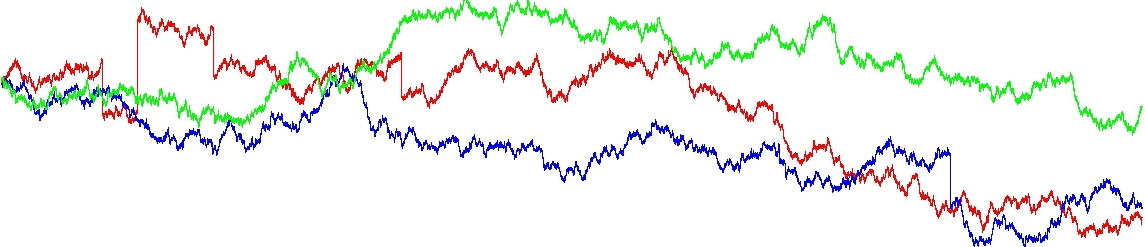
About us
Probability theory is concerned with evaluation of chances where the central subjects include discrete or continuous random variables, probability distributions, and stochastic processes, which provide mathematical abstractions of non-deterministic or uncertain processes or measured quantities that may either be single occurrences or evolve over time in a random fashion.
Classical areas of research in probability are Limit Theorems and Large Deviations. It goes back to Jacob Bernoulli in 1713 that, if we repeat independently some stochastic experiment many times, then the frequency of some event stabilises in a long run, this law is known as the law of large numbers. Modern probability theory provides further developments in this direction, among them the central limit theorem which is one of the great results of mathematics. It explains the ubiquitous occurrence of the normal distribution in nature.
Another classical area of research concerns Markov Processes which goes back to the early 20th century. It is an extension of independent random sequences where predictions can be made regarding future outcomes based solely on the present state and—most importantly—such predictions are just as good as the ones that could be made knowing the process's full history. Research has reported the application and usefulness of Markov chains in a wide range of topics such as physics, chemistry, biology, medicine, music, game theory and sports.
Among other areas of research in our department is one related to random graphs. Random graphs arise in several different contexts. Classical models give insight into how the density of a network affects its typical properties, and often a suitably modified random graph can be used to prove that graphs with certain properties exist, even when it is difficult to construct a specific example. Random graphs are also studied as models of networks that grow in an unpredictable way, and in recent years there has been particular interest in models that more closely resemble large real-world networks, such as preferential attachment and geometric random graphs. Percolation theory analyses the large-scale properties of random perturbations of highly structured networks, motivated by applications such as modelling fluid flow in disordered media. Interacting stochastic processes on graphs can be used to model opinion formation, the spread of infection through a community or the dynamics of a chemical reaction, and in many cases it is natural for the underlying graph also to be random.
Research is also conducted on stochastic processes and their interplay with models in mathematical physics, integrability, random matrices and interacting particle systems. Examples include random walks on matrix spaces, Brownian motion and h-transforms, directed polymers, exclusion processes and random recursive equations. The underlying mathematical structure of the latter objects can be analysed using methods from various fields of mathematics and some quantities of interest can often be computed explicitly in terms of special functions and/or determinants.
Noncommutative Probability is also an active research area in our department. In particular, quantum probability and quantum symmetry.
-
Group Members
Loading People
Projects
Permutations with random cycle weights and Bose-Einstein condensation
01/02/2023 → 31/01/2025
Research
Applied Matrix Positivity
01/03/2021 → 30/06/2021
Research
The Alliance Hubert Curien Programme 2021: Noncummuatative Probabilty, Matrix Analysis and Quantum Groups (NP, MA & Q Groups)
01/01/2021 → 31/12/2022
Research
Permutation Patterns as Noncommutative Central Limits
01/10/2020 → 30/09/2022
Research
Mathematical Theory of Symbiosis
01/08/2020 → 31/07/2022
Research
Support of Collaborative Research with Prof E. Steingrimmson at Hrísey, Iceland
01/08/2020 → 31/07/2022
Research
Support of collaborative research with Professor Volker Betz at University of Darmstadt, Germany
22/03/2020 → 04/04/2020
Research
An introduction to quantum filtering
24/06/2019 → 13/09/2019
Research
Quantum Random Walks and Quasi-Free Quantum Stochastic Calculus
05/01/2015 → 04/01/2017
Research
KTP:Industrial Mathematics shorter KTP with BT Research: TV Whitespace Interference Modelling
20/02/2013 → 19/07/2013
Research
FP7 Marie Curie People Action - Risk Analysis, Ruin and Extremes
01/12/2012 → …
Other
DFG - Lamperti type limit theorems for stochastic processes with asymptotically zero drift
01/01/2012 → …
Other
RFBR - Limit Theorems in Probability Theory and Their Applications
01/01/2011 → …
Other