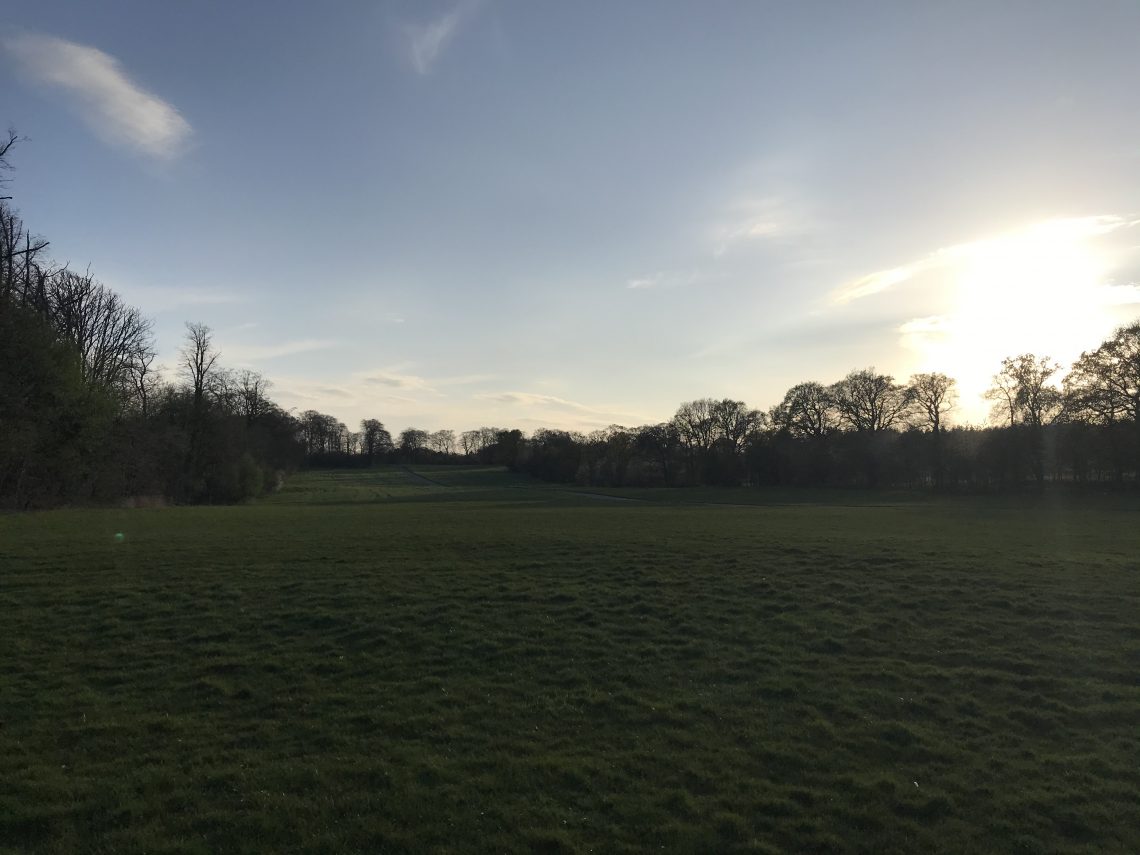
Missing Data: Introducing the Missingness Mechanism
Often when we collect data, some is missing. What do we do? Well, there is a load of stuff to cover here (and I’m going to do it over a few posts). This post is going to cover an important question: what is causing the data to be missing?
What causes the data to be missing is known as the Missingness Mechanism. There are three main types: Missing Completely at Random (MCAR), Missing at Random (MAR), and Missing Not at Random (MNAR).
You can think of these as traffic lights: MCAR is green (easy to deal with), MAR is amber (a bit problematic but there are some decent methods out there) and MNAR is red (a total pig to deal with).
Missing Completely at Random
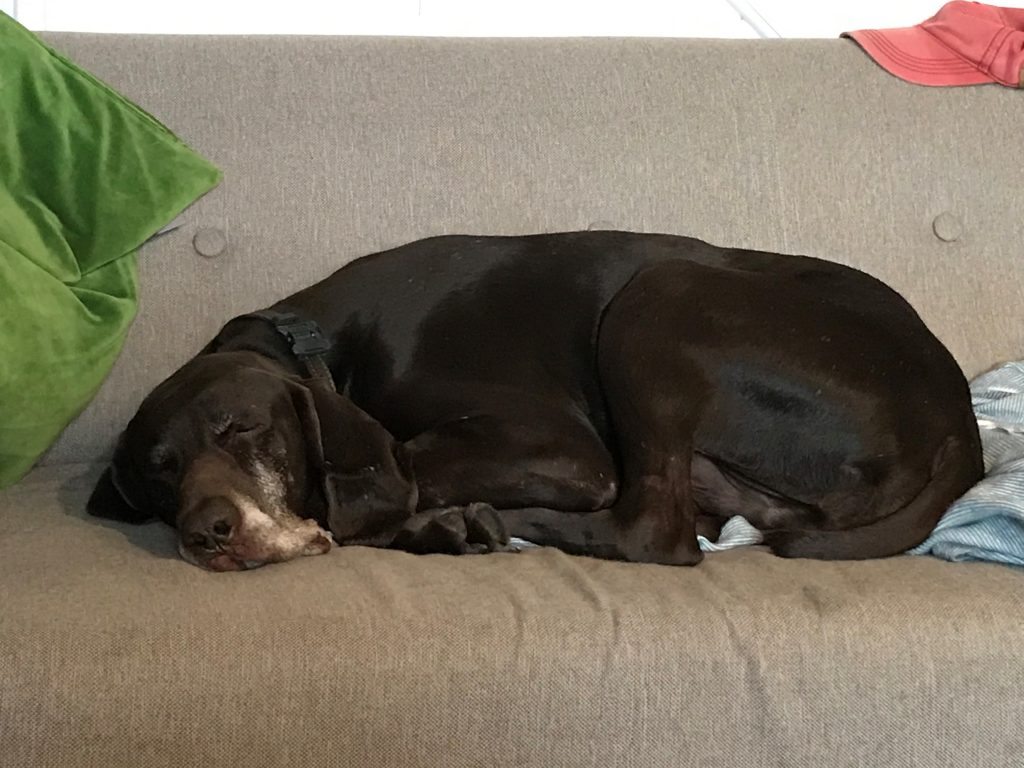
As the name suggests, Missing Completely at Random data means that the missingness presenting in your data is in a totally random pattern. There isn’t anything in the data driving it that you need to be further concerned with. This is nice, because you can get away with some simplistic methods to deal with it.
I describe some of those methods in my next post on missing data.
Missing at Random
Missing at Random data is where what drives the missingness is something in the data we are collecting, but that what drives it is something we have observed. The preceding sentence starts to give me a headache if I think about it too much, so I prefer to think of it in terms of an example.
Imagine a university does a survey of previous students, to find out where they are working, what their income bracket is, etc.
Let’s say that alumni that work in a particular sector are less likely to disclose their income. But, they do disclose what sector it is that they work in. That data would be Missing at Random.
Missing Not at Random
But, what if students are less likely to respond to that income question the more they earn? Then we have Missing Not at Random data. The missingness depends on something we do not observe.
This is very difficult to deal with and often causes bias in our analysis. To make it even more difficult, we cannot test whether the missingness mechanism is Missing at Random or Missing Not at Random.
Want to know more?
You can read more about missingness mechanisms in Chapter 1 of the book below — this is a really good book on missing data in general.
Little, R. J. A. and Rubin, D. B. (2020). Statistical analysis with missing data. Wiley Series in Probability and Statistics. Wiley, Hoboken, NJ, third edition.
I’ve also included a link to the paper that introduced the idea of considering missingness mechanisms.
Rubin, D. B. (1976). Inference and missing data. Biometrika, 63(3):581-592.
Thank you for reading. Click here to see my next post in this series. This will discuss some simple methods to deal with missing data.
Or you can skip to my final post on missing data: this will discuss a method that allows you to quantify the uncertainty that you are introducing into your analysis by using some of the methods discussed in my second post.
I wrote a 20 page report on Missing Data as part of my studies at STOR-i. It discusses the ideas above in more depth. If you want to take a look, click here.

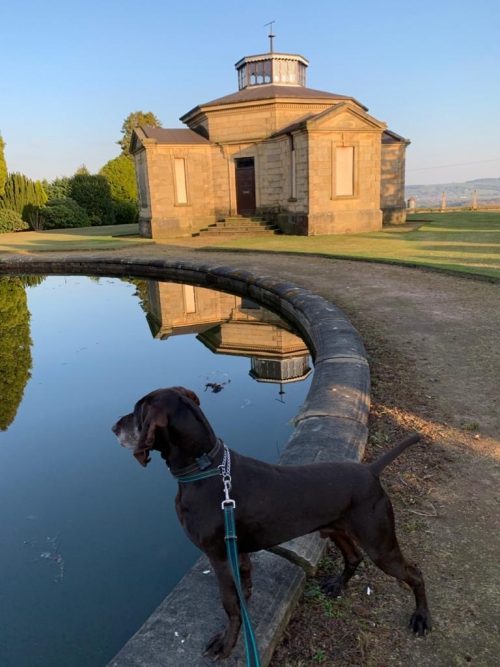
2 Comments
Pingback:
Pingback: