As promised in my previous blog post I will be talking today about my first experience of an academic conference.
This year STOR-i hosted its ninth annual conference, with talks from a wide variety of speakers from the UK — including some of its own PhD students and alumni — and from overseas. We listened to 12 presentations, so for the sake of brevity I will mention all of them but only go into more detail for a few.
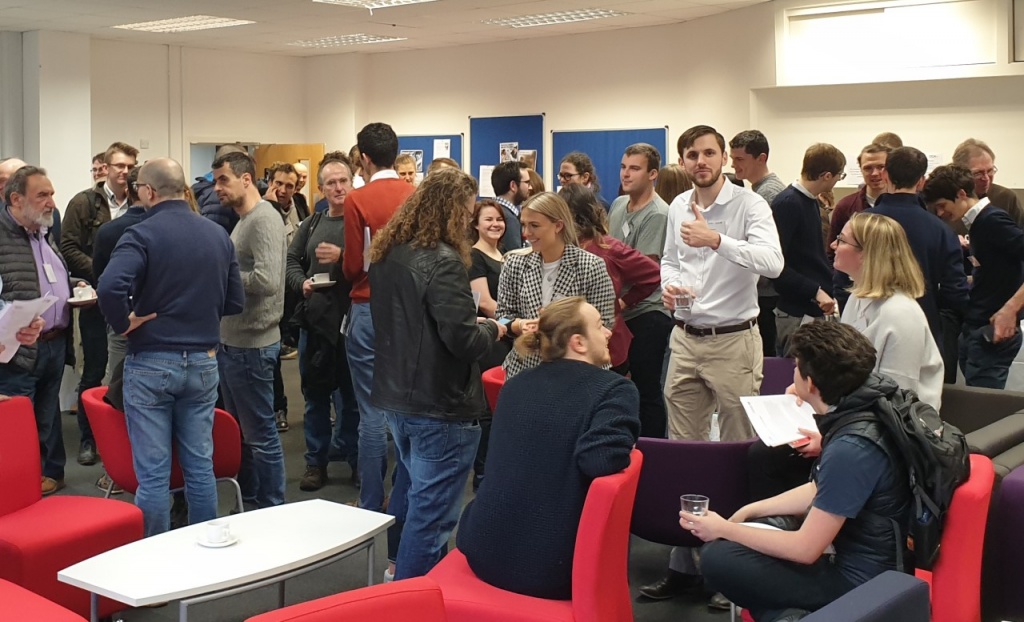
We were welcomed to the conference by Prof. Kevin Glazebrook who spoke a bit about STOR-i’s new round of funding and introduced the first speaker: Alex Jacquillat from the MIT Sloan School of Management.
Jacquillat spoke about analytics in air transportation. In particular he discussed how we can use air traffic flow management to absorb delays upstream by holding planes on the runway so they don’t get held up in the air, expending extra fuel as they wait for their turn to land. He also spoke about the benefits of adjusting existing integer programs for scheduling so that they are optimised to minimise passenger delays. This would give greater priority to larger flights and ensuring connecting flights arrive on time.
The second talk was by third year STOR-i PhD student, Henry Moss, who introduced us to a Bayesian optimisation method called MUMBO, which he has been developing for his thesis.
Next up was Valeria Vitelli from the University of Oslo who talked about her work with the Mallows ranking model as well as some applications and recent advances in the area.
After lunch we were given two more talks. The first by Miguel Anjos, from the University of Edinburgh, and the other by Georgia Souli, a third year STOR-i PhD student, before another break for refreshments.
We came back to a presentation titled “The Use of Shape Constraints for Modelling Time Series of Counts” by Richard Davis from Columbia University.
Tom Flowerdew, a STOR-i alumni then talked to us about his work at Featurespace using machine learning to detect fraud. One of the major problems here is that machine leaning requires data to help it learn but as the nature of fraud changes the algorithm must be able to adapt. The difficulty here is that since all the obvious fraud attempts are blocked future iterations will have no experience of them and so will have difficulties detecting them. Flowerdew suggested that allowing suspected fraudulent transactions be completed with some small probability and then proportionally increasing the weight of these outcomes in the learning stage would allow the algorithm to learn more effectively and therefore prevent more fraud in the long run.
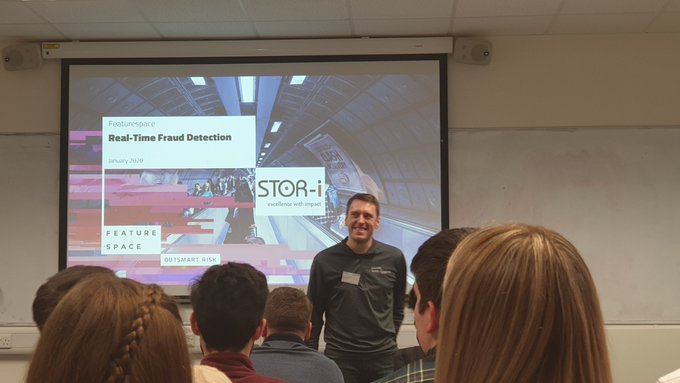
The final presentation of the day was: “Making random things better: Optimisation of Stochastic Systems” by Christine Currie from the University of Southampton.
We reconvened in the evening to look at posters made by the PhD students about each of their projects. This was a really good opportunity for them to develop their presentation skills by explaining their findings to knowledgeable academics in closely related fields. It was also an opportunity for us MRes students to learn a bit more about the research going on at the university and the sort of projects we might be interested in.
The following day we kicked off with a presentation by Veronica Vinciotti from Brunel University. This focused on using the network of transactions between small and mediums sized businesses to improve credit risk models. Since transaction network data is difficult to get hold of, she also spoke about what approaches one can use without access to this data.
Next up was Dolores Romero Morales from Copenhagen Business School who spoke about the balance between accuracy and interpretability for data science models and how this can be achieved.
Another STOR-i alumni, Ciara Pike-Burke, then talked about her recent work with multi-armed bandits. A multi-armed bandit can be thought of as a slot machine where pulling each arm will give a reward from some unknown distribution. The usual problem is balancing exploration to learn more about the different reward distributions for each arm while also trying to maximise the total rewards by exploiting the arm that is performing best. The reward distributions are usually constant but Pike-Burke considered the case where the rewards are dependent on the previous actions of the player. For example a company can suggest different products to a customer on their website and the reward is dependent upon whether the customer follows that link. If the customer has just bought a bed they are probably less likely to buy another bed. However, that same customer might be more likely to buy new pillowcases.
Finally Brendan Murphy from University College Dublin presented his talk on “Model Based Clustering with Sparse Covariance Matrices.”