Tails, Droughts and Extremes
8th February 2016
The topic of this blog post follows from one of the talks we had last week on various research topics. The overall topic it came under
was Extreme Value Theory (EVT) and one section was Covariate Modelling in this context. The talk was given by
Emma Eastoe and after looking at the theory described how these ideas can be
applied to two environmental issues, namely ground level water and the Greenland ice sheet. Both of these are projects she is currently
engaged with.
The simplest case in which Extreme Value Theory comes in useful when we have a series of iid random variables $X_t$ which are assumed
to be from some unknown probability distribution $F$. Often Statistics is aimed at modelling the main part of $F$ and often the tails
may not the focus. But in some cases, such as storm modelling, it is the tails of the distribution that are most important in
describing rare events. This is the arena of EVT. One cannot hope to design flood defenses effectively for extreme conditions simply by
looking near the mean and mode of the distribution of data. Unfortunately, rare events mean that not much data actually exists, but
with some clever tricks, these can be modelled quite well.
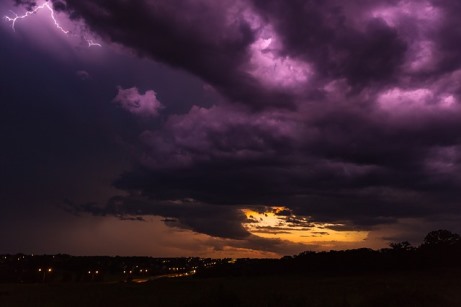
Thankfully, there is an analogous result to the Central Limit Theorem that applies to the maxima of a distribution. This is called the
Unified Extremal Types Theorem:
Let $M_n$ be the maximum of a set of random variables $\{X_1,...,X_n\}$, then there exist normalising constants $a_n>0$ and $b_n$ such that, as
$n\rightarrow\infty$
$$Pr\left[\frac{M_n-b_n}{a_n}\leq x\right]\rightarrow G(x)$$
then $G(x) = exp\{-[1+\xi x]_+^{-1/\xi}\}$.
$G$ is known as the generalised extreme value distribution. For large enough $n$, this allows the approximation for the distribution of the
maxima, and so is very important in EVT. It applies for any
given $F$. It can also be scaled and shifted by replacing $x$ with $(x-\mu)/\sigma$, for parameters $\mu$ and $\sigma$. From this, the
conditional distribution of $X>x|X>u$ can be shown to have the asymptotic distribution of
$$Pr\left[\frac{X-b_n}{a_n}\leq x|\frac{X-b_n}{a_n}\leq u\right]\approx [1+\xi \frac{x-u}{\sigma}]_+^{-1/\xi}.$$
This is called the Generalised Pareto distribution and is also very useful. Its parameters are scale
What Emma Eastoe considered was the case when $X_t$ is either not independent, or each $X_t$ comes from a different distribution $F_t$.
In this situation, there are two different methods, Linear Regression Models and random Effects Models, both of which can be applied to
the Generalised Pareto and Generalised Extreme Value distributions.
The example that was used presented was Groundwater level. This gives an indication of the overall amount of water in an aquifer.
Aquifers are useful in times of little rainfall as the water can be extracted from them and then supplied to residences. The problem is
that monthly data of groundwater levles is fairly correlated with the data from that month in previous years and the months before it.
This means that simply applying a Generalised Pareto or Generalised Extreme Value will not effectively model the situation. To deal
with this, Eastoe has used linear regression models and included rainfall, potential evaporation and a year to year trend as covariates
for the parameter $\mu$ of the Generalised Extreme Value distribution. The results were interesting in that the minima and maxima showed
different significant covariates. For minima, the year and potential evaporation proved to be very important, whereas for the maxima,
it was the rainfall that showed up as significant. The next thing to look at is whether or not this is because the information is reacted
to in slightly differently ways.
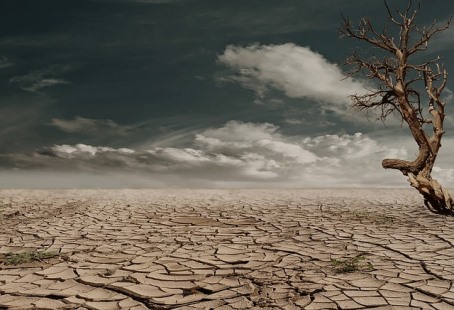
After this Eastoe briefly discussed some work on the greenland ice sheet she is about to begin. She has yet to start modelling, but her early
analysis of the data suggests that observational data and numerical models from scientists differ a lot in the tails. This idea is an example of
something Professor Jon Tawn (director of STOR-i) said to us at the beginning of the year,
that serious study of the data is very important before leaping
into modelling.
The
other parts of the talks discussed what one should do when the data is correlated and methods to tackle this, as well as some example applications
such as flood risk modelling. The flood risk management required real thought about how to model the data. In particular, although two points may be
close together in a spatial sense, this does not mean that their flood risks and water levels will be similar, possibly due to being near a joining
of two rivers. A more appropriate measure of closeness must therefore be considered in order to take this into account. The idea settled upon was to
compare the centres of the areas from which that point recieves water. I found the whole talk very intersting, and EVT seems very interesting
mathematically as well as having some very important applications.