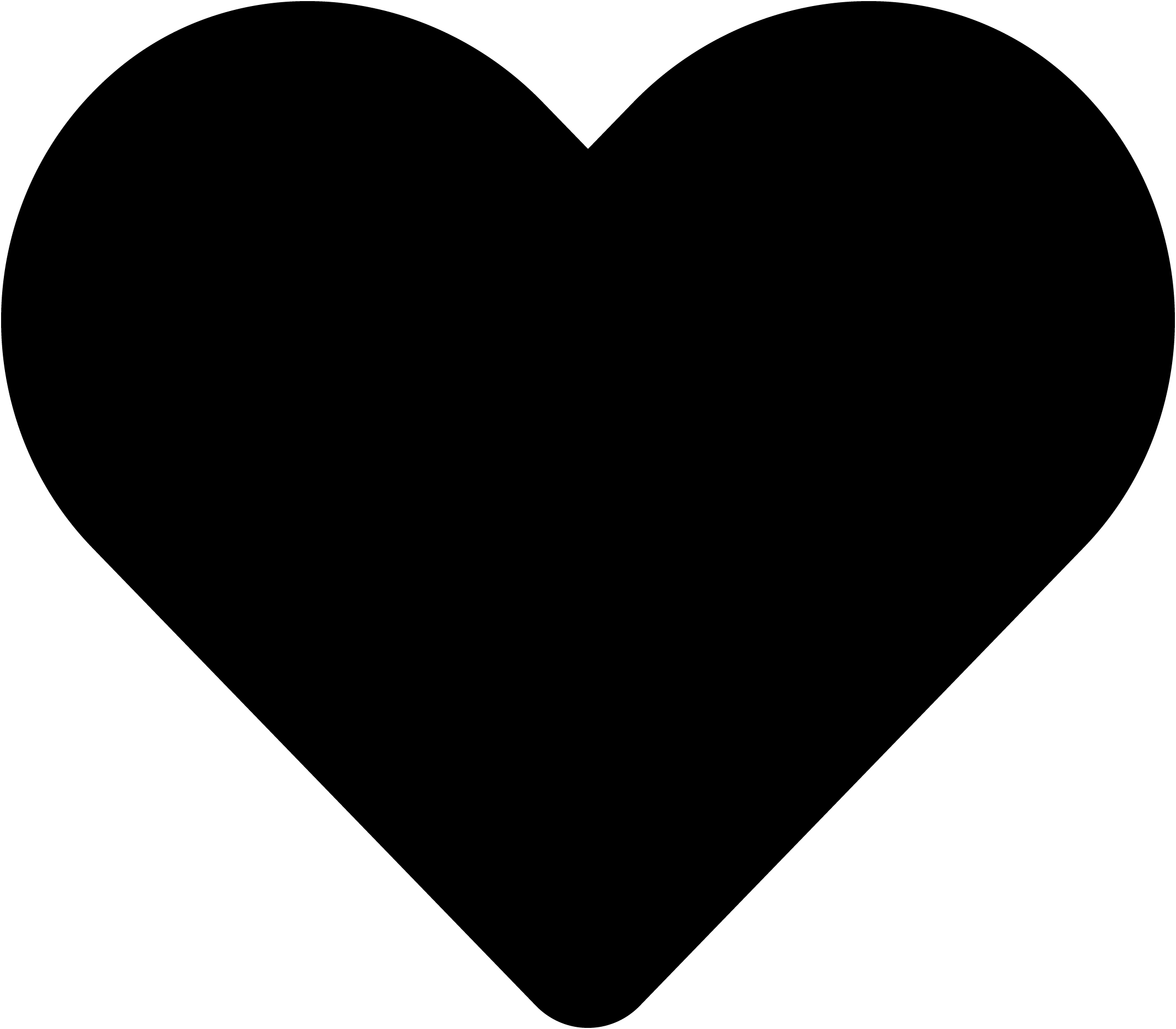
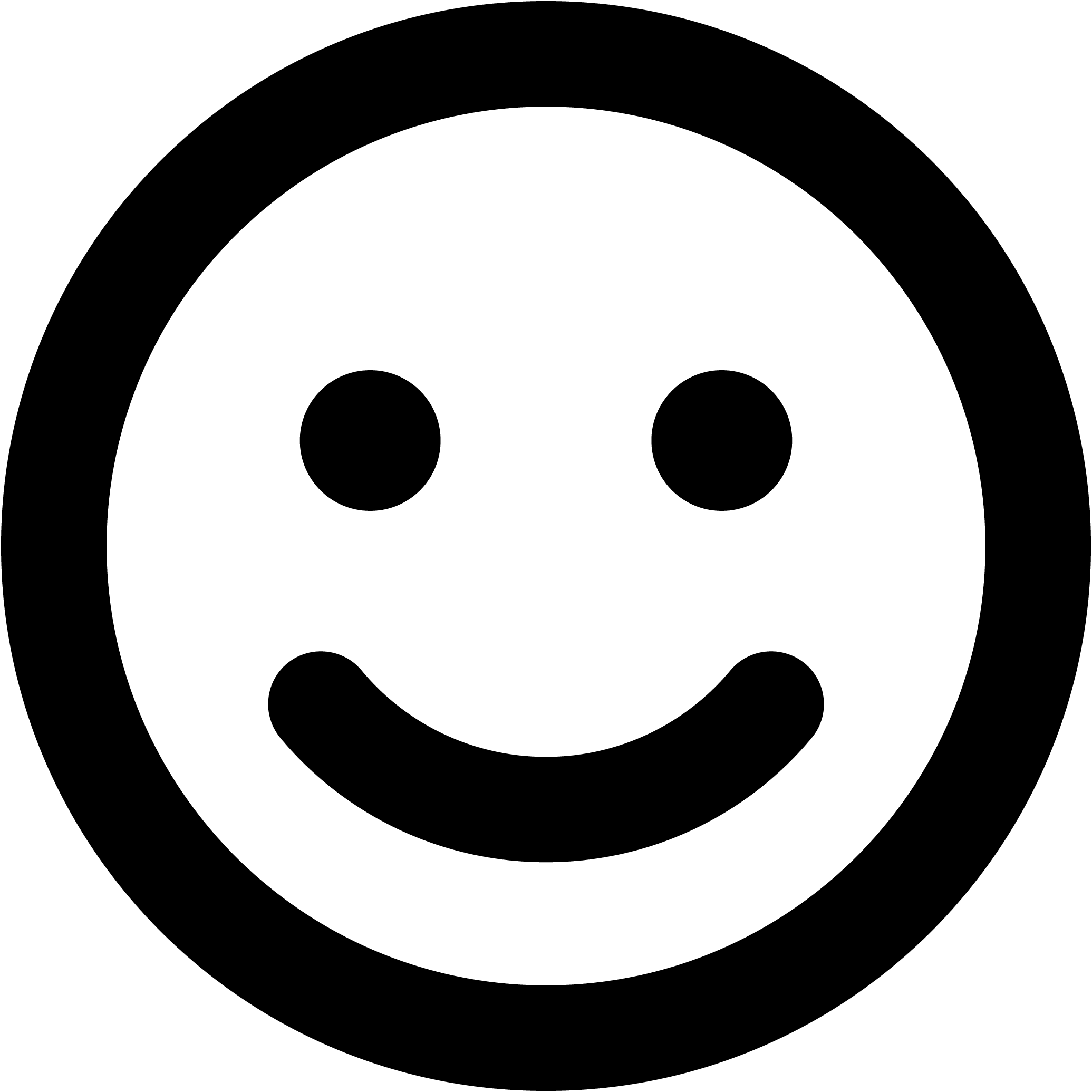
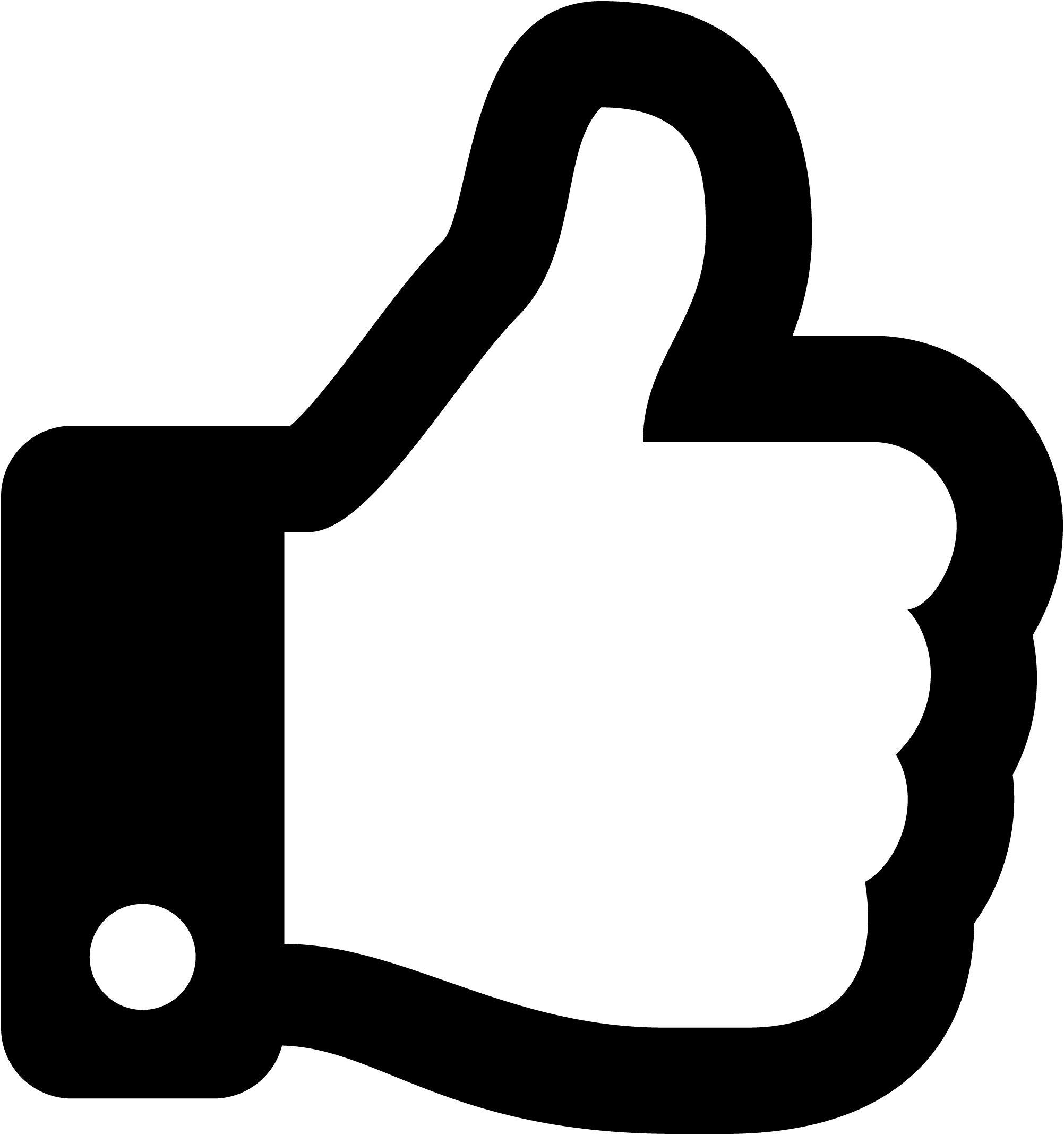
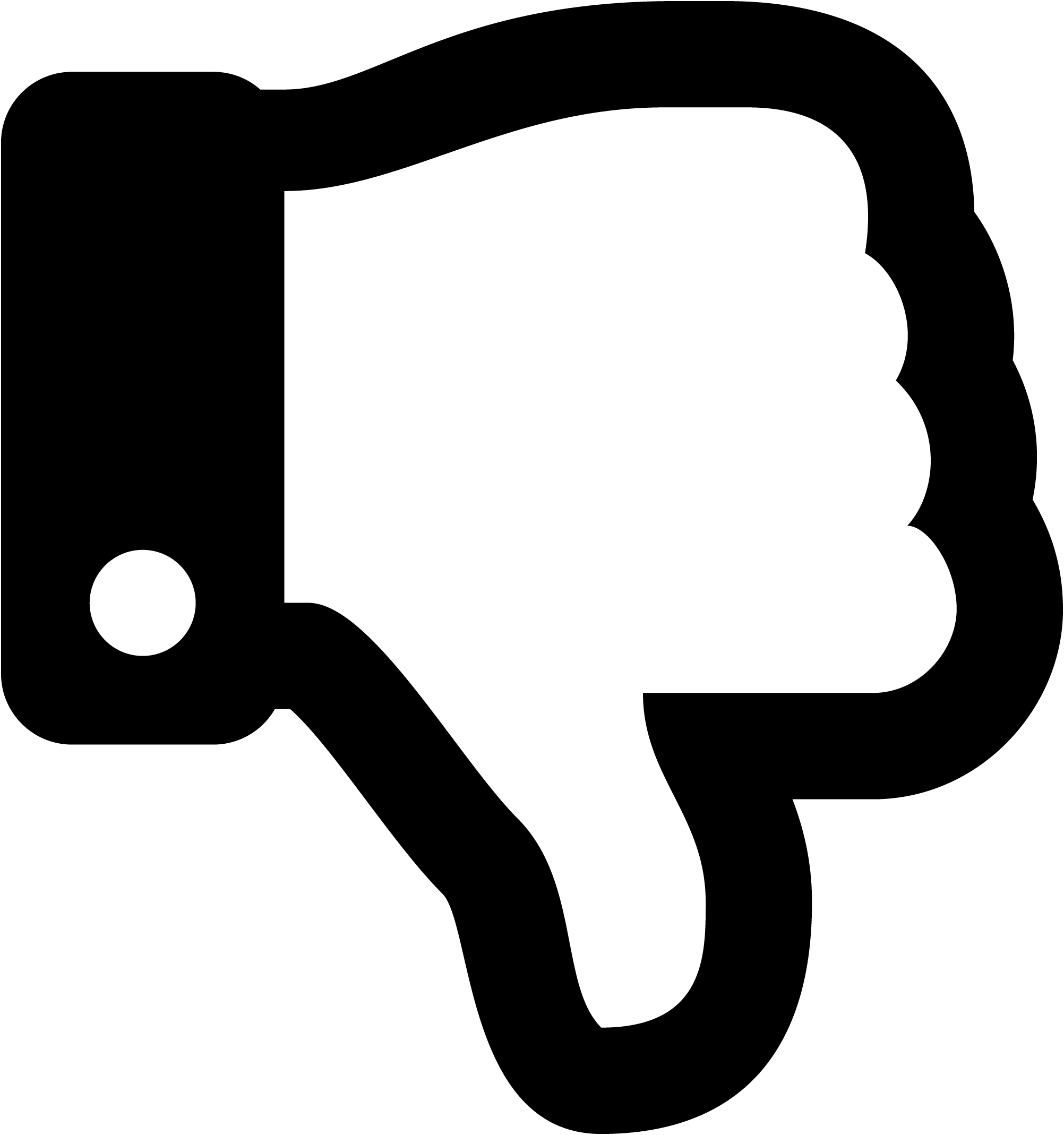
Image description/alt-tag
Image caption
Image link
Rollover Image (max size: 2mb)
Or drag a symbol into the upload area
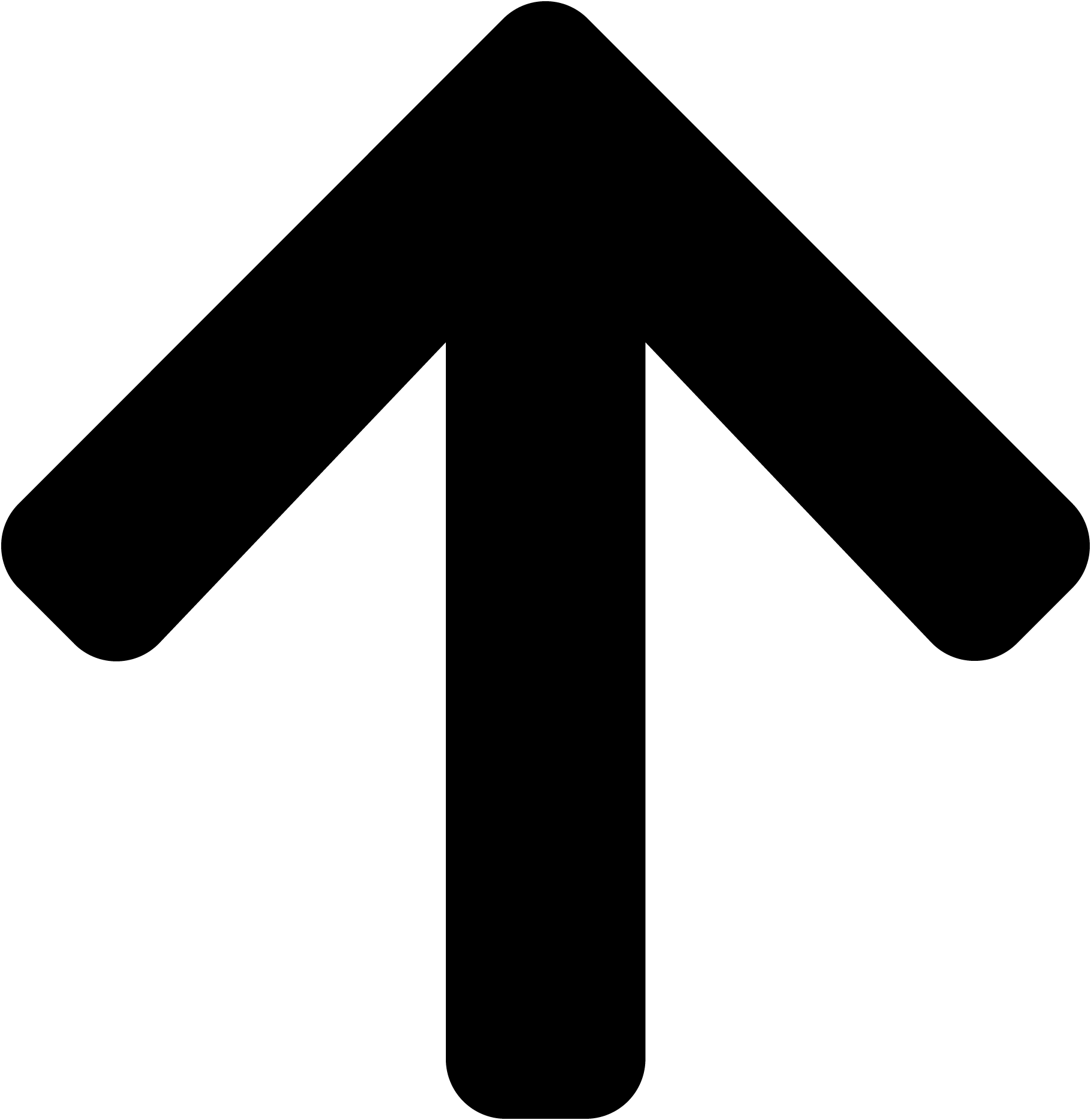
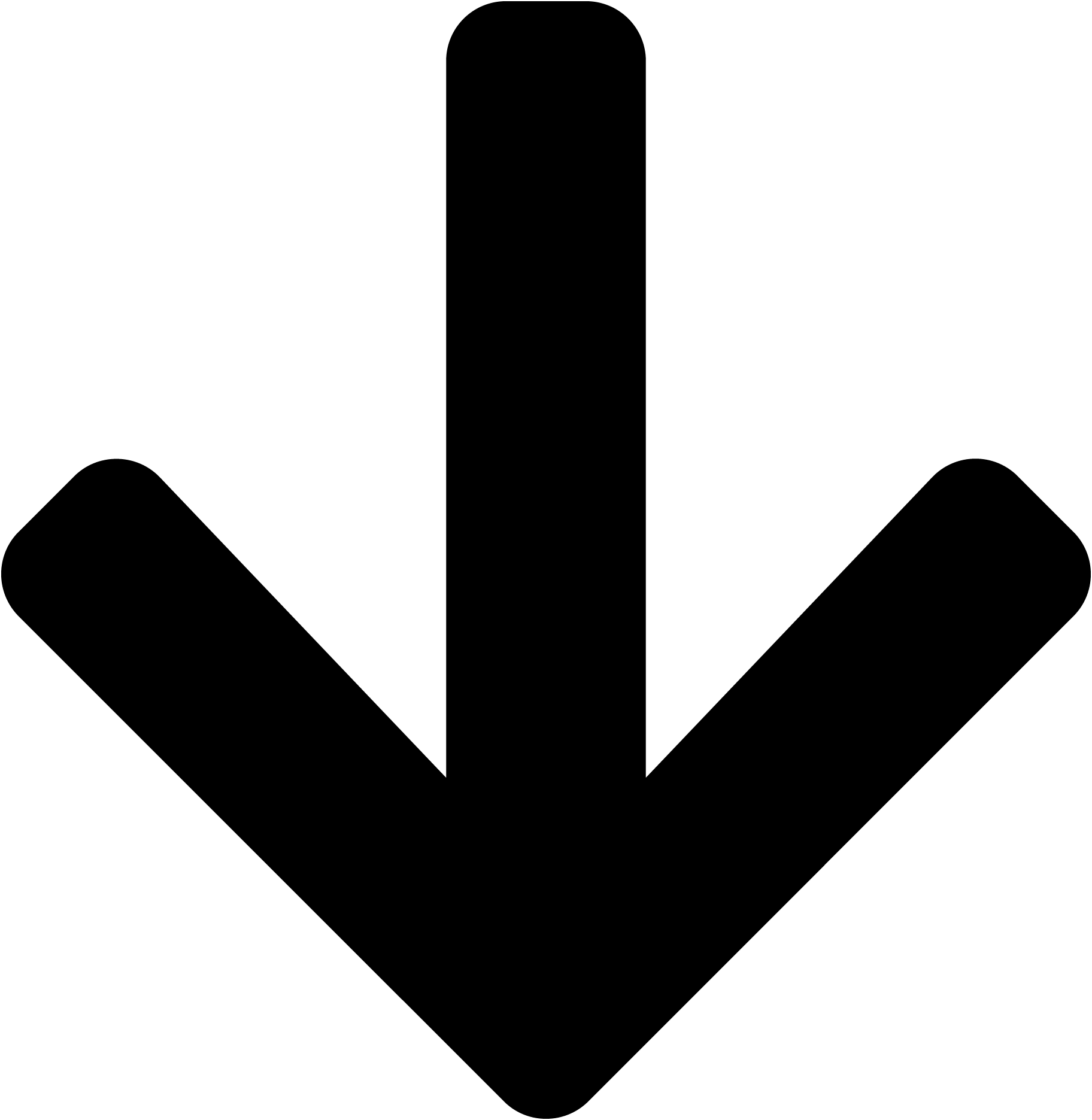
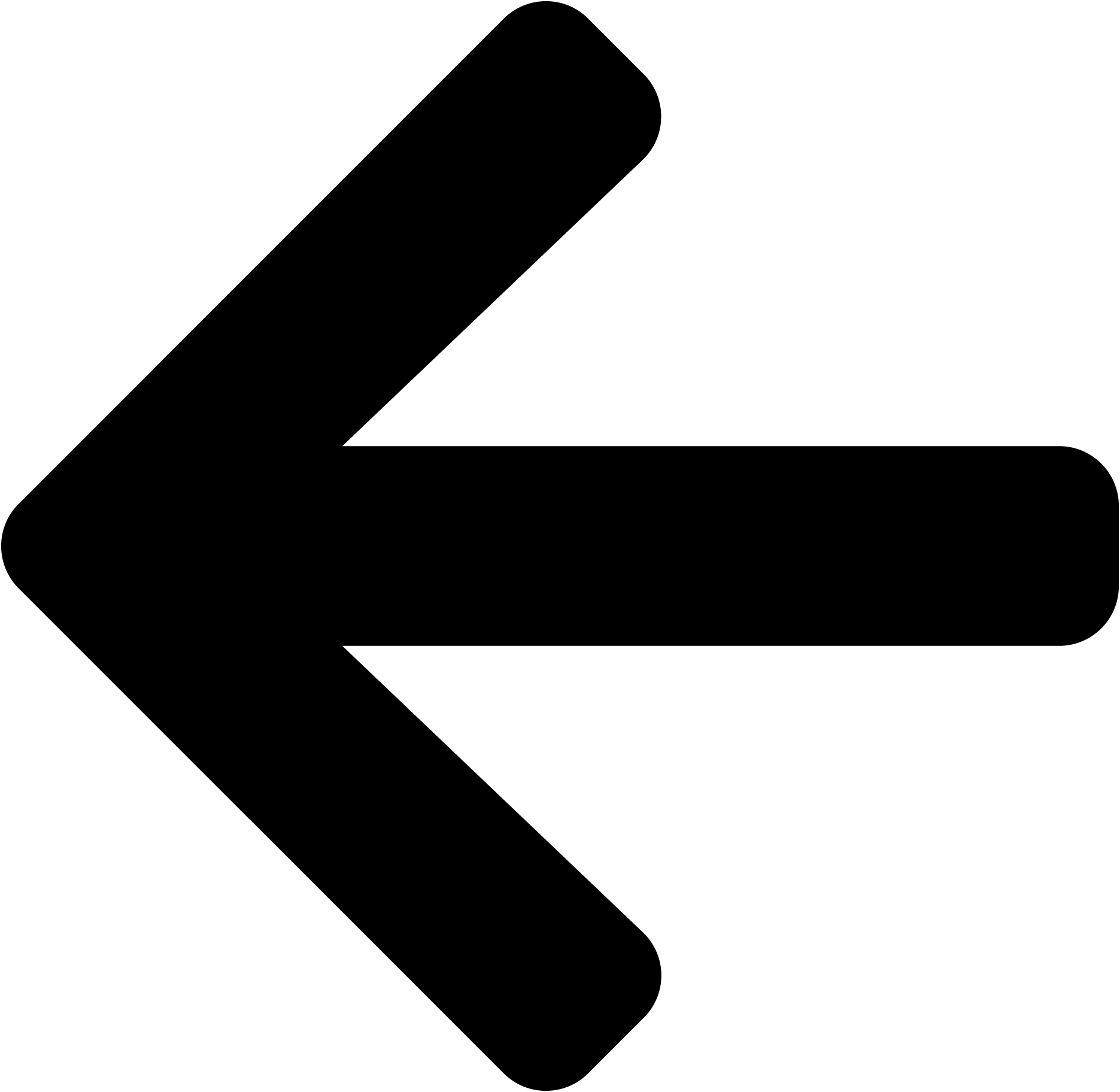
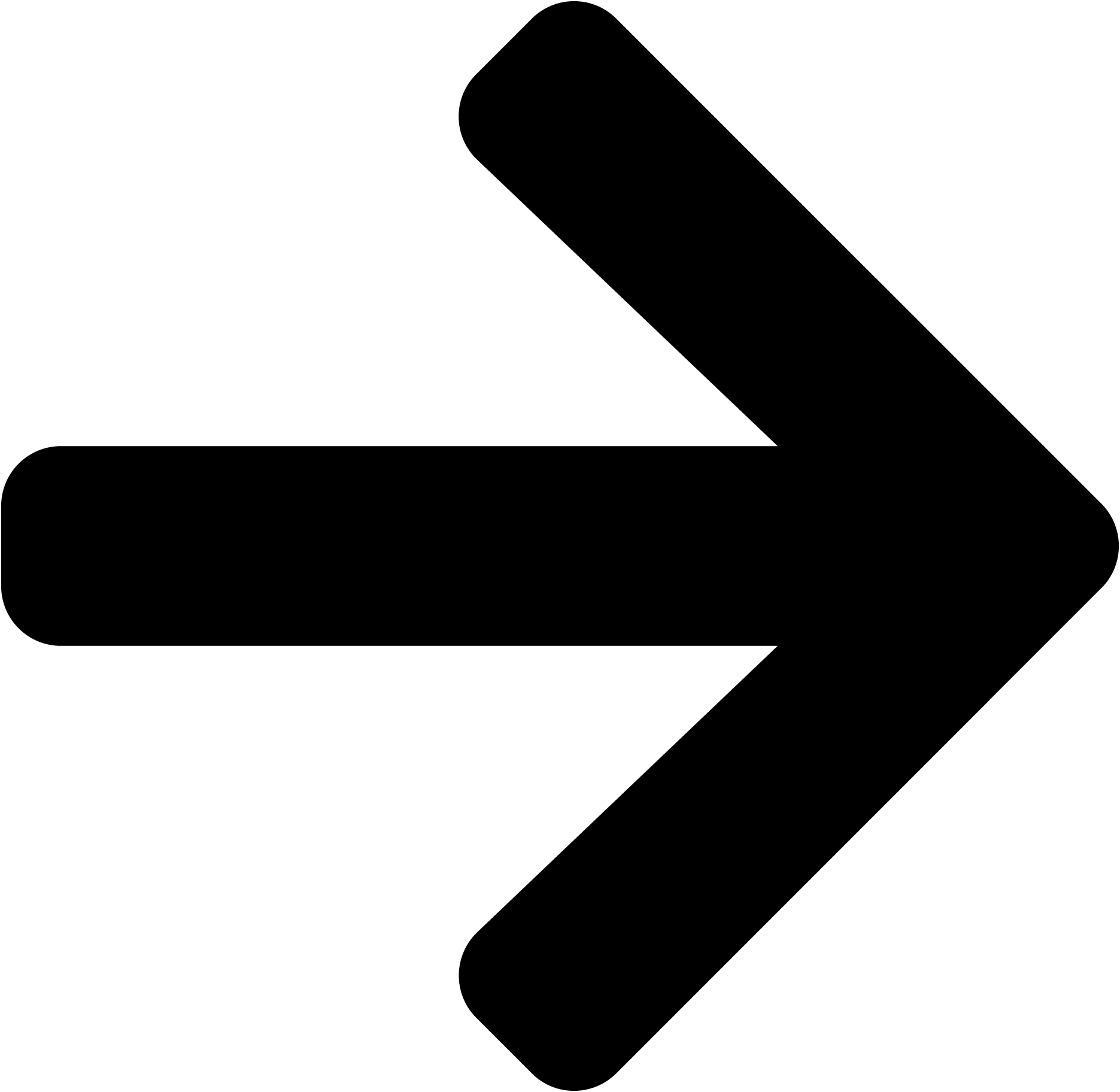
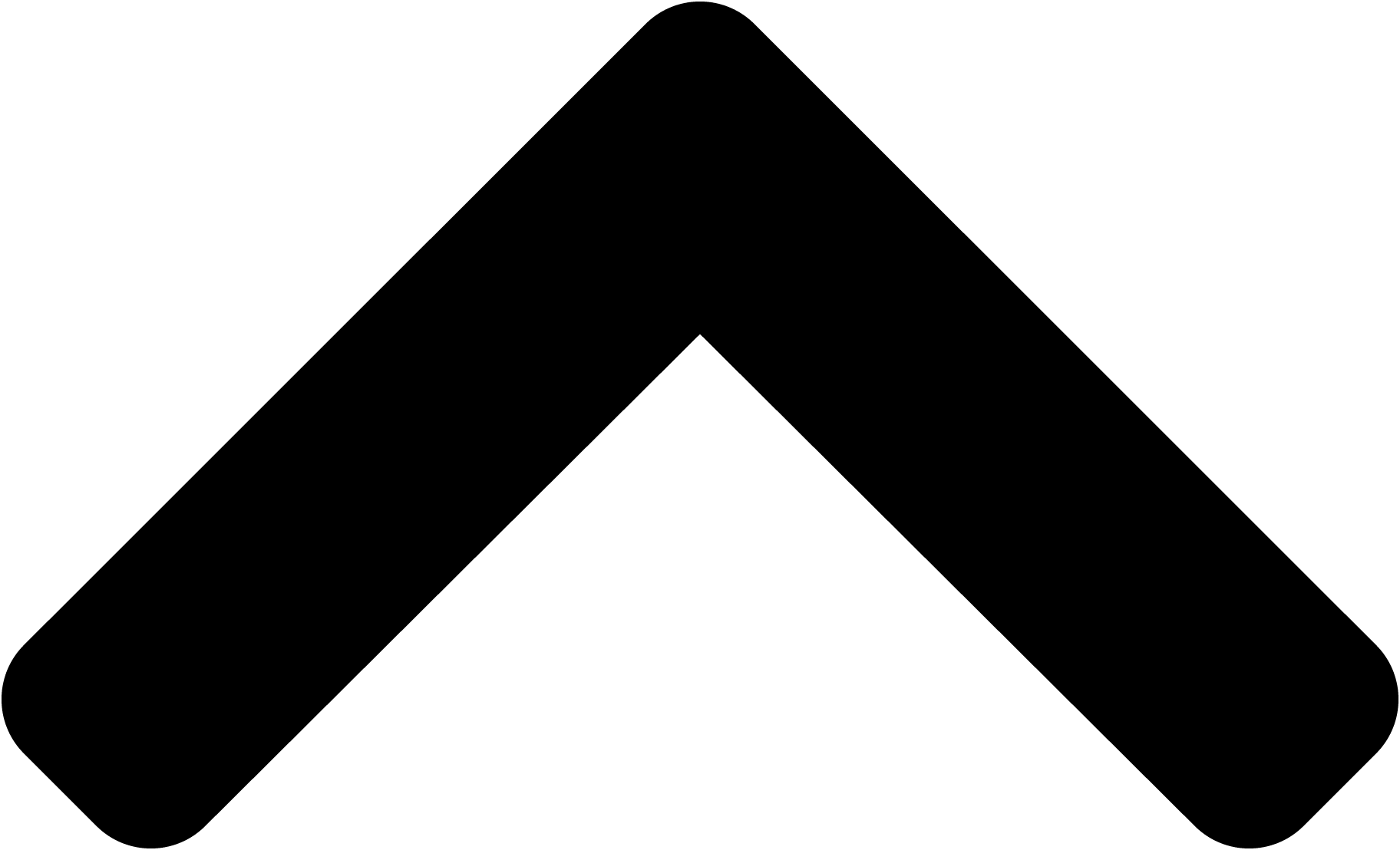
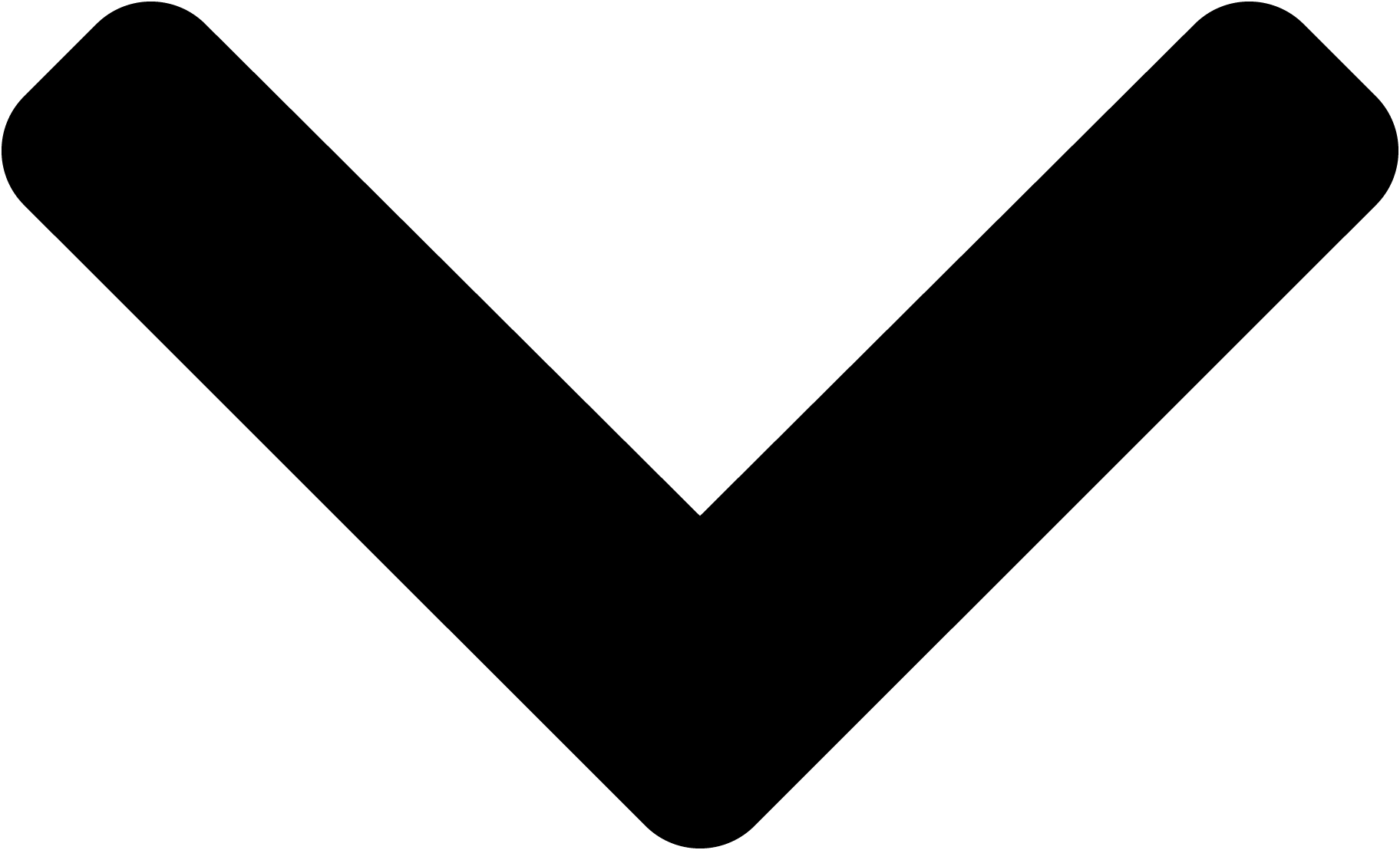
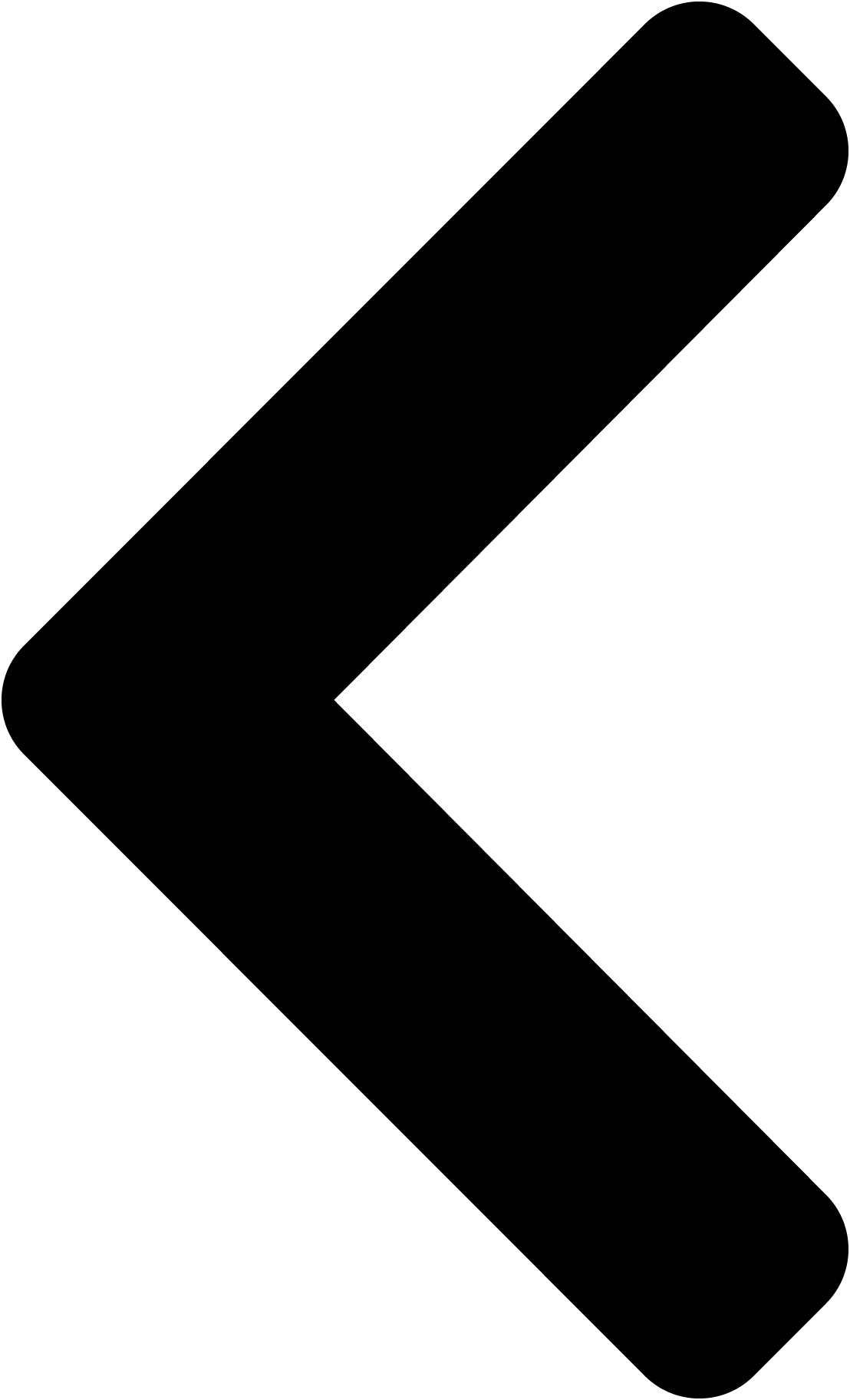
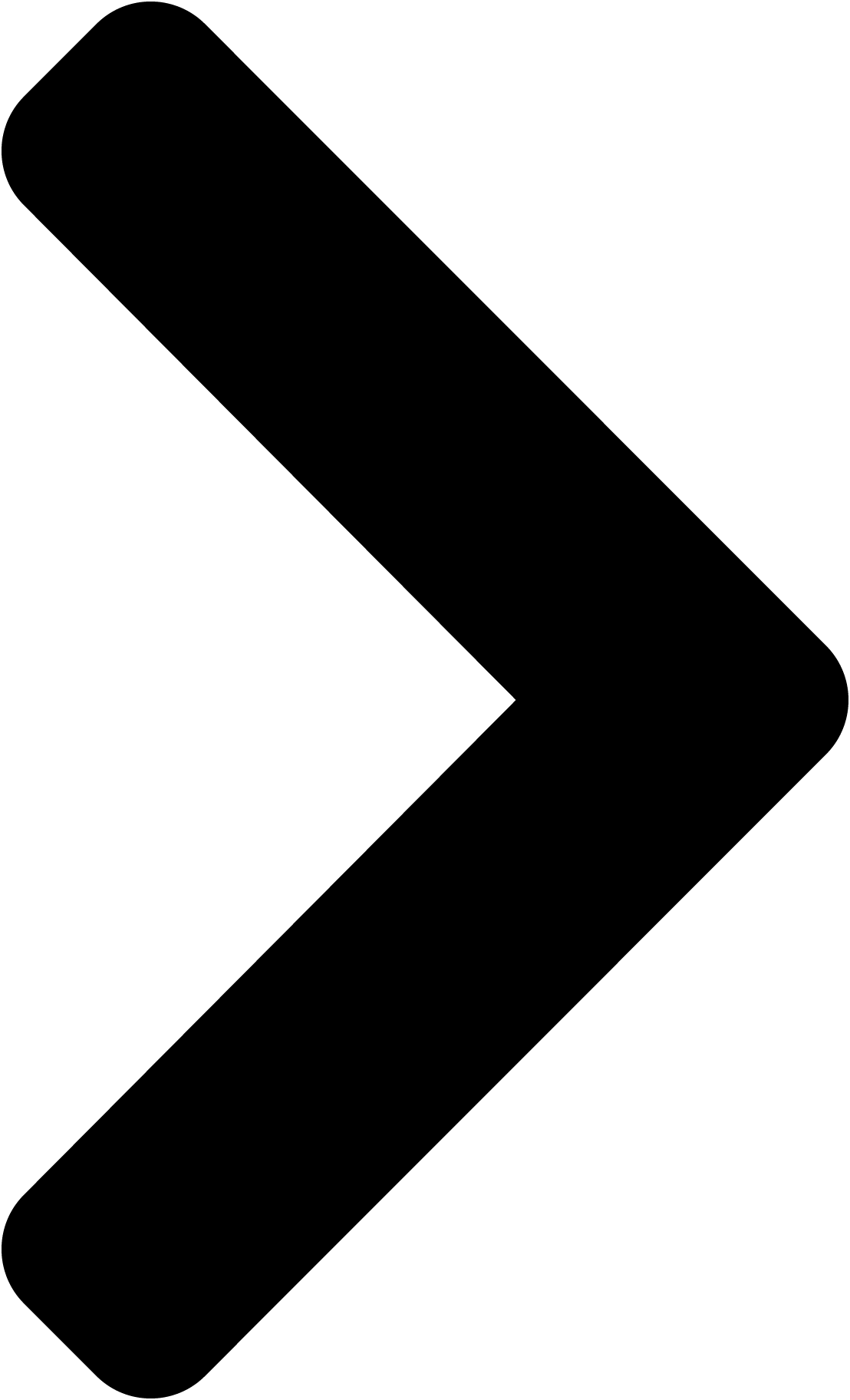
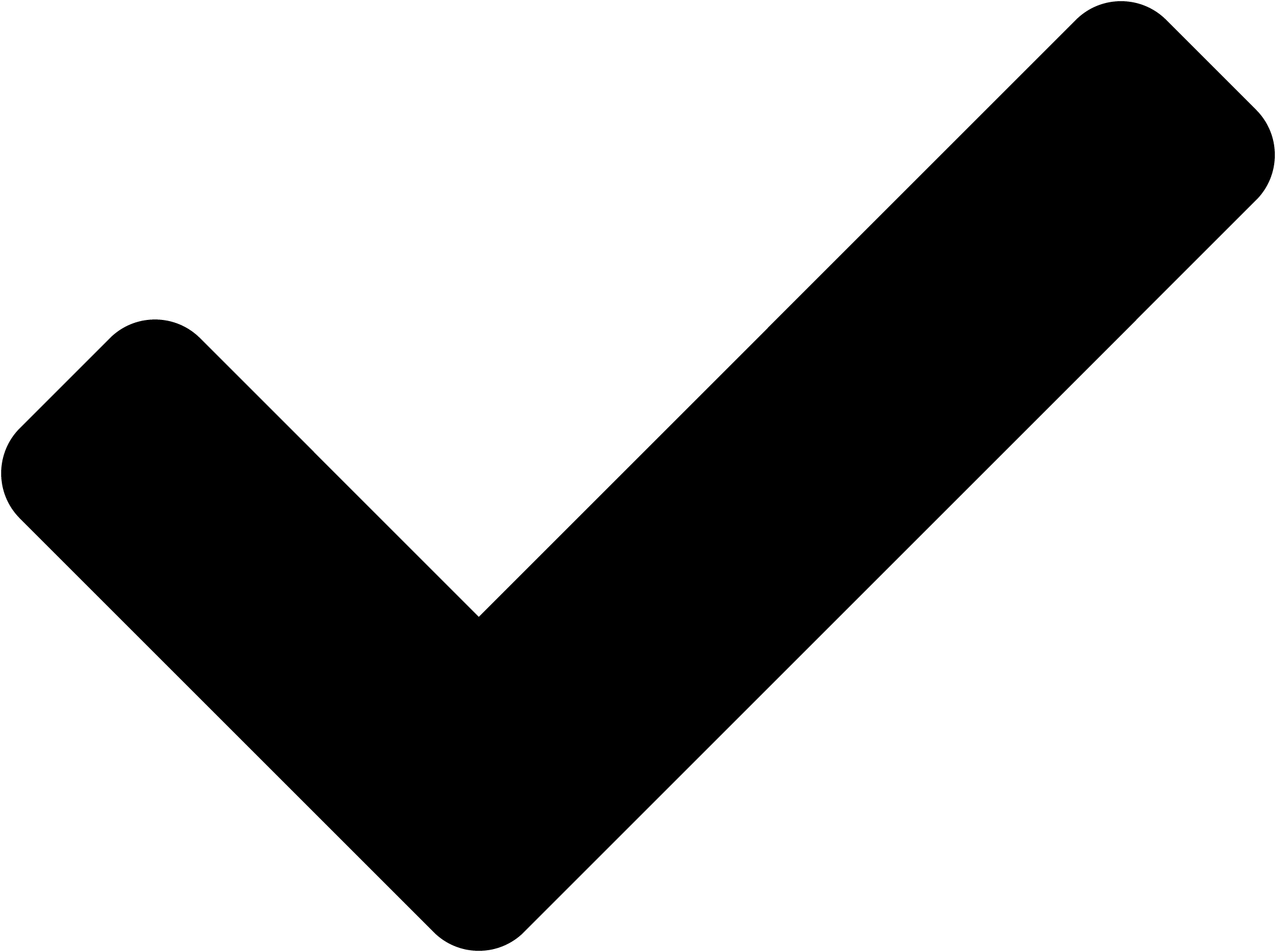
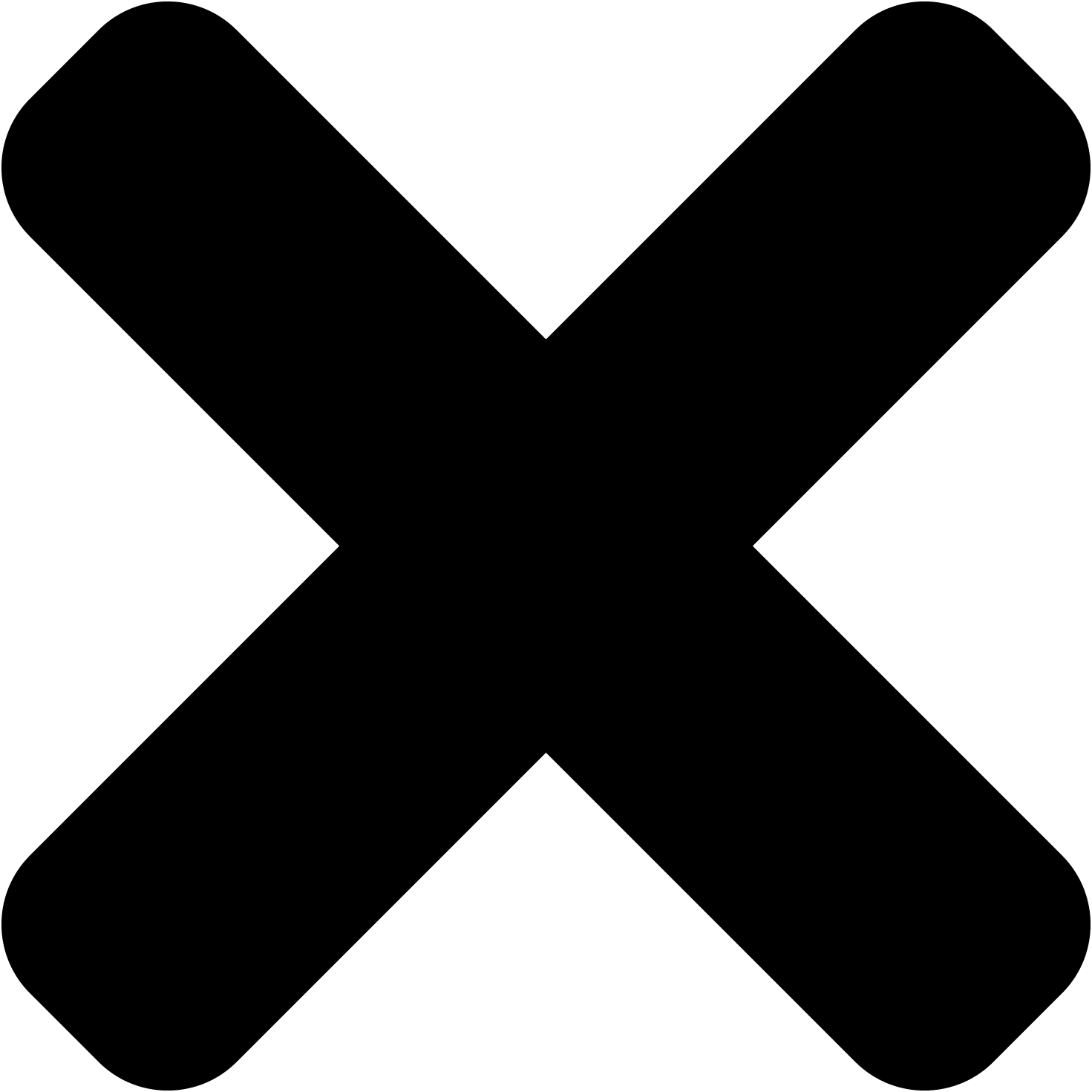
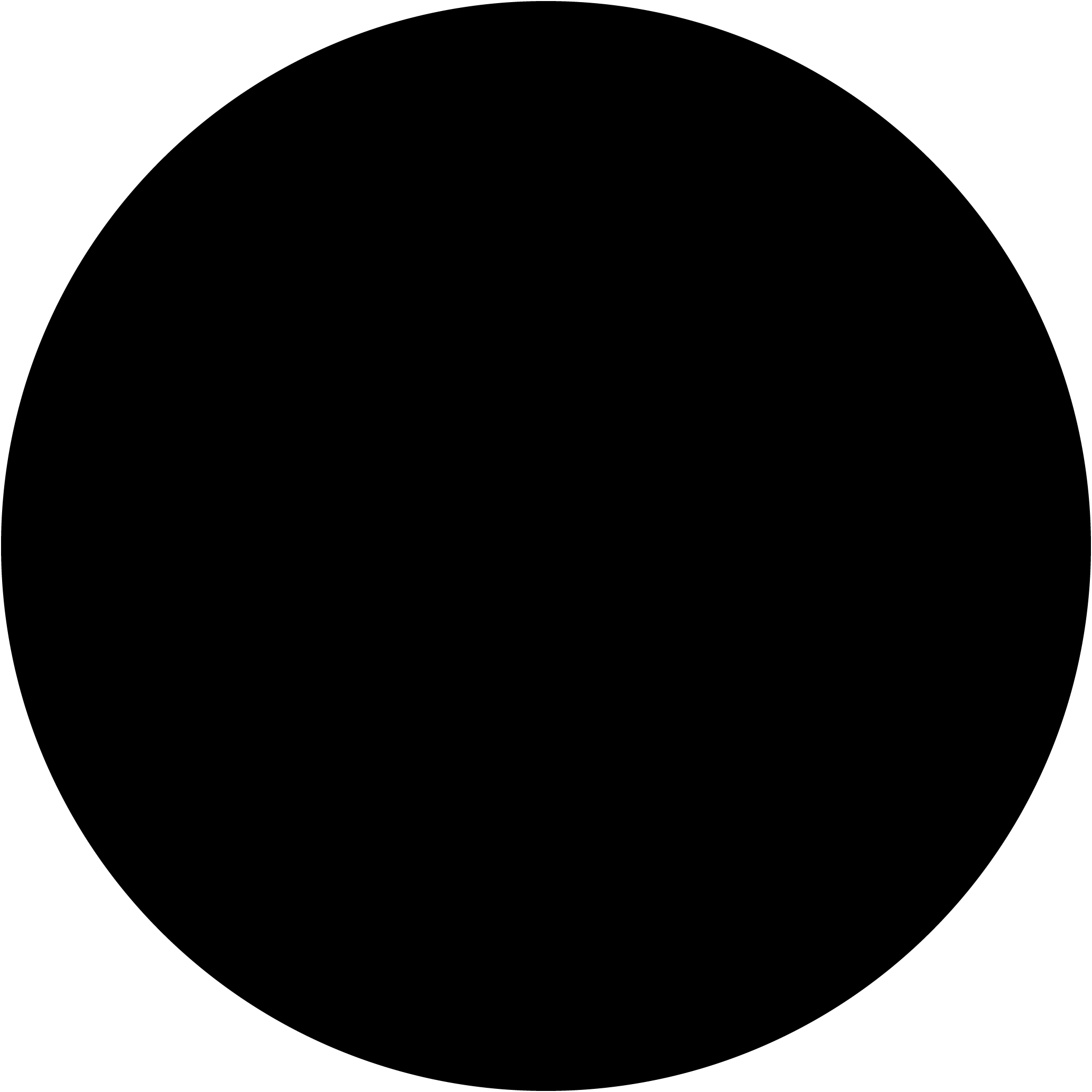
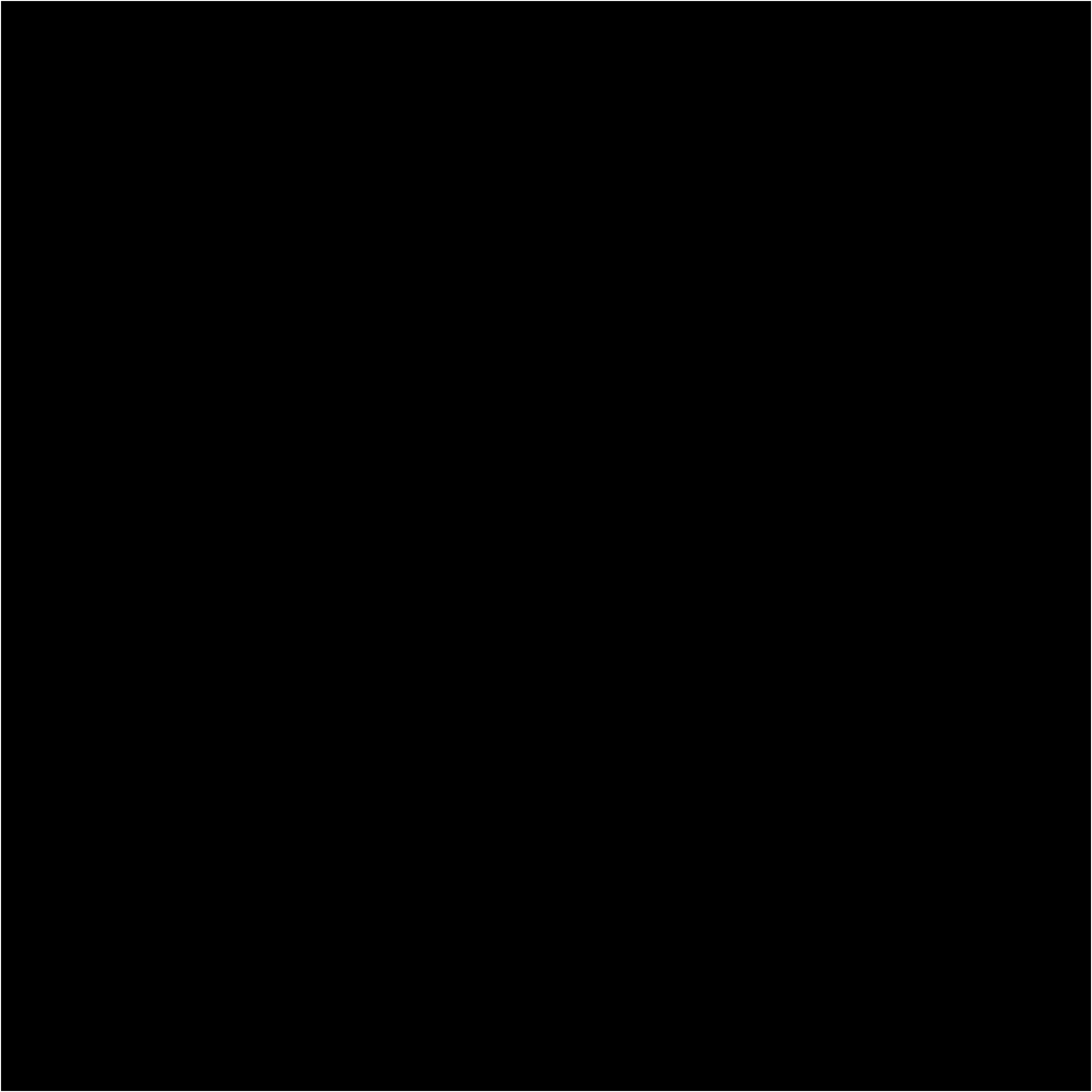
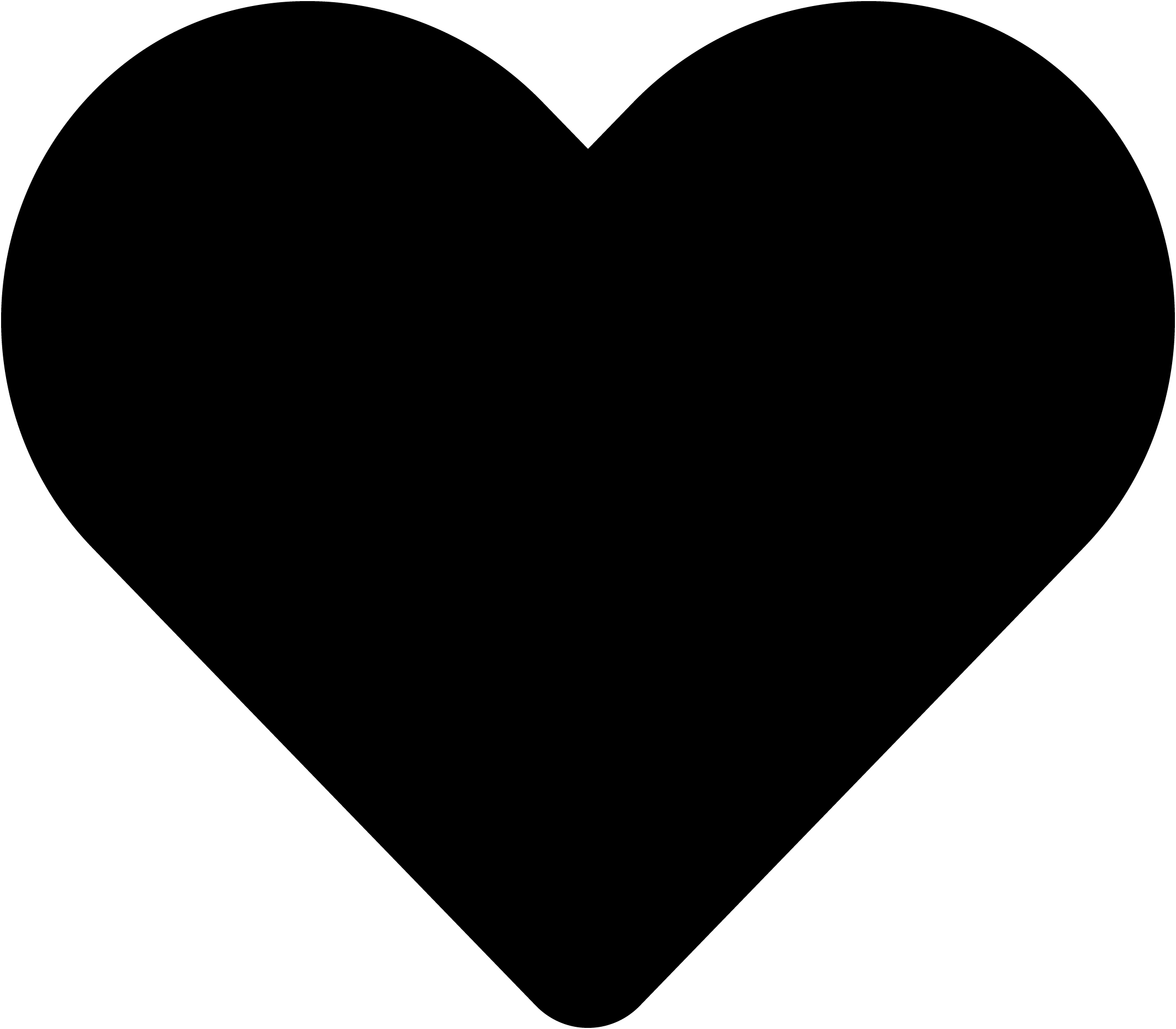
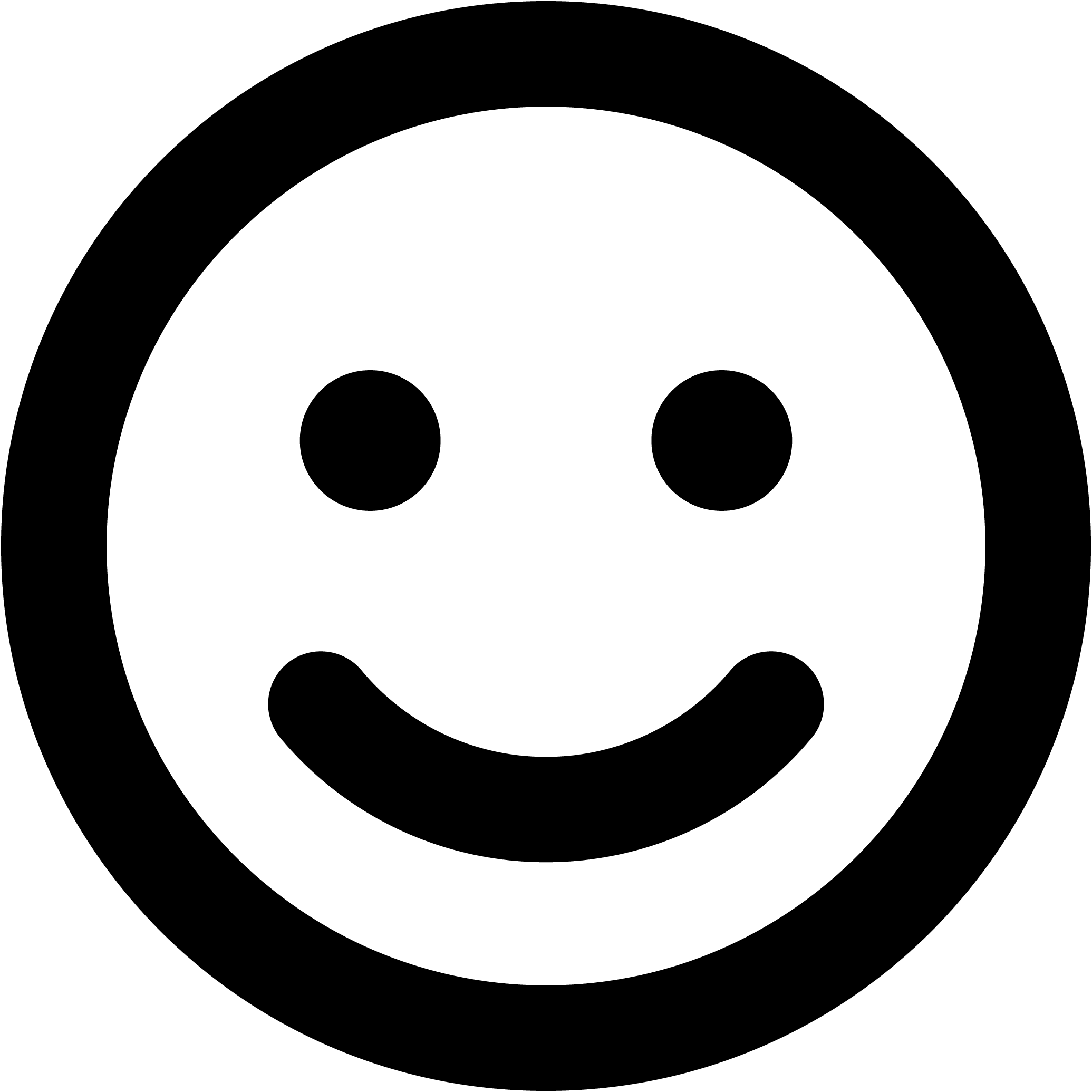
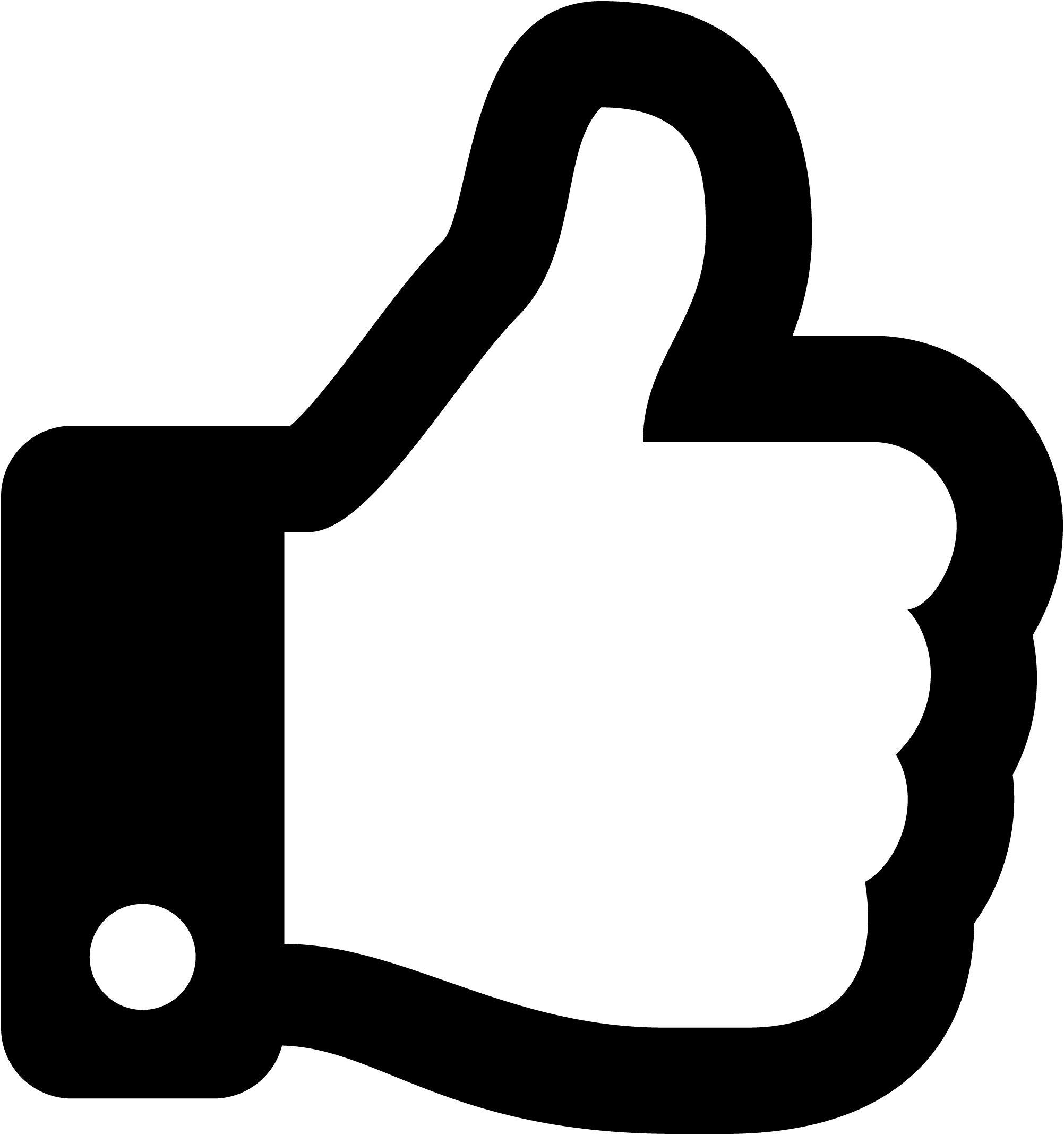
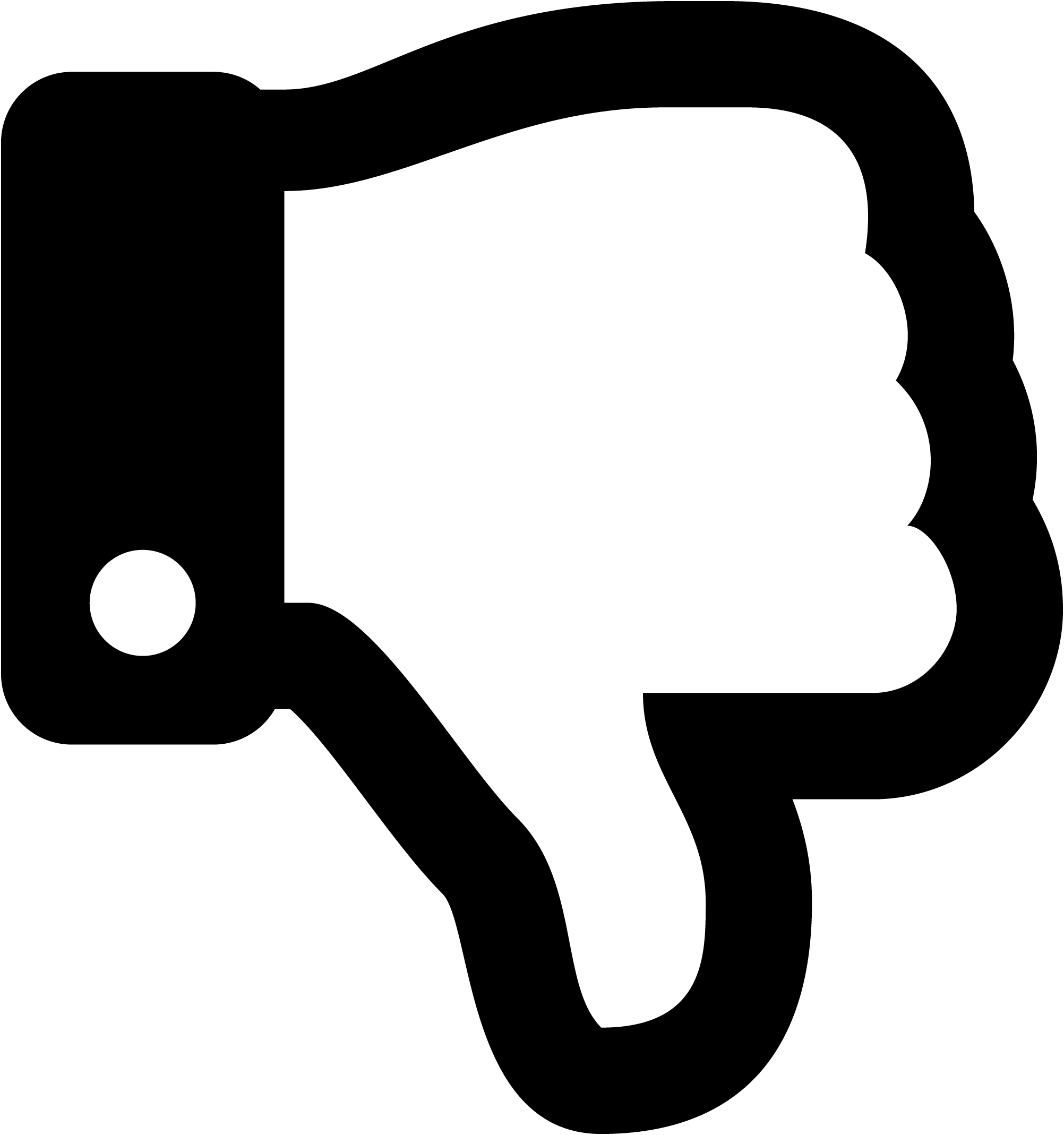
Border colour
Rotate
Skew (x-axis)
Skew (y-axis)
Image (max size: 2mb)
Or drag a symbol into the upload area
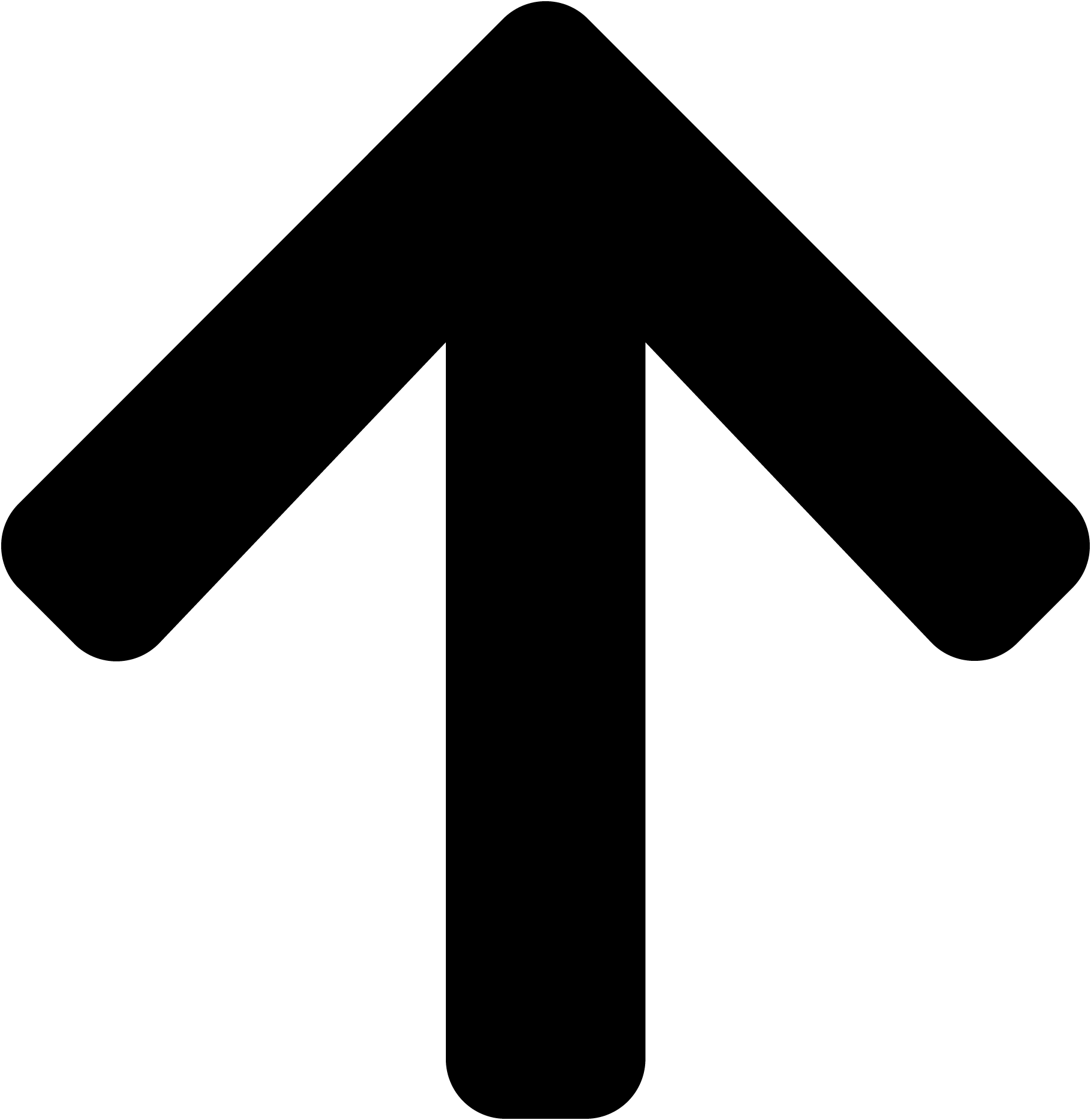
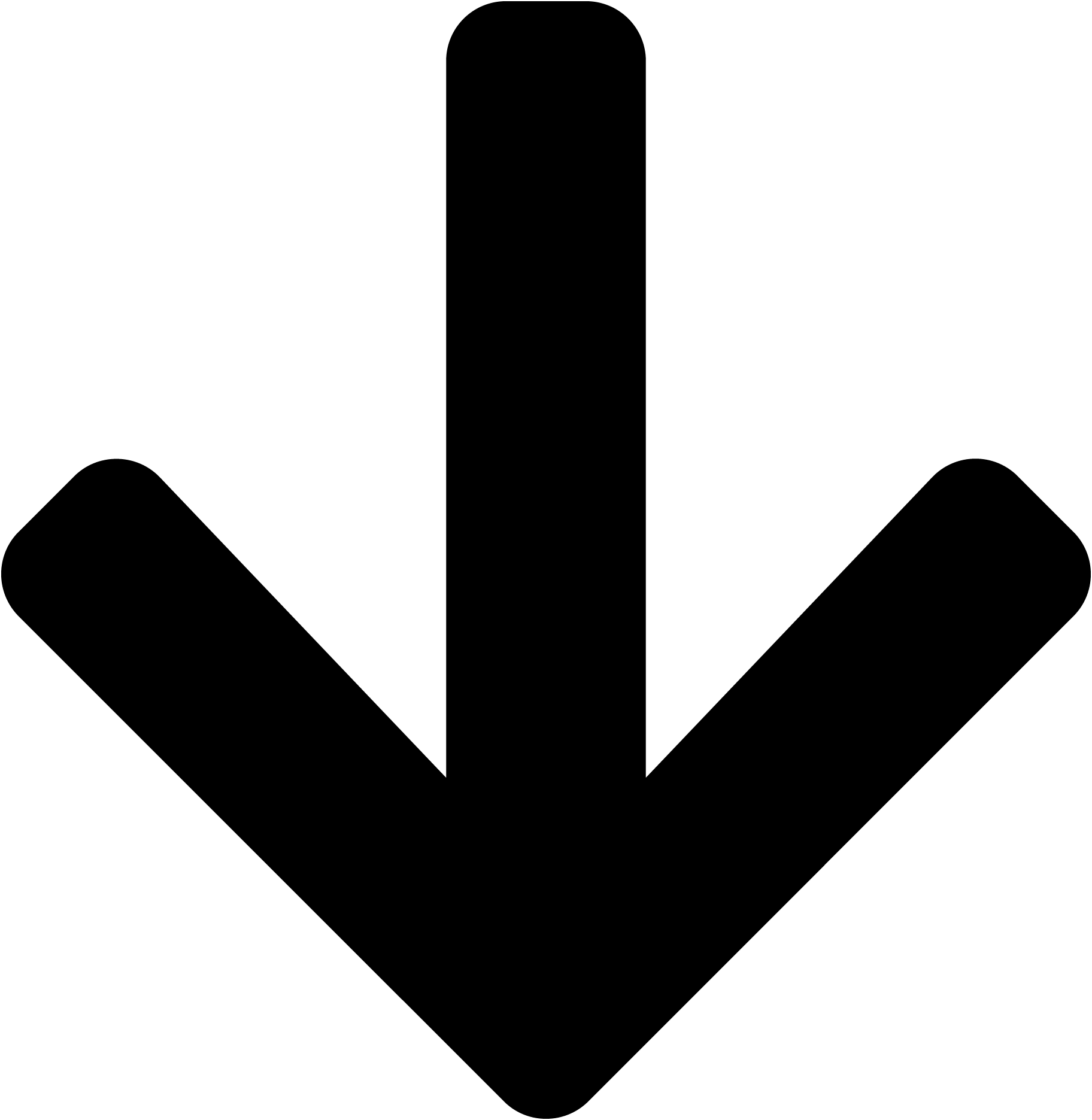
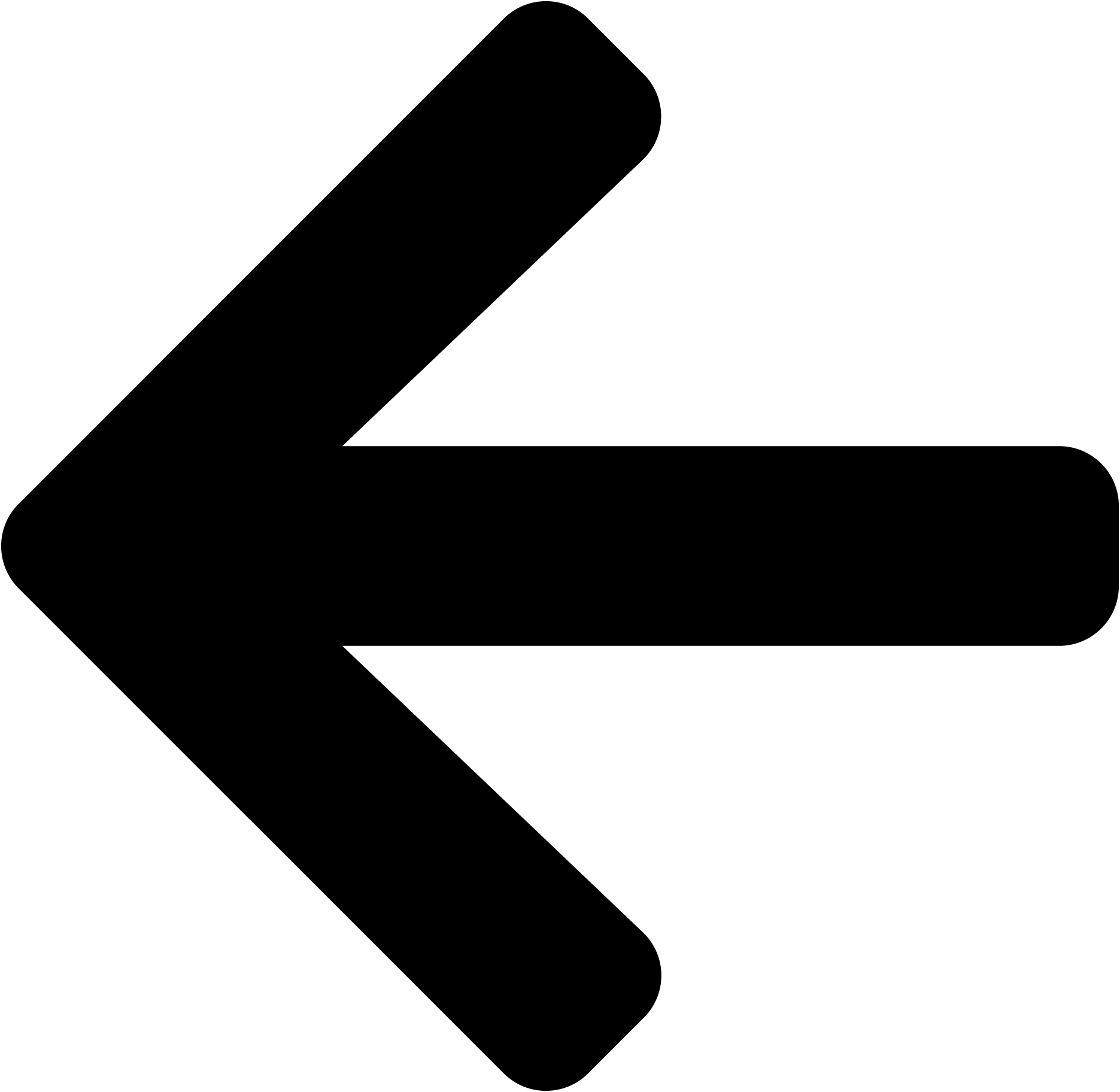
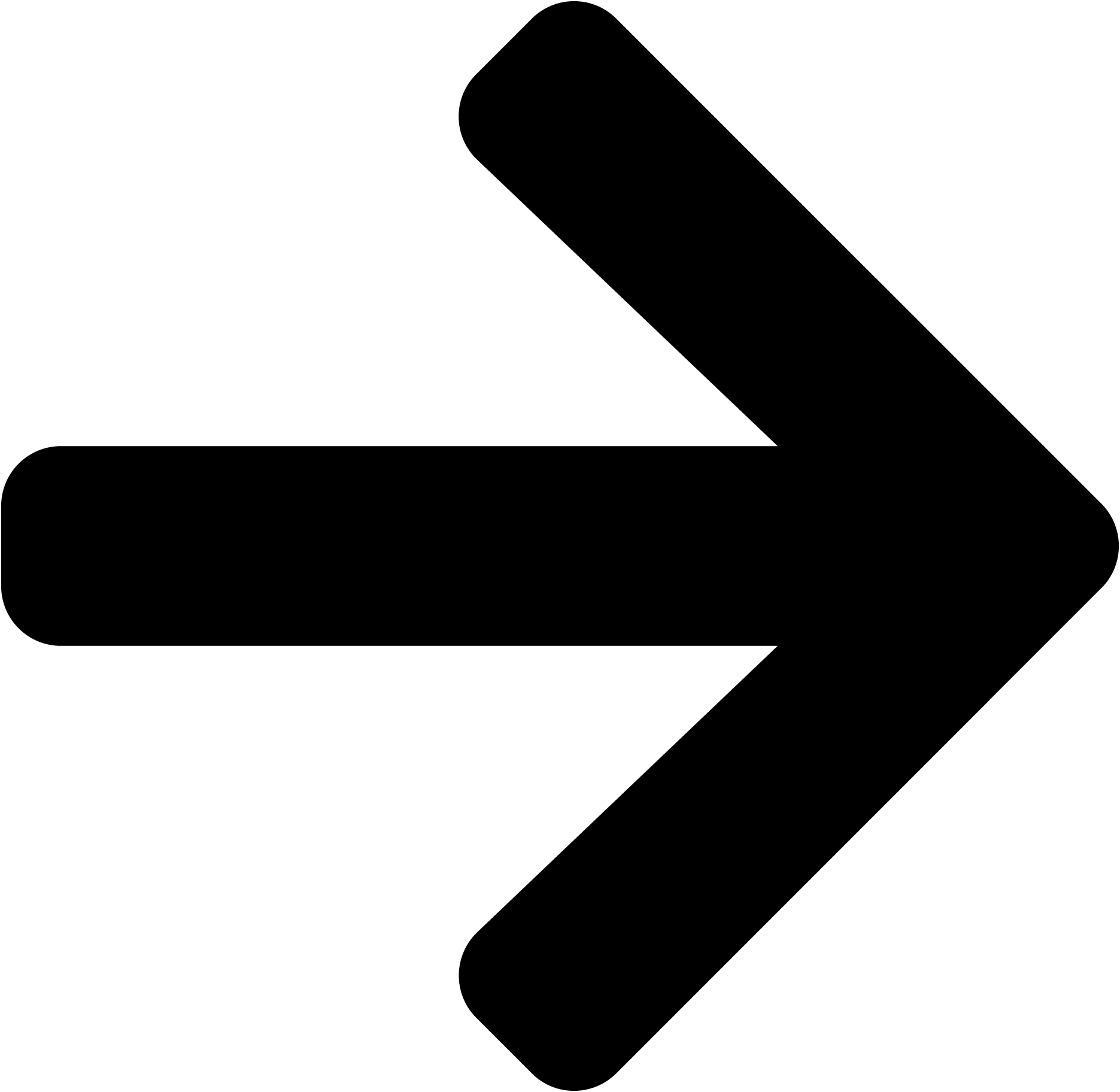
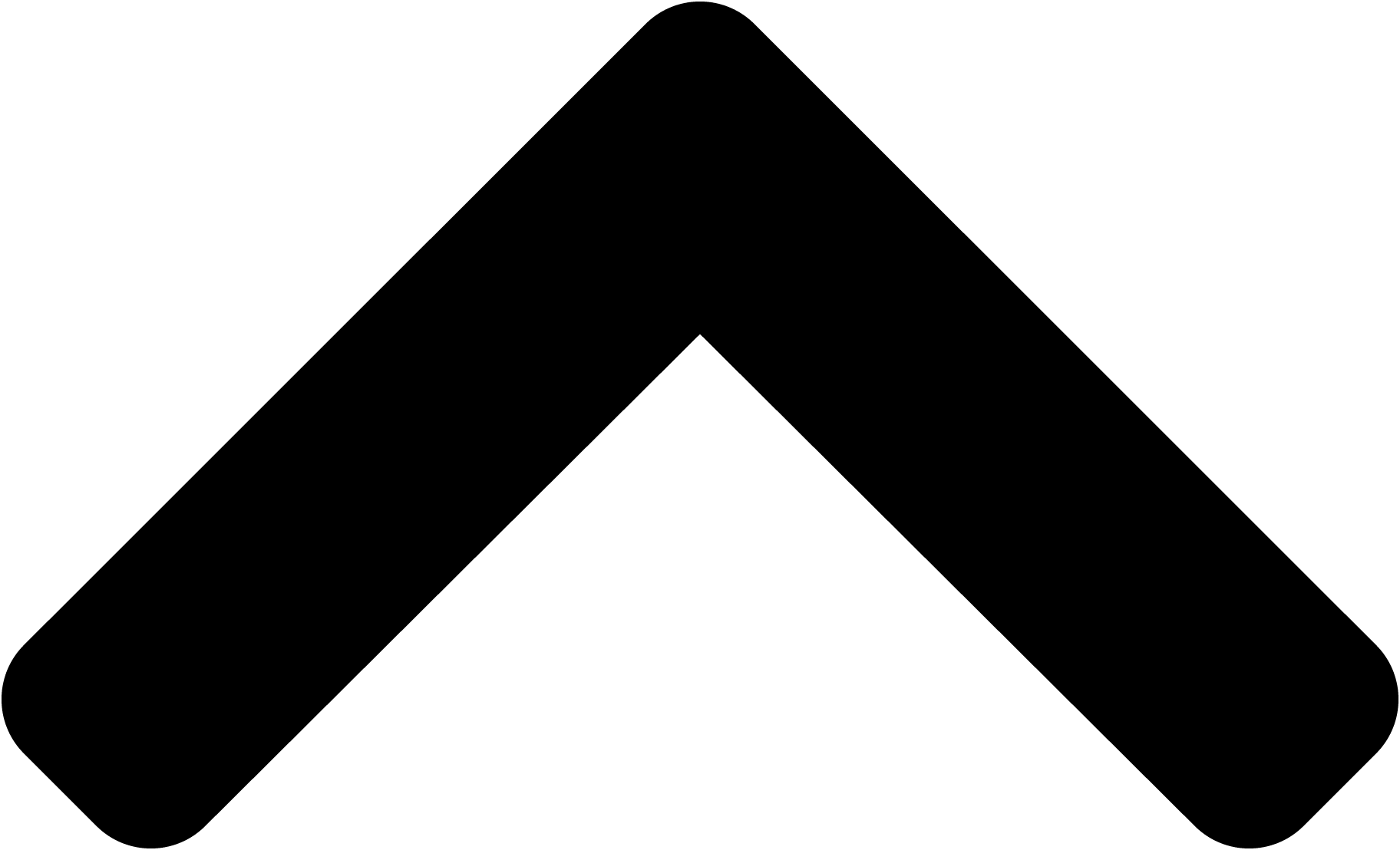
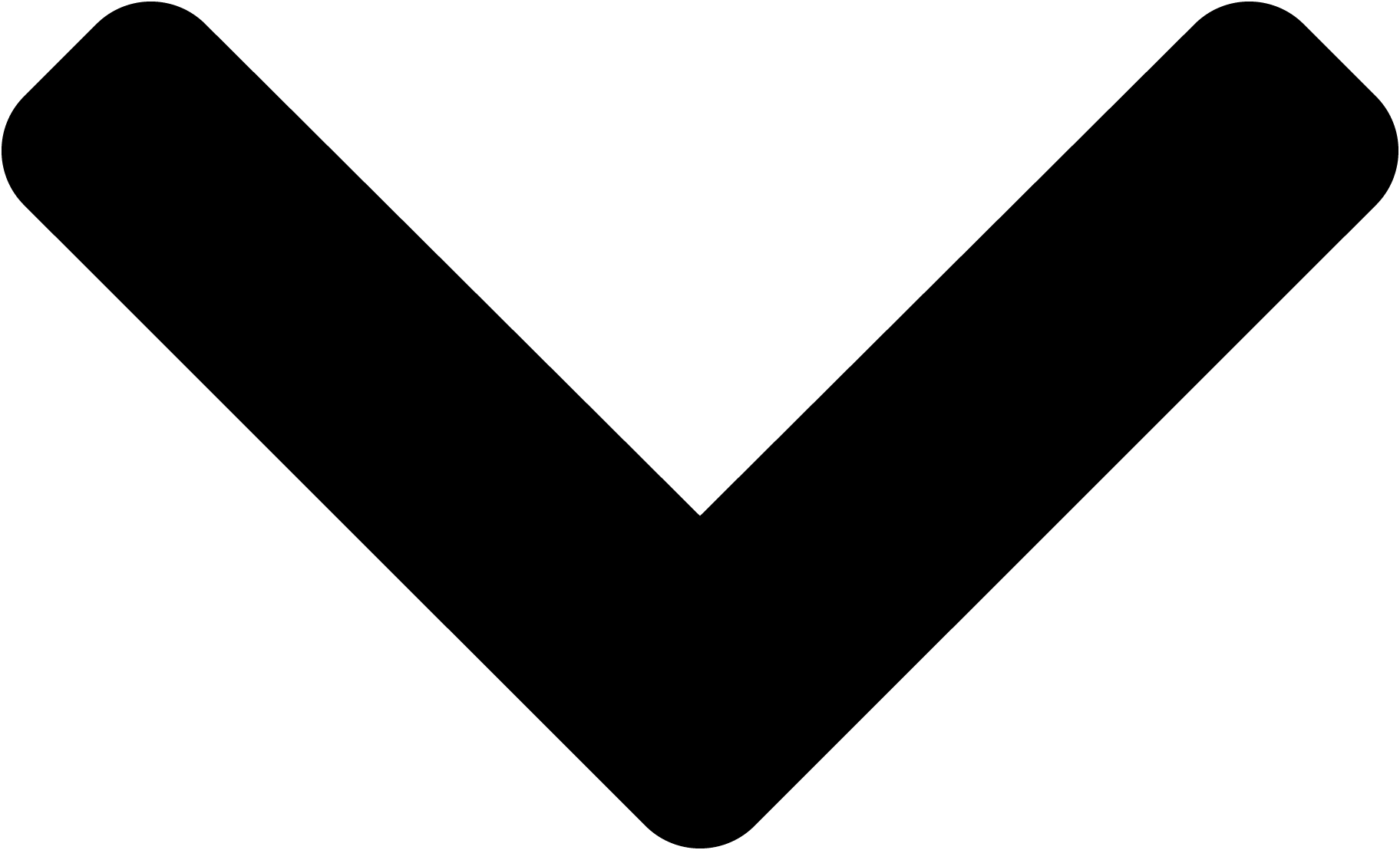
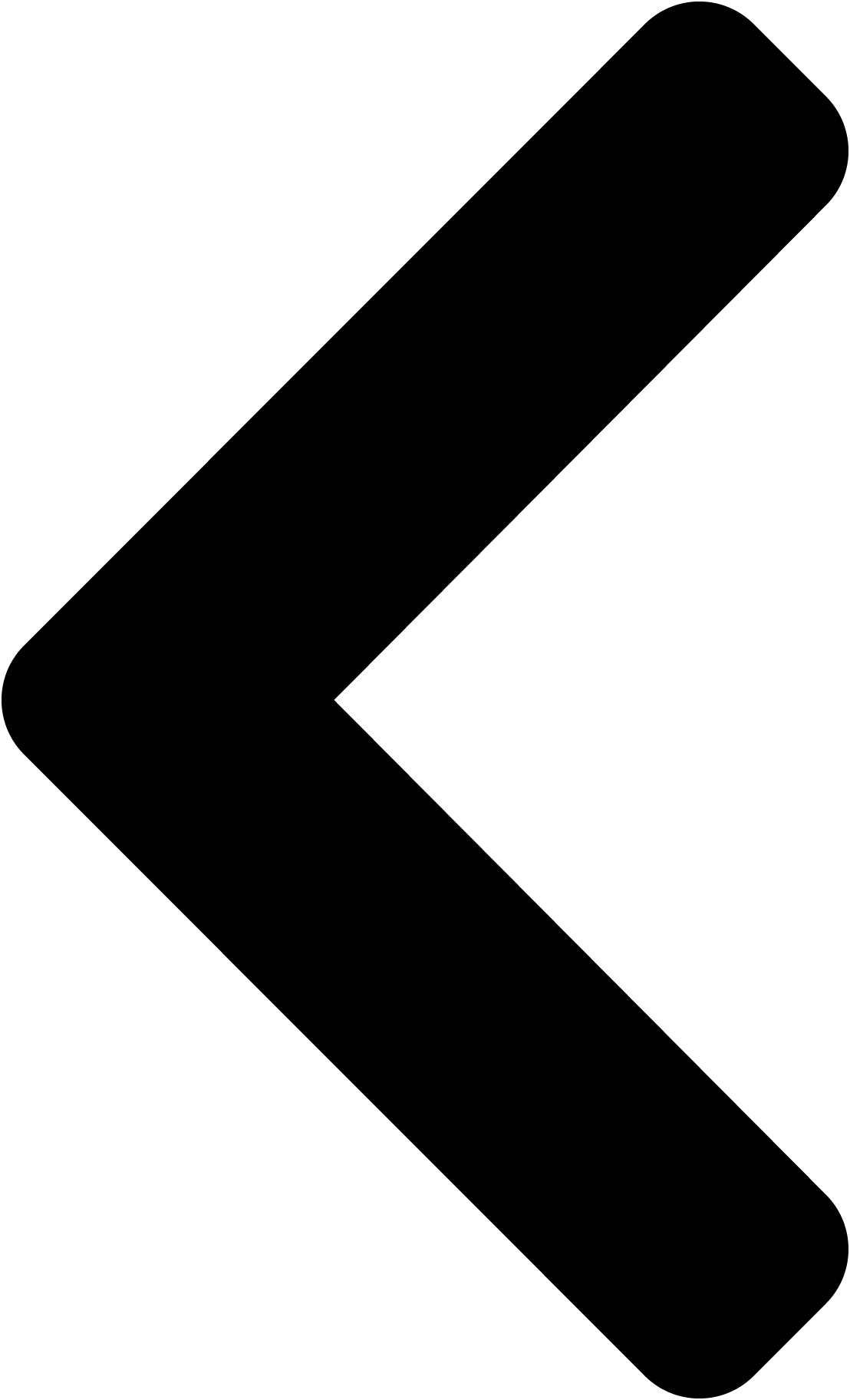
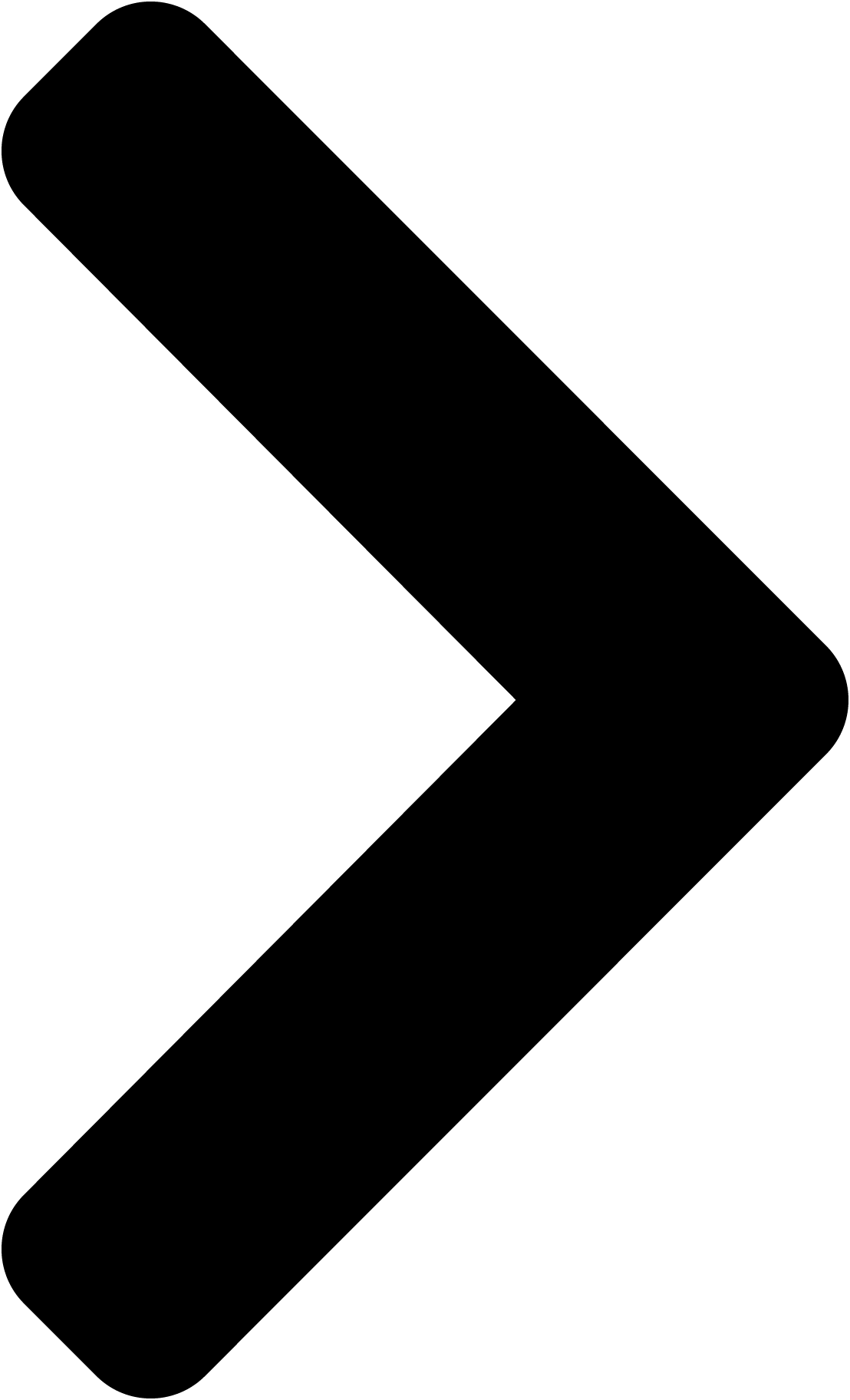
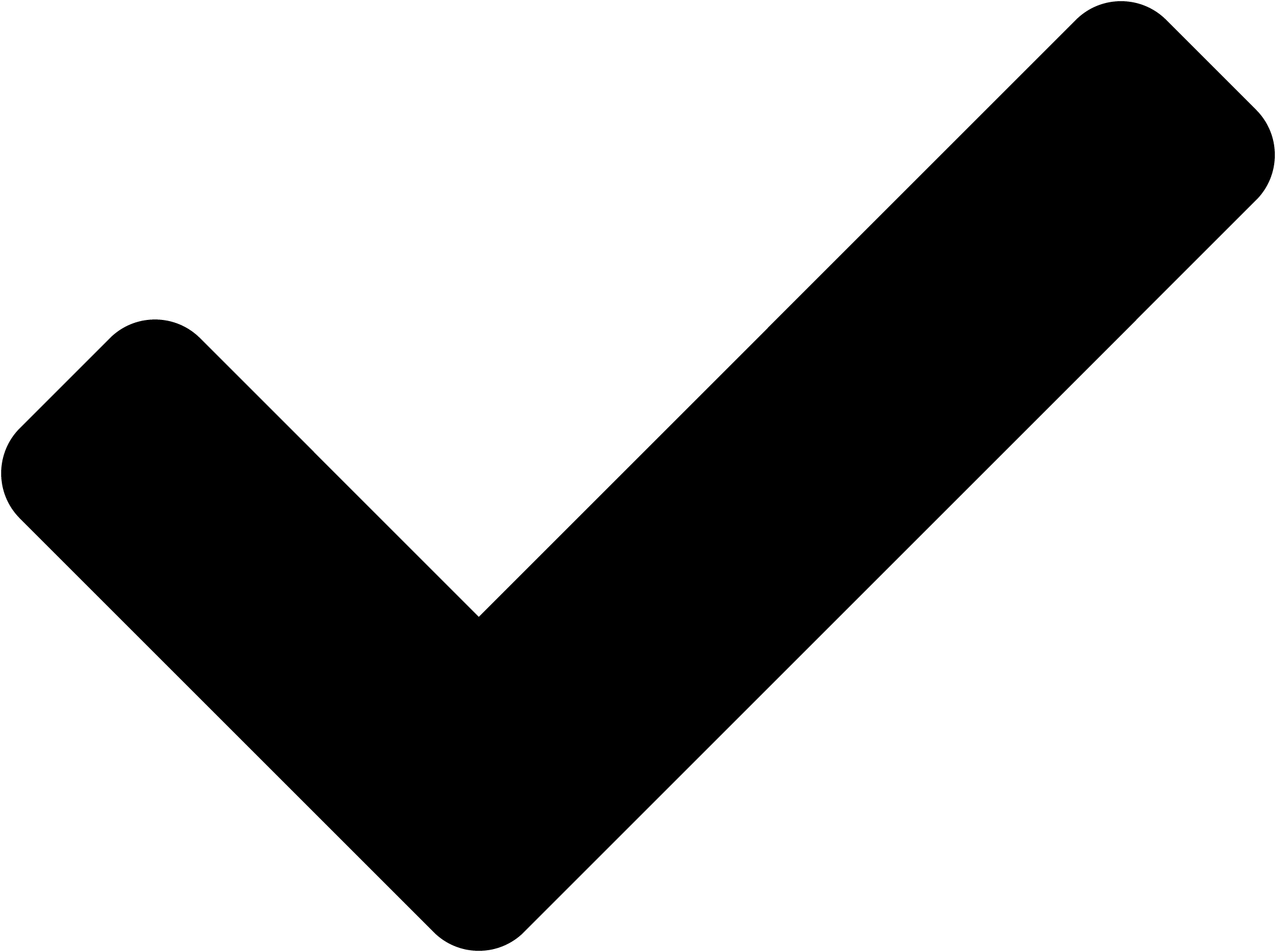
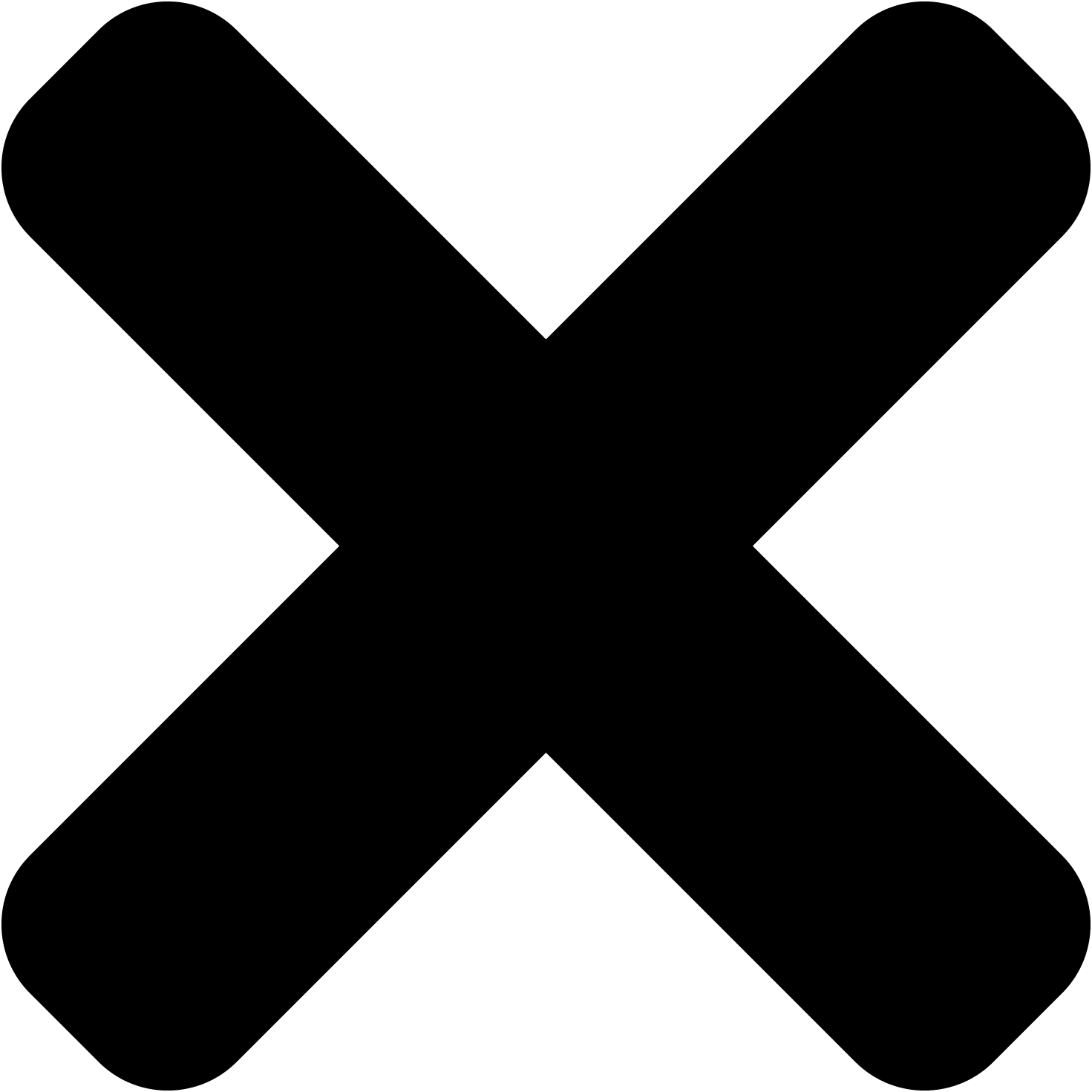
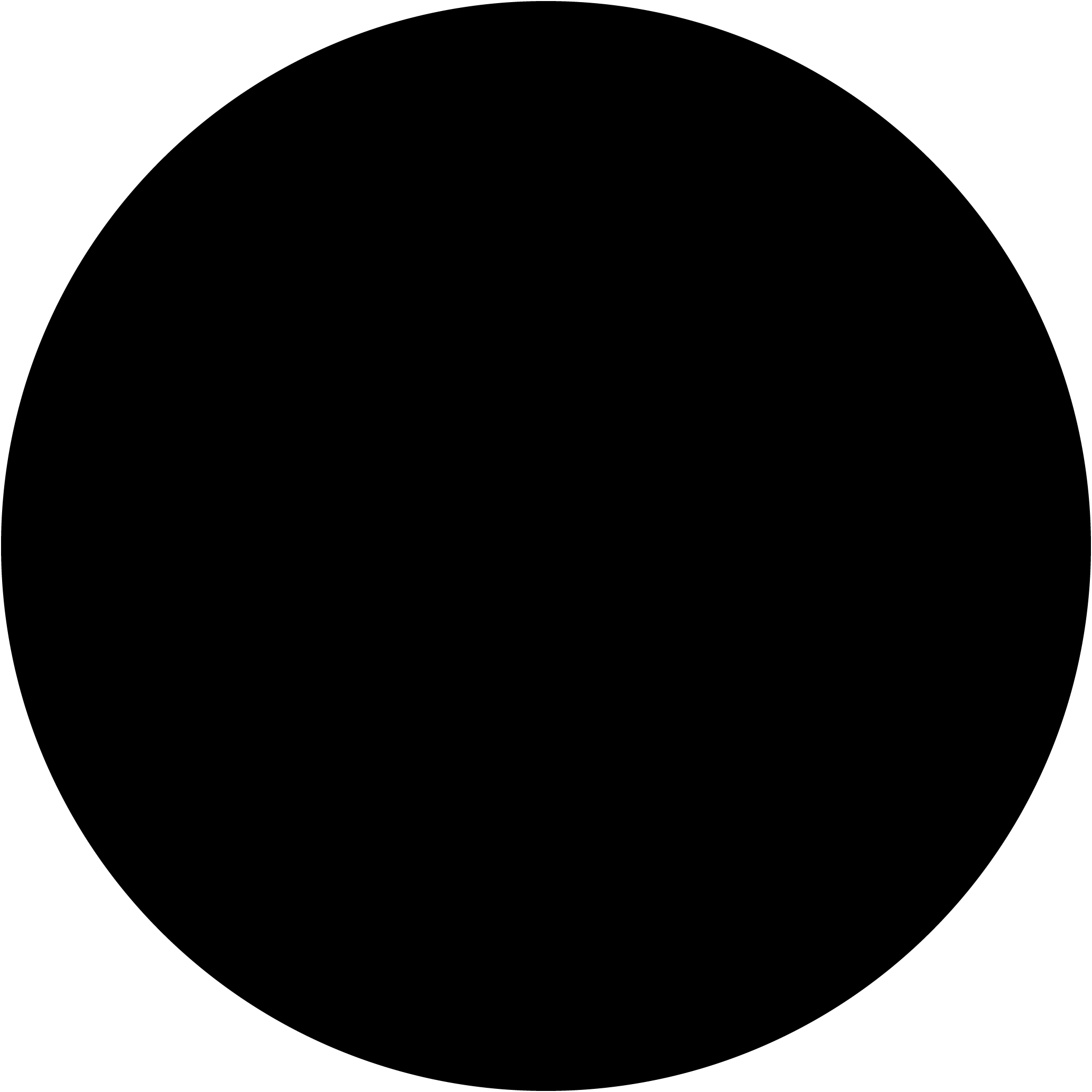
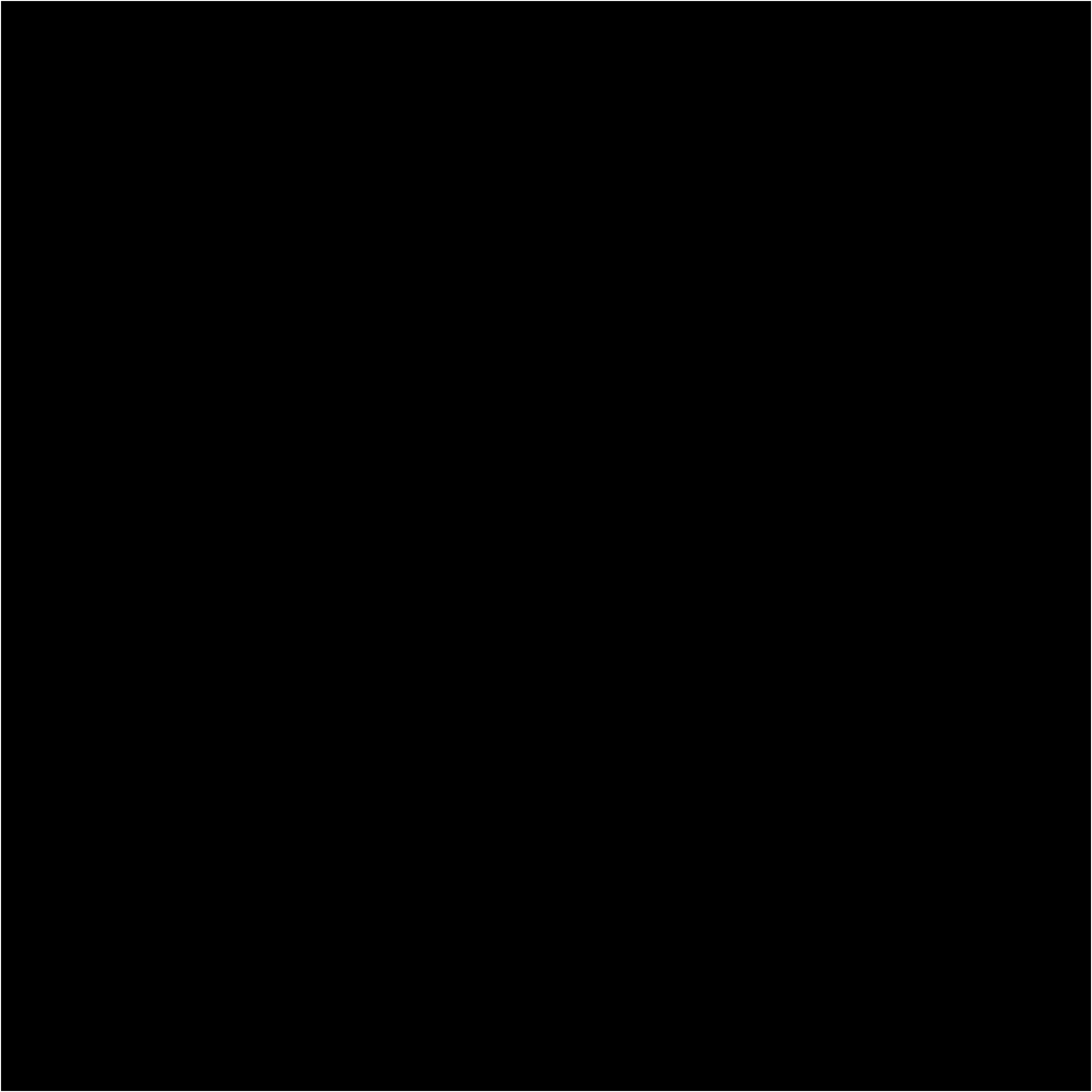
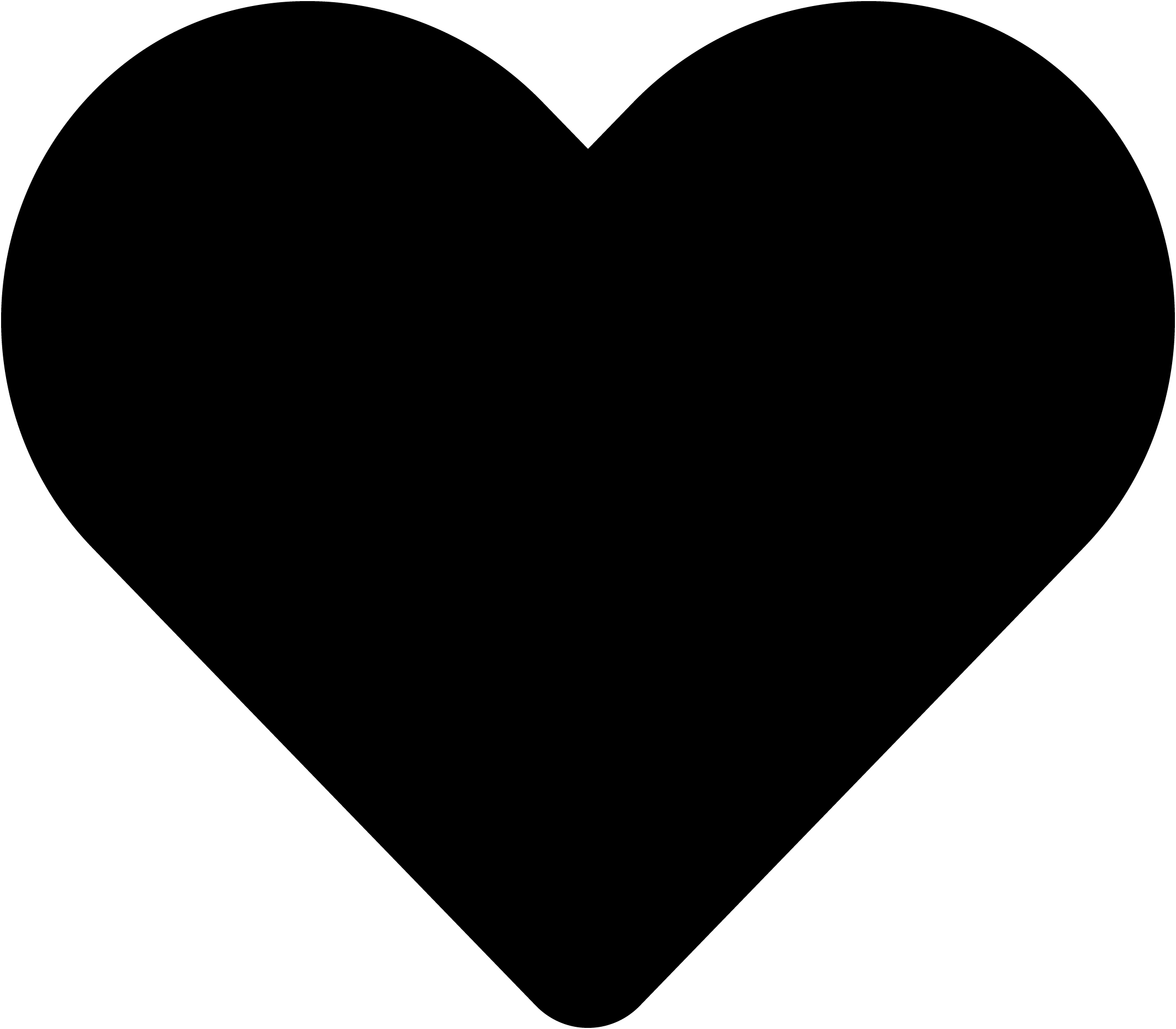
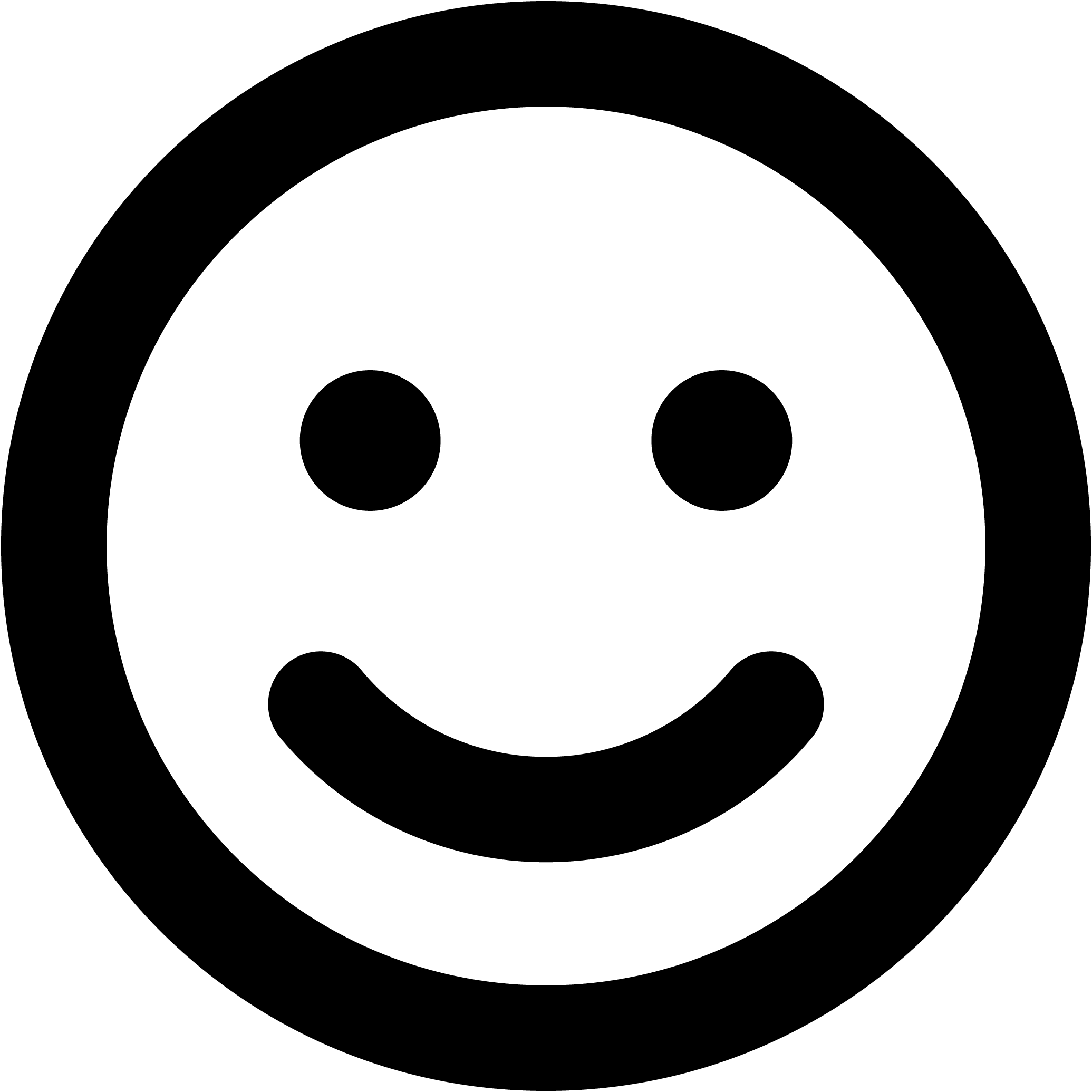
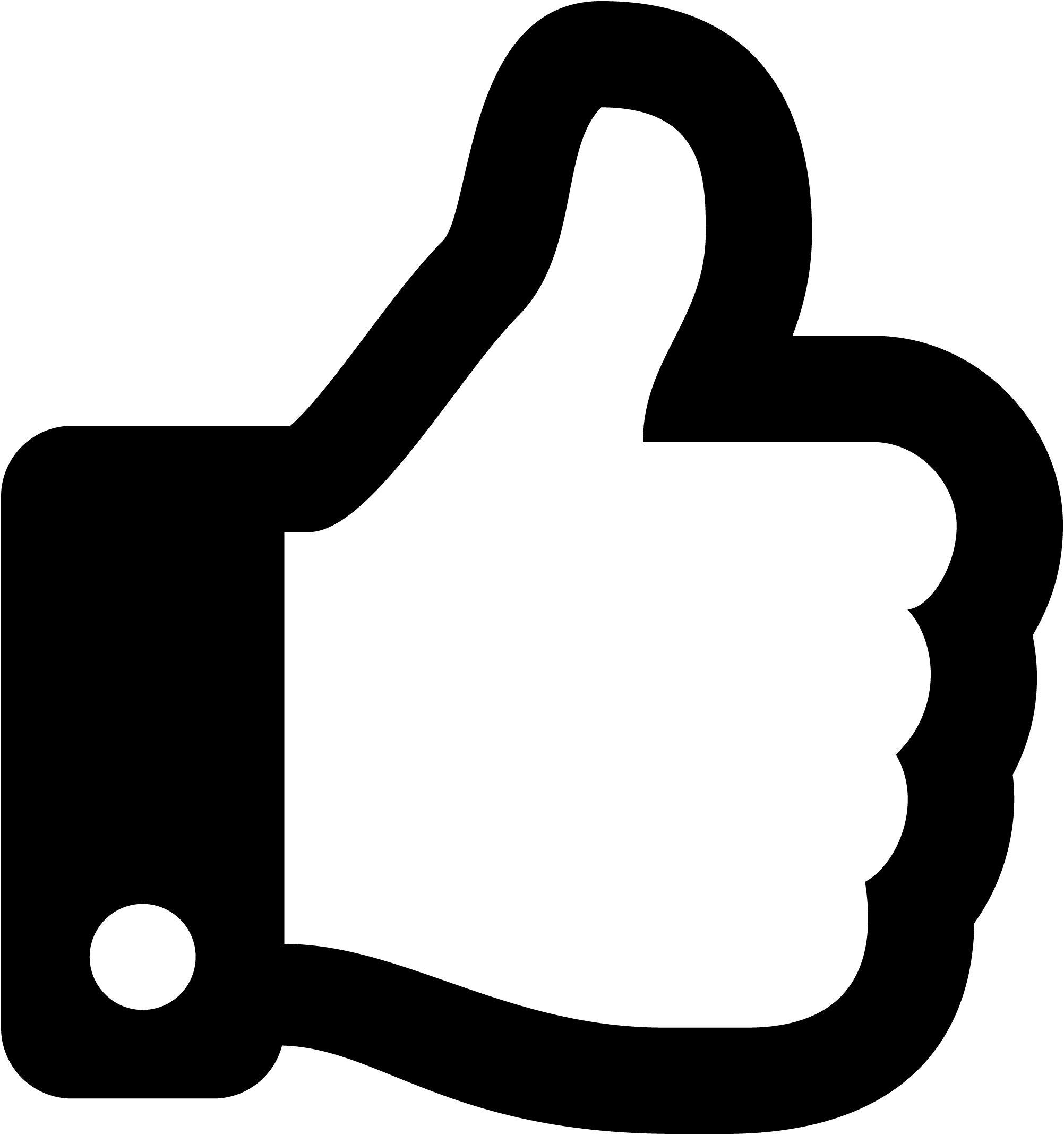
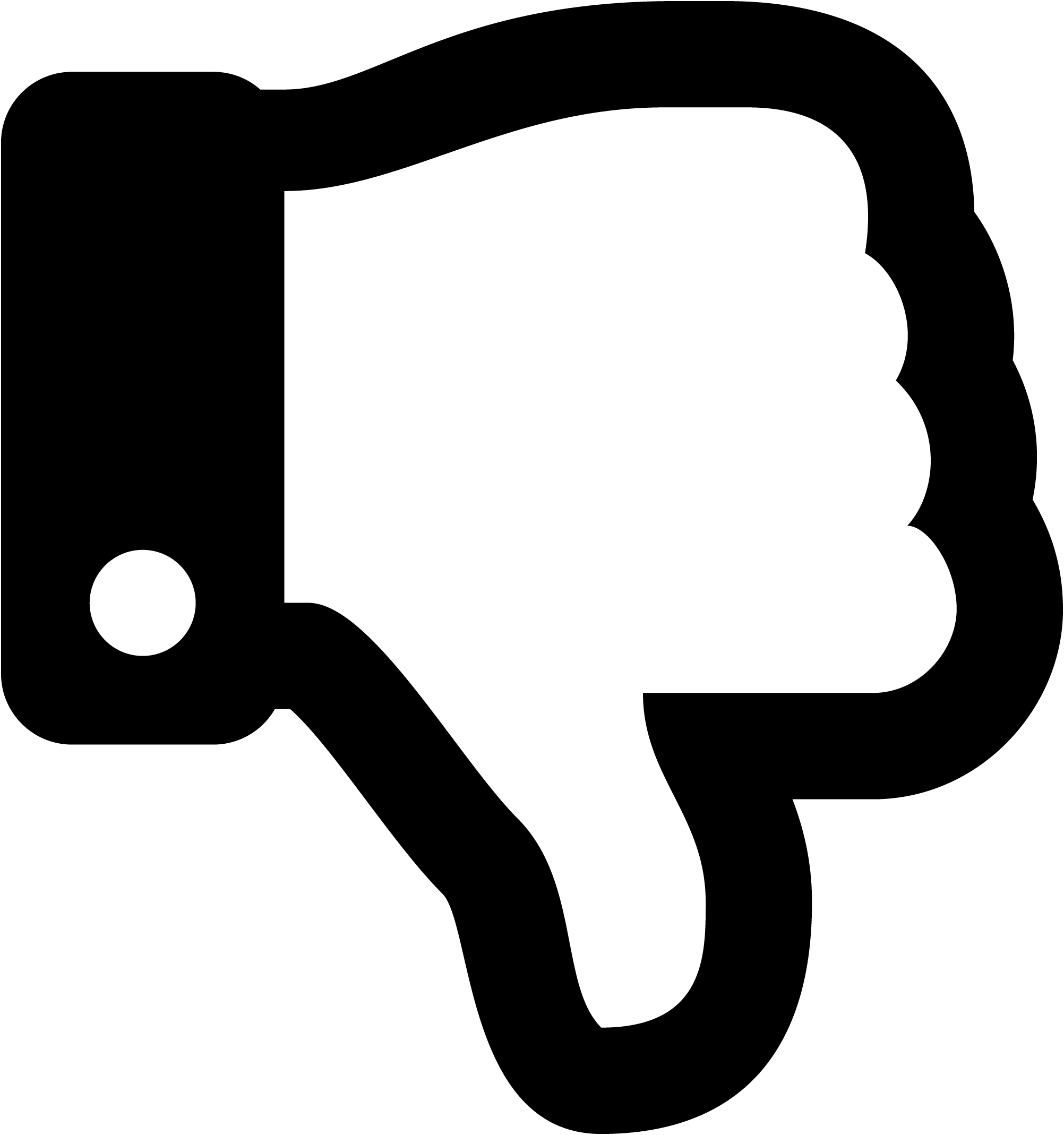
Image description/alt-tag
Image caption
Image link
Rollover Image (max size: 2mb)
Or drag a symbol into the upload area
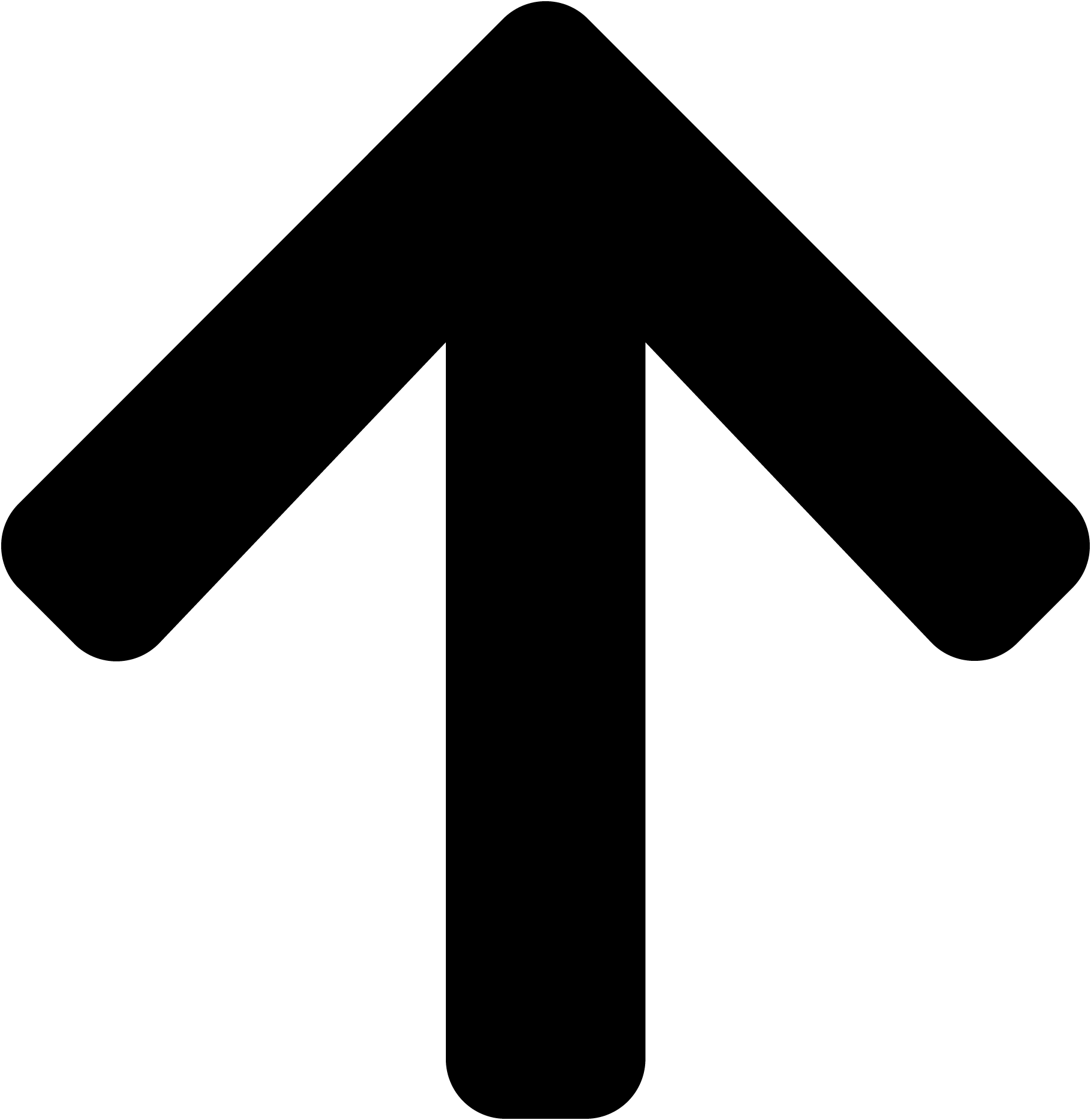
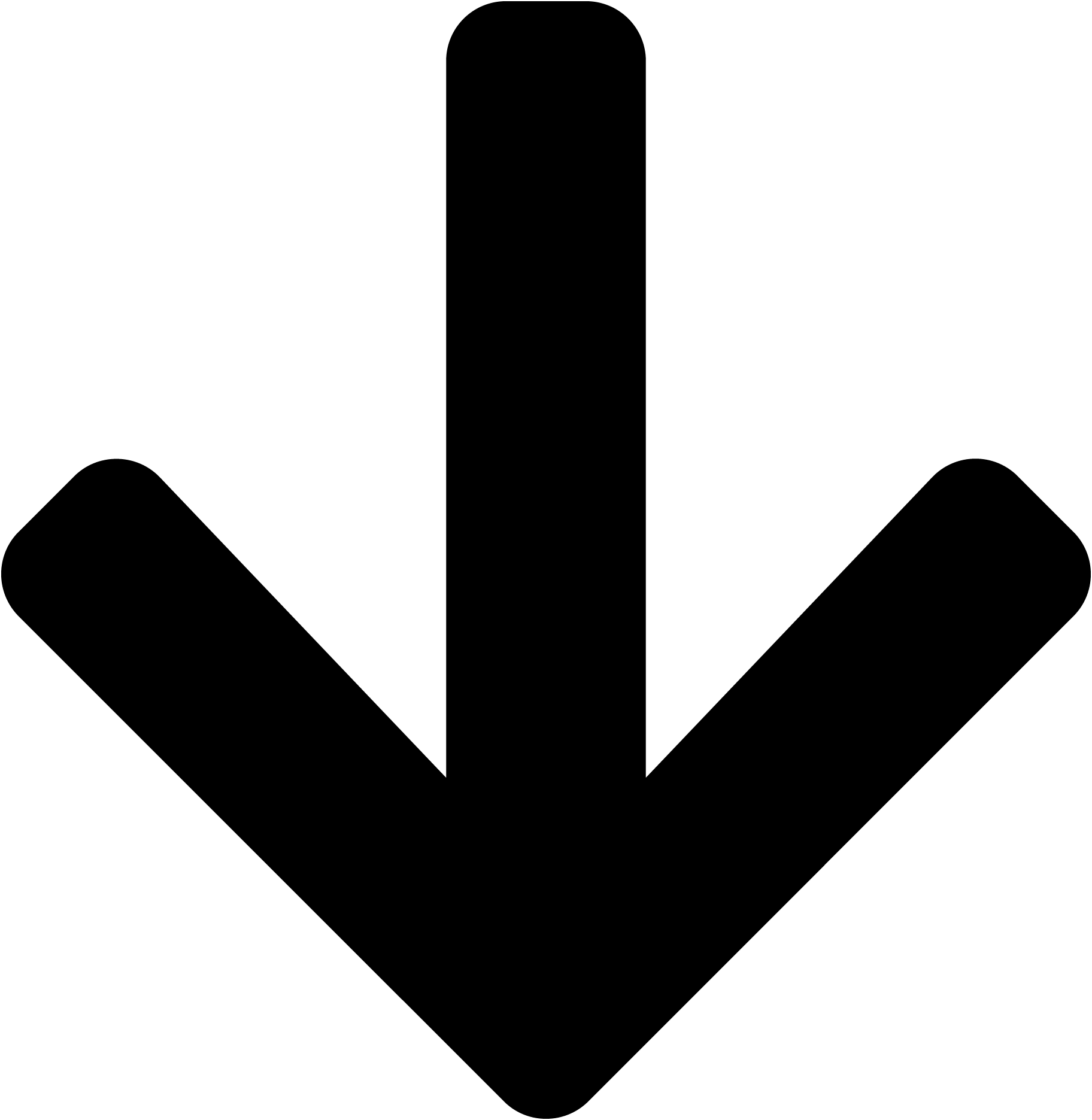
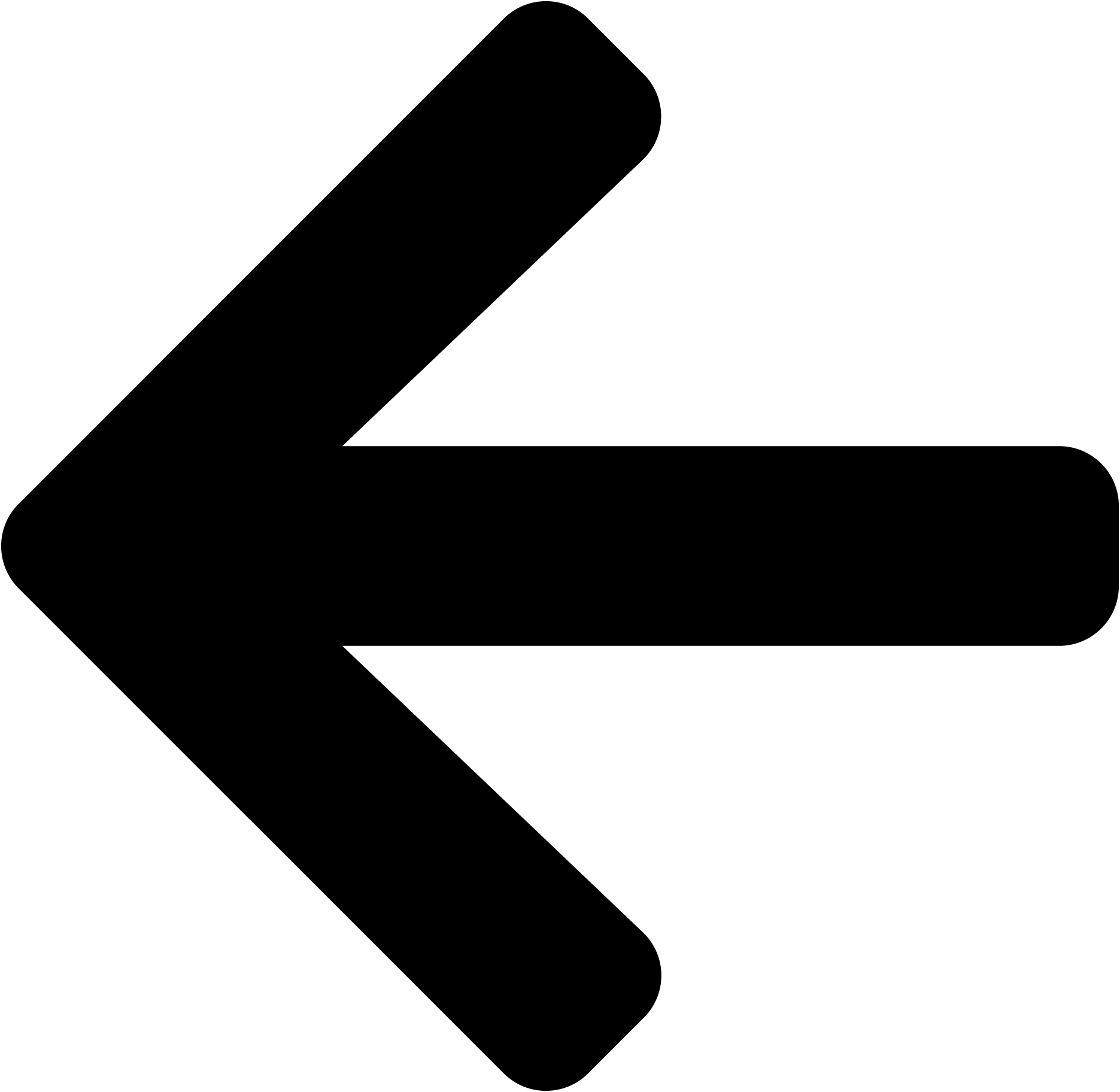
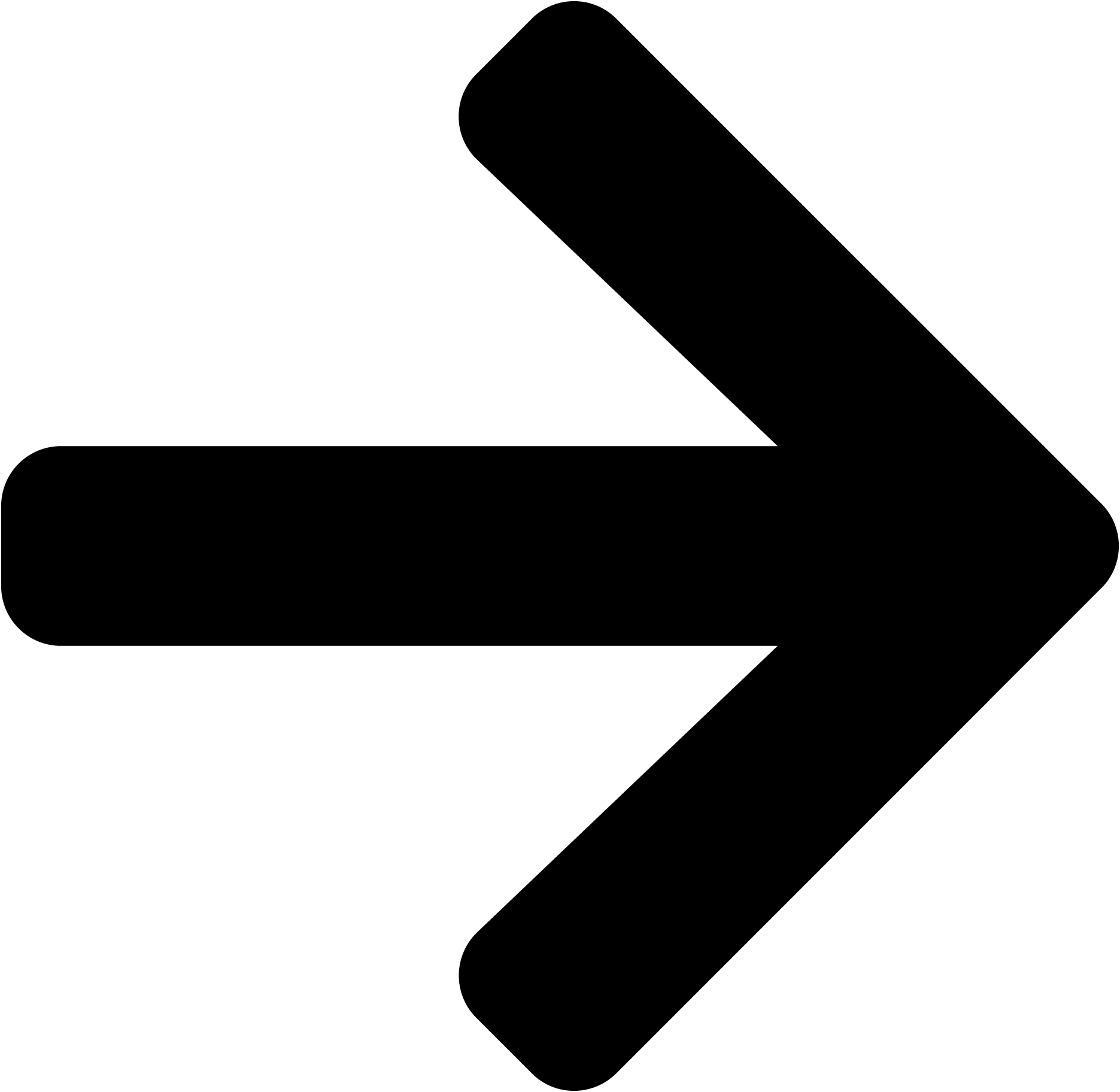
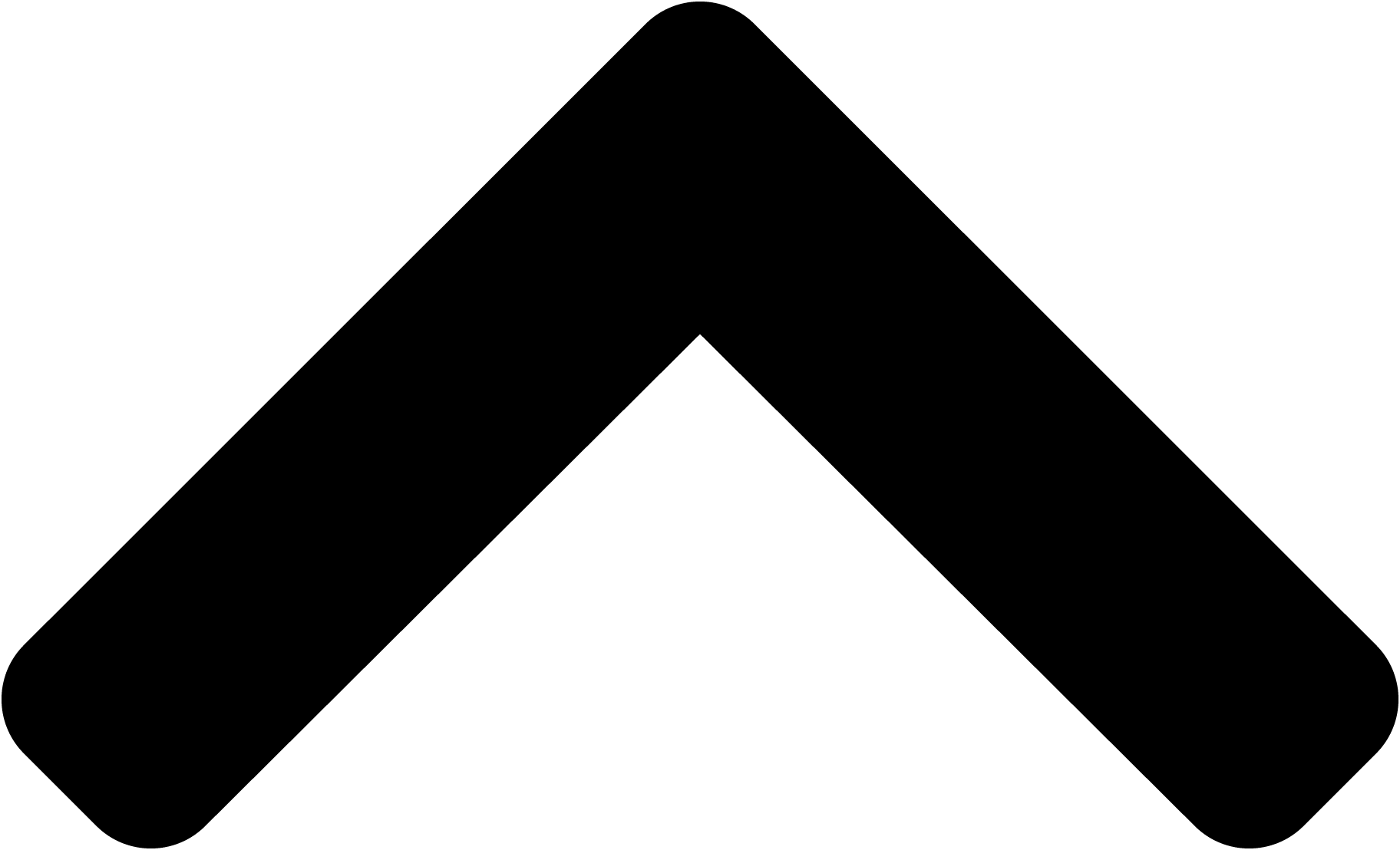
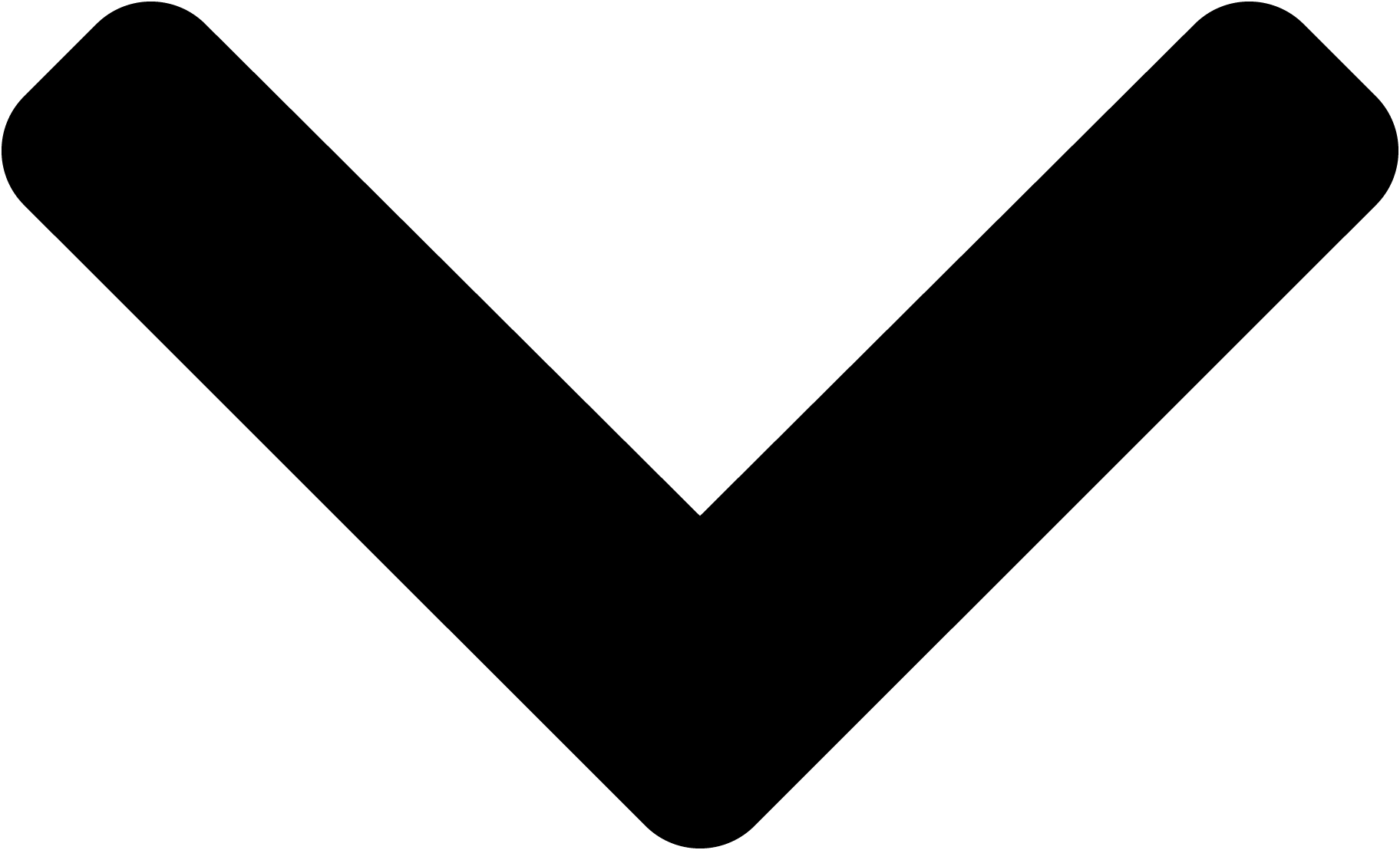
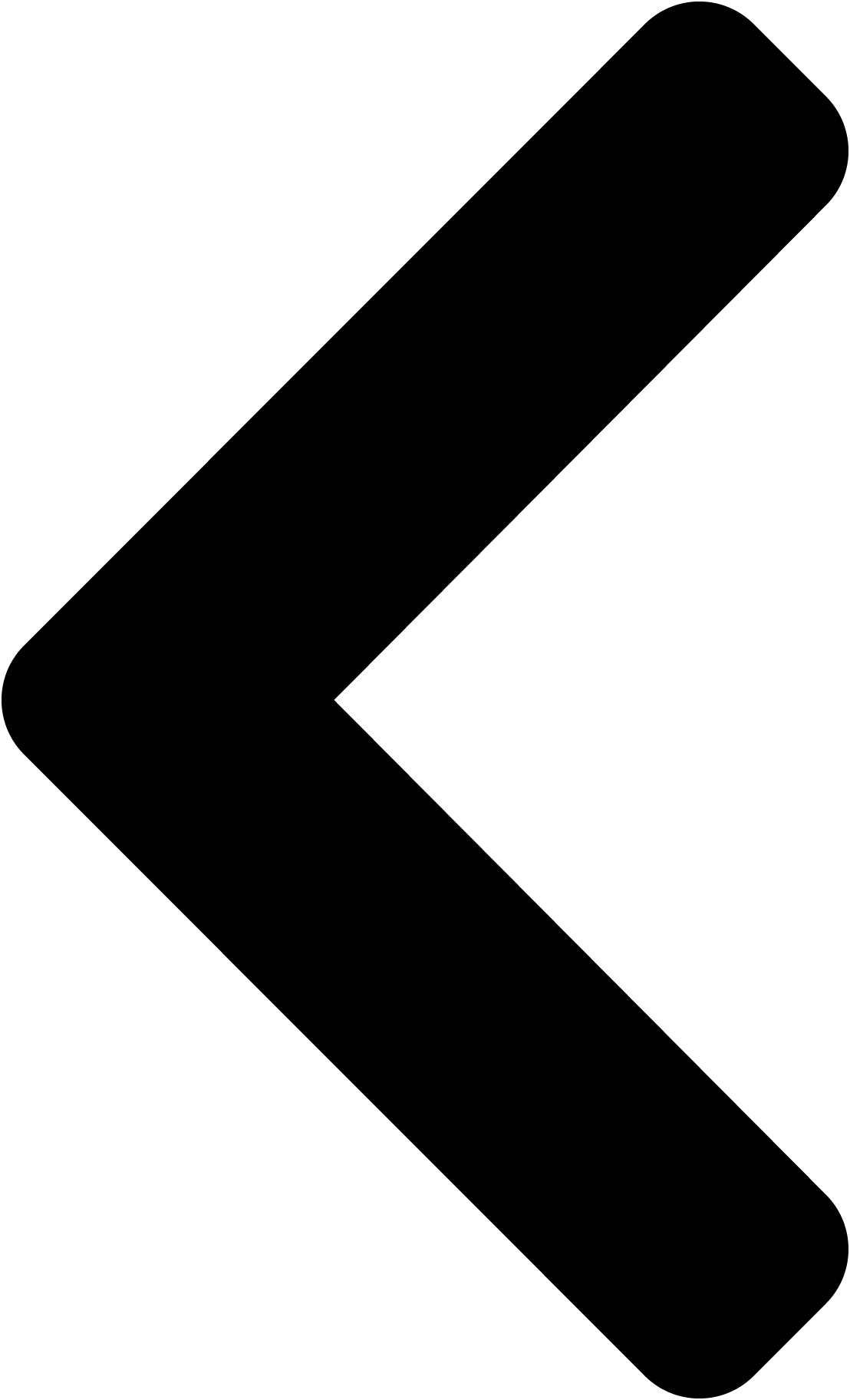
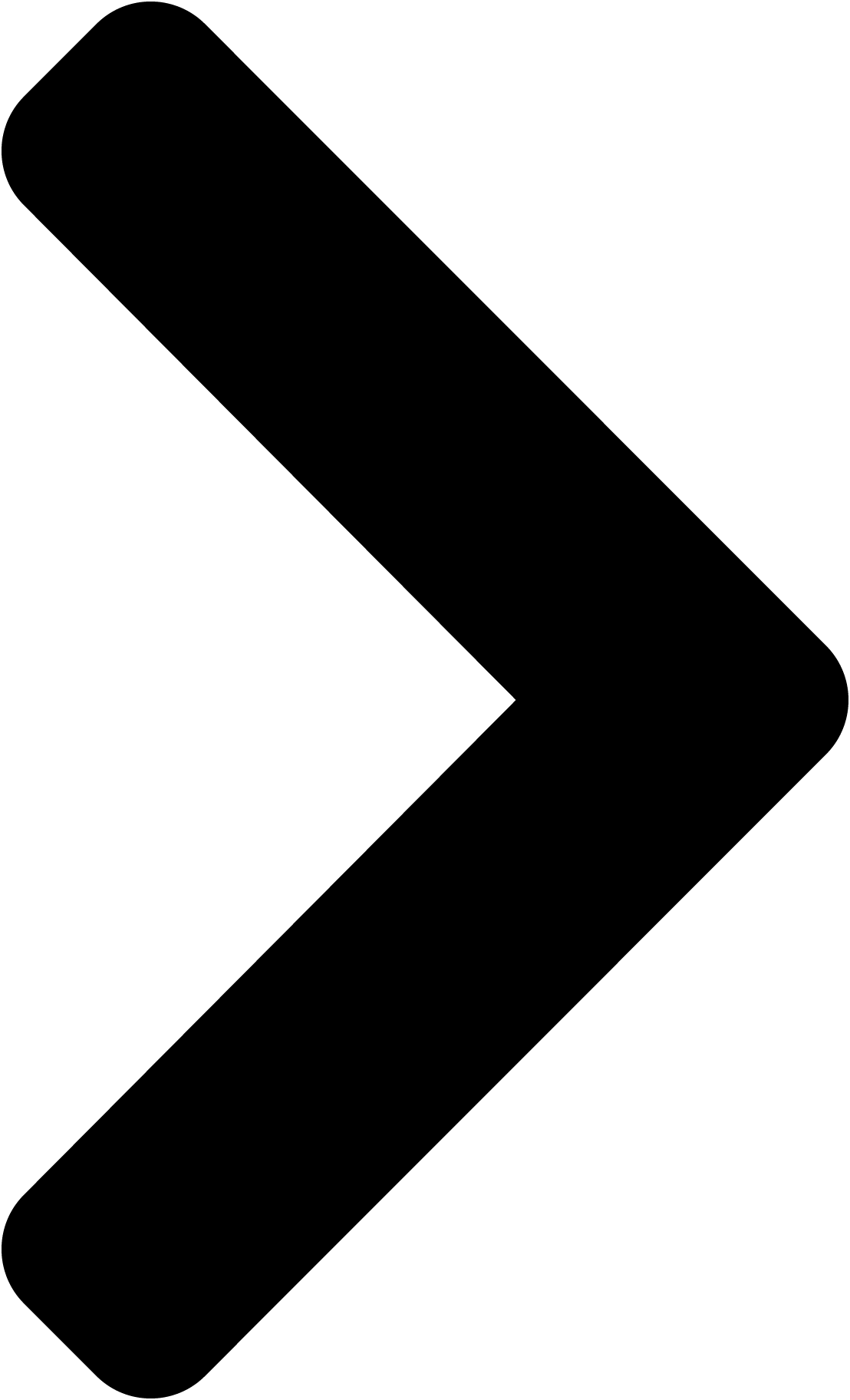
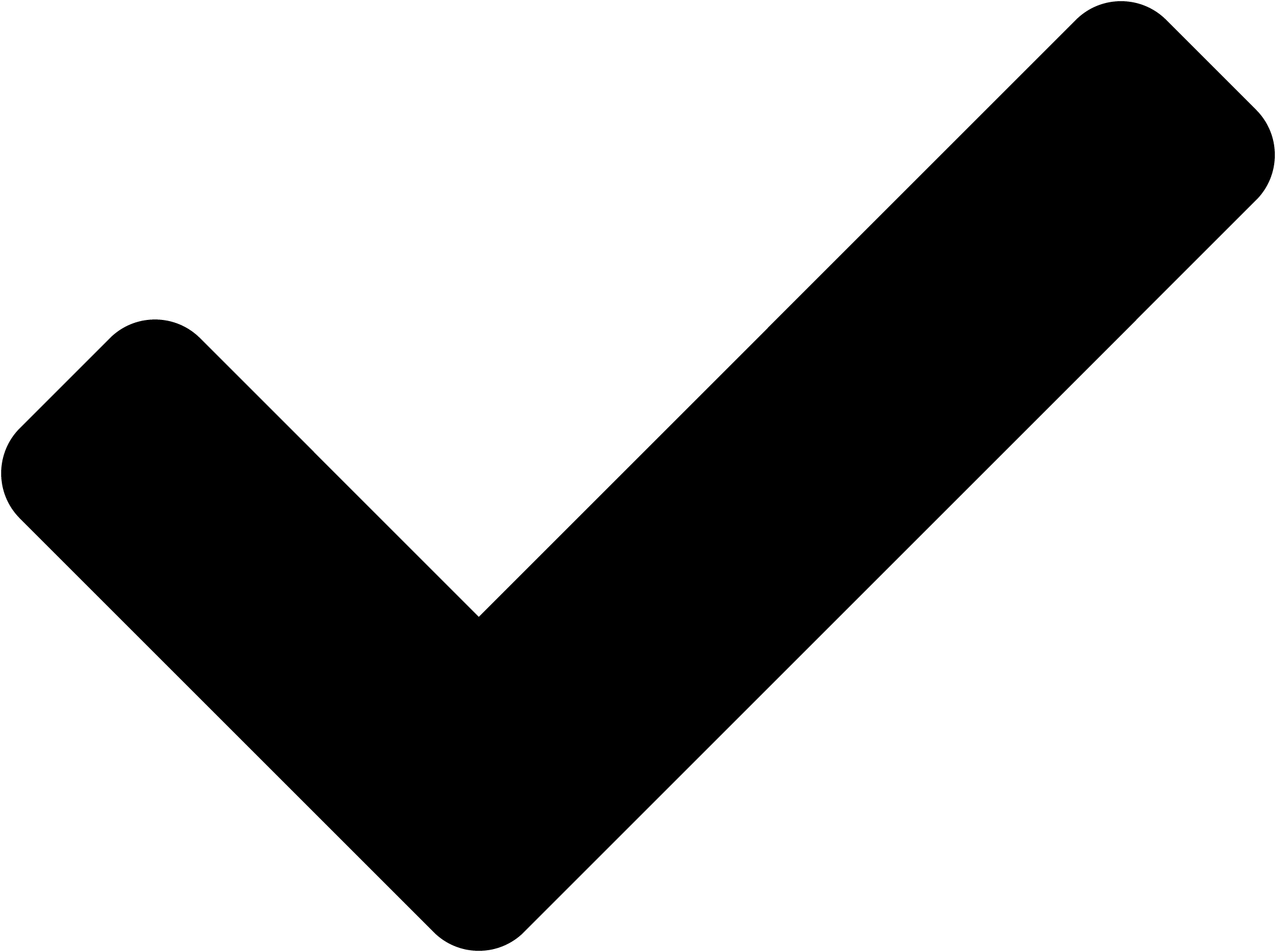
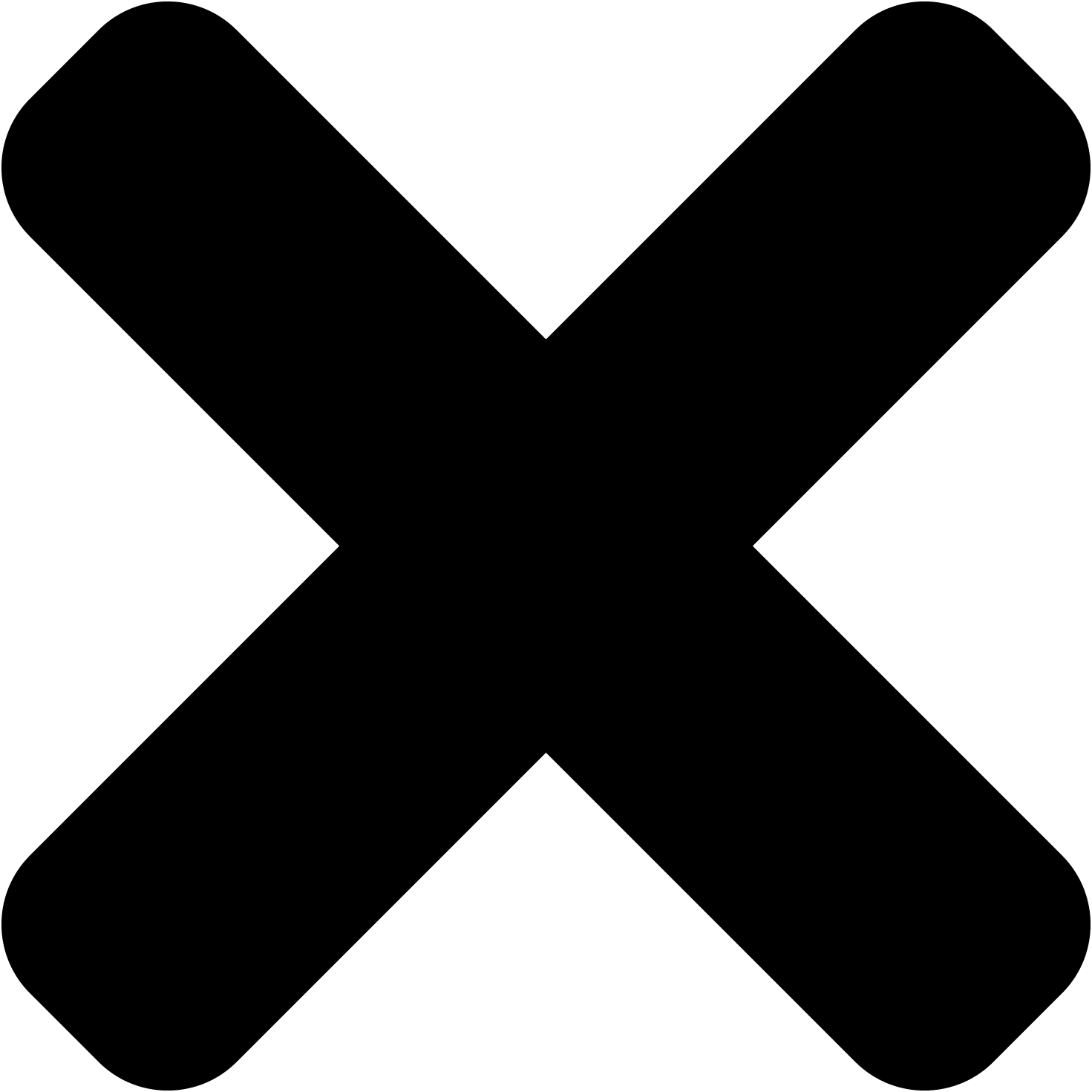
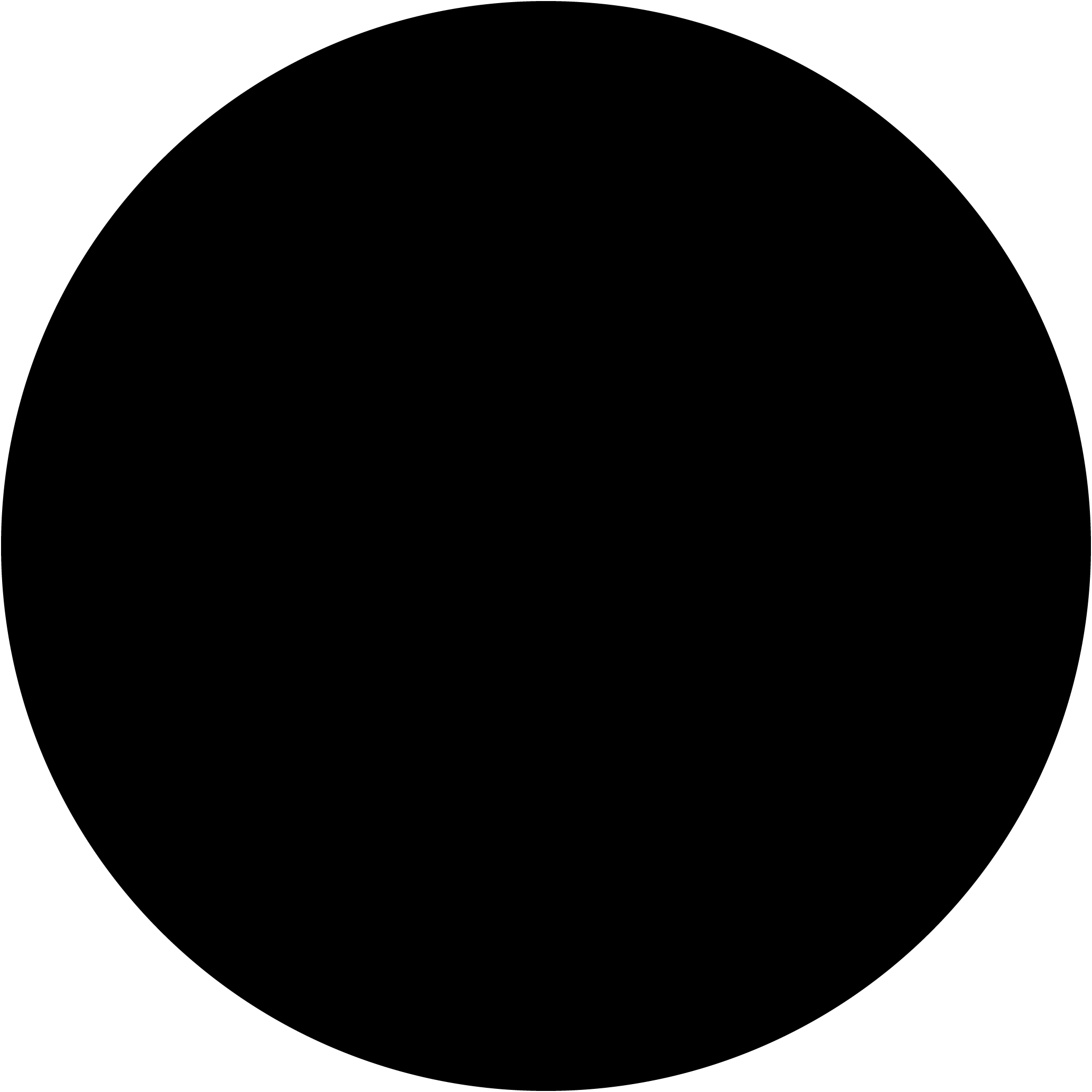
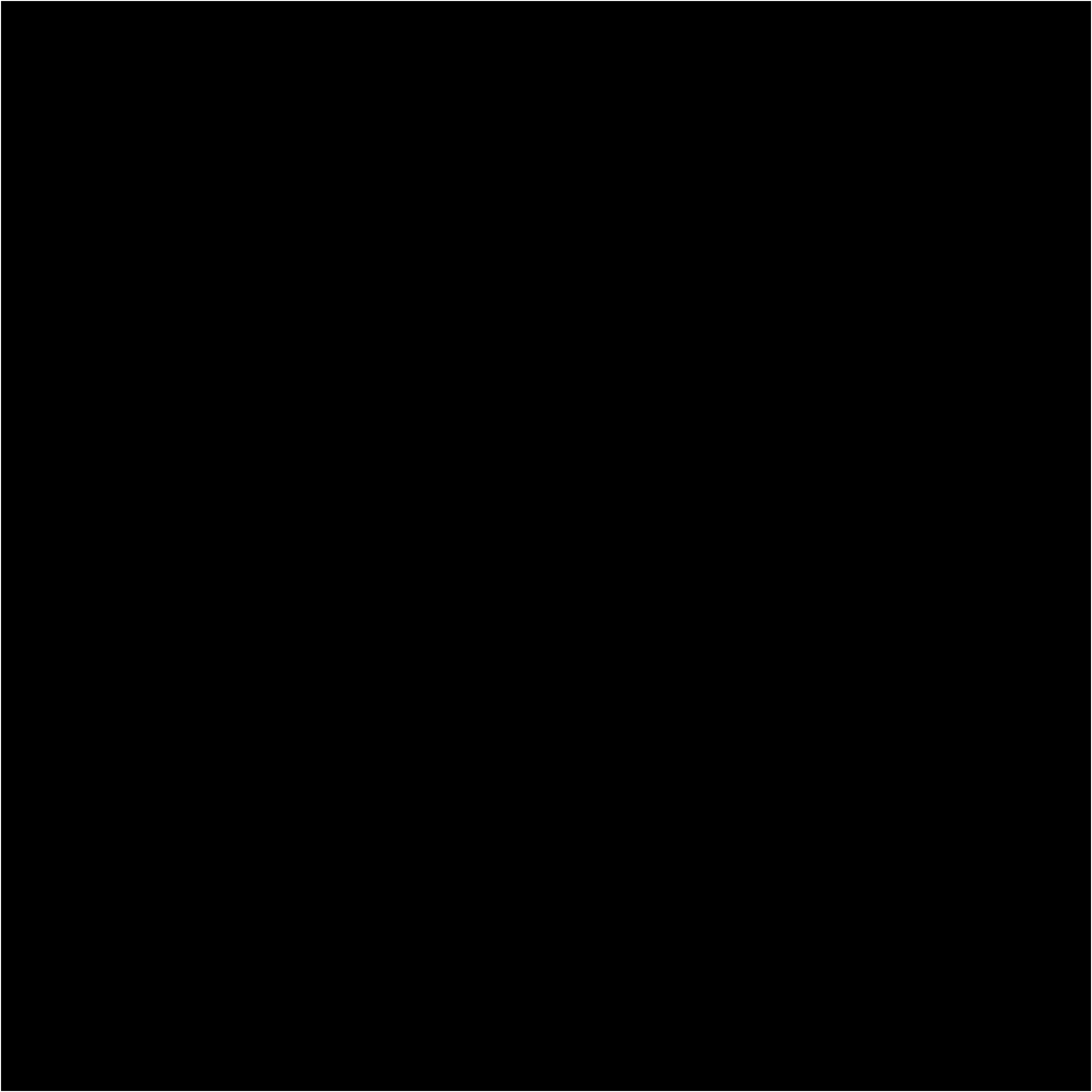
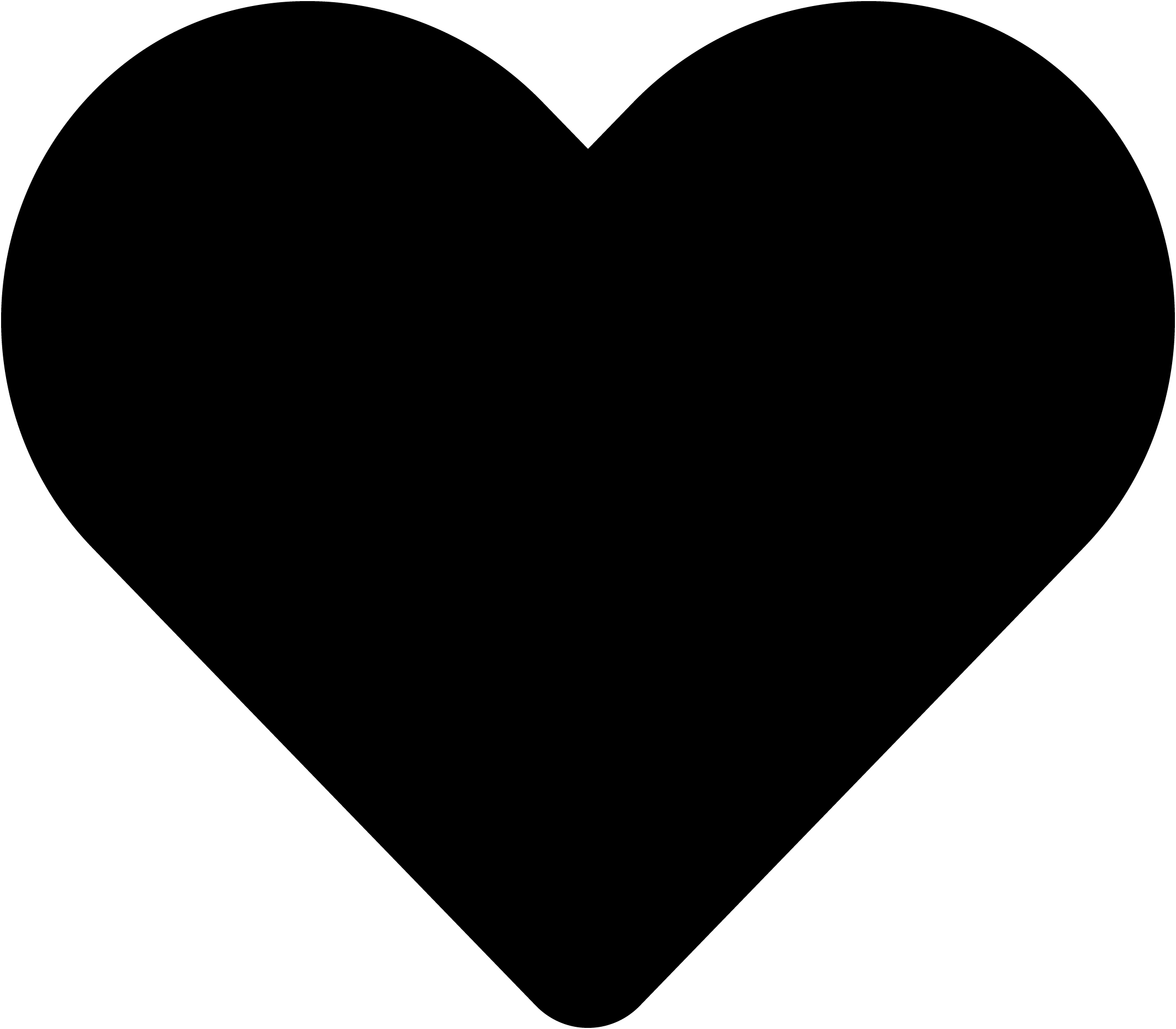
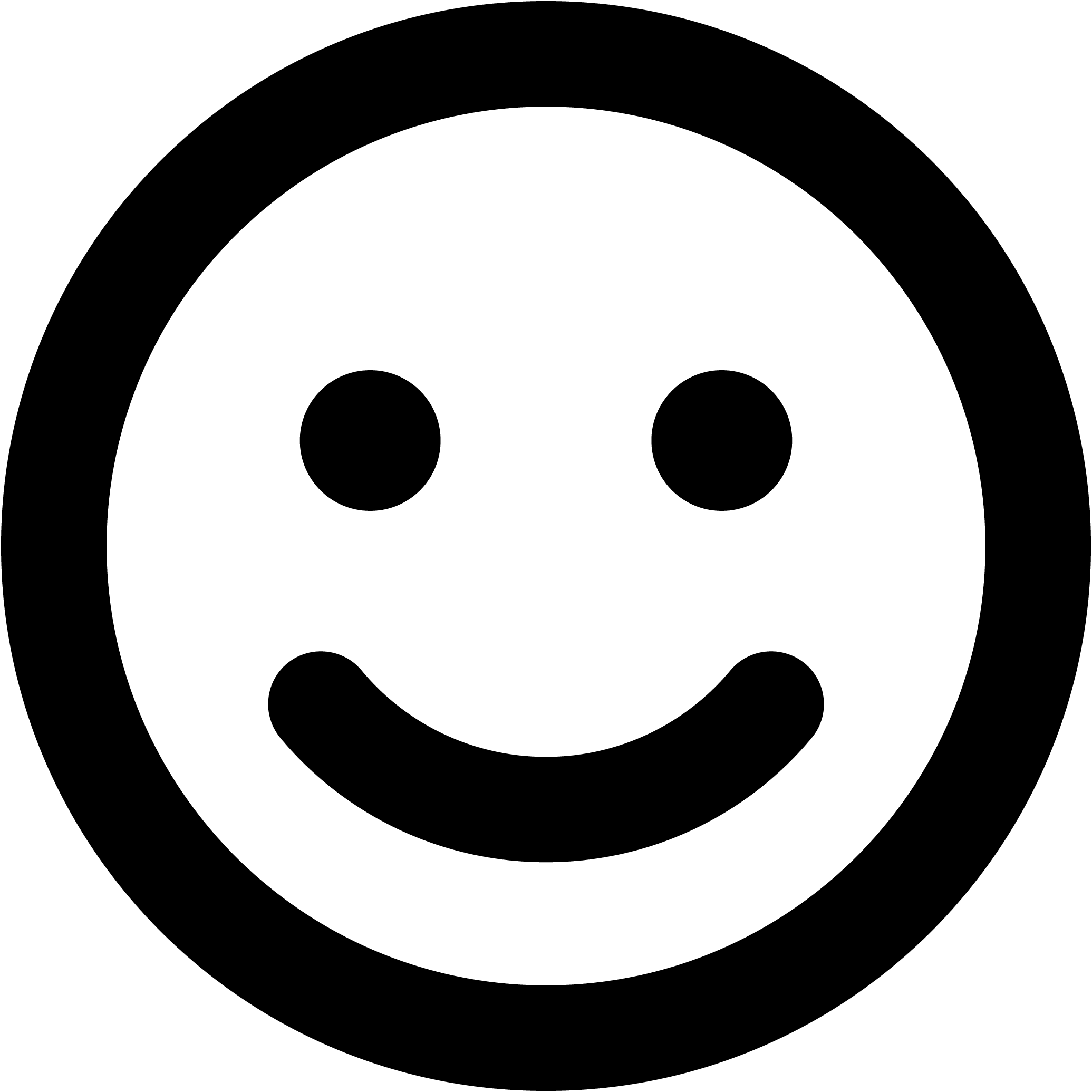
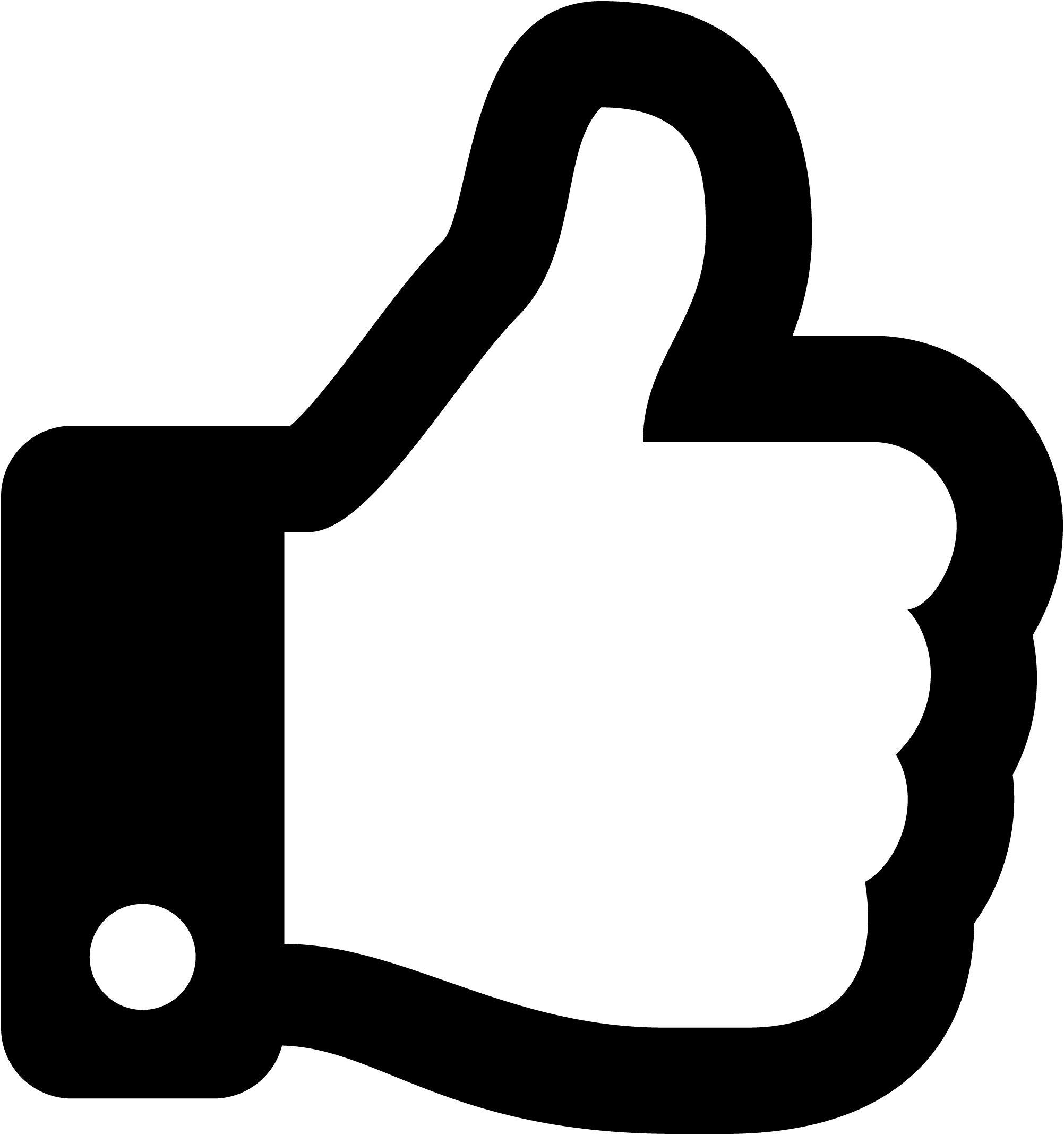
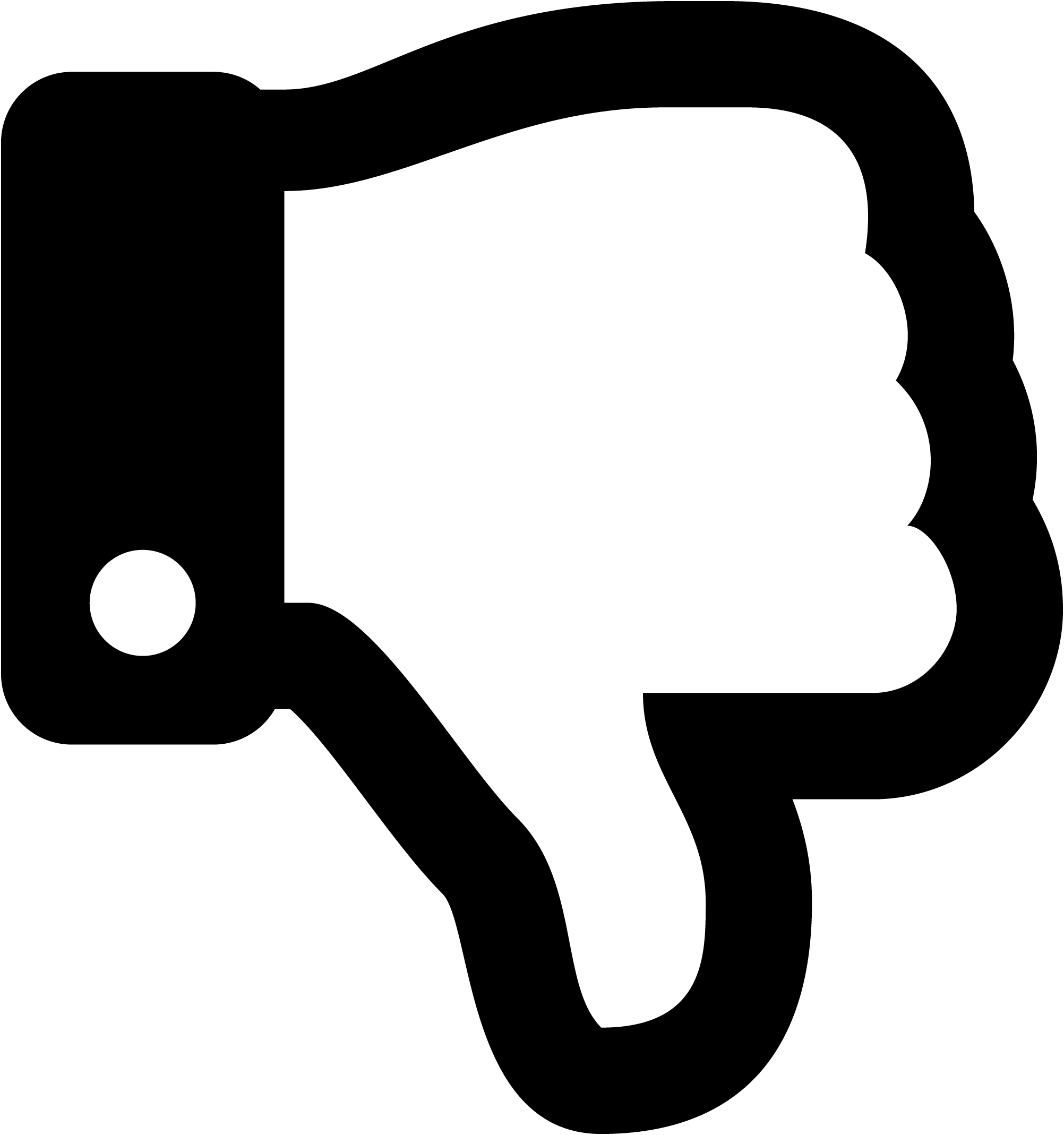
Border colour
Rotate
Skew (x-axis)
Skew (y-axis)