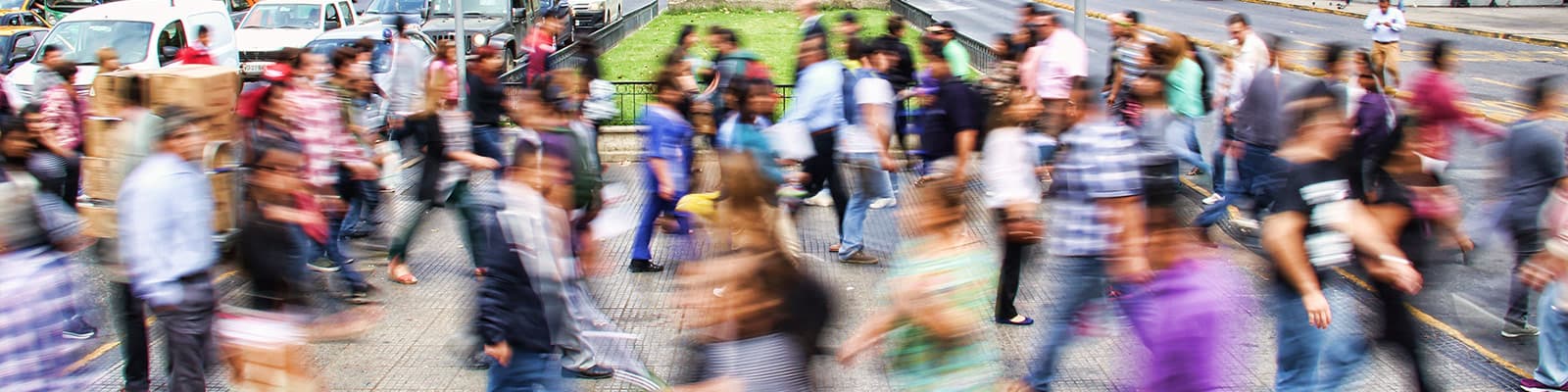
The Social and Economic Statistics group specialises in interdisciplinary social research, psychometrics, quantitative criminology and economic time series methods.
Interdisciplinary Social Research
Our social research focuses on developing methods for the analysis of social data and applying those methods to research problems in the health and social sciences. Application areas focus on foetal development and movement; psychology (child development), sociology (social care and looked after children) and crime (modern slavery and human trafficking). Members of the group often work in close collaboration with other departments at Lancaster, and with police and government organisations.
Statistical areas of development include missing data, new latent class analysis methods, statistical disclosure control, high-frequency count data in surveys; score building through log-linear modelling; estimation of hidden populations; longitudinal trajectory methods and synthetic data.
Psychometrics
Research in the social and behavioural sciences often hinges upon objective measurement of latent variables representing unobservable latent constructs. Associated statistical techniques include item response modelling, structural equation modelling, and more general latent variable models.
Methodological work in this area is focussed on topics such as the structural learning of latent variable models, multivariate outlier detection, change-point detection and algorithmic fairness. Recent or ongoing practical applications of such work include detection of cheating or item bias in standardised education tests, validation of indicators of infant and child development (in collaboration with the WHO) and evaluation of the psychometric properties of tools to assess patients' psychosocial needs.
Quantitative Criminology
The focus of this research area has been the analysis of criminal career data. This has involved the development of group-based trajectory models and latent growth mixture models to develop methods for the analysis of concepts such as escalation in offending , patterns of offending over time, precursors to homicide and serious sexual assault, and sexual and general recidivism, using police offending data. More recently, focus has shifted to victimisation surveys. examining issues of measurement of interpersonal violence, domestic violence and threats of violence and developing measures of harm. Cross-national comparative work has also been undertaken.
Economic Time Series Methods
Governments and institutions such as central banks are constantly looking to obtain more frequent, and timely information on the economy. However, relying on traditional survey-centric economic statistics presents challenges as they often lack granularity and are published with significant delays. Our research in collaboration with the Office for National Statistics, considers how modern model-selection techniques can be used to increase the resolution of survey-based measurements by using information from so-called “fast-indicators”, such as traffic flow, supermarket scanner data, or VAT returns. Extending these approaches within a Dynamic Factor model framework enables the construction of real-time estimates of economic activity whilst accounting for missing data.
A further area of methodological interest is the analysis and computation of parameter estimators associated with the volatility models of financial time series, such as the GARCH model. In particular, estimators are proposed that are robust and perform well even under various deviations from the underlying model assumptions. Applications of robust estimators are applied to the analysis of financial risk measures. Both theoretical and empirical properties of these estimators and the bootstrap approximations of their distributions are of interest.
-
Group Members
Loading People
Projects
Statistical Methods for Cost-Effectiveness Analyses (Oliver Chi)
01/10/2023 → 30/09/2025
Research
Statistical modelling of post-stroke disability and survival (Elijah Dawson)
01/10/2023 → 30/09/2025
Research
New stochastic models to quantify illegal trade
01/02/2023 → 31/01/2024
Research
Detecting soil degradation and restoration through a novel coupled sensor and machine learning framework (extension)
15/01/2023 → 14/07/2024
Research