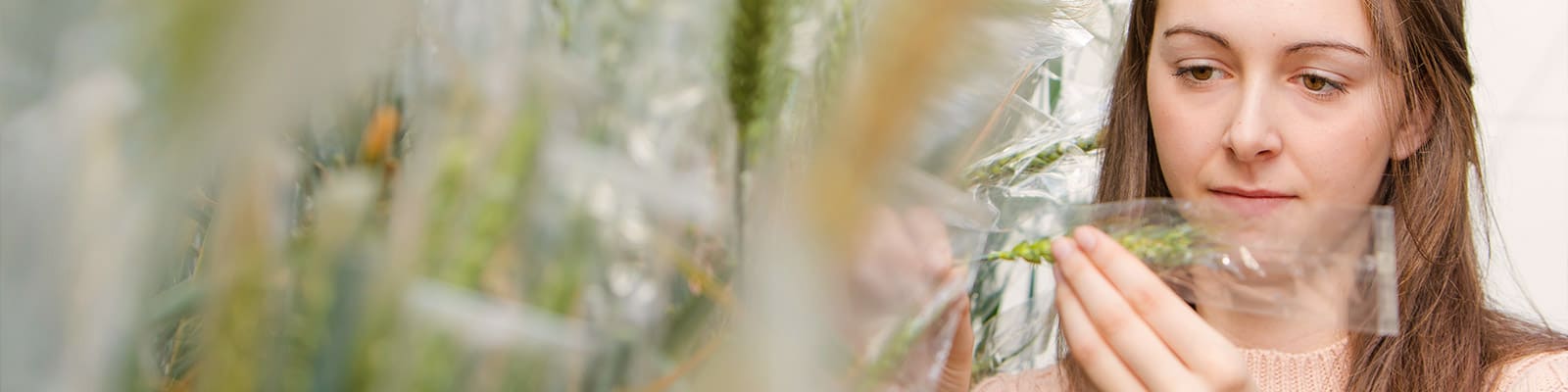
Motivating Challenges
Ice Sheet Melt Prediction
Accurate predictions of ice sheet change are crucial in planning global sea level adaptation and mitigation measures. The current state of the art is based on science-driven process models, which are calibrated against observations by summary statistics (e.g. mean temperature).
Preliminary work shows this approach to be unsatisfactory, largely due to spatiotemporal variability in the polar climate, resulting in both imperfect understandings of physical drivers of ice sheet change and uncertain predictions. Novel data science approaches will be deployed to provide more robust predictions of ice sheet change, with well-calibrated uncertainty estimates, and user-centric decision support, providing a beacon of good practice for the ice sheet modelling community.
We will deploy methods to construct spatiotemporal extreme value statistical models to enable regional climate model predictions to capture these events. Calibrating a climate model in a statistically principled manner requires multiple computationally intensive runs of a model. We will use Bayesian optimisation to choose parameter values/configuration options to efficiently optimise the likelihood, using the community firn model as an exemplar.
Polar data is typically sparse and/or heterogeneous in both space and time. We will use techniques to better model the uncertainty around predictions of ice sheet change in this context. Combining these approaches, and using active learning theory, we will inform strategies for further data capture and ice sheet process model development. The virtual labs frameworks will be used to produce an ice sheet melt scenario explorer.
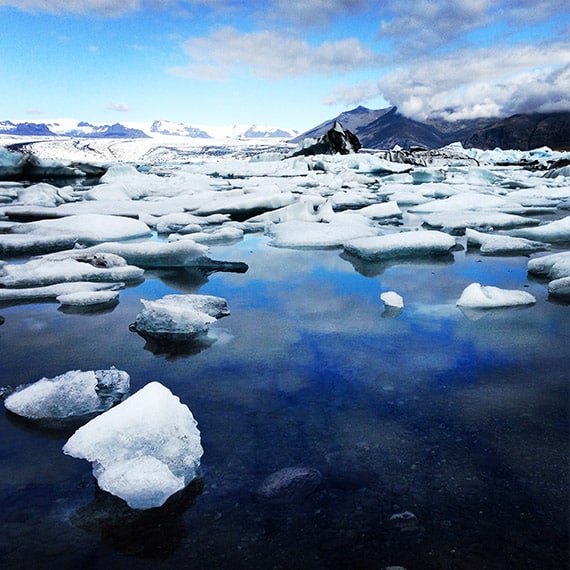
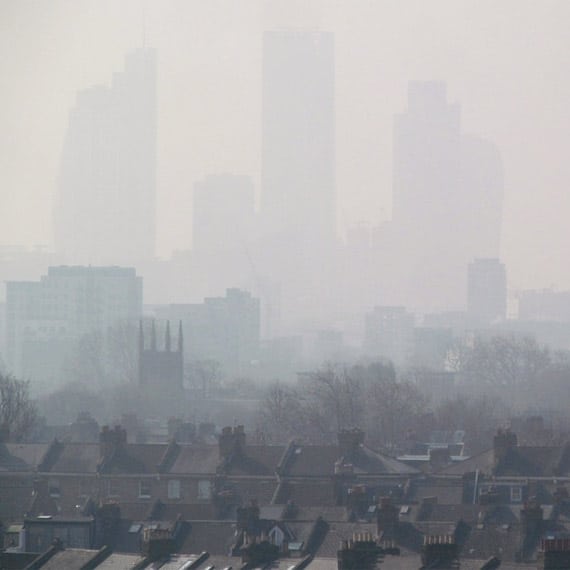
Air Quality Modelling
Effective air quality modelling and mitigation is necessary to reduce the 3m global deaths per year attributable to this cause. The key challenge is coupling global drivers to human-scale effects.
Air quality scientists typically couple process-based and statistical models working at different spatiotemporal scales, with simplistic model calibration, and without correct uncertainty propagation. For the first time, existing but under-utilised petabyte-scale global model simulation output will be combined with regional air quality models, and diverse environmental data streams and health records, linking heterogeneous models and data sources across vastly different spatial and temporal scales. We will build emulators of global simulator archives and regional atmospheric models to provide uncertainty-aware cross-scale inference. The vast quantities of data will require novel scalable Gaussian process emulation techniques.
Downscaling techniques will provide a mechanism for data integration, with calibration of combined models and data to predict local air quality issues under future climate and emissions scenarios. The combined framework will allow linkage with models of health impacts of air quality, providing an evidence base and decision support for vehicle emissions policies. Using a virtual lab we will address questions such as how to best use BT’s environmental IoT infrastructure to inform intervention strategies.
Land Use Change
In a changing climate, land use decisions must be made which trade-off between food, timber, energy, recreation, urban settlement, employment and aesthetic benefits. However, land management policy is fragmented, and decision-makers face difficult-to-integrate data deriving from new technologies.
Integrated assessment models have been developed which couple together multiple sectoral models to simulate land use change at the global and European levels, but the model assumptions are rarely rigorously tested against data, nor transparent, and they operate deterministically at coarse spatial scales. We will deploy new data science tools to interface these technologies and data, resulting in better-informed policy-making.
We will build emulators of the black box components comprising the CLIMSAVE Integrated Assessment Platform (IAP) to enable interfacing with (emulated) climate models and proper calibration of the ensemble to large volumes of available data. We will downscale this European model to UK scale, calibrated against an existing Scotland-only version of the IAP.
An agent-based model will be built which takes account of behavioural characteristics of land use managers to explore dynamic responses to national policy decisions for the first time; our techniques will interface this model with other modelling approaches. We will develop a virtual lab to facilitate discovery and provide a decision-support framework for policy-makers.
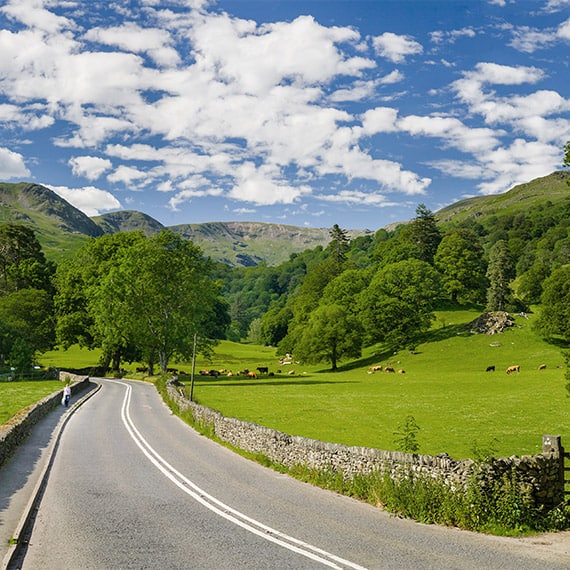