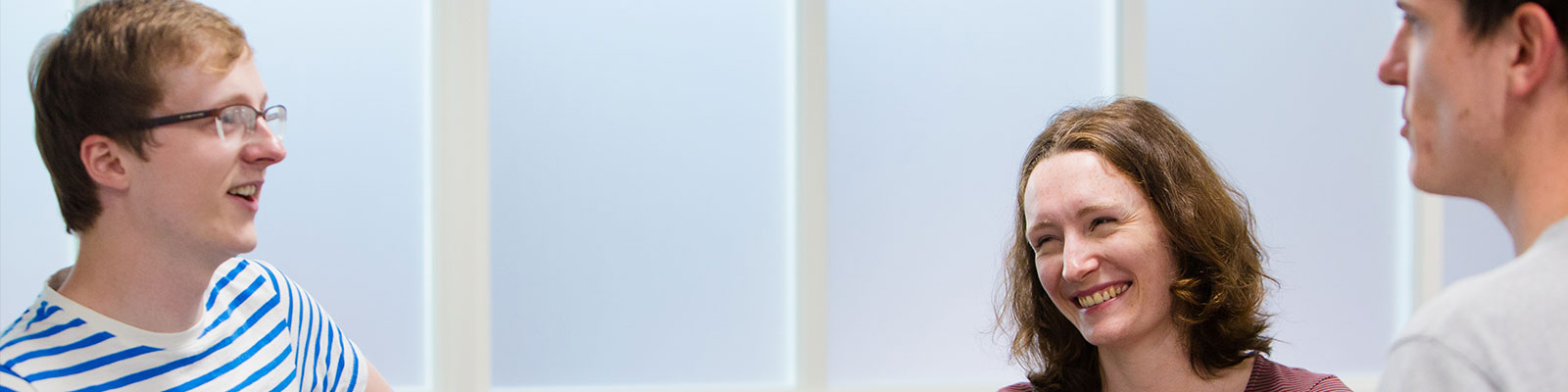
Postgraduate Research
Selecting a PhD Topic
The process of PhD project identification and vetting, the construction of supervisory teams, and the allocation of projects to students is managed closely by the STOR-i’s Executive Committee. Cross-disciplinary work is intrinsic to the operation of STOR-i and all students are supervised by a team representing at least two of the centre’s three constituencies (Statistics, OR, and industry). The majority of projects are with industry, but we also have a number of projects with our academic strategic partners. Typically 50% more projects are offered than are needed to ensure a wide range of options.
Approved projects are presented to the students in written form and via a series of talks at a Project Market, which leads to in-depth discussions between students, supervisors and external partners at the end of the second term.
At the start of the third term students select a sub-list of projects that they are interested in. Through a series of meetings with the Leadership Team their motivation for selecting the topics is explored. An allocation of projects is arrived at in May.
The three-month PhD Research Proposal project (STOR603) which concludes the MRes year (June-September) gives an opportunity to test the fit of students to projects/supervisory teams. In exceptional cases, students are able to change projects at the end of the MRes year.
PhD Projects
STOR-i projects have been developed with our industrial partners and use real-life issues to ensure our graduates are equipped to make a significant impact in the commercial world.
To see current and previous PhD projects choose a cohort below:
2023 PhD Cohort
Here you can find details of the PhD research projects undertaken by students who joined STOR-i in 2022 and started their PhD research in 2023. You can also access further technical descriptions by clicking on the links to the student's personal webpage below each project description.
Structured extreme value models for environmental science
Student Kristina Bratkova
Supervisor Jon Tawn
Academic Partner Oslo University
Environmental processes such as precipitation, temperature or wind can have devastating consequences, i.e., floods, drought, or wildfires. These extreme events can have severe impact on society, but they are quite rare, which means that making inference about them is complex. My work uses a particular type of statistical methodology based on extreme value theory. The focus is on statistical modelling of both multivariate and spatial-temporal extreme value problems. I will look to improve on existing methods, which typically require a large number of parameters, by making pragmatic and physically-motivated simplifying assumptions about the underlying structure of the extremal dependence. The type of assumptions I will look to make includes graphical model structure, vines, clustering and mixtures.
In the mutivariate setting, the aim is to develop generic methods and to explore their implementation to air pollutant data. For example, when multiple different chemicals are of interest, some chemicals are more directly related than others given they have common pollutant sources. In the spatial-temporal analysis, I will investigate adapting existing methods to very large spatial-scale studies, e.g., rainfall or temperature over the whole of Europe, which can have multiple separate extreme events occurring simultaneously; a feature that is not possible to describe in currently used models. The outcome of the research will be improved modelling and understanding of the extremal characteristics of a range of natural hazards.
Simulation optimisation for the housing of homeless populations
Student Graham Burgess
Supervisors Luke Rhodes-Leader, Rob Shone, Dave Worthington
Academic Partner Naval Postgraduate School
Government bodies around the world are responsible for housing which ensures that homeless people are not left unsheltered for extended periods of time and have access to good quality accommodation in the long run. To do this, they must balance the urgent requirements for emergency shelter with the long-term need for permanent housing, all within a limited budget.
The flow of people through government homeless care systems can be modelled as a queue. Due to the complex nature of such queueing systems, simulation modelling plays a vital role in estimating the societal impact of housing development plans. Simulation models are good at capturing the complexities of the real-world system but using them to find an optimal development plan is difficult. This project aims to use novel simulation optimisation algorithms to find near-optimal development plans in the context of homeless care systems. These algorithms will use a mathematical description of teh queue dynamics alongside a simulation model to efficiently explore different development options.
The simulation model will rely on forecasts for future homelessness which are highly uncertain. The challenge here is to appropriately consider the impact of this uncertainty on the simulation output and on the optimisation results. A further challenge is the fact that housing development plans are typically revisited – to account for this in the context of simulation optimisation will be a novel development.
Triggered bandits within streaming data settings
Student Theo Crookes
Supervisors James Grant, Idris Eckley
Industrial Partner BT
The main aim of this project is to develop novel decision-making alogrithms to integrate with current anomaly detection techniques in the streaming data setting. This project is partnered with BT; BT are a large multi-national telecommunications provider, managing around 28 million telephone lines within the UK alone, alongside providing maintenance for other areas of crucial national telecommunication infrastructure. A wide rang of important telecommunications data is collected across the BT network and is monitored by BT.
Anomaly detection methods have been developed for streamed data; these methods can be applied to the telecommunications data. Anomalies within telecommunications data are sometimes consequences of critical incidents; therefore, fast optimal decision-making after anomalies have been detected within BT is important to ensure critical national infrastructure is maintained.
The novel decision-making algorithms we will develop will be self-optimising and adaptive. Furthermore, the alogrithm will give feedback to the anomaly detection method to improve the accuracy and delay of detection.
Research questions regarding this project include, but are not limited to: How does the algorithm integrate with the anomaly detection method? What feedback does the algorithm provide to the anomaly detection method? How are anomalies classified?
This project has a Mathematical Sciences research theme, focused in the areas of Statistics, Operational Research and AI technologies.
Stastistical solutions for the open challenges of integrated population models
Student Max Howell
Supervisors Rachel McCrea, Chris Sherlock
Academic partner Oslo University
In the world of animal conservation, it is important to obtain accurate estimates of features such as population size, survival rates and fecundity. Historically, when data are collected on a species' population, a model is created to estimate these important features. However, when multiple different data types are collected on the same species, it is useful to find a way to combine these datasets to leverage the shared information. This is acheived through Integrated Population Models. However, there are still some issues with these models. For instance, choosing the best model that fits the data best becomes challenging when in the presence of multiple data types. Additionally, model-fitting algorithms that require a long running time and are difficult to implement make them less accessible for many ecologists. More efficient solutions are needed to make Integrated Population Models useful for a broader audience of ecologists. My PhD is looking at adapting and improving current model fitting algorithms, maintaining the use of closed form solutions, and thus, efficiency.
Research areas; Statistical Ecology, Computational Statistics
Optimising stochastic systems using streaming simulation
Student Robert Lambert
Supervisors James Grant, Rob Shone, Kevin Glazebrook
Academic Partner Naval Postgraduate School
Queuing theory is a well-established field of mathematics and methodologies from this field are often used to model stochastic systems. However, in many complex, real-world situations, deriving closed-form solutions from such models becomes impractical or even impossible. It is in these situations that we must turn to alternative optimisation methods.
One assumption that cannot be easily captured within classical queueing theory methodologies is the concept of customer impatience in the form of customer abandonment. The most common forms of these are reneging (leaving the system before receiving service) and balking (failing to join the system in the first place). Customers decide whether to balk or renege according to their own rules or preferences that might be unknown to the system controller.
We aim to develop novel sequential decision making policies to adaptively optimise these systems. Within these policies we must tackle the problems of parameter estimation, handling missing data and balancing learning with effective decision-making.
High-dimensional inference for models of antimicrobial resistance transmission in open populations
Student James Neill
Supervisors Chris Jewell, Lloyd Chapman
Industrial Partner Liverpool School of Tropical Medicine, Unilever
Antimicrobially resistant (AMR) bacteria are those for which antibiotics are significantly less effective than expected. The spread of AMR bacteria is a major and increasing global public health concern, as the introduction of any new antibiotic eventually leads to the spread of resistant bacteria. We will be mathematically modelling the transmission of AMR bacteria through open populations, and using data to learn about the underlying properties of the bacteria (e.g., rate of transmission). Performing this kind of inference is often complicated by incomplete data — we do not always know when an individual has been infected (only when they develop symptoms), and test results are not always 100% accurate.
This project is part of an investigation into the spread of AMR bacteria in the North West of England, which will be using data collected from hospitals, care homes, and across the community (since people in care settings are at particular risk of AMR-related infection). In order ot explain the transmission of AMR bacteria through open populations, we will be developing novel methods and inference methods, which we can use to analyse the data. We will start by developing methods for small-scale settings, such as hospitals and care homes, then develop methods for open settings, such as the wider Liverpool area.
Safety in reinforcement learning decision making
Student Adam Page
Supervisors James Grant, Chris Kirkbride, Anna-Lena Sachs
Industrial Partner Datasparq
Reinforcement Learning (RL) is the precursor to many AI systems and is a tool used to find a best-possible strategy for problems. On such problem is the dynamic pricing of goods that have a limited stock and will perish at the end of a sales period. The RL decision-maker balances two objectives – setting prices each day to generate the largest amount of profit possible, or varying prices ot learn about the customers' reaction to different prices, which would help to make better decisions in the future. To find the optimal pricing strategy, the decision-maker must consider expected demand, and find a balance between increasing the price as the good becomes scarcer while also keeping the price low enough that it is still able to generate a reasonable amount of revenue. Common examples where this problem would be applicable are hotels, pre-booked car parks, car rentals, and plane tickets.
The second aim of this project is to explore the aspect of safety. RL algorithms rely upon exploring and sampling every action to learn an optimal strategy. Depending on the context sampling every action will have negative side-effects. Within the dynamic pricing problem, we can highlight two main unsafe actions: setting a low price resultin in financial losses and setting a high price resuling in reputational damage to the seller. We aim to find a novel way to induce safety within RL algorithms, such that they can be used for general problems.
Evolution of statistical earthquake models to account for measurement errors and dependence
Student Wanchen Yue
Supervisors Jon Tawn, Israel Martinez-Hernandez
Industrial Partner Shell
Numerous strategies have been proposed and developed to lower CO2 emissions within the environment. Carbon Capture and Storage (CCS) systems have been identified as one of the most effective strategies. To enable a safe growth of such geological formations, any dangers over the linked induced seismic activity should first be evaluated. This evaluation requires extending existing approaches for assessing the potential hazards of tectonic earthquakes and human-induced earthquakes.
Specifically, our research aims to enhance existing statistical models, such as stochastic point process models (ETAS models) and extreme value methods, commonly used in earthquake analysis, incorporating features overlooked in current approaches. For instance, our research challenges assumptions about earthquake records and covariate information, investigating the effects of assumed independence in aftershock magnitudes.
Another novel aspect of our project involves accounting for measurement errros in both point process and extreme value methods, recognising the error properties of earthquake magnitudes, time and locations inferred from sensor network vibrations. Within this research project, we use historical earthquake catalogues. We aim to explore how strong assumptions affect results and incorporate related studies of uncertainties and dependence in seismicity modelling.

2022 PhD Cohort
Here you can find details of the PhD research projects undertaken by students who joined STOR-i in 2021 and started their PhD research in 2022. You can also access further technical descriptions by clicking on the links to the student's personal webpage below each project description.
Linear Models over the Space of Phylogenetic Trees
Student George Aliatimis
Supervisors James Grant, Burak Boyaci
Academic Partner Naval Postgraduate School
Discovering evolutionary relationships among various groups of organisms and the e-construction of ancestral relationships have applications including predicting evolution of fast evolution species, such as HIV, the tree of life project, coevolutions among different species and human evolutionary history. These methodologies can be applied to the evolutionary history of COVID-19 to analyse how they mutate so that scientists can develop effective vaccinations and they can also study how it spreads.
This proposal aims to develop new and powerful machine-learning models for genome-wide phylogenetic analysis (phylogenomics). Evolutionary hypotheses provide important underpinnings of biological & medical sciences and comprehensive genome-wide understanding of evolutionary relationships among organisms, including parasitic microbes, are needed to test and refine such hypotheses. Theory and empirical evidence clearly indicate that phylogenies (trees) of different genes (loci) should not display precisely matched topologies. The main reason for such phylogenetic incongruence is reticulated evolutionary history of most species due to meiotic sexual recombination in eukaryotes, or horizontal transfers of genetic material in prokaryotes. Nevertheless, most genes should display topologically related phylogenies and should group into one or more (for genetic hybrids) clusters in poly-dimensional tree space.
With the development of genetics, aligned gene sequences are used to reconstruct evolutionary history between species (phylogenetic tree), which has led to a mathematical and algorithmic approach to tree reconstruction. Our goal is to develop statistical methods over the space of all phylogenetic trees and investigate their strengths over classical methods. In this project we develop statistical methods that conform to tropical geometry. The project will start off by considering tropical logistic regression to classify gene trees to certain species, by providing a criterion for MCMC convergence in phylogenetic MLE tree estimation, and by creating a tropical linear regression with continuous response variables.
Keeping the Routes Open: Optimal Maintenance of Infrastructure Networks
Student Luke Fairley
Supervisors Peter Jacko, Rob Shone
Academic Partner Naval Postgraduate School
The maintenance and repair of large-scale transportation infrastructure is known to be very costly, as is the loss of efficiency and capacity when components of such infrastructure have been damaged. It is therefore of interest to allocate resources optimally to maintain such infrastructure. Specifically, given that the infrastructure is in some given state of repair or disrepair, with certain routes operating well and other routes damaged and not fit for use, we want to select or prioritise certain routes for repair so as to allocate resources as efficiently as possible. These decisions must also be made sequentially: every time a new route is known to have been damaged, or an ongoing repair has been completed, we want to make a new decision on what to do next.
Generic algorithms already exist which learn to control these sequential decision-making problems optimally. For our problem, optimal control would mean that we minimise the average combined cost of ongoing repairs and loss of capacity over a long period of time. However, for complicated problems such as ours, these algorithms are known to be extremely slow and are therefore unsuitable, so we must turn our attention to more approximate or heuristic (rule-of-thumb) techniques. Such techniques can explicitly incorporate any theoretical understanding of the problem, allowing optimal approaches to be learned much faster by exploiting any known properties. It is the creation and evaluation of such techniques, and the discovery or proof of theoretical properties, that is the focus of our research.
Our hope is that by finding suitable approximations or heuristics, these could be rolled out and applied to any network-based infrastructure, and yield results that outperform any simple naïve approaches.
A Multi-objective Optimization Framework for Supporting Decisions for Hazardous Waste Transportation and Disposal
Student Harini Jayaraman
Supervisors Burak Boyaci, Konstantinos Zografos
Large quantities of hazardous materials are transported and distributed annually throughout the world. Shippers, carriers, package makers, freight forwarders, consignees, insurers, and governments are just a few of the parties involved in securely transporting hazardous commodities from their origins to their destinations. With different priorities and viewpoints involved from various parties, hazmat transportation is a typical multi-objective optimization problem which makes it further complicated to solve these problems as there is a high level of public concern surrounding hazardous material transport problems due to the risks involved. Hence, it is fundamentally important to design and operate hazardous (nuclear) waste processes in a way that is safe, efficient, and economical.
Our goal is to develop effective and innovative optimization models that handle these three main objectives simultaneously; the transportation, storage and handling cost, the environmental cost, e.g. greenhouse gas emissions, related to transportation, and risks related to transport, e.g. handling and storage of the waste.
The multi-objective and dynamic character of the challenge at hand necessitates the creation of efficient algorithms capable of producing high-quality three-dimensional efficient frontiers in a reasonable time. Our research will explore and develop mathematical models and heuristic algorithms problems of this type.
End of Life Planning for Mobile Phones
Student Ben Lowery
Supervisors Anna-Lena Sachs, Idris Eckley
Industrial Partner Tesco Mobile
The management and control of stock in a business with integrated online and offline storefronts facing uncertainty in demand poses a great deal of operational challenges. Historically there has been much research into inventory control under uncertainty. However, inventory control at the end of sales life remains an under-researched area within the literature. In particular, the challenge of optimising stock levels to ensure remaining stock is sold before the end of sales life, as well as preventing stockouts and unsatisfied customers, is of vital importance. It is this challenge that lies at the heart of this PhD and will lead to development of novel operational research methodology at the intersection of three research areas: omnichannel retailing, end-of-life product management and stochastic inventory control. Novel methods and heuristics will be proposed to create effective inventory control policies.
Bayesian inverse modelling and data assimilation of atmospheric emissions
Student Thomas Newman
Supervisors Chris Nemeth, Phil Jonathan
Industrial Partner Shell
The exponential increase of gas emissions is in part responsible for Earth’s global warming. Today, we emit around 50 billion tonnes of greenhouse gases each year, with the majority produced by the burning of fossil fuels, industrial production, and land use change. Methane can be released during oil and gas extraction, this is often referred as “fugitive emissions”. The short lifetime of methane implies that reductions in its emissions rapidly results in lowering its concentration in the atmosphere. Hence, tackling methane emissions could be an effective and rapid way to mitigate some of the impacts of climate change. Stochasticity is an overarching problem in this research as there are many sources of randomness.
My research focuses on locating source(s) and quantifying emission rate(s) of anthropogenic greenhouse gases; with a focus on methane. To do so, I am modelling gas dispersion in the atmosphere and implementing probabilistic inversion for source characterisation. I am predicting spatio-temporal gas dispersion using Gaussian plume and other models from computational fluid dynamics based on Navier-Stokes equations and assessing their computational cost and accuracy under different atmospheric conditions. Additionally, I am developing novel methodologies involving gradient-based MCMC algorithms and Gaussian Processes to perform efficient probabilistic inversion, which identifies source(s) location based on gas concentration measurements. Due to the high-dimensional nature of the problem, MCMC inversion is computationally expensive. Hence, this research is undertaken with the aim to create models which are computationally fast and applicable, including for live tracking of emissions by drones or satellites.
A Prediction Model for Algorithm Selection in Solving Combinatorial Optimisation Problems
Student Danielle Notice
Supervisors Ahmed Kheiri, Nicos Pavlidis
Industrial Partner Tesco
The main objective of this project is to develop a model to predict which from a set of algorithms is most suitable for solving different instances of combinatorial optimisation problems. A large number of Tesco operations, such as delivery planning, vehicle routing problems and distribution systems, involve combinatorial optimisation. This model will be designed to meet the requirements of such problems large-scale retail problems. While these problems have been widely studied, the decision about which algorithm performs best on a particular instance, or class of instances is still unresolved.
Problems can be characterised using different features, and we will model the relationship between such features and the performance of different heuristic algorithms. We will also explore these models for problems with dynamic features that change with time.
The project will develop new analysis methods to help explain the performance of the algorithms for different problem instances. The techniques developed will be able to explain to decision-makers under which conditions we can expect those algorithms to provide trustworthy solutions and when we may expect that the solutions provided to be infeasible or of low quality.
Understanding Neuronal Synchronization in High-Dimensions
Student Carla Pinkney
Supervisors Alex Gibberd, Carolina Euan
Academic Partner University of Washington
Understanding the complex dynamics of the human brain is a challenging task involving researchers in both neuroscience and statistics. An area of statistical research concerns the characterisation of dependence between neurons as evidenced via their firing patterns and rates. Traditionally, the time-varying activity of neurons has been measured at an aggregate scale via methods such as functional magnetic resonance imaging (fMRI) and electroencephalography (EEG). However, due to recent technological advances, electrical activity can now be measured at an individual neuron level, e.g. via electrodes implanted directly into the brain or via calcium fluorescence imaging methods. These direct measurements provide the gold standard for quantifying localised activity.
After adequate pre-processing of the signals, measurements known as spike-trains can be obtained, which represent when a given neuron is firing. Statistically, these can be thought of as observations from a marked multivariate point process. While existing statistical methodology can capture the behaviour of a handful of neurons, the development of new technologies has enabled the recording of activity from hundreds of neurons. Therefore, there is both an opportunity, and a need, to develop new statistical methods capable of handling this increase in dimensionality.
In this project, we are primarily interested in characterising dependencies between neuronal point processes. To do so, fundamental techniques based on spectral analysis will be explored, with a particular focus on obtaining smoothed spectral estimates. In doing so, we hope to extract some meaningful dependencies in the signalling dynamics of neurons, and consequently provide an enhanced understanding of connectivity in the brain network.
Multivariate extremes of the ocean environment driving extremes in responses for ocean-structure interactions
Student Matthew Speers
Supervisors Jon Tawn, Phil Jonathan
Industrial Partner Shell, University of Western Australia
My project uses multivariate extreme value theory and methods to model and make statistical inferences about the behaviour of the physical ocean environment, in order to aid the design of marine structures. Characterising the ocean environment requires jointly modelling the evolution of variables representing multiple components of ocean wave surfaces, currents and near-surface wind fields. We are also interested in determining the forces induced on marine structures by the ocean environment. These environmental and structural loading variables often pose the greatest risk to structural integrity when their values are large. For this reason, we are most interested in modelling the behaviour of individual variables in their extremes, the extremal dependence between them, and their evolution during extreme events.
The project brings together a number of fields, including hydrodynamics and modelling of wave-structure interactions. Combined with extreme value analysis, expertise in these areas from Shell and the University of Western Australia will inform the development of statistical methodologies including environmental contour construction methods, which aim to characterise multivariate tails of distributions efficiently and informatively for improved marine design.
Diffusion-based Deep Generative Models for Assessing Safety in Autonomous Vehicles
Student Connie Trojan
Supervisors Chris Nemeth, Paul Fearnhead
Industrial Partner Transport Research Laboratory
It is hoped that the use of autonomous vehicles will significantly reduce the number of road accidents due to human error. However, extensive testing will be necessary to demonstrate that they satisfy a high standard of safety before they can be introduced. Much of this testing must be carried out in simulated environments, which allow for a far greater degree of safety and flexibility than road testing. This is done by testing the vehicle AI on a set of predetermined scenarios. Examples of scenarios include recovering from a loss of control due to road conditions, performing manoeuvres in the presence of oncoming traffic, and responding to a sudden deceleration by a leading vehicle. A key issue in autonomous vehicle safety is the need for a huge, diverse set of scenarios that reflect both normal driving conditions and difficult situations. This project aims to develop methods for automating scenario generation, both by reconstructing conditions from real life driving datasets, and by creating completely new scenarios using statistical generative modelling techniques from the statistical AI literature, such as generative adversarial networks and variational auto encoders.
The problem with current generative modelling techniques is to generate realistic scenarios from the same underlying distribution as a given dataset. Diffusion-based models are a recent advance in generative modelling, which use stochastic differential equations to gradually transform random noise into data via a diffusion process. This is learned by training a neural network to remove small amounts of noise from existing data. They are efficient to train and have been shown to be effective at generating diverse samples from complex, high-dimensional distributions. This research project will focus on the statistical properties of diffusion models and how they can be adapted for the scenario generation problem to generate realistic new scenarios given an existing database.
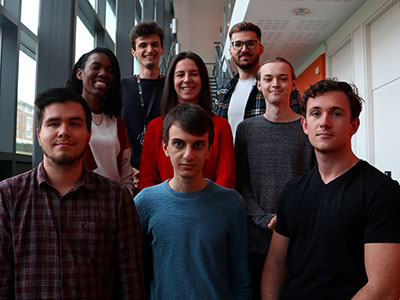
2021 PhD Cohort
Here you can find details of the PhD research projects undertaken by students who joined STOR-i in 2020 and started their PhD research in 2021. You can also access further technical descriptions by clicking on the links to the student's personal webpage below each project description.
Dependence Models for Actuarial Data
Student Lidia Andre
Supervisors Jenny Wadsworth
Industrial partner University College Dublin
When we fit a model to data, we are usually interested in representing the data the best way possible, and therefore in finding a model that fits the entire data set well. However, when the focus is on capturing the behaviour of extreme events – such as floods or large financial losses, for example - these models won’t perform well. As a solution, extreme value methods can be applied to model only the data in the tail. But what about the situation where the non-extreme data are also of interest? When the data available concerns a single variable - for example, the modelling of the rainfall distribution at a particular location - there are statistical methods available that aim to accurately model the body of the distribution (i.e., data that aren’t extreme) and the tail of the distribution (i.e., extreme events). However, when we have more than one variable - for example when an actuary is interested in modelling the dependence between liabilities to understand the exposure to risk – the situation is more complex, and sparse work has been done concerning the case where both extremes and the body are of interest. This project aims to fill this gap by investigating and developing dependence models which allow the tails to be considered appropriately, as well as accurately modelling the body of the data.
Modelling and Solving Generic Educational Timetabling Problems
Student Matthew Davison
Supervisors Ahmed Kheiri, Konstantinos Zografos
Industrial partner MIT
Universities have a large number of students enrolled on many different programs of study. They also employ lots of staff to facilitate the teaching of these courses. Ultimately, an individual event, such as a lecture or a workshop, needs to occur in a particular space at a particular time. This assignment is represented as a timetable and the aim is to produce a timetable that everyone is happy with.
Two questions arise from this description: How do you quantify how good a timetable is and how do you produce a good timetable? The novel work within this research will be to manage how people move around campus and use physical space efficiently as well as consider “virtual” online spaces which are becoming more popular. This will be done by mathematically forming the problem and researching methods that can be used to solve it.
A broader research question is to ask if this work can be used to guide strategic decision-making. A strategic decision is one that changes the long-term operation of an organisation. The aim is to establish a firm relationship between the timetabling problem and the strategic decisions that universities have to make.
Inspection Regime Optimisation in the Offshore Industry
Student Daniel Dodd
Supervisors David Leslie, Phil Jonathan
Industrial partner Fugro
Scientific experiments are essential for improving our perception and understanding of the world around us. Often these procedures are time-consuming and expensive, and as a result, deciding where and how to gather data is challenging. Our area of research, optimal design, concerns how to allocate resources when experimenting to learn as much as possible about the subject of interest.
This project is an international academic collaboration with the Australian Research Council (ARC) Research Hub for Transforming Energy Infrastructure through Digital Engineering (TIDE) based at the University of Western Australia (UWA) Oceans Institute, a multidisciplinary marine research centre. TIDE strive to create new research and technologies based on digital engineering to optimise the management of offshore energy infrastructure in consortium with leading offshore industry partners.
The primary objective of our research is to design tools that efficiently target inspections of submarine pipelines and renewable energy cables to minimise the risk of failures (which would have catastrophic environmental consequences). We aim to develop a methodology for sequential decision-making within a nonparametric paradigm, with considerations for sparse data and the costs of acquiring new observations. Our research is critical not only for the global offshore industry and applies to a wide range of other problems in maintenance, healthcare, epidemiology, search and rescue, and more.
Novel Methods for Changepoint Detection
Student Jacob Elman
Supervisors Paul Fearnhead, Idris Eckley
Often times we wish to detect when a process which generates data changes in some way. The times when the process changes are called “changepoints”, and their detection is of vital importance to many different fields. Changepoints for single processes are relatively well studied, but often we observe multiple, related processes, and changepoints in this setting are much harder to detect. One source of difficulty is that there can be changes in any subset of the processes, and the number of subsets grows exponentially with the number of processes, and so an additional computational cost is introduced in determining which subset of series undergoes a change. One potential approach to solving this problem is to associate a network with the processes, and then use the structure of the network to help inform us as to which subsets undergo changes. One of the goals of this project is to improve changepoint detection for multiple series; with an initial aim of exploiting the network idea described above to improve computational cost whilst retaining accuracy of estimated changepoints.
Reducing Obsolescence Waste at Automotive Manufacturers by Better Forecasting and Inventory Management
Student Robyn Goldsmith
Supervisors Anna-Lena Sachs, John Boylan
Automotive manufacturers operate large-scale aftermarket organisations. The aftermarket industry aims to satisfy customer requirements and can generate significant revenue. As part of this after-sales service, spare parts are intended to be available to vehicle owners for many years after the production of a vehicle ceases. This project concerns the decisions surrounding the parts of discontinued vehicles that need to be made to avoid customer dissatisfaction and waste. This includes the last purchase decision which is made to a supplier in order to cover the demand of a part during the remaining service period. Additionally, we are interested in an order decision which is made whilst switching suppliers and must sustain part availability for a period time, typically for one to two years.
This project aims to develop innovative, accurate and effective demand forecasting and inventory optimization models for spare parts subject to long-term purchase decisions. To address the objectives of this PhD, approaches will involve characterising demand structures of spare parts, incorporating demand uncertainty and specifying appropriate forecast accuracy criteria. More widely, this project will contribute to and extend the research areas of final ordering and forecasting in the end of life phase of spare parts.
Efficient Design of Grocery Distribution Networks
Student Rebecca Hamm
Supervisors Ahmed Kheiri
Supermarket chains require a large number of different products which are produced by many different suppliers to sell in a large number of stores. Hence they use a very large distribution network to transport products from suppliers to stores. The aim of this project is to produce a model which designs an efficient distribution network. Intermediate facilities are used to improve the network by allowing products to be transported in the most efficient manner.
The aim of this PhD is to develop a model/method which will support the decision-making with decisions corresponding to:
• Intermediate facilities: Which intermediate facilities should we use? We will need to decide whether to use intermediate facilities that already exist and/or build new ones. When building new ones we will need to consider the best location to build these facilities and which stores will be served by which intermediate facility.
• Routes: What routes through facilities should vehicles take?
• Flow through the network: What is the appropriate quantity of each product to transport between facilities?
The objective is to make the most cost-efficient network in terms of economic benefits and reduction in harmful emissions. Our plan is to use heuristic methods given the highly complicated nature of the optimisation problem.
Spatio-Temporal Modelling of COVID Prevalence Scenarios
Student Jordan Hood
Supervisors Chris Jewell, Carolina Euan
Industrial partner ONS
Since early 2020, the UK has been battling the SARS-CoV-2 (Covid19) pandemic. It has impacted the lives of every one of us, and no region of the UK has been able to avoid the virus. Ultimately, it is not an exaggeration to say the Covid 19 pandemic has rewritten much of the rulebook on successful epidemic modelling.
As the Covid 19 pandemic progresses, it is inevitable that we will experience waves and troughs of infections as the prevalence of the virus changes. In the future, the UK will likely reach an endemic state. This is after infection rates decline because of greater proportions of the population gaining resistance from both vaccine programs and surviving infections. Eventually, the UK will experience low but sustained infection rates. Currently, we are not equipped with the tools to provide meaningful UK-wide surveillance in the aforementioned low prevalence, “Endgame”, scenario.
The primary goal of the PhD project is to fill this gap in Spatio-Temporal statistical epidemiologic modelling, and to develop a meaningful method providing Covid 19 prevalence modelling across the UK as we progress into the Covid 19 Endgame. As the state of the SARS-CoV-2 pandemic is fast-moving at the best of times, it is realistic to expect the project to adapt to future concerns identified by the Office of National Statistics, this project’s sponsor, as the need arises.
The PhD project can be extended to address some open challenges the UK faces, such as Optimal Allocation of Randomised Survey Resources to Minimise Prevalence Uncertainty and extending current methods for Early Detection of Areas at Risk of High Prevalence Increase (hotspot detection). Altogether, the project hopes to help fill in the missing pages in successful epidemic modelling as the country at large looks to a future beyond Covid 19.
Optimising In-Store Price Reductions
Student Katie Howgate
Supervisors Jamie Fairbrother, Chris Nemeth
Industrial Partner Tesco
When selling products, demand for that product does not remain consistent throughout its lifetime. As time progresses a product is deemed less desirable by customers due to factors such as declining quality or newer improved products being released. We often wish to maximise revenue and keeping prices consistent while demand is decreasing is not likely to achieve this. This project looks at pricing strategy for products towards the end of their saleable lifetime, known as markdowns. In particular, we wish to find methods that are quick and efficient whilst also accurate. The project is in collaboration with Tesco and will focus on in-store markdown pricing of a vast array of types of products thus we may need to consider adaptable solutions. Some of the issues encountered are due to the historic sales data on past markdowns being truncated and censored: only a limited number of discount levels have been applied in practice (such as 25%, 50% and 75%) and sales figures do not equal demand (demand may be greater than available inventory). This can lead to a large amount of bias within our models. Additionally, there are a lot of uncertainties coming from a lack of knowledge on product quality and the availability of alternate colours and sizes. Current methods use a two-stage approach: first predicting the demand for products and then using this to find the optimal price(s) for the remaining sales period. We will explore novel methods for predicting demand and optimisation within markdowns and are interested in considering a holistic approach where the uncertainty of demand is taken into account within the optimisation.
Fusing Multi-Frequency Data Sources for Improving Health
Student Owen Li
Supervisors Rebecca Killick
Industrial partner Intelesant Ltd
In 2019, the Office for National Statistics reported over 4 million people aged 65 and over living alone. Families and relatives seek reassurance and comfort that their older relatives are looking after their health and wellbeing. This is especially important for the older generation as noticing any abnormal behaviour, such as inability to sleep or decrease in activity levels, and acting on this early can prevent more serious problems, like hospitalisations.
The goal is to accurately detect changes to an individual's routine behaviour and alert these changes to the individual and their relatives. In statistics, changepoint analysis is used to detect abrupt changes over time. This PhD will look into taking different data sources, e.g., smart meters, and using these additional pieces of information to enhance our ability to predict changes in an individual's daily behaviour. We will develop a new changepoint methodology, specifically taking advantage of the periodic nature of the problem.
Assessment of hazard and risk due to induced seismicity for underground CO2 storage and oil and gas production assets
Student Conor Murphy
Supervisors Jonathan Tawn, Peter Atkinson, Zak Varty
Industrial partner Shell
Production of oil and gas can cause seismic activity. Problematic levels of seismic activity are rare and occur only in a small minority of oil and gas fields. However, the potential impact of high levels of seismic activity justifies careful monitoring and modelling of hazards and risks. Such induced earthquakes also arise from a new strategy used to combat climate change, specifically where CO¬2 is captured and stored in underground reservoirs. Accurate forecasting of hazards under scenarios for future extraction/injection is vital in ensuring the process is operated safely. Statistical methodology plays an important role in the design of monitoring strategies and the assessment of these hazards and risks.
This project aims to improve upon current statistical models of marked point pattern data to inform the assessment of seismic hazards. Improvements to the geophone network at the site over time have allowed smaller magnitude earthquakes to be detected. This leads to the problem of missing data from the years where the geophone network was not dense enough to detect all small-magnitude seismic events. This project will explore a spatio-temporal threshold selection method using an extreme value analysis to address this issue.
Approaching Modern Problems in Multivariate Time Series via Network Modelling and State-Space Methods
Student Maddie Smith
Supervisors Adam Sykulski, Nicos Pavlidis
A sequence of time-indexed observations, describing how a particular variable evolves with time, is referred to as a time series. In many applications, we record multiple observations of the same phenomena, leading to multiple different time series describing the same variable. The challenge of combining the differing time series into one model, which captures the dependencies and allows the prediction of future time series values, is a non-trivial task. This project is in collaboration with an industry partner and aims to develop an effective method for the combination of such time-series measurements to enable accurate predictions of future values.
We will be addressing the question of how best to combine measurements from multiple different sources describing the same phenomenon, in addition to considering how dependent time series can be used to aid our predictions. This method should be able to deal with challenges presented by characteristics such as missing data, irregular sampling, unknown dependencies with other time-series and uncertainty about the observations. An ideal solution will take into account the uncertainties associated with each individual time series, and will aim to minimise and quantify the uncertainty of the combined prediction. Furthermore, it will be able to determine the best possible combination of the individual time series and adapt this combination in the case that some change occurs. The developed method will aim to be computationally efficient, and effective in substantive applications.
Reinforcement Learning to Improve Algorithms
Student Jack Trainer
Supervisors David Leslie, Tony Nixon
Industrial partner Heilbronn Institue
To make a computer perform a task, we need to supply it with step-by-step instructions. These instructions are called algorithms. In certain algorithms, there are steps where a choice needs to be made about which instruction to give next. How the rest of the algorithm performs depends on the choice of instruction. For many of these algorithms, rules have been devised to make these choices that try to maximise the amount of times a “good” decision is made leading to better overall performance for the algorithm.
Reinforcement learning (RL) is a mathematical method that enables us to learn the optimal way to behave with respect to a given task by interacting with it and observing the consequences of that interaction. In many cases, the strategies learnt using reinforcement learning can be different and better to any strategies devised by a human to accomplish that task. This has been demonstrated by reinforcement learning strategies that have been used to outperform the greatest human players in board games such as chess and go.
In this project, we want to explore whether RL can be used to devise strategies for making choices in algorithms that outperform the best strategies we have been able to devise so far. To accomplish this, we will look at an algorithm known as Buchberger’s algorithm which depends critically on such decisions. We will try to extend existing work in this area to solve problems in geometric rigidity, a pure mathematical research specialism at Lancaster University which has applications in a wide range of areas from civil engineering to molecular chemistry. If RL can boost the performance of Buchberger’s algorithm then this would enable the solution of more complex and interesting problems in this domain.
Anomaly Detection in the Internet of Things (IoT)
Student Ziyang Yang
Supervisors Idris Eckley, Paul Fearnhead
Industrial partner BT
The Internet of Things is a term used to describe a network of instrumented physical objects that are able to connect and exchange data with other devices and systems via the internet, such as smart homes, autonomous vehicles, etc. The torrent of data arising from the Internet of Things brings with it many new statistical challenges. For example, one might seek to synthesise information across multiple related streams to identify common (rarely seen) features. We call these unusual behaviours anomalies, and identifying and explaining these anomalies could provide meaningful insight.
In recent years, substantial work has been undertaken to detect changes and anomalies in individual signals. However, these are often constructed under idealised settings, failing to take into account the realities of constrained computation or limited communication within and between different devices. In this project, in partnership with BT, we seek to develop the statistical theory and methods required to deliver efficient and dependable detection within the constraints of the Internet of Things setting.
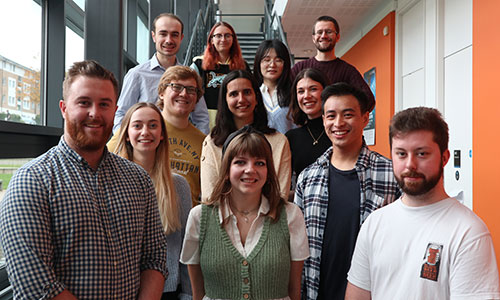
2020 PhD Cohort
Here you can find details of the PhD research projects undertaken by students who joined STOR-i in 2019 and started their PhD research in 2020. You can also access further technical descriptions by clicking on the links to the student's personal webpage below each project description.
Machine Learning Predictions and Optimization: Working together for better decisions
Student Aaditya Bhardwaj
Supervisors Christopher Kirkbride, Vikram Dokka
Industrial partner Tesco
I am working on a multidisciplinary PhD project related to non-perishable product pricing in association with Tesco. We aim to develop a robust pricing algorithm by using recent advancements in Operational Research, Artificial intelligence, and Combinatorics. Pricing decisions need to satisfy both the short-term business needs and the long-lasting impact on the future growth prospects of the organisation. In a competitive market with primarily homogeneous products, price is a key incentive for any consumer when making a purchase decision. Many companies rely on manual inputs for a pricing decision. However, these human-based methods are suboptimal, expensive, and prone to behavioural bias.
Developing an automated approach to set prices at all outlets of a supermarket chain is a significant challenge; current literature typically attempts this on a station-by-station basis, which ignores the inherited network structure. Furthermore, the pricing problem can be subdivided into two parts. First prediction, we require estimates of various model parameters such as demand, and competitor’s price. Second optimisation, we need to get an optimal selling price satisfying all the business objectives. The most common framework to integrate these two subproblems is a sequential process. However, any projections made on historical data are subject to uncertainty, but the underlying sequential process does not include this prediction uncertainty at upstream optimisation; thus, results in a suboptimal decision. We will develop a framework to optimally price non-perishable products across the network while accounting for the uncertainty in predictions.
Energy Spatial Pooling for Extremes Value Inference
Student Eleanor D'Arcy
Supervisor Jonathan Tawn
Industrial partner EDF
Safety is an overriding priority in the nuclear industry; strict rules and regulations must be maintained to avoid nuclear disasters. Such disasters often result from extreme environmental processes, such as flooding or storms. This project is in collaboration with EDF, their nuclear research and development team focus on programmes supporting the safety, performance, and life extension of existing nuclear fleet. EDF must demonstrate that their power plants are robust to rare natural hazards. This involves studying unusually high or low levels of an environmental process, then using this extreme data to extrapolate beyond what is observed to provide an insight into the probability of future extreme events.
The main statistical approach for understanding the risks associated with rare events is extreme value inference, where a statistical model is fit to the extreme values of a process. Estimates of the probabilities of future extreme events are subject to large amounts of uncertainty due to a lack of available data on such rare events. Reducing this uncertainty is desirable. Since data are usually available at multiple locations, it is sensible to try to incorporate this extra information into the inference at a single site. Additionally, we will explore the joint analysis of different environmental hazards, such as wind speed, sea level and rainfall. We plan to use these approaches for borrowing information to improve current methods for estimating extreme levels of a process.
Design and Analysis of Basket and Umbrella Trials
Student Libby Daniells
Supervisor Thomas Jaki, Pavel Mozgunov
Industrial partner Roche
Prior to treatments’ release onto the market, they undergo rigorous testing in clinical trials. Patients' responses to such treatments can vary based on their intrinsic factors, and thus it is desirable to target treatments to patient’s presenting different characteristics. To address this issue, so-called basket and umbrella trials are proposed. Basket trials consist of a single treatment applied to different patient groups who share a common characteristic but suffer from different diseases. Umbrella trials are composed of multiple treatments tested in parallel on patients who share the same disease but present a different genetic make-up.
We must also consider that, although the primary goal of a trial is to identify the most effective treatment, a secondary goal is to deliver the best treatment to patients within the trial. This is achieved through response-adaptive randomisation, a technique that alters the chance of being allocated a treatment on the basis of data from previously treated patients within the trial.
STOR-i and Roche have collaborated to create a project that aims to answer the following questions: How do we borrow information between treatment groups in basket trials? How can we utilize response-adaptive randomisation to improve the number of patients benefiting from the trial? And finally, how can we change the study populations of sub-studies within umbrella trials in order to incorporate newly identified targeted treatments? To tackle these problems, we first focus in on borrowing information between sub-studies and how to efficiently add a sub-group to a basket trial within this borrowing structure. Adding a sub-group is a highly beneficial feature of the aforementioned trial designs, as a new patient population may be identified part-way through the trial. Following this, we will explore the operating characteristics of response-adaptive randomisation and how it is impacted on by temporal changes in the disease or healthcare provision.
The Border Patrol Game
Student Matthew Darlington
Supervisors David Leslie, Kevin Glazebrook, Rob Shone
Industrial partner Naval Postgraduate School
There are many reasons we need to defend borders in the modern world. Not only are there the physical borders between countries where we wish to stop illegal trafficking and smuggling, there are the metaphorical borders in cybersecurity and intelligence collection. Whilst in an ideal world we would be able to simultaneously protect the whole border all of the time, due to constraints on budget or other factors it is common to patrol the border focusing on only a small section at a time.
We are using game theory and reinforcement learning techniques to develop strategies with which the defender can use to protect their border. We do this by considering the optimal actions both the smuggler and defender could take, and how they could then play against this.
The project will entail many different aspects of the applied probability and operational research literatures such as: multi-armed bandits, Stackelberg security games and Markov decision processes. We hope to bring these together to solve various problems in this project.
Design and Analysis of Platform Trials
Student Peter Greenstreet
Supervisors Pavel Mozgunov, Thomas Jaki
Industrial partner Roche
Bringing a new treatment to market is a long and expensive process, which can often end in failure. Platform trials are a class of clinical trials, which aim to increase efficacy compared to traditional trial designs via a possibility of adding new treatments to ongoing trials. Therefore, a statistical methodology for platform trials that allows new experimental treatments to be tested as efficiently as possible while satisfying the regulatory bodies’ standards is essential. Therefore, STOR-i and Roche have partnered together in order to create a project which is focused on answering the following three questions:
- When, why and how to add new treatments to an ongoing study?
- How can a sequence of trials be designed?
- How can a trial be best designed which has analyses conducted part way through the trial and where the trial focus is on comparing each treatment to one another?
The initial aim of the project is to develop methods that allow for the addition of new experimental treatments as the trial progresses. This is beneficial as during a course of confirmatory clinical trials - which can take years to run and require considerable resources - evidence for a new promising treatment may emerge. Therefore, it may be advantageous to include this treatment into the ongoing trial as this could benefit patients, funders and regulatory bodies by shortening the time taken comparing and selecting experimental treatments, thus allowing optimal therapies to be determined faster and reduce costs and patient numbers. The key part of the solution for this problem is making sure that the correct number of patients are recruited and that only treatments with enough evidence that they are better than the control treatment go on to the next phase, in order to meet regulatory bodies’ standards. After studying this question, the methodology will then be further developed for the other two questions.
Optimal Discrete Search with a Map
Student Edward Mellor
Supervisors Kevin Glazebrook, Rob Shone
Industrial partner Naval Postgraduate School
Effective search strategies are necessary in a wide range of real-world situations. The unsuccessful search for Malaysian Airlines flight 370 cost more than two hundred million Australian dollars. It is therefore important to understand how such large amounts of money can be used in the most efficient way possible were a similar event to happen in the future. Not only can the act of searching be very expensive but the risk of not finding the target of the search in time can be even more costly. For example, the earlier a rescue squad can find a missing person after a natural disaster the greater that person’s chances of survival.
The classical search problem assumes that the target of the search is hidden in one of multiple distinct locations and that when searching the correct location there is a known probability of discovery. In this case, the best possible order to search the locations can be found by modelling the search process as a multi-armed bandit. This is a well-studied mathematical model inspired by slot machines where a series of decisions are made to maximise some reward.
In the existing literature, most search models assume that these locations can be moved between instantaneously and at no additional cost. This assumption massively simplifies the problem but doesn’t hold in many real-world applications. Over the course of this PhD, our aim is to develop and evaluate the effectiveness of search strategies that incorporate the time or financial costs of travelling between locations.
Methodology and theory for unbiased MCMC
Student Tamas Papp
Supervisor Chis Sherlock
Industrial partner University College Dublin
As the power and thermal limits of silicon are being reached, modern computing is moving towards increased parallelism. Fast computation is primarily achieved through the usage of many independent processors, which split up and perform the computation task simultaneously. This poses a challenge for Markov chain Monte Carlo, the gold standard of statistical computing, which is an inherently sequential procedure.
A recently proposed methodology enables principled parallel processing for Markov chain Monte Carlo and offers the potential to overcome this challenge. While straightforward to implement, the method may incur a significant computational overhead, rendering it impracticable unless the number of available processors is in the order of thousands, or even more.
This project aims to enhance the practicality of the aforementioned methodology, making it competitive with other methods even when the number of processors is in the tens or hundreds. The focus is on: 1) reducing the computational overhead, either through direct refinements or by applying post-processing techniques, and 2) producing practical guidelines for the optimal performance of the new methodology, through theoretical analyses. The work undertaken in this project will be of use to practitioners and researchers who rely on simulation to draw conclusions from their statistical models, throughout science, technology, engineering, and mathematics.
Efficient network design with optimal slot offering for grocery home delivery
Student Matthew Randall
Supervisor Ahmed Kheiri, Adam Letchford
Industrial partner Tesco
Online grocery delivery is an increasingly used service in society and is becoming something people rely on more and more by the day. The main challenge of online grocery delivery problems, as opposed to other kinds of delivery problems, is the requirement for the customer to select a time slot in which they wish to receive their delivery, which the route then has to accommodate. In principle, being able to choose any slot they desire is ideal for customers; however it can lead to the creation of highly inefficient routes. Consider for example a scenario in which there are two deliveries on the same road which have been ordered for delivery windows six hours apart: from a perspective of minimising the distance being travelled, this is not a good situation to be in, as it will involve sending delivery vans to the same road twice in one day.
The aim of this project is to research methods that can help the supermarket decide which delivery slots to offer customers when they place an order on the company website. There are two objectives, which typically conflict: maximizing customer choice, and minimising the total driving time. As well as having two objectives, the problem has stochastic and dynamic aspects, since customers visit the website more or less at random over time. Moreover, the problem has combinatorial aspects, due to the selection of delivery slots and the need to produce routes for the vehicles. In order to solve this highly complicated optimisation problem, heuristic methods are likely to be necessary. It may also be necessary to use simulation, to assess the (expected) performance of various heuristics.
Resource allocation under uncertain demand in Royal Mail Centres
Student Hamish Thorburn
Supervisors Anna-Lena Sachs, Jamie Fairbrother, John Boylan
Industrial partner Royal Mail
Mail and parcel delivery companies have thousands of letters and parcels arriving at distribution centres each hour, each needing to pass through multiple different work areas to be sorted by their destination and size (letter vs parcel). In many work areas, items are sorted by hand, requiring the company to roster workers in the mail centres to sort these letters and parcels.
There are two considerations here. Firstly, the delivery company is required to sort the post within given timelines (e.g. certain proportions of different items need to be sorted on time). Rostering on more staff means that the letters and parcels will be sorted quicker. However, more staff members lead to higher operating costs for the company. Therefore a balance needs to be found.
If this were the whole problem, this could be solved with a number of existing methods. However, there is another complication. The staff rosters need to be determined before the number of letters and parcels incoming during a shift is known.
My PhD will involve developing and extending methods to determine optimal staffing levels in a mail centre. While initially applied to the parcel sorting problem, some of the techniques we will develop may apply more generally to the area of decision-making under uncertainty, and the wider field of Operational Research.
Novel Anomaly Detection Methods for Telecommunication Data Streams
Student Kes Ward
Supervisors Idris Eckley, Paul Fearnhead
Industrial partner BT
Anomaly detection is used in many places, and almost everywhere lots of data are processed, to answer questions like "is this transaction fraudulent?" or "do we need to switch off this expensive piece of machinery and do a maintenance check?" or "is there a planet orbiting this star?". The methods used to do this are complex and varied, and not all of them are fast enough to function well on streams of data that arrive in real-time.
This PhD approaches the anomaly detection problem from a statistical standpoint. Instead of heavy models that require lots of training data and computational power, it looks at lighter-touch algorithms that can work well in applications where efficiency is important and detect anomalies in real-time as soon as they develop. This involves testing, evaluating, and developing methods using both real and simulated datasets.
The project is sponsored by BT, and some of the problems they tackle are about flagging up issues in the telecommunications network that engineers need to go out and fix. These show up as strange blips in overall network usage over time against a backdrop of normal human behaviour (which can itself be very strange). Dealing with ways to distinguish anomalies from the varying structure in the data signal is one of the project's focus areas.
Anomaly Detection for real-time Condition Monitoring
Student Tessa Wilkie
Supervisors Idris Eckley, Paul Fearnhead
Industrial partner Shell
The aim of this project is to develop reliable methods of flagging strange behaviour in real-world data sets.
One such data set might consist of several series of measurements monitoring a system over time. Odd behaviour is often a precursor to something going wrong in a system. Condition monitoring — detecting early warnings of problems in a system for maintenance purposes — is based on this idea.
We are interested in two particular types of odd behaviour: anomalies — where behaviour departs from and then returns to the typical; and changepoints — where there is a permanent shift in the typical behaviour shown in a series.
Many methods exist to detect anomalies and changepoints, but they can struggle in the face of the difficulties that real-world data sets present: such as large size, dependence between series, and changing typical behaviour. The aim of this PhD is to develop methods that work well on data sets that exhibit one or more of these issues.
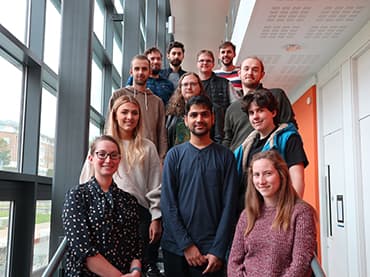
2019 PhD Cohort
Here you can find details of the PhD research projects undertaken by students who joined STOR-i in 2018 and started their PhD research in 2019. You can also access further technical descriptions by clicking on the links to the student's personal webpage below each project description.
Novel methods for the detection of emergent phenomena in data streams
Student Edward Austin
Supervisors Idris Eckley and Lawrence Bardwell
Industrial partner BT
Every day, more than 90% of households and over 95% of businesses rely on the BT network for internet access. This is not only for personal use, such as streaming content or browsing social media but also for commercial use such as performing transactions. Given the number of users and the importance of digital networks in our everyday lives, any faults on the network must be detected and rectified, as soon as possible.
In order to facilitate this, the volume of data passing through the network is monitored at several locations. The aim of this PhD is to develop new statistical approaches that are capable of detecting when the volume of data being observed differs substantially from that expected. These differences can take a variety of forms, emerging gradually over time. The project aims to not only detect the onset of these phenomena but to perform the detection in real-time.
Multivariate extremes for nuclear regulation
Student Callum Barltrop
Supervisor Jenny Wadsworth
Industrial partner Office for Nuclear Regulation
The Office for Nuclear Regulation (ONR) is responsible for the regulation of nuclear safety and security of GB nuclear-licensed sites. ONR’s Safety Assessment Principles (SAPs)* expect nuclear installations to be designed to withstand natural hazards with a return frequency of one in 10,000 years, conservatively defined with adequate margins to failure and avoiding ‘cliff-edge’ effects. This involves extrapolating beyond the observed range of data. A statistical framework is used to model and estimate such events.
For my PhD project, I am working with the ONR to investigate methods for applying multivariate extreme value theory. In particular, I am looking at techniques for estimating ‘hazard curves’ (graphs of frequency and magnitude) for combinations of natural hazards that could inform the design bases for nuclear installations. I am also considering new methods for incorporating factors such as climate change and seasonal variability into the analysis of environmental data.
Automated resource planning through reinforcement learning
Student Ben Black
Supervisors Chris Kirkbride, Vikram Dokka and Nikos Kourentzes
Industrial partner BT
BT is the UK’s largest telecommunications company, and they employ over 20,000 engineers that work in the field. The engineers do jobs relating to television, internet and phone, and these jobs require different skills to complete. For BT, planning is very important in letting them have enough person-hours available to be able to complete all of the jobs that they have appointed, and also enough engineers with the required skills to do so. Planning the workforce entails, for example, deciding on how many hours of supply BT should make available for each type of job and assigning the engineers’ hours to their different skills.
My project is concerned with these two aspects of planning. Due to the size of the problem at hand, it is naturally best to solve it automatically. The main approach we will use to help automate BT’s planning process is reinforcement learning (RL). RL is a set of methodologies based on how humans and animals learn through reinforcement. For example, dogs learn to sit down on command by being given a treat when they sit, which acts as positive reinforcement. This is the general idea we aim to use; good planning actions will be rewarded, and bad ones will be penalised. Over time, this allows us to learn which planning actions should be taken in which demand scenarios. This approach is not common in workforce planning, and so the research we do here will provide a novel, automatic and fast planning approach that will provide optimal plans for even the largest of workforces.
Learning to group research profiles through online academic services
Student George Bolt
Supervisors Simon Lunagomez and Chris Nemeth
Industrial partner Elsevier
Elsevier is a company which specialises in the provision of online content and information to researchers. Through a large portfolio of products, such as the reference manager Mendeley, or the searchable database of literature ScienceDirect, they aim to help academics with every aspect of the research life cycle.
As a joint venture between STOR-i and Elsevier, this PhD project looks to develop and apply tools from network analysis to make sense of their often high-dimensional but structured datasets. Of particular interest is using data for their various platforms, which lends itself to a natural network-based representation. Successful analysis of these data would allow Elsevier to understand better how its platforms are being used, thus guiding their future development and the improvement of the user experience. The end goal is the development of methodologies which are not only applicable and useful for the problems at hand, but also novel within the wider network analysis literature.
Route optimisation for waste collection
Student Thu Dang
Supervisors Burak Boyaci and Adam Letchford
Industrial partner Webaspx
Many countries now have curbside collection schemes for waste materials, including recyclable ones (such as paper, card, glass, metal, and plastic) and non-recyclable ones (such as food and garden waste). Optimising the routes taken by the vehicles can have dramatic benefits, in terms of cost, reliability and CO2 emissions. Although there is a huge academic literature on vehicle routing, many councils still use relatively simple heuristic methods to plan their routes. This PhD project is concerned with the developments of improved algorithms for this task.
The routing problems that emerge in the context of waste collection have several key characteristics. First, they are often large-scale, with thousands of roads or road segments needing treatment. Second, the area under consideration usually has to be partitioned into regions or districts, with each region being served on a different day. Third, the frequency of service may depend on the material being collected and on the season (e.g., garden waste might be collected more often in summer than in winter). Fourth, the vehicles have limited capacity, in terms of both weight and volume. As a result, they periodically need to travel to specialised facilities (such as recycling plants or landfill sites) to unload, before continuing the rest of their route. Fifth, there is a limit on the total time spent travelling by each driver. Finally, one must consider the issues of fairness between drivers.
Due to the complexity of these problems, it is unlikely that they can be solved to proven optimality in a reasonable amount of time. Thus, in this PhD, we will develop fast heuristics that can compute good feasible solutions, along with lower-bounding techniques, which will enable us to assess the quality of the heuristic solutions.
Methods for streaming fraud detection in online payments
Student Chloe Fearn
Supervisors David Leslie and Robin Mitra
Industrial partner Featurespace
Whilst credit cards and online purchases are very convenient, the presence of fraudulent transactions is problematic. Fraud is both distressing for the customer and expensive for banks to investigate and refund, so where possible, transactions made without the cardholder’s permission should be blocked. Featurespace have designed a modelling approach which is successful at blocking fraudulent transactions as frequently as possible, without often blocking transactions that were genuinely attempted by the customer.
Due to ever-evolving behaviours by fraudsters to avoid getting caught, the classifier that decides whether or not transactions are fraudulent needs to be updated frequently. We call this model retraining, and the process requires up-to-date labelled data. However, when transactions are blocked, the truth on whether they really were fraud or not is unknown. As a result, these transactions are difficult to use for model retraining so they must be used, or not used, with caution. My project is concerned with how to utilise best the information we have. We aim to first look at how to accept transactions in a way that provides the classifier with the most information, and second, to think about using the transactions that were blocked for model training, by carefully predicting whether they were fraudulent or genuine.
Modelling wave interactions over space and time
Student Jake Grainger
Supervisors Adam Sykulski and Phil Jonathan
Industrial partner JBA Trust
The world’s oceans play an important role in many aspects of modern life, from transportation to energy generation. Ocean waves are one of the main challenges faced by vessels and structures operating in the oceans and drive the waves that cause coastal flooding and erosion. In certain conditions, these waves can cause severe damage, endangering structures, vessels, communities and lives.
The resulting scientific challenge is to understand the conditions that can cause instances of catastrophic damage. To do this, it is common to describe the conditions in a given area of the ocean. It is then possible to understand what kind of impacts we would expect on a structure or vessel that is in these conditions or on coastal communities when these waves propagate onshore.
To do this, we use data, taken from a measuring device, such as a buoy, situated in the area of interest. We then try to estimate the conditions that could have given rise to these observations. Usually, scientists and engineers do this by developing general models for ocean wave behaviour that they then fit the observed data. In most cases, these models have to account for multiple wave systems. The waves systems behave differently if they are generated locally (wind sea waves) than if they have travelled from elsewhere in the ocean (swell waves). An added complexity is that these weather systems interact with one another in ways that are very difficult to predict, presenting an extra challenge to those interested in modelling wave behaviour.
Throughout the course of this project, we aim to utilise state of the art techniques from time series analysis to improve the way in which practitioners can estimate model parameters and model how conditions can change over time. More advanced techniques can also be employed to explore the non-linear interactions between swell and wind sea systems, which plays an important role in determining the conditions that are experienced in practice.
Simulation analytics for deeper comparisons
Student Graham Laidler
Supervisors Lucy Morgan and Nicos Pavlidis
Industrial partner Northwestern University (Evanston, USA)
Businesses and industries across every sector are reliant on complex operations involving the movement of commodities such as products, customers or resources. Many manufacturing processes, for instance, move a constant flow of products through a production sequence. To allow for informed and cost-effective decision-making, managers need to understand how their system is likely to perform under different conditions. However, the interactions of uncertain variables such as service times and waiting times lead to complex system behaviour, which can be difficult to predict.
Building a computer model of such a system is an important step towards understanding its behaviour. Stochastic simulation provides a probabilistic modelling approach through which the performance of these systems can be estimated numerically. With a combination of machine learning and data analytic techniques, this project aims to develop a methodology for simulation output analysis which can uncover deeper insights into simulated systems.
Information fusion for non-homogeneous panel and time-series data
Student Luke Mosley
Supervisors Idris Eckley and Alex Gibberd
Industrial partner Office for National Statistics
The Office for National Statistics (ONS) has the responsibility of collecting, analysing and disseminating statistics about the UK economy, society and population. Official statistics have traditionally been reliant on sample surveys and questionnaires; however, in this rapidly evolving economy, response rates of these surveys are falling. Moreover, there exists a concern of not making full use of new data sources and the continuously expanding volume of information that is now available. Today, information is being gathered in a countless number of ways, from satellite and sensory data to social network and transactional data. Hence, ONS is exploring how administrative and alternative data sources might be used within their statistics. In other words, how might they remodel the 20th century survey-centric way into the 21st-century combination of structured survey data, with administrative and unstructured alternative digital data sources?
My PhD project is to assist the ONS with this transformation, by developing novel methods for combining insight from the alternative information recorded at a different periodicity and reliability, with traditional surveys, in order to meet the ever-increasing demand for improved and more detailed statistics.
Input uncertainty quantification for large scale simulation models
Student Drupad Parmar
Supervisors Lucy Morgan, Richard Williams and Andrew Titman
Industrial partner Naval Postgraduate School
Stochastic simulation is a well-known tool for modelling and analysing real-world systems with inherent randomness such as airports, hospitals, and manufacturing lines. It enables the behaviour of the system to be better understood and performance measures such as resource usage, queue lengths or waiting times to be estimated, thus facilitating direct comparisons between different decisions or policies.
The stochastic in stochastic simulation comes from the input models that drive the simulation. These input models are often estimated from observations of the real-world system and thus contain an error. Currently, few consider this source of error known as input uncertainty when using simulation as a decision support tool. Consequently, decisions made on the basis of simulation results are at risk of being made with misleading levels of confidence which can have significant implications. Although existing methods allow for input uncertainty to be quantified and hence any risk to be nullified, these methods do not work well for simulation models that are large and complex. This project aims to develop a methodology for quantifying input uncertainty in large-scale simulation models so that crucial and expensive decisions can be made with better risk assessments.
Statistical analysis of large-scale hypergraph data
Student Amiee Rice
Supervisors Chris Nemeth and Simon Lunagomez
Industrial partner The University of Washington (Seattle, USA)
Connections between individuals happen countless times every day in a plethora of ways; from the messages sent on social media to the co-authorship on papers. Graphs provide a way for representing these relationships, with individuals represented by points (or nodes) and the connection between them represented with a line (or edge). This graph structure has been well studied in statistics, and it is known that when a connection involves more than two individuals (maybe an email chain with three or more individuals in it), a graph might not capture the whole story. An alternate construction that enables us to represent connections involving two or more individuals is known as a hypergraph. Hypergraphs can capture a single connection between three or more individuals and so statistical analysis on these kinds of connections is made more feasible.
As technology advances, the ability to collect and store data is becoming increasingly easy. The abundance of data makes the analysis of large-scale groups of connections between individuals problematic. The PhD will focus on exploring the way that hypergraphs can be used to represent connections as well as aiming to make scalable methods that can handle many individuals.
Multivariate oceanographic extremes in time and space
Student Stan Tendijck
Supervisors Emma Eastoe and Jonathan Tawn
Industrial partner Shell
In the design of offshore facilities, e.g., oil platforms or vessels, it is very important - both for safety and reliability reasons - that structures - old and new - can survive the most extreme storms.
Hence, the focus of this project is centred around modelling the ocean during the most extreme storms. In particular, we are interested in the aspects of the ocean that are related to structural reliability. Wave height is widely considered to be the most important; however, also other environmental variables such as wind speed can play a significant role. Together with Shell, we intend to develop models that can be used to capture (1) the dependence between environmental variables, such as wind speed and wave height, to characterise the ocean environment, (2) the dependence of these variables over time, as it should be taken into account that large waves occur throughout a storm, and (3) the dependence of all these characteristics of the ocean at different locations. These models can then be used to, for example, estimate whether or not old oil rigs are strong and safe enough.
Moreover, a key part of the research will be to develop novel methods to model mixture structures in extremes. This is also directly applicable to the above since waves can be classified into two types: wind waves and swell waves. Even though both types of waves have different characteristics, and it is impossible to classify a wave with a 100% certainty in most scenarios. Hence, it is of practical importance that models need to be developed that can deal with these types of dependency structures.
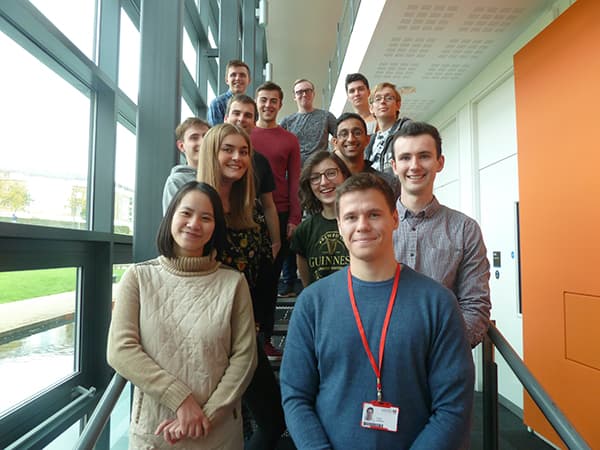
2018 PhD Cohort
Here you can find details of the PhD research projects undertaken by students who joined STOR-i in 2017 and started their PhD research in 2018. You can also access further technical descriptions by clicking on the links to the student's personal webpage below each project description.
Optimal Scheduling for the Decommissioning of Nuclear Sites
Student Matthew Bold
Supervisors Christopher Kirkbride, Burak Boyaci and Marc Goerigk
Industrial partner Sellafield
With production having come to an end at the Sellafield nuclear site in West Cumbria, the focus is now turning to the decommissioning of the site, and safe clean-up of legacy nuclear waste. This is a project that is expected to take in excess of 100 years to complete and cost over £90 billion. Given the large scale and complexity of the decommissioning project, it is crucial that each task is systematically choreographed according to a carefully designed schedule. This schedule must ensure that the site is decommissioned in a way that satisfies multiple targets with respect to decommissioning speed, risk reduction and cost, whilst accounting for the inherent uncertainty regarding the duration of many of the decommissioning tasks. My research aims to develop optimisation methods to help construct such a schedule.
Online Changepoint Methods for Improving Care of the Elderly
Student Jess Gillam
Supervisors Rebecca Killick
Industrial partner Howz
The NHS is under great pressure from an ageing population. Due to great advancements in modern medicine and other factors, the NHS and other social care services must provide the necessary care to a growing population of elderly people. This PhD project is partnered with Howz. Howz is based on research that implies changes in daily routine can indicate potential health risks. Howz use data from sensors placed around the house and other low-cost sources such as smart meter data to detect these changes. Alerts are then sent to the household or immediate care facilitators, where permission has been granted, to check on their safety and wellbeing. To the NHS, early intervention such as this is likely to result in fewer ambulance call-outs for elderly patients and fewer elderly requiring long hospital stays.
The objective of this PhD is to provide novel ways of automatically detecting changes in human behaviour using passive sensors. The first focus of the PhD will be in sensor-specific activity and considering changes in behaviour as an individual evolves over time.
On Topics Around Multivariate Changepoint Detection
Student Thomas Grundy
Supervisors Rebecca Killick
Industrial partner Royal Mail
Royal Mail deliver between forty and fifty million letters and parcels daily. In order for this process to run smoothly and efficiently, the data science team at Royal Mail are using innovative techniques from statistics and operational research to improve certain application areas within the company.
My research will aim to create and develop time-series analysis techniques to help tackle some of the open application areas within Royal Mail. Time-series data are collected over time and a key analysis is to identify time-points where the structure of the data may change; a changepoint. Current changepoint detection methods for multivariate time-series (time-series with multiple components) are either highly inefficient (take too long to get an answer) or highly inaccurate (do not correctly identify the changepoints) when the number of time-points and variables grows large. Hence, my research will aim to produce a multivariate changepoint detection method that is computationally efficient, as the number of time-points and dimensions grows large, while still accurately detecting changepoints. This method will be extremely useful within many of the open application areas within Royal Mail.
Rare Disease Trials: Beyond the Randomised Controlled Trial
Student Holly Jackson
Supervisors Thomas Jaki
Industrial partner Quanticate
Before a new medical treatment can be given to the public, it must first go through a number of clinical trials to test its safety and efficiency. Most clinical trials at present use a randomised control design, such that a fixed proportion (usually 50%) of patients are allocated to the new treatment and the other patients are given the control treatment. This design allows the detection of the best treatment with high probability so that all future patients will benefit. However, it does not take into account the wellbeing of the patients within the trial.
Response-adaptive designs allow the allocation probability of patients to change depending on the results of previous patients. Hence, more patients are assigned to the treatment that is considered better, in order to increase the wellbeing of patients within the trial. Multi-Armed bandits are a form of response-adaptive design, which maximise the chance of a patient to benefit from the treatment. They balance ‘learning’ (trying each treatment to decide which is best) and ‘earning’ (allocating the patients to the current best treatment to produce more patient successes).
Response-adaptive designs are not often used in practice, due to their low power. This low power means it can be difficult to find a meaningful difference between the treatments within a trial. Hence more research is needed to extend response adaptive methods such that they both: maximise patient successes and produce high enough power to find a meaningful difference between the treatments.
Statistical Learning for GPS trajectories
Student Michael O'Malley
Supervisors Adam Sykulski and David Leslie
Evaluating risk is extremely important across many industries. For example, in the motor insurance industry, a fair premium price is set by fitting statistical models to predict an individual's risk to the insurer. These predictions are based on demographic information and prior driving history. However, this information does not account for how an individual drives. By accurately assessing this factor insurers could better price premiums. Good drivers would receive discounts and bad drivers penalties.
Recently insurers have started to record driving data via an onboard diagnostic device known as a black box. These devices give information such as speed and acceleration. In this project, we aim to gain an understanding of how this information can be used to better understand driving ability. This will involve developing statistical models that can predict risk more accurately than traditional methods.
Scalable Monte Carlo in the General Big Data Setting
Student Srshti Putcha
Supervisors Christopher Nemeth and Paul Fearnhead
Industrial partner The University of Washington (Seattle, USA)
Technological advances in the past several decades have ushered in the era of “big data”. Typical data-intensive applications include genomics, telecommunications, high-frequency financial markets and brain imaging. There has been a growing demand from industry for competitive and efficient techniques to make sense of the information collected.
We now have access to so much data that many existing statistical methods are not very effective in terms of computation. In recent years, the machine learning and statistics communities have been seeking to develop methods which can scale easily in relation to the size of the data.
Much of the existing methodology assumes that the data is independent, where individual observations do not influence each other. My research will seek to address a separate challenge, which has often been overlooked. We are interested in extending “big data” methods to dependent data sources, such as time series and networks.
Data-Driven Alerts in Revenue Management
Student Nicola Rennie
Supervisors Catherine Cleophas and Florian Dost
Industrial partner Deutsche Bahn
In industries such as transport and hospitality, businesses monitor and control customer demand by either optimising prices or adjusting the number of products available to customers in different price buckets, in a process called revenue management. The objective being to increase revenue. Forecasts of customer demand are made, based on data collected from previous booking curves. Customer booking behaviour which deviates from the expected demand, for example around the time approaching carnivals or major sporting events, needs to be brought to the attention of a revenue management analyst. Due to the large networks and the complexity of the forecasts, it is often difficult for analysts to correctly adjust forecasts or product availability.
My PhD aims to develop methods which highlight such deviations between real-world observations and the expected behaviour in order to assist analysts in targeting booking curves and potentially make a recommendation to those analysts about what action should be taken. Data-driven alerts rely on pattern recognition and are already common in the domain of credit card fraud detection. A similar principle could apply in revenue management, detecting booking behaviour that deviates significantly from the automated forecasts. By employing similar approaches to those in the practice of fraud detection, the project will lead to the development of a prototypical alert system that is able to predict, with a degree of confidence, likely targets for analyst interventions.
Aggregation and Downscaling of Spatial Extremes
Student Jordan Richards
Supervisors Jonathan Tawn and Jenny Wadsworth
Industrial partner The Met Office
Historical records show a consistent rise in global temperatures and intense rainfall events over the last 70 years. Climate change is an indisputable fact of life, and its effect on the frequency and magnitude of extreme weather events is evident from recent events. The Met Office develops global Climate Models, which detail changes and developments in global weather patterns caused by climate change. However, very little research has been conducted into establishing a relationship between the extreme weather behaviour globally, and locally; either within smaller regions or at specific locations.
My PhD aims to develop statistical downscaling methods that construct a link between global, and local, extreme weather. We hope that these methods can be used by the Met Office to improve meteorological forecasting of future, localised, extreme weather events. This improvement will help to see the avoidance of the large-scale costs associated with avoidable damage to infrastructure caused by extreme weather; such as droughts or flooding.
Ranking Systems in Sport
Student Harry Spearing
Supervisors Jonathan Tawn
Industrial partner ATASS
The age-old question: "who is the best?"
Pick your favourite sport. Chances are, you have an opinion on who the best in the world is, at this current moment, or of all time, or who would win if A played B. But is it possible to develop a system which returns an objective answer to these questions?
In developing such systems, it is crucial to capture as much information as possible about the dynamic world in which we live. Understand it. Learn from it. Predict it. Athlete’s injuries, the weather, and even economic factors all impact the outcome of these events and the implied ability of the athletes or teams. This project requires a wide range of strategies in order to capture these signals, from graph theory to extreme value theory, and contextual information from news websites, so that the most accurate system of ranking sports teams or athletes is formulated.
Ranking systems in sport are not only interesting to the inquisitive fan, but a fair and accurate system is at the core of all sports organisational bodies and the multi-billion pound industries that they represent.
But these systems are not exclusive to sports.
Methodological advances in the field of sports ranking systems have far-reaching consequences. Ranking systems are used to rank webpages, or to rank schools and hospitals, or even to determine the most essential medical treatments. So, a ranking system based on poor methodology can have much more severe repercussions than incorrectly seeding a tennis tournament… Ultimately, the importance of ranking systems is self-evident, and sport creates a fruitful playground in which ample advancements can be made.
Evaluation of the Intelligence Gathering and Analysis Process
Student Livia Stark
Supervisors Kevin Glazebrook and Peter Jacko
Industrial partner Naval Postgraduate School
Intelligence is defined as the product resulting from the collection, processing, integration, evaluation, analysis and interpretation of available information concerning foreign nations, hostile or potentially hostile forces or elements or areas of actual or potential operations. It is crucially important in national security and anti-terror settings.
The rapid technological advancement of the past few decades has enabled a significant growth in the information collection capabilities of intelligence agencies. Said information is collected from many different sources, such as satellites, social networks, human informants, etc. to the extent that processing and analytical resources may be insufficient to evaluate all the gathered data. Consequently, the focus of the intelligence community has shifted from collection to efficient processing and analysis of the gathered information.
We aim to devise effective approaches to guide analysts in identifying information with the potential to become intelligence, based on the source of the information, whose characteristics need to be learnt. The novelty of our approach is to consider not only the probability of an information source providing useful intelligence but the time it takes to evaluate a piece of information. We aim to modify existing index-based methods to incorporate this additional characteristic.
Recommending Mallows
Student Anja Stein
Supervisors David Leslie and Arnoldo Frigessi
Industrial partner Oslo University, Norway
Recommender systems have become prevalent in present-day technological developments. They are machine learning algorithms which make recommendations, by selecting a specific range of items for each individual, which they are most likely to be in interested in. For example, on an e-commerce website, having a search tool or filter is simply not enough to ensure good user experience. Users want to receive recommendations for things, which they may not have considered or knew existed. The challenge recommender systems face is to sort through a large database and select a small subset of items, which are considered to be the most attractive to each user depending on the context.
In a recommendation setting, we might assume that an individual has specified a ranking of the items available to them. For a group of individuals, we may also assume that distribution exists over the rankings. The Mallows model can summarise the ranking information in the form of a consensus ranking and a scale parameter value to indicate the variability in rankings within the group of individuals.
We aim to incorporate the Mallows model to a recommender system scenario, where there are thousands of items and individuals. Since the set of items that an individual may be asked to rank is too large, we usually receive data in the form of partial rankings or pairwise comparisons. Therefore, we need to use methods to predict a user's ranking from their preference information. However, many users will be interacting with a recommender system regularly in real-time. Here, the system would have to simultaneously learn about its unknown environment that it is operating in whilst choosing alternative items with potentially unknown feedback from users. Hence, the open problem we are most concerned about is how to use the Mallows model to make better recommendations to the users in future.
Predicting Recruitment to Phase III Clinical Trials
Student Szymon Urbas
Supervisors Christopher Sherlock
Industrial partner AstraZeneca
In order for a new treatment to be made available to the general public, it must be proven to have a beneficial effect on a disease with tolerable side effects. This is done through clinical trials, a series of rigorous experiments examining the performance of a treatment in humans. It is a complicated process which often takes several years and costs millions of pounds. The most costly part is Phase III which is composed of randomised controlled studies with large samples of patients. The large samples are required to establish the statistical significance of the beneficial effect and are estimated using the data from the Phases I and II of the trials.
The project concerns itself with the design of new methodologies for predicting the length of time to recruit the required number of patients for Phase III trials. It aims to use available patient recruitment data across multiple hospitals and clinics including early data from the current trial. The current methods rely on unrealistic assumptions and very often underestimate the time to completion, giving a false sense of confidence in the security of the trial process. Providing accurate predictions can help researchers measure the performance of the recruitment process and aid them when making decisions on adjustments to their operations such as opening more recruitment centres.
Interactive Machine Learning for Improved Customer Experience
Student Alan Wise
Supervisors Steffan Grunewalder
Industrial partner Amazon
Machine learning is a field which is inspired by human or animal learning and has the objective to create automated systems, which learn from their past, to solve complicated problems. These methods often appear as algorithms which are set in stone. For example, an algorithm trained on images of animals to recognise the difference between a cat or a dog. This project instead concentrates on statistical and probabilistic problems which deal with an interaction between the learner and some environment. For instance, if our learner is an online store which wishes to learn customer preferences by recommending adverts and receiving feedback on these adverts through whether or not customer clicks on them. Multi-armed bandit methods are often used here. These methods are designed to pick the best option out of a set of options through some learner-environment interaction. Multi-armed bandit methods are often unrealistic, therefore, a major objective of this project is to design alterations to the multi-armed bandit methods for use in real-world applications.
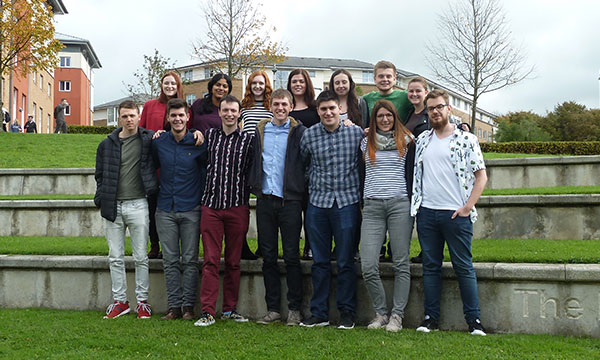
2017 PhD Cohort
Here you can find details of the PhD research projects undertaken by students who joined STOR-i in 2016, and started their PhD research in 2017. You can also access further technical descriptions by clicking on the links to the student's personal webpage below each project description.
Anomaly detection in streaming data
Student Alexander Fisch
Supervisors Idris Eckley and Paul Fearnhead
Industrial partner BT
The low cost of sensors means that the performance of many mechanical devices, from plane engines to routers, is now monitored continuously. This is done in order to detect problems with the underlying device in order to allow for action to be taken. However, the amount of data gathered has become so large that manual inspection is no longer possible. This makes automated methods to monitor performance data indispensable.
My PhD focusses on developing novel methods to detect anomalies, or untypical behaviour, in such data streams. More effective methods would allow detecting a wider range of anomalies, which in turn would allow detecting problems earlier, thus reducing their impact. Anomaly detection methods are also used for a range of other applications ranging from fraud prevention to cybersecurity.
Dynamic Allocation of Assets Subject To Failure and Replenishment
Student Stephen Ford
Supervisors Kevin Glazebrook and Peter Jacko
Industrial partner Naval Postgraduate School
It is often the case that we have a set of assets to assign to some tasks, in order to reap some rewards. My problem is as follows: we have a limited number of drones, which we wish to use to search in several areas. These drones have only limited endurance, and so will need to return and be recharged or refuelled at some point.
The complication of failure and replenishment adds all sorts of possible difficulties: what if one area takes more fuel to traverse so that drones deployed there fail more quickly? What if the drones are not all identical, with some capable of searching better than others?
These sorts of problems are simply too complicated to solve exactly, so my research will look at heuristics – approximate methods that still give reasonably good results.
Novel wavelet models for nonstationary time series
Student Euan McGonigle SupervisorsRebecca Killick and Matthew Nunes Industrial partner NAG
In statistics, if a time series is stationary – meaning that its statistical properties, like the mean, do not change over time – there is a huge wealth of methods available to analyse the time series. However, it is normally the case that a time series is nonstationary. For example, a time series might display a trend – slow, long-running behaviour in the data. Nonstationary time series arise in many diverse areas, for example, finance and environmental statistics, but these types of time series are less well-studied.
The Numerical Algorithms Group (NAG), the industrial partner of the project, is a numerical software company that provides services to both industry and academia. There is an obvious demand to update and improve existing software libraries continually: statistical software for use with nonstationary time series is no exception.
The main focus of the PhD is to develop new models for nonstationary time series using a mathematical concept known as wavelets. A wavelet is a “little wave” – it oscillates up and down but only for a short time. Wavelets allow us to capture the information in a time series by examining them at different scales or frequencies. The ultimate aim of the PhD is to develop a model for nonstationary time series that can be used to estimate both the mean and variance in a time series. Such a model could then be used, for example, to test for the presence of a trend in a time series.
Real-Time Speech Analysis and Decision Making
Student Henry Moss
Supervisor David Leslie and Paul Rayson
Many techniques from machine learning, specifically those that allow computers to understand human speech and writing, are used to aid decision-making. Unfortunately, these procedures usually provide just a final prediction. No information is provided about the underlying reasoning or the confidence of the procedure in its output. This lack of interpretability means that the decision-maker has to guess the validity of the analysis, and so limits their ability to make optimal decisions.
We plan to combine procedures from computer science and statistics to analyse these transcriptions. By using statistical models for grammar, style and sentiment, we will be able to provide interpretable and reliable decision aids.
Estimating diffusivity from oceanographic particle trajectories
Student Sarah Oscroft
Supervisor Adam Sykulski and Idris Eckley
The ocean plays a major role in regulating the weather and climate across the globe. Its circulation transports heat between the tropics and the poles, balancing the temperatures around the world. Ocean currents impact weather patterns worldwide while transporting organisms and sediments around the water. Studies of the ocean have a number of practical applications, for example, knowledge of the currents allows ships to take the most fuel-efficient path across the ocean, track pollution such as an oil or sewage spill, or aid in search and rescue operations. These studies can help with building models of the climate and weather which can be used in predicting severe weather events such as hurricanes.
To accurately build models for the ocean, we require knowledge of how it varies geographically in space and time. Such data is obtained from a variety of sources including satellites, underwater gliders, and instruments which freely drift in the ocean, known as floats and drifters.
This project will build new statistical methods for analysing such data, using novel methods from time series analysis and spatial statistics. A particular focus will be to find accurate methods for measuring key oceanographic quantities such as mean flow (a measure of currents), diffusivity (the spread of particles in the ocean), and damping timescales (how quickly energy in the ocean dissipates over time). Such quantities feed directly into global and regional climate models, as well as environmental and biological models.
An early focus of the project will be on diffusivity. Knowledge of how particles spread with time allows us to gain a better understanding of, for example, how an oil spill will spread in the water and the impact that it will cause. Diffusivity can also be used to give an insight into the spreading of radioactive materials which are released into the water or how ocean life such as fish larvae and plankton will disperse. Another application is in aeroplane crashes in the ocean, as using the diffusivity to predict where the debris came from can aid recovery missions.
Efficient clustering for high-dimensional data sets
Student Hankui Peng
Supervisor Nicos Pavlidis and Idris Eckley
Industrial partner ONS Data Science Campus
Clustering is the process of grouping a large number of data objects into a smaller number of groups, where data within each group are more similar to each other compared to data in different groups. We call these groups clusters, and clustering analysis involves the study of different methods to group data in a reasonable way depending on the nature of the data. Clustering permeates almost every facet of our lives: music is classified into different genres, movies and stocks into different types and sectors, food and groceries that are similar to each other are presented together in supermarkets, etc.
The Office for National Statistics (ONS) are currently using web-scraping tools to collect price data from three leading websites (TESCO, Sainsbury’s, Waitrose) in the UK. We are motivated by the problem of efficiently grouping and transforming a large number of web-scraped price data into a price index that is competitive to the current CPI index. Exploring novel clustering schemes that are able to conduct computationally efficient clustering in the face of missing data, and monitor the changes in each cluster over time, will be the main focus of my PhD.
Real-time Railway Rescheduling
Student Edwin Reynolds
Supervisor Matthias Ehrgott
Industrial partner Network Rail
Punctuality is incredibly important in the delivery of the UK’s railway system. However, more than half of all passenger delay is caused by the late running of other trains in what is known as a knock-on, or reactionary delay. Signallers and controllers attempt to limit and manage reactionary delay by making good decisions about cancelling, delaying or rerouting trains. However, they often face multiple highly complex, interrelated decisions that can have far-reaching and unpredictable effects and must make these decisions in real-time. I am interested in optimisation software which can help them out by suggesting good, or even optimal solutions. In particular, my research concerns the mathematical and computational techniques behind the software. I am sponsored by Network Rail, who hope to benefit from improvements to decision making and therefore a reduction in reactionary delay.
Changepoint in Multivariate Time Series
Student Sean Ryan
Supervisor Rebecca Killick
Industrial partner Tesco
Whenever we examine data over time, there is always a possibility that the underlying structure of that data may change. The time when this change occurs is known as a change point. Detecting and locating changepoints is a key issue for a range of applications.
My research focuses on the problem of locating changepoints in multivariate data (data with multiple components). This problem is challenging because not all of the individual components of the data may experience a given change. As a result, we need to be able to find the location of the change points and the components affected by the change. Current methods that try to solve this problem are either computationally inefficient (it takes too long to calculate an answer) or don't identify the affected components. The aim of my project is to develop methods that can locate changepoints alongside their affected components accurately and efficiently.
Large-Scale Optimisation Problems
Student Georgia Souli
Supervisors Adam Letchford and Michael Epitropakis
Industrial partner Morgan Stanley
Optimisation is concerned with methods for finding the ‘best’ among a huge range of alternatives. Optimisation problems arise in many fields, such as Operational Research, Statistics, Computer Science and Engineering. In practice, Optimisation consists of the following steps. First, the problem in question needs to be formulated mathematically. Then, one must design, analyse and implement one or more solution algorithms, which should be capable of yielding good quality solutions in reasonable computing times. Next, the solutions proposed by the algorithms need to be examined. If they are acceptable, they can be implemented; otherwise, the formulation and/or algorithm(s) may need to be modified, and so on.
In recent years, the optimisation problems arising have become more complex, due for example, to increased legislation. Moreover, the problems have increased in scale, to the point where it is now common to have hundreds of thousands of variables and/or constraints. The goal of this project is to develop new mathematical theory, algorithms and software for tackling such problems. The software should be capable of providing good solutions within reasonable computing times.
Statistical methods for induced seismicity modelling
Student Zak Varty
Supervisor Jonathan Tawn and Peter Atkinson
Industrial partner Shell
The Groningen gas field, located in the north-east of the Netherlands, supplies a large proportion of the natural gas that is used both within the Netherlands and in the surrounding regions. This natural resource is an important part of the Dutch economy, but the extraction of gas from the reservoir is associated with induced seismic activity in the area of the extraction.
The aim of my PhD is to allow seismicity forecasts to be used to inform future extraction procedures so that future seismicity can be reduced. In order to do this, a framework needs to be produced for comparing the abilities of current and future forecasting methods. Extra challenges, and therefore opportunities, are added to this task by the sparse nature of the events being predicted and the evolving structure of the sensor network that is used to detect them.

2016 PhD Cohort
Here you can find details of the PhD research projects undertaken by students who joined STOR-i in 2015, and started their PhD research in 2016. You can also access further technical descriptions by clicking on the links to the student's personal webpage below each project description.
Statistical models of widespread flood events as a consequence of extreme rainfall and river flow
Student Anna Barlow
Supervisors Jonathan Tawn and Christopher Sherlock
Industrial partner JBA
Flooding can have a severe impact on society causing huge disruptions to life and a great loss to homes and businesses. The December 2015 floods across Cumbria, Lancashire and Yorkshire caused widespread damage and tens of thousands of properties were left without power. Governments, environmental agencies and insurance companies are keen to know more about the causes and the probabilities of the re-occurrence of such events to prepare for future events. Therefore we wish to understand better the flood risk and the magnitude of losses that can be incurred. This PhD project with JBA Risk Management is concerned with modelling such extreme events and estimating the total impact.
In order to assess the risk from flooding, one needs to simulate extreme flood events, and improving upon the existing model for this is the main focus of this project. The simulation of flood events is important in understanding the flood risk and determining the potential loss. This part of the project is based on extreme value theory since we are interested in the events that create the greatest losses for which there may be little or no past data. Extreme value theory is the development of statistical models and techniques for describing rare events.
The second part of the project will be concerned with improving the efficiency of the estimation of large potential losses from the simulated flood events. Current methods involve multiple simulations of the loss at an extremely large number of properties over many flooding events. So we wish to improve the computational burden by reducing the number of simulations while retaining an acceptable degree of accuracy.
Optimal Search Accounting for Speed and Detection Capability
Student Jake Clarkson
Supervisors Kevin Glazebrook and Peter Jacko
There are many real-life situations which involve a hidden object needing to be found by a searcher. Examples include a bomb squad seeking a bomb or a land mine; a salvage team the remains of a ship or plane; and a rescue team survivors after a disaster. In all of these applications, there is a lot at stake. There can be huge costs involved in conducting the searches, within marine salvage, for example. Or, there can be consequences if the search is unsuccessful, valuable equipment could be forfeited or damaged, or, even worse, human life could be lost. Therefore, it is very important to search in the most efficient manner, so the search ends in a minimal amount of time.
When the space to be searched is split into distinct areas, the search process can be modelled as playing a multi-armed bandit, which is a mathematical process, named after slot machines, in which consecutive decisions must be made. Existing bandit theory can then be used to easily calculate the optimal order in which areas should be searched, thus solving this classical search problem.
The main focus of this PhD is to expand the existing theory for the classical search problem to accommodate search problems with two extra features. The first is to allow the searcher a choice of fast or slow search speed. This idea is often prominent in real-life problems, for example, the bomb squad may have a choice between travelling quickly down a stretch of road in a vehicle with sensors, and proceeding on foot with trained sniffer dogs. The vehicle travel, analogous to the fast search, covers the road more quickly, but the chance of missing a potential bomb upon that road will increase. Being on foot with the trained dogs, corresponding to the slow speed, will take more time to cover the same distance, but may well detect a hidden bomb with a larger probability. The second feature removes the ability of the searcher to search any area at any time, another often realistic assumption. For example, the bomb squad can only examine roads adjacent to their current location, to reach roads a further distance away, they must first make other searches.
Late-stage combination drug development for improved portfolio-level decision-making
Student Emily Graham
Supervisors Thomas Jaki, Nelson Kinnersley and Chris Harbron
Industrial partner Roche
Pharmaceutical companies will often have a variety of drugs undergoing development, and we call this collection a pharmaceutical portfolio. Since drug development is a long, expensive and uncertain process, it is important that the decisions we make regarding this portfolio are well informed and are expected to be the most beneficial to the company and the patient population. We are interested in the problem of optimal portfolio decision making in the context of a pharmaceutical portfolio containing combination therapies.
Combination therapies combine existing drugs and new molecular entities with an aim to produce an efficacious effect but with fewer side effects. While some methods do exist for portfolio decision making, they do not take into account combination therapies or the information which can be gained from trials containing similar combinations. For example, if drug A+B is performing well, this may influence the beliefs that are held about how A+C will perform and whether or not it should be added to the portfolio. We believe that taking into account similarities between combinations and sharing information across trials could lead to better decision making and hence better outcomes for the portfolio.
Automated Data Inspection in Jet Engines
Student Harjit Hullai
Supervisors David Leslie, Nicos Pavlidis, Azadeh Khalegh and Steve King
Industrial partner Rolls Royce
Advances in technology have seen an explosion of high-dimensional data. This has brought a lot of exciting opportunities to gain crucial insights into the world. Developing statistical methods for gaining meaningful insights from this rich source of data has brought some interesting challenges and some very notable failures. There is a need for consistent statistical methods to understand and utilise the vast amounts of data available.
My PhD is focused on developing statistical techniques for finding anomalies with Jet engine data. A Jet engine is a complicated system, with various sensors monitoring a huge number of features from temperature, air pressure etc. Applying standard anomaly detection methods on this data would be computationally expensive, taking potentially years to run. Therefore the challenge is finding methods for capturing the important information from this vast amount of data, and make meaningful inferences.
We need to find ways of extracting the important information from this high-dimensional data in a computationally efficient way. We must also ensure this information contains the necessary information for identifying the true anomalies in the full data. My focus will, therefore, be on developing novel methods for identifying and extracting meaningful information and finding anomalies that correspond to issues in the full data.
Operational MetOcean Risk Management under Uncertainty
Student Toby Kingsman
Supervisors Burak Boyaci and Jonathan Tawn
Industrial partner JBA
One of the main ways that the UK is increasing the amount of renewable energy it generates is by building more offshore wind farms. With the advent of new technologies, it is possible to both build bigger turbines and situate them further out at sea. Though these developments are big improvements, wind turbines still require a large amount of government subsidy to make them competitive with fossil-fuelled power stations. One way of helping to reduce the need for this subsidy is by carrying out maintenance activities more efficiently.
An example of this is the question of how to route vessels around the wind farm to carry out repairs in the most cost-efficient manner. Sending a large number of ships to deal with the tasks will get them completed quickly but at a high cost, whereas sending only a few ships will be cheap but risks leaving some failures unaddressed overnight. As a result, it is important to find a balance between the two approaches.
This problem is further complicated by the fact that there is a large degree of uncertainty in the accessibility of the wind farm. If the conditions are too choppy or too windy, then vessels will be unable to travel to the wind farm. To account for this, we will need to build a statistical model of how the metocean conditions change over time near the wind farm.
The aim of the PhD will be to develop an optimisation model that can account for the key factors and constraints that affect the problem to help determine which vessels should be utilised at which times.
Symbiotic Simulation in an Airline Operations Environment
Student Luke Rhodes-Leader
Supervisors Stephan Ongo, Dave Worthington and Barry Nelson
Industrial partner Rolls Royce
Disruption within the airlines' industry is a severe problem. It is quite rare that an airline will operate a whole day without some form of delay to their schedule. The causes of these vary widely, from weather to mechanical failures. This often means that the schedule has to be revised quickly to minimise the impact on passengers and the airline. This could potentially be done with a form of a simulation called Symbiotic Simulation.
A simulation is a computer model of a system that can estimate the performance of the system. A symbiotic simulation involves an interaction between the system being modelled and the simulation by exchanging information. This allows the simulation to use up to date information to improve its representation of the system. In turn, the predictions of the simulation can then be used to improve the way that the system operates. In our application, the simulation will estimate how well a schedule performs, and the airline can then implement the best one.
However, there are issues with the current state of Symbiotic Simulation. These include choosing how to use the up to date information in the best way and in finding a “good” schedule quickly. Such areas will be part of the research during my PhD.
Realistic Models for Spatial Extremes
Student Robert Shooter
Supervisors Jonathan Tawn, Jenny Wadsworth and Phil Jonathan
Industrial partner Shell
Being able to model wave heights accurately is very important to Shell - for both economic and safety reasons. By knowing the characteristics of waves allows the safe design of offshore structures (such as oil rigs) while also meaning that the appropriate amount of money is spent on each structure; a small increase in necessary strength of the structure costs a significant amount. As it is large waves in particular that have to be factored into the assessment of meeting safety criteria, Extreme Value Analysis (EVA) is used, since this allows appropriate modelling for extreme waves. For this project, attention will largely be paid to modelling of waves in the North Sea off the coast of Scotland.
The particular focus of this project will be to consider the effect of altering location on the properties of the extreme waves, as well as direction, and to model these appropriately. For instance, it could be expected that as Atlantic storms (as seen in the UK autumn and winter) create large waves, while very little extreme weather approaches from the East so that there are fewer extreme waves from this direction.
Another issue to be considered is that distant sites are very unlikely to exhibit extreme values at the same time, whilst nearby locations are likely to be very similar in nature. Practically, a mix of these two possibilities is the probable underlying situation. The exact nature of this kind of behaviour needs to be determined both for modelling and important theoretical reasons.
Change and Anomaly Detection for Data Streams
Student Sam Tickle
Supervisors Paul Fearnhead and Idris Eckley
Industrial partner BT
It's a changing world.
Pick a system. Any will do. You could choose something simple, like the population dynamics in Lancaster's duck pond, or something fantastically complex, such as the movements of the stock markets around the world or the flow of information between every human being on the planet. Ultimately, every action in every system is governed by change and reaction to it. Every problem is a changepoint problem.
And yet, despite the increased interest in studying the detection of change in recent years, understanding in many aspects of the problem still lag behind where we would like them to be. The first major issue arises from the necessary consideration of multiple variables simultaneously. In most real-life situations, it will be necessary to examine the evolution of more than one quantity (in our duck pond example, the population of ducks and of the students who feed them would both be pertinent considerations). Yet, unpacking what a change means in this context, and how to detect it, is still very much in its infancy.
The second important strand of the project will involve speeding up existing changepoint detection methods. In order for such a detection method to be of any real-world use, changes need to be detected as soon as possible, especially in situations where the nature of the change is subtle. Failure to do so can lead to the retention of policies which can be actively detrimental to the system in the medium to long term.
At the same time, however, distinguishing between true changes and mere anomalies in the data is important. Correctly identifying an anomalous occasion, and setting it apart from a true, persistent change, is vital for any decision-maker. Doing this effectively and quickly, in the context with multiple data sets (known as a data stream) being observed simultaneously is the central goal of this research.
Optimising Aircraft Engine Maintenance Scheduling Decisions
Student David Torres Sanchez
Supervisors Konstantinos Zografos and Guglielmo Lulli
Industrial partner Rolls Royce
In our modern era, thousands of flights are in operation every minute. Each of these requires a meticulous inspection of their mechanical components to ensure that they can operate safely. As there are several types of maintenance interventions, varying in rigorousity and duration, they have to be scheduled to occur at certain times. One of the world's major jet engine manufacturer, Rolls-Royce (RR), is interested in knowing not only when, but also where and what type of intervention is optimal to perform.
My PhD focusses on exploring the most appropriate ways of modelling the problem and ultimately solving it. This involves developing a mathematical formulation which then has to be solved via an efficient algorithm. The efficiency is linked with the ability of the algorithm to cope with the scale of the combinatorial problem, which due to the level on which RR operates, is very large.
Efficient Bayesian Inference for High-Dimensional Networks
Student Kathryn Turnbull
Supervisors Christopher Nemeth, Matthew Nunes and Tyler McCormick
Network data arise in a diverse range of disciplines. Examples include social networks describing friendships between individuals, protein-protein interaction networks describing physical connections between proteins, and trading networks describing financial trading relationships. Currently, there is an abundance of network data where, typically, the networks are very large and exhibit complex dependence structures.
When studying a network, there are many things we may be interested in learning. For instance, we may want to understand the underlying structures in a network, study the changes in a network over time, or predict future observations.
There already exists a collection of well-established models for networks. However, these models generally do not scale well for large (high dimensional) networks. The dimension of the data and dependence structures present interesting statistical challenges. This motivates my PhD, where the focus will be to develop new and efficient ways of modelling high dimensional network data.
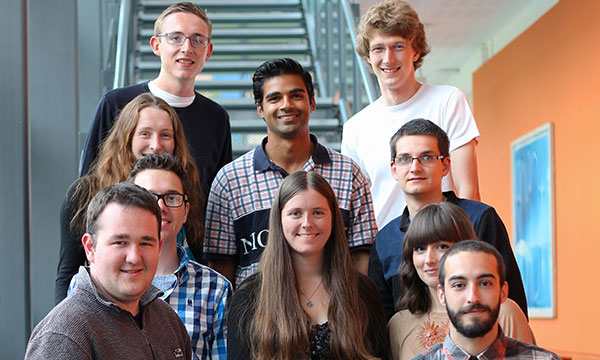
2015 PhD Cohort
Here you can find details of the PhD research projects undertaken by students who joined STOR-i in 2014, and started their PhD research in 2015. You can also access further technical descriptions by clicking on the links to the student's personal webpage below each project description.
Computational Statistics for Big Data
Student Jack Baker
Supervisors Paul Fearnhead and Chris Nemeth
Medical scientists have decoded DNA sequences of thousands of organisms, companies are storing data on millions of customers, and governments are collecting traffic data from around the country. These are examples of how information has exploded in recent years. But broadly, these data are being collected so they can be analysed using statistics.
At the moment, statistics are struggling to keep up with the explosion in the quantity of data available. In short - it needs a speedup. This is the area I'll be working on over the next few years.
Schemes to speed up statistical methods have been proposed, but it's not obvious how well they all work. So to start with, I'll be comparing them in different cases. This comparison should outline any issues, which I can then try and resolve.
Optimal Partition-Based Search
Student James Grant
Supervisors Kevin Glazebrook and David Leslie
Industrial partner Naval Postgraduate School
As within industry, optimal allocation of resources is an important planning consideration in military activities. Some instances of military search and patrol (such as tracking border crossings, detecting hostile actions in some planar region, searching for missing objects or parts etc.) can be performed remotely using Unmanned Aerial Vehicles (UAVs). A UAV is a class of drone which is capable of detecting events from the sky and relaying the event locations to searchers on the ground.
Searching in this context will typically be performed by a fleet of several UAVs, with each UAV being allocated a distinct portion of the search region. UAVs awarded a broader search region will have to spread their time more thinly, and as such, this may reduce their probability of detecting an event. Furthermore, some UAVs may be better equipped than others to search certain parts of the region – this may be due to varying terrains or altitudes.
The question this project seeks to answer is how should the resources (UAVs) be allocated to maximise the number of events detected. To answer this question, information on the capabilities of the UAVs and estimated information on where events are most likely to occur must be considered. Then an 'Optimisation method' which identifies the best of many options should be used to select the optimal partitioning of the search region. Existing methods do little to account for the fact that information on where events will occur is merely estimated and a novel aspect of this project will be to take account of this uncertainty.
Forensic Sports Analytics
Student Oliver Hatfield
Supervisors Chris Kirkbride, Jonathan Tawn and Nicos Pavlidis
Industrial partner ATASS Sports
When data are collected about a process occurring over time, it is often of interest to be able to tell when its behaviour departs from the norm. Because of this, an important research area is the detection of anomalies in random processes. These anomalies can take many forms - some may be sudden, whereas others may see gradual drift away from expected behaviour. This project aims to develop new ways of identifying both sorts of abnormal patterns in random processes of a variety of structures and forms, both when observations are independent, and when the processes evolve. Anomaly detection has a vast range of applications, such as observing fluctuations in the quality of manufactured goods to detect machine faults.
The application that forms the focus of this project is match-fixing in sport. Corrupt gamblers with certainty about the outcomes of matches can bet risk-free, and hence have the potential to make substantial illegal gains. The cost of fixing matches, for example via bribes, can be high, and so the corrupt gamblers may need to wager significant amounts of money to make a profit. However, betting large amounts can distort the markets, whether gambled in lump sums or disguised more subtly, as bookmakers alter their odds to mitigate potentially significant losses. This project attempts to detect suspicious betting activity by looking for unusual behaviour in the odds movements over time. These are considered both before matches and during them when the in-play markets also react to match events themselves. The aim is to be able to identify fixed matches as early as possible so that gambling markets can be suspended with the lowest potential losses.
Novel Inference methods for dynamic performance assessment
Student Aaron Lowther
Supervisors Matthew Nunes and Paul Fearnhead
Industrial partner BT
Organisations are complex, continually changing systems that can be responsible for carrying out many essential tasks. The importance of these tasks encourages us to have a sound understanding of how the system behaves, but the complex structure makes this difficult.
My PhD focuses on modelling and understanding how aggregations of variables (or tasks, for example) evolve, where we think of these variables as components of a system. The aggregation of such variables is vital since the effect on the system from an individual may be negligible, but also vast numbers of variables means that modelling each one is impractical.
Ultimately we are interested in how the change in behaviour of these variables impacts the performance of the system. Still, in order to predict the future state of the system, we must have a thorough understanding of the variables. We may achieve this by generating accurate models and deriving methodology that can determine the structure of the aggregations, which currently is quite limited.
Uncertainty Quantification and Simulation Arrival Process
Student Lucy Morgan
Supervisors Barry Nelson, David Worthington and Andrew Titman
Simulation is a widely used tool in many industries where trial and error testing is either too expensive, time-consuming or both. It is therefore imperative to build simulation models that mimic real-world processes to high fidelity. This means utilising complex, potentially non-stationary, input distributions. Input uncertainty describes the uncertainty that propagates from the input distributions to the simulation output and is, therefore, key to understanding how well a model captures a process.
Currently, there are methods to quantify input uncertainty when input distributions are homogenous, but non-stationary input models have yet to be considered. My project will aim to create methods that can quantify the input uncertainty in a simulation model with non-stationary inputs — starting by looking at queueing models with non-stationary arrival processes.
Large Scale Statistics with Applications to the Bandit Problem and Statistical Learning
Student Stephan Page
Supervisors Steffen Grünewälder, Nicos Pavlidis and David Leslie
The bandit problem is a name given to a large class of sequential decision problems and derives from a term for slot machines. In these problems, we are faced with a series of similar situations, and for each one, we receive a reward by selecting an action based on what has happened so far. It is necessary to choose actions in such a way that we learn a lot about the different rewards while still obtaining a good reward from the situation we currently face. Often these objectives are referred to as exploration and exploitation. Usually, we are interested in making the sum of our rewards as big as possible after having faced many situations.
The multi-armed bandit problem in which the rewards we receive are only influenced by the actions (or arms) we select has been well-studied. However, if we adjust this to the contextual bandit problem in which for each situation the rewards are also influenced by extra information (or context) that we find out before having to select an action, then we get something which is much less understood. When we are given a large amount of extra information, it is necessary to work out what parts of this information are relevant. This requires the use of large scale statistical methods.
Statistical Learning for Interactive Education Software
Student Ciara Pike-Burke
Supervisors Jonathan Tawn, Steffen Grünewälder and David Leslie
Industrial partner Sparx
In recent years, the education sector has moved away from the traditional pen and paper approach to learning and started to incorporate new technologies into the classroom. Sparx is an education research company that uses technology, data and daily involvement in the classroom to investigate how students learn scientifically. As students interact with the system, data on the way they are learning can be securely gathered. This project aims to be able to use a discreet and anonymised data set to improve students' experience and attainment.
Multi-armed bandits are a popular way of modelling the trade-off between exploration and exploitation which arises naturally in many situations. As part of the PhD, they will be applied alongside other statistical learning techniques to help develop systems that interact with the students to provide a personalised route through the content and exercises. Another aim of this research will be to develop more accurate predictions of student performance. An accurate prediction of student performance in exams is vital for students, teachers and parents.
Multivariate extreme value modelling for vines and graphic models
Student Emma Simpson
Supervisors Jenny Wadsworth and Jonathan Tawn
There are many real-life situations where we might want to know the chance that a rare event will occur. For instance, if we were interested in the building of flood defences, we would want to take into account the amount of rainfall that the construction should be able to withstand, and knowing how often particularly adverse rainfall events are likely to occur would be an essential design consideration. Often with rare situations, it may be the case that we are interested if has never happened before, making modelling a considerable challenge. The area of statistics known as extreme value theory is dedicated to studying rare events such as these and allows the development of techniques that are robust to the fact that there is an intrinsically limited amount of data available concerning these infrequent events.
The main aim of this PhD project is to develop techniques related to extreme value theory where there are multiple variables to consider, and of particular interest in developing models that can encapsulate the various ways that these different variables may affect one another. This aim will be achieved by drawing on methods from other areas of statistics that are also concerned with capturing dependence between different variables, and more information about this is available on my webpage.
Although this project is not associated with a specific application, it is hoped that the methods developed could be useful in a variety of areas, with the most common uses of extreme value theory come from environmental and financial sectors.
Supporting the design of radiotherapy treatment plans
Student Emma Stubington
Supervisors Matthias Ehrgott
Radiotherapy is a common treatment for many types of cancers. It uses ionising radiation to control or kill cancerous cells. Although there has been rapid development in radiotherapy equipment in the past decades, it has come at the cost of increased complexity in radiotherapy treatment plan design.
Treatment planning involves multiple interlinked optimisation problems to determine the optimal beam direction, radiation intensities machine parameters etc. The process is complicated further by conflicting objectives; an ideal plan would maximise the radiation to the tumour while minimising the radiation to surrounding healthy cells. This is not possible as minimising would result in no radiation therapy and maximising would result in all the healthy cells being killed. Therefore, a compromise must be struck between these two objectives. Currently, this is done by comparing a plan to a set of clinical criteria. If a plan does not meet all the requirements, the plan must be re-optimised by trial and error until an acceptable proposal is found.
The project will aim to remove the trial and error process from treatment planning. Data Envelopment Analysis (DEA) will be used to assess the quality of individual treatment plans against a database of existing achievable plans to highlight strategies that could be improved further. The project will focus mainly on prostate cancer cases due to the frequency and relative conformity in shape and location of the tumour. The hope is that methods can then be extended to all cancer types. There is also scope to develop an automatic treatment planning technique to remove clinician subjectivity and speed up the planning process. These aim to ensure individual patients receive the best possible treatment for their unique tumour.
Customer Analytics for Supply-Chain Forecasting
Student Daniel Waller
Supervisors John Boylan and Nikolaos Kourentzes
Industrial partner Aimia
Forecasting demand in retail has long been a fundamental issue for retailers. Long-term strategic planning is all about prediction, and demand forecasts inform such processes at the top level. At a lower level, marketing departments find the capacity to predict demand under various arrays of promotions valuable. At the micro-level, supply chain and inventory management processes are reliant on fast, accurate, tactical forecasts for each stock-keeping unit (SKU), to keep stock levels at a suitable level.
Demand forecasting techniques traditionally employed in industry have focussed on extrapolation of past sales data to predict future demand. However, as demand forecasting becomes more complex, with ever-increasing ranges of products, there is an increasing need for forecasting tools which use more information. Causal factors, such as promotional activity, have a driving effect on demand patterns and accurate modelling of these can prove crucial to forecasting accuracy.
A further challenge is the considerable amount of data now collected at point-of-sale in retail, right down at the micro-level of individual SKUs and transactions. The massive datasets that are compiled as a result pose challenges for forecasting, but also may hold the key to the significant gains that can be obtained in the development of prescriptive models for demand.
My PhD aims to bring together these different strands of thought to develop a demand forecasting framework that harnesses the potential in big datasets and incorporates causal factors in demand, such as promotions, to produce accurate forecasts which can provide value at all levels of a retail business.
Novel methods for distributed acoustic sensing data
Student Rebecca Wilson
Supervisors Idris Eckley
Industrial partner Shell
Distributed Acoustic Sensing (DAS) techniques involve the use of fibre-optic cable as the measurement instrument. The whole cable is treated as the sensor rather than individual points which allows for a higher degree of control over the measurements that are collected.
In recent years, the use of DAS has become more widespread with this approach being implemented across a range of applications including security, e.g. border monitoring and the oil and gas industry. While DAS has proven to be incredibly useful since it allows for a real-time recording that is relatively cheap compared to other methods, there are drawbacks related to its use. As with most data collection methods, the measurements that are obtained from such techniques can be corrupted easily.
This PhD aims to develop methods that allow us to detect corruption in DAS signals so that this can be removed, leaving as much of the original signal intact as possible.
Modelling and solving dynamic and stochastic vehicle routing and scheduling problems using efficiently forecasted link attributes
Student Christina Wright
Supervisors Konstantinos Zografos, Nikos Kourentzes and Matt Nunes
There are many risks associated with the transport of hazardous materials. An accident can escalate into something much worse, such as a fire or explosion due to the hazardous material being carried. Of most pressing concern is the danger to those nearby should an accident occur. Fewer people are likely to be injured or even killed on a country lane than if the accident happens in a busy city centre.
Vehicles carrying hazardous materials should travel upon routes where they are least likely to crash and that pose the least danger should an accident occur. The selection of the best way uses an optimisation model. Some of the things that contribute towards the risk such as vehicle speed are unknown beforehand. These values can be predicted using forecasting methods. My PhD will focus upon using forecasting with an optimisation model to try and find the best routes for hazardous material vehicles to take.
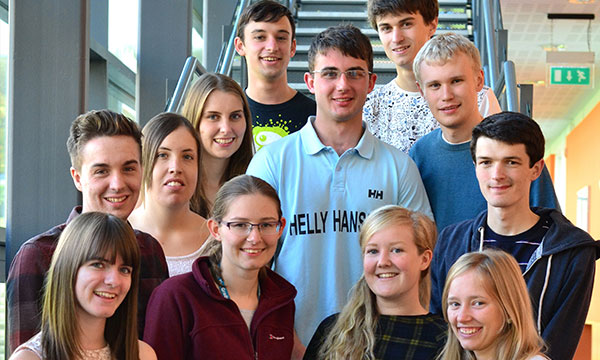
2014 PhD Cohort
Here you can find details of the PhD research projects undertaken by students who joined STOR-i in 2013 and started their PhD research in 2014. You can also access further technical descriptions by clicking on the links to the student's personal webpage below each project description.
Optimising Pharmacokinetic Studies Utilising Microsampling
Student Helen Barnett
Supervisor Thomas Jaki
Industrial partner Janssen Pharmaceutica
In the drug development process, the use of laboratory animals has long been a necessity to ensure the protection of both human subjects in clinical studies and future human patients. The parts of the process that involves the use of animals are called pre-clinical studies. The motivation for the development of laboratory techniques in pre-clinical studies is to reduce, refine and replace the use of animals. Pre-clinical pharmacokinetic studies involve using measurements of drug concentration in the blood taken from animals such as rats and dogs to learn about the movement of the drug in the body. The technique of microsampling takes samples of considerably less blood than previous sampling techniques in the hope of reducing and refining the use of animals.
In my PhD, I aim to make a formal comparison between the results of traditional sampling techniques and micro sampling in preclinical pharmacokinetic studies in order to show the results from microsampling are of the same quality as traditional methods. I also aim to develop optimal trial designs for trials utilising microsampling, which includes designing when and how many blood samples to take from the animals in order to achieve the best quality of results. I aim to do this for single-dose studies, where one dose of the drug is given at the beginning of the trial and repeated dose studies when doses are given at regular intervals throughout the trial.
Predicting the Times of Future Changepoints
Student Jamie-Leigh Chapman
Supervisors Idris Eckley and Rebecca Killick
Changepoint detection and forecasting are, separately, two well-established research areas. However, literature focusing on the prediction, or forecasting, of changepoints is quite limited.
From an applied perspective, there is a need to predict the existence of changepoints. Some examples include:
- Finance – changepoints in financial data could be a result of major changes in market sentiments, bubble bursts, recessions and a range of other factors. Being able to predict these would be very beneficial to the economy.
- Technology – predicting changepoints in the data produced from hybrid cars, for example, would allow proactive control of the vehicle. This could also apply to drones.
- Environment – being able to predict changes in wind speed would allow us to predict when turbines need to be turned off. This would improve efficiency and maintenance.
- This PhD aims to develop models which can predict the times of future changepoints.
Inference Methods for Evolving Networks: Detecting Changes in Network Structure
Student Matthew Ludkin Supervisors Idris Eckley and Peter Neal Industrial partner DSTL
The world around us is made up of networks, from the roads we drive on to the emails we send and the friendships we make. These networks can change in structure over time and, in some cases, the changes can be sudden. In a network of computer connections, a sudden change could mean an attack by hackers or email spam. Predicting such a change could reduce the effect of such an attack.
The project will look at modelling the structure of a network as groups of nodes with similar patterns of network links. This modelling technique can then be adapted to account for the network changing through time and, finally, developing methods to detect sudden changes.
Much work has been done in the areas of 'network modelling’ and 'detecting changes through time’, but the two areas have only overlapped in recent years thanks to the availability of data on networks through time.
Inference using the Linear Noise Approximation
Student Sean Moorhead
Supervisor Chris Sherlock
The Linear Noise Approximation provides a tractable approximate transition density to Stochastic Differential equations (SDEs). This transition density, given the initial point, is, in fact, Gaussian distribution and allows one to simulate the evolution of an SDE quickly. This is particularly useful in statistical inference schemes where the transition density is needed to simulate sample paths of the SDE.
My research involves developing more efficient algorithms that use the LNA as an approximate transition density within a statistical inference for SDEs framework.
An application of my research will involve applying these efficient algorithms to SDE approximations to Markov Jump Processes (MJPs). In particular, my research will focus on data collected on the number of different types of fish from waters off the North coast of Scandinavia in the Barents sea. This data is provided by Statistics for Innovation (a Norwegian Centre for Research-based Innovation that is partnered with STOR-i) and poses a computational complexity challenge due to the multi-compartmental nature of the data. This highlights the need for more efficient algorithms.
Physically-Based Statistical Models of Extremes arising from Extratropical Cyclones
Student Paul Sharkey
Supervisors Jonathan Tawn and Jenny Wadsworth
Industrial partners Met Office and EDF Energy
In the UK, major weather-related events such as floods and windstorms are often associated with complex storm activity in the North Atlantic Ocean. Such events have caused mass infrastructural damage, transport chaos and, in some instances, even human fatalities. The ongoing threat of these North Atlantic storms is of great concern to the Met Office and its clients. Accurate modelling and forecasting of extreme weather events related to these cyclones are essential to minimise the potential damage caused, to aid the design of appropriate defence mechanisms to protect the threat to human life and to limit the economic difficulties such an event may cause.
Floods and windstorms are both examples of extreme events. In this context, an extreme event is one that is very rare, with the consequence that datasets of extreme observations are usually quite small. The statistical field of extreme value theory is focused on modelling such rare events, with the goal of predicting the size and rate of occurrence of events with levels that have not yet been observed. This allows a rigorous statistical modelling procedure to be followed in spite of the data constraints.
This PhD research will focus on building an extreme value model that is a statistically consistent representation of the physics that generate the extremes of interest. This will involve exploring the effect of covariates related to the atmospheric dynamics of these storms as well as the joint relationship of rain and wind over space and time.
Bayesian Bandit Models for the Optimal Design of Clinical Trials
Student Faye Williamson
Supervisors Peter Jacko and Thomas Jaki
Faye Williamson
Lead supervisors: Peter Jacko and Thomas Jaki
Before any new medical treatment is made available to the public, clinical trials must be undertaken to ensure that the treatment is safe and efficacious. The current gold standard design is the randomised controlled trial (RCT), in which patients are randomised to either the experimental or control treatment in a pre-fixed proportion. Although this design can detect a clinically meaningful treatment difference with a high probability, which is of benefit to future patients outside of the trial, it lacks the flexibility to incorporate other desirable criteria, such as the participant’s wellbeing.
Bandit models present a very appealing alternative to RCTs because they perform well according to multiple criteria. These models provide an idealised mathematical decision-making framework for deciding how to optimally allocate a resource (i.e. patients) to a number of competing independent experimental arms (i.e. treatments). It is clear that a clinical trial which aims to identify the superior treatment (i.e. explore) whilst treating the participants as effectively as possible (i.e. exploit) is a very natural application area for bandit models seeking to balance the exploration versus exploitation trade-off.
Although the use of bandit models to optimally design a clinical trial has long been the primary motivation for their study, they have never actually been implemented in clinical practice. Further research is, therefore required in order to bridge the gap between bandit models and clinical trial design. It is hoped that the research undertaken during this PhD will help achieve this goal, so that one day, bandit models can finally be employed in real clinical trials.
Classification in Dynamic Streaming Environments
Student Andrew Wright
Supervisors Nicos Pavlidis and Paul Fearnhead
Industrial partner DSTL
A data stream is a potentially endless sequence of observations obtained at a high frequency relative to the available processing and storage capabilities. Data streams arise in a number of “Big Data” environments including sensor networks, video surveillance, social media and telecommunications. My PhD will focus on the problem of classification in a data stream setting. This problem differs from the traditional classification problem in two ways. First, the velocity of a stream means that storing anything more than a small fraction of the data is infeasible. As such, a data stream classifier must use minimal memory and must be capable of being sequentially updated without access to past data. Second, the underlying data distribution of stream can change with time; a phenomenon known as concept drift. Datastream classifiers must, therefore, have the ability to adapt to changes in the underlying data-generating mechanism. The aim of my PhD is to develop robust classification methods which address both of these problems.
Evolutionary Clustering Algorithms For Large, High-dimensional Data Sets
Student Katie Yates
Supervisors Nicos Pavlidis and Chris Sherlock
In recent years, increased computing power has made the generation and subsequent storage of large datasets commonplace. In particular, it is possible that information is available for a large number of features relating to a particular system or item of interest. These such datasets are thus high dimensional and pose a number of additional challenges in data analysis. My PhD project is concerned in particular with how one may locate “meaningful groups” within these high dimensional datasets, this problem is commonly known as clustering. It is assumed that objects belonging to the same group are in some way more similar to each other than objects assigned to other groups. If such groups can be located effectively, it may then be possible to model each cluster independently given that all members exhibit similar behaviours. This may allow the detection of outlying data points as well as the definition of possible patterns present within the dataset. There exist a number of methods capable of performing this task for low dimensional datasets, but the additional challenges faced in the high dimensional setting indicate the requirement for specialist techniques. The initial focus of this project will be to consider methods which first aim to reduce the dimensionality of the problem in some way, without loss of information required for analysis, thus reducing the problem to one which may be solved more efficiently.
A further consideration is that a system may be monitored over time, and hence new datasets will be generated as time progresses. In this instance, it is desirable to maintain some level of consistency between the successive groupings of datasets such that the results remain meaningful for the user. This is made possible by considering not only how the current data is grouped but also considering how previous datasets, observed earlier were grouped. In general, it is considered inappropriate for radical changes in the clustering structure to be possible. In our opinion, there is a lack of methodology allowing the analysis of high dimensional data which evolve over time. Hence, it is our intention to extend any methodology developed for clustering high dimensional data to further allow the incorporation of historical information, giving rise to more meaningful groupings of evolutionary data.
Non-stationary environmental extremes
Student Elena Zanini
Supervisors Emma Eastoe and Jonathan Tawn
Industrial partner Shell
As one of the six oil and gas "supermajors", Shell has a vested interest in the design, construction and maintenance of marine vessels and offshore structures, a common example of which are oil platforms. The design of robust and reliable offshore sites is, in fact, a key concern in oil extraction. Design codes set specific levels of reliability, expressed in terms of annual probability of failure, which need to be met and exceeded by companies. A correct estimate of such levels is essential to prevent structural damage which could lead not only to losses in revenue but also to environmental pollution and staff endangerment. Hence, it is essential to understand the extreme conditions marine structures are likely to experience in their lifetime.
Environmental phenomena that have very low probabilities of occurrence are here of interest and are characterised by scarce data, with the events that need to be estimated often being more extreme than what has already been observed. Extreme Value Theory (EVT) provides the right framework to model and study such phenomena. This project will focus on the extreme wave heights which affect offshore sites, and their relationship with known and unknown factors, such as wind speed and storm direction. These need to be selected and properly included in the model, and this project will focus on developing such a theory. Further in the future, existing methods will also be considered, and attention will be devoted to optimising the model fit they provide.

2013 PhD Cohort
Here you can find details of the PhD research projects undertaken by students who joined STOR-i in 2012 and started their PhD research in 2013. You can also access further technical descriptions by clicking on the links to the student's personal webpage below each project description.
Efficient search methods for high dimensional data
Student Lawrence Bardwell
Supervisors Idris Eckley and Paul Fearnhead
Industrial partner BT
My PhD is concerned with finding efficient statistical methods for detecting changepoints in high dimensional time series. Much work has already been done in the case of a single dimension, however, when we increase the number of dimensions in a time series there are many more subtleties introduced which complicate the matter and make existing techniques either too limited or too inefficient to be of practical use.
These problems are beneficial to study as combining many of these one-dimensional time series together provides more information and leads to better inferences. A potential application of this work could be to assess when and what parts in a network become defective and then to be able to react quickly to this situation so that delays emanating from this breakage would be minimised. We have begun looking at these sort problems in a simplified context where the individual time series are mostly at some baseline level and then abnormal regions occur where the mean value is either raised or lowered. This is an interesting problem in its own right and has applications in genomics but for the most part, it allows us to simplify the main problem somewhat and to focus on certain aspects of it.
Location, relocation and dispatching for the North West Ambulance Service
Student Andrew Bottomley
Supervisors Richard Eglese and David Worthington
Industrial partner Northwest Ambulance Service
Ambulance services are responsible for responding to the demand for urgent medical care. The level of such demand is unpredictable and resources to meet this demand are limited, so decisions must be made for how to position these resources in order to best meet the response targets in place.
Such decisions involve the positioning of stations, the dispatching of ambulances, and the movement of available ambulances to continue to provide satisfactory coverage across the region. Analogies from results about classical queuing situations can be implemented to help model the possible unavailability of resources more realistically. Different computing strategies can then incorporate such a model and solve this simplified problem to suggest the most preferable placement and movement of the vehicles.
Approaches for the static positioning of ambulances have already been quite extensively studied but building models that allow for dynamic movement of ambulances throughout the day is a newly emerging field that I will be researching.
Detection of Abrupt Changes in High-Frequency Data
Student Kaylea Haynes
Supervisors Idris Eckley and Paul Fearnhead
Industrial partner Defence Science and Technology Laboratory
High-frequency data (or "Big Data") has recently become a phenomenon across many different sectors due to the vast amount of data readily available via sources such as mobile technology, social media, sensors and the internet. An example of data collected and stored at a high frequency is data from an accelerometer which monitors the activity of the object it is attached to. This project will look at big data sets which have abrupt changes in the structure; these changes are known as changepoints. For example, this could be data from an accelerometer attached to a person who is alternating from walking to running.
Changepoints are widely studied in many disciplines with the ability to detect changepoints quickly and accurately having a significant impact. For example, the ability to detect changes in patients' heartbeats can help doctors' spot signs of disease more quickly and can potentially save lives.
Current changepoint detection methods do not scale well to high-frequency data. This research aims to develop methods which are both accurate and computationally efficient at detecting changepoints in Big Data.
Modelling ocean basins with extremes
Student Monika Kereszturi
Supervisors Jonathan Tawn and Paul Fearnhead
Industrial partner Shell
Offshore structures, such as oil rigs and vessels, must be designed to withstand extreme weather conditions with a low level of risk. Inadequate design can lead to structural damage, lost revenue, danger to operating staff and environmental pollution. In order to reduce the probability of a structure failing due to storm loading, the most extreme events that could occur during its lifetime must be considered. Hence, interest lies in environmental phenomena that have very low probabilities of occurrence. This means that, by definition, data are scarce, and often the events that need to be estimated are more extreme than what has already been observed. Such extreme and rare environmental events can be characterised statistically using Extreme Value Theory (EVT).
EVT is used to estimate the size and rate of occurrence of future extreme events. Offshore structures are affected by multiple environmental variables, such as wave height, wind speed and currents, so the joint effect of these ought to be estimated. Storms may affect multiple structures in different locations simultaneously; hence spatial models are needed to estimate the joint risk of several structures failing at the same time.
This research aims to develop spatial models for extreme ocean environments, estimating the severity and rate of occurrence of extreme events in an efficient manner over large spatial domains.
Spatial methods for weather-related insurance claims (joint with SFI)
Student Christian Rohrbeck
Supervisors Deborah Costain, Emma Eastoe and Jonathan Tawn
Industrial partner Statistics for Innovation
Technical description Christian Rohrbeck Research Interests
Storms, precipitation, droughts and snow lead to a high economic loss each year. Nowadays, insurance companies offer protection against such weather events in the form of policies which insure a property against damages. In order to set appropriate premiums, the insurance companies require adequate models relating the claims to observed and predicted weather events.
The modelling of weather-related insurance claims is unique in several ways. Firstly, the weather variables vary smoothly over space but their effect on insurance claims in some locations depends on other factors such as geography, e.g., a location close to a river gives a higher risk of flooding. Secondly, past weather data does not provide a reliable basis for predicting future insurance claim sizes as the climate is changing. Specifically, the IPCC report reveals a change in the climate leading to higher sea levels, increasing average temperatures in the coming decades. Therefore the fundamental questions any approach to model insurance claims needs to address are: (i) which events lead to a claim? (ii) what is the expected number of insurance claims given a weather forecast? and (iii) what is the impact of climate change? Unfortunately, the existing methods cannot answer these questions adequately.
This PhD project aims to improve existing models for weather-related insurance claims by better accounting for the spatial variation of weather and geographical features. In order to build up an appropriate model, statistical methods from spatial statistics, statistical modelling and extreme value theory will be used in the research.
Multi-faceted scheduling for the National Nuclear Laboratory
Student Ivar Struijker Boudier
Supervisors Kevin Glazebrook and Michael G. Epitropakis
Industrial partner National Nuclear Laboratory
The National Nuclear Laboratory (NNL) operates a facility which undertakes work covering research into nuclear materials and waste processing services. Each job that passes through this facility requires specialist equipment and skilled operatives to carry out the work. This means that a job cannot be processed until such resources have become available. It is therefore of interest to schedule each job to take place at a time when the required equipment and operative(s) are not engaged in the processing of another job.
Radioactive materials have to be handled with great care and it is not always possible to know the duration of each job in advance. If a job takes longer than expected, the equipment may not be available on time for the next job and this introduces delays to the schedule. Additionally, the equipment being used sometimes breaks down, causing further delays. This PhD aims to develop tools to schedule the work at the NNL facility. Such scheduling tools will have to take into account the uncertainty in job processing times, as well as the possibility of equipment unavailability due to breakdowns or planned maintenance.
Inference and Decision in Large Weakly Dependent Graphical Models
Student Lisa Turner
Lead supervisors Paul Fearnhead and Kevin Glazebrook
Industrial partner Naval Postgraduate School
In the world we live in, the threat of a future terrorist attack is very real. In order to try and prevent such attacks, intelligence organisations collect as much relevant information as possible on potentially hostile forces. The timely processing of this intelligence can be critical in identifying and defeating future terrorists. However, improvements to technology have resulted in a huge amount of data being collected, far more than can be processed and analysed. This is particularly applicable to communications intelligence as a result of an increase in the use of social media, emails and text messaging. Hence, the problem becomes one of deciding which intelligence items to process such that the amount of relevant intelligence information analysed is great as possible.
My research looks at how this problem can be dealt with for communications intelligence. The set of communications can be modelled as a network, where nodes represent the people involved in the communications and an edge exists between nodes if they share at least one conversation. Once a conversation has been processed and analysed, the outcome can provide valuable knowledge on the communication network. The research looks at how the outcome can be incorporated in the model such that it learns from the outcome and how this updated model can then be used to decide which item to screen next.
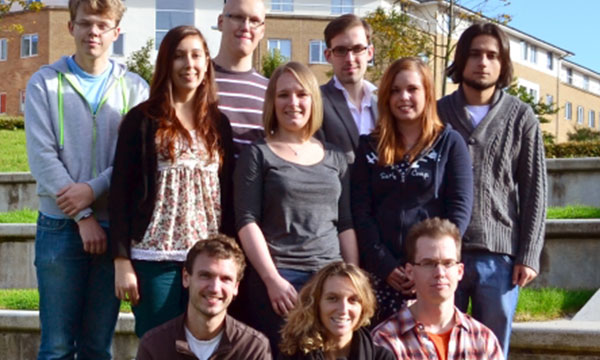
2012 PhD Cohort
Here you can find details of the PhD research projects undertaken by students who joined STOR-i in 2011, and started their PhD research in 2012. You can also access further technical descriptions by clicking on the links to the student's personal webpage below each project description.
Stochastic methods for video analysis
Student Rhian Davies
Supervisor Lyudmila Mihaylova and Nicos Pavlidis
Surveillance cameras have become ubiquitous in many countries, always collecting large volumes of data. Due to an over-abundance of data, it can be challenging to convert this into useful information. There exists considerable interest in being able to process such data efficiently and effectively to monitor and classify the activities which are identified in the video.
We aim to develop a smart video system with the ability to classify behaviour into normal and abnormal activities which could allow the user to be alerted to anomalous behaviour in the monitored area without the need to manually sift through all of the videos. For example, the system could be used to notify a shop owner to a customer placing goods into their bag instead of their shopping trolley.
In order to develop such a system, we intend to start by adapting simple background subtraction techniques to improve their accuracy. These algorithms are used to separate foreground from the background, allowing us to monitor the activities of interest clearly.
Effective learning in sequential decision problems
Student James Edwards
Supervisor Kevin Glazebrook
Many significant issues involve making a sequence of decisions over time, even though our knowledge of the problem is incomplete. Learning more about the problem can improve the quality of future decisions. Good long term decisions, therefore, require choosing actions that yield useful information about the problem as well as being effective in the short term.
The complexity involved in solving these problems often leads to the learning aspects of the problem being modelled only approximately. This simplification can result in poor decision making. This research aims to use modern statistical methods to overcome this difficulty.
Potential applications include: choosing a route to bring emergency relief into a disaster zone with disrupted communications; setting and adjusting the price for a new product; allocating a research budget between competing projects; planning an energy policy for the UK; and responding to an emerging epidemic of uncertain virulence and seriousness.
Betting Markets and Strategies
Student Tom Flowerdew
Supervisor Chris Kirkbride
Industrial partner ATASS
When gambling on the outcome of a sporting event, or investing in the stock markets, no one would turn down the opportunity to hold an ‘edge’ on the market. An edge could be either some form of added analysis, not seen by the market as a whole, or from more nefarious means, such as insider trading.
When an edge has been found, the problem remains concerning how best to invest money in order to take advantage of this favourable opportunity. A strategy proposed by John Kelly in the 1950s involves betting some proportion of your current bankroll, depending on the magnitude of your edge. Therefore, when you have a bigger edge, you would bet a larger proportion of your current wealth.
This scenario is simplistic and only applies for very simple situations. When more interesting betting or investing opportunities arise (for example, betting on accumulators, or investing in options), the Kelly criterion is not suitable to deal with the new scenario. This project investigates methods to expand the Kelly criterion (or other similar strategies) to new areas and is in partnership with ATASS Sports, a statistical analysis company based in Exeter.
Sports data analysis
Student George Foulds
Supervisor Mike Wright and Roger Brooks
Industrial partner ATASS
Sports data analysis often uses basic techniques and draws conclusions from little more than common sense. The importance of applying better statistical techniques to sports data analysis and model building can be seen through the rise of investment strategies based on sports betting. Centaur Galileo, the first sports betting hedge fund, collapsed in early 2012 due to investments guided by inferior models. Therefore, the proposal of more advanced methods to obtain better results is an important one. Two areas of sports data analysis which could be better served by a higher level of analysis are those of home advantage and the effect of technology in sport:
Home advantage is a term used to describe the positive effect experienced by a home team. Although a well-documented phenomenon, most research does little to quantify the underlying factors - an issue that will be addressed. A more subtle analysis will allow a much greater insight into the effect, from which better predictions may be produced.Some level of technology is used in most sports, whether it is a simple pole for vaulting or a relatively advanced piece of engineering such as a carbon fibre bicycle. Identifying the effect of technology on performance, consistency and other factors important to outcome is an essential step in creating models which give better predictions. This will allow us to update our predictions about the outcome in sports faster and more accurately, upon the introduction of new technologies and equipment.
Machine learning in time-varying environments
Student David Hofmeyr
Supervisor Nicos Pavlidis
We all know the feeling that what we’ve learnt is somehow out of date; that our skills have become redundant or obsolete. The fact of the matter is that times change, and we need to be able to adapt our skills so that they remain relevant and useful.
Machine learning refers to the idea of designing computer programs in a way that they become better at performing some predefined tasks, the more experience they have. Much in the same way we, as people, become better at our jobs, at sports, at everything, the more time we spend doing them, computer programs can get better at handling information the more information they have been given. Just like for us, however, these abilities can become redundant when the nature of information changes. It is therefore crucially important to design these programs so that they are adaptive and thus able to accommodate information change without their skill sets become obsolete.
Not all changes, even those who would fundamentally affect the nature of information, however, render old skills irrelevant. In being adaptive, therefore, it is important to be able to be selective when adjusting the way we do things since these adjustments might be time-consuming and unnecessary if the changes do not affect the specific tasks of interest.
This research will approach the problem of information change in two ways. Firstly, by factoring in the nature of change, rather than just detecting it, it should be possible to be more discerning when deciding whether or not to implement an adjustment when changes occur. Secondly, knowledge will be partitioned into multiple simple aspects; therefore, only those aspects which are not relevant in the current environment will be “forgotten”.
Detecting Abrupt Changes in Ordered Data
Student Rob Maidstone
Supervisor Paul Fearnhead and Adam Letchford
When data are collected over time, this is called a time series. Often the structure of the time series can change suddenly; we call such a change a “changepoint”. To model the data effectively, these changepoints need to be detected and subsequently built into the model.
Changepoints occur in many real-world situations and detecting them can have a significant impact. For example, when analysing human genome data, it can be noticed that the average DNA copy value is usually about the same level; however, occasionally sudden changes away from this level occur. These sudden changes in average DNA level often relate to tumorous cells, and therefore the detection of these changes is critical for classifying the tumour type and progression.
Another example of where changepoint detection methods are effective is in finance. Stock data (such as the Dow Jones Index) exhibits a constantly changing time series. Many changes in mean and variance occur and can be detected. This is of use when it comes to modelling the data and forecasting future returns.
This research looks at some of the methods for detecting these changepoints efficiently across a variety of different underlying models. The required methods combine statistical techniques for data analysis with optimisation tools typically used in Operational Research.
Detecting Changes in Multiple Sensor Signals
Student Ben Pickering
Supervisor Idris Eckley
Industrial partner Shell
Companies in the oil industry often place sensors within their equipment in order to monitor various properties such as the temperature of the local geology or the vibration levels of the flowing oil at multiple locations throughout the extraction system. This is done in order to ensure that the system continues to run smoothly. For example, a change in the vibrations of flowing oil could indicate the presence of an impurity deposit in the oil well, which could cause a blockage in the valve of the top of the well. Hence, knowledge of any changes in the properties of the data recorded by the sensors is extremely valuable.
Such changes in the properties of data are known as changepoints. The ability to effectively detect changepoints in a given set of data has significant practical implications. However, the task of developing such changepoint detection methods is complicated by the fact that the data sets are often very large and consist of measurements from multiple variables which are related in some way.
This research aims to utilise cutting-edge techniques to develop changepoint detection methods which are able to efficiently detect changes in data arising from multiple related variables, improving upon some of the weaknesses of current detection methods.
Resource Planning Under Uncertainty
Student Emma Ross
Supervisor Chris Kirkbride
Industrial partner BT
As markets have grown increasingly competitive, the efficient use of available resources has become paramount for the maximisation of profits and increasingly to ensure the survival of companies. For example, to run the UK's telecommunications network, BT deploys thousands of engineers to repair, maintain and upgrade the network infrastructure. This ensures a high level of network reliability which results in customer satisfaction.
To deliver this service, the engineering field force must be carefully allocated to tasks in each time period. Of particular concern are the risks to BT of a sub-optimal allocation. The over-supply of engineers to tasks can result in unnecessary costs to the business; external contracts may need to be brought in at additional expense, and other tasks may suffer without adequate resourcing. Conversely, the under-supply of engineers may lead to missed deadlines and failure to meet customer service targets.
This allocation task is made extremely complex by the unpredictable nature of demand. Plans for the workforce are made extremely far in advance when we can only make vague forecasts of the level of demand we expect to materialise. Supply of engineers is also rendered uncertain by varying efficiency, absence and holidays.
This research explores effective methods for optimal decision making under uncertainty with particular emphasis on modelling the risk (or cost-implications) of an imbalance or gap between supply and demand.
Modelling droughts and heatwaves
Student Hugo Winter
Supervisor Jonathan Tawn
Industrial partner The Met Office
Natural disasters, such as droughts and heatwaves, can cause widespread social and economic damage. For example, a drought in the UK may lead to a decrease in soil moisture and a reduction in reservoir levels. In this situation, water companies will be economically affected as they are required to ensure regions are supplied with water. Sustained dry weather may require government policy such as the hosepipe bans seen in recent years. In Saharan regions of Africa, a period of drought can lead to crop failure and famine. This situation can lead to large death tolls if the required aid is not supplied in time.
Heatwaves and droughts occur when there are days that are very hot or very dry, respectively. These events are referred to as extreme events and by definition, rarely occur. Since extreme events do not occur often, there is little data in the historical record. It might also be possible to observe future events that are more extreme than any that have been previously seen. Such a scenario is possible due to global climate change being driven by greenhouse gas emissions. A mathematical modelling technique often used in this type of situation is the extreme value theory.
This research aims to model different aspects of dependence within extreme events. Broadly, the main goal is to characterise the severity, spatial extent and duration of extreme events. For example, if an extreme event has been observed at a specific location, is it possible to infer other locations where extreme events might occur? Of particular interest will be how the above aspects of extreme events may change under different climate change scenarios.
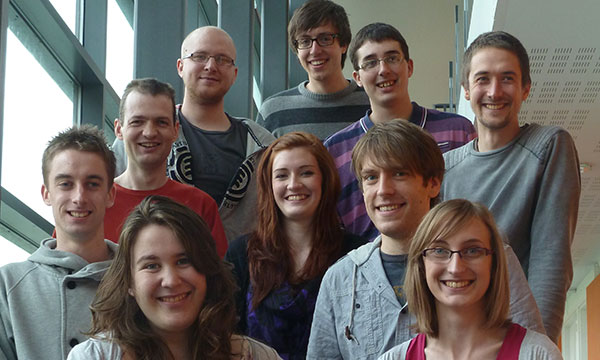
2011 PhD Cohort
Here you can find details of the PhD research projects undertaken by students who joined STOR-i in 2010, and started their PhD research in 2011. You can also access further technical descriptions by clicking on the links to the student's personal webpage below each project description.
Maintaining the telecommunications network
Student Mark Bell Supervisor Dave Worthington Industrial partner BTContinuous access to the UK’s growing telecommunications network is essential for a huge number of organisations, businesses and individuals. If access to the network is interrupted, even for a short period, the effects on public services and businesses can be severe. The network has a complex structure, so faults can occur frequently and for many reasons. When a fault does occur it is vital that repair work is performed as soon as possible.
Openreach, part of the BT Group, is solely responsible for maintenance and repair of the vast majority of the UK’s network. Performing this effectively means ensuring that at any time they have enough staff available to meet the current demands, which can vary considerably. Models that can understand the effects of these changing demands on the available workforce and the existing workload are of considerable benefit in ensuring that the organisation is prepared for the ‘busiest’ periods. Of particular importance is the model’s ability to understand key performance measures, such as the expected time for a repair job to be completed. Keeping these measures within the targets is central to public satisfaction.
The current models are required to understand behaviour across the entire UK and so there are limits regarding the level of detail they can capture; otherwise, the time required to run the models would be impractical. It is therefore vital that the detail included in the model is as accurate as possible. The performance measures output from the models are partly determined by the model inputs which are selected by the analyst; these are based on current knowledge of the system. The research aims to find accurate and robust techniques for the estimation of these input parameters using statistical techniques. This will enable calibration of the models, improving their accuracy when modelling behaviour of the key performance measures, which in the real system are subject to regular fluctuations in the short-term.
Effective Decision Making under Uncertainty
Student Jamie Fairbrother Supervisor Amanda TurnerOften we have to make decisions in the face of uncertainty. A shop manager has to decide what stock to order without knowing the exact demand of each item. An investment banker has to choose a portfolio without knowing how the values of different assets will evolve. Taking this uncertainty into account allows us to make good robust decisions.
Using available information and data, a scenario tree describes many possible different futures and importantly is in a form which can be used to "optimise" our decision.
Generally, the more futures a scenario tree takes into account, the more reliable the decision it will yield. However, if the scenario tree is too large the problem becomes intractable. The aim of this research project is to develop a way of generating scenario trees which are small but give reliable decisions. This research would have applications in finance, energy supply and logistics.
Defensive Surveillance
Student Terry James Supervisors Kevin Glazebrook and Professor Kyle Lin Industrial Partner Naval Postgraduate SchoolDefensive surveillance is of great importance in the modern world, motivated by the threats faced on a daily basis and the technology which now exists to mitigate these threats. Adversaries wishing to complete an illicit activity are often intelligent and strategic, wishing to remain covert as they do so. Surveillance must then take the strategic nature of adversaries into account when designing surveillance policies.
This research project aims to explore the task of identifying defensive surveillance policies which can mitigate the threats faced by adversaries in a public setting. For example, consider a surveillance resource responsible for a number of public areas, each of which is a potential target for an adversary. How should the resource be controlled given that the adversary can strike at any time in amongst any of the randomly evolving public crowds?
Fighting Terrorism in the Information Swamp
Student Jak Marshall Supervisors Kevin Glazebrook and Roberto Szchetman Industrial Partner Naval Postgraduate SchoolIn a world where the threat of terrorist activity is a very real one, intelligence and homeland security organisations across the globe have an interest in gathering as much information about such activities so that preventative measures can be taken before something like a bomb attack is executed. Problems arise as these agencies generate intelligence data in enormous volumes and it is of highly varying quality. Satellites taking countless images, field agents submitting their reports and various other high traffic streams all add up to more intelligence than can be reasonably processed in high-pressure scenarios.
Further problems arise as any piece of information received from this glut of incoming information needs to be processed by technical experts before it can be contextualized by analysts to fight terrorist threats. It is usually the case that the processing staff aren't completely aware of the importance (or unimportance!) of these pieces of intelligence before they commit their attention to working on them. This research concentrates on modelling the role of the processors in this situation and on the development of methods that can efficiently search this information swamp for vital information.
Two approaches to the problem are considered. The first is a time-saving exercise that asks how a processor should decide whether an individual piece of intelligence needs further scrutiny by them and if not whether it should be flagged as important or cast aside. The second approach takes that decision away from the processor and instead the processor only ever considers the latest report that arrives in their inbox and decides its fate only when the next report arrives. The problem then is to determine how stringent the quality control on intelligence should be given that high arrival rates of reports can result in a small amount of time for the processor to consider each report.
Fuel Pricing
Student Shreena Patel Supervisor Chris Sherlock Industrial partner KSSIn the market for home-delivered fuel, price takes on a number of different roles. Given that capacity on delivery, the truck has zero value once the truck has left the depot, pricing should minimise the risk of capacity being left unfilled. However, this must be balanced against the firm’s ultimate aim of maximising profit. Hence prices need to be varied over time and across customers to manage demand and make the best use of a limited capacity of delivery vehicles.
Ongoing work with a fuel consultancy firm is looking to develop a model which combines these roles into a single pricing strategy. In particular, price customisation will be achieved using statistical techniques which group together customers according to their price sensitivity.
Patient flows in A&E Departments
Student Daniel Suen Supervisor Dave WorthingtonSteadily rising patient numbers and a shrinking budget has been a major concern for the NHS for many years. Rising pressure to maintain the quality of care while coping with a limited budget motivates the need to improve the efficiency of hospitals, in particular, the way they utilise their available resources.
Understanding patient flows in healthcare systems is an important tool when trying to improve hospital efficiency and, among other things, reduce patient waiting times. A better insight into how hospitals help decision-makers improve the management of hospital resources (e.g. hospital beds, staff) and avoid patient blockages, where a build-up for one type of resource can have knock-on effects on the rest of the system.
The focus of this research will be on how to best describe these healthcare systems and looking at improving existing modelling techniques such as simulation-based methods.
These are just a few of the very real and practical issues our graduates will be well equipped to tackle, giving them the skills and experience to enable their careers to progress rapidly.
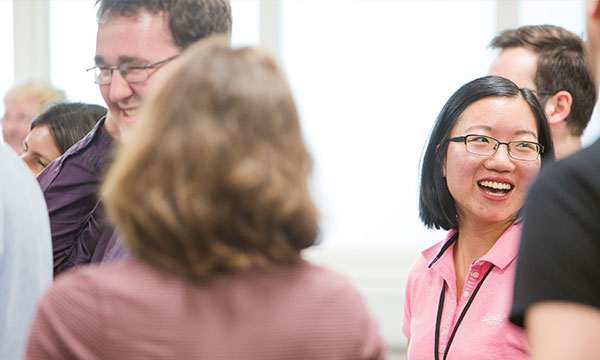
Associated Students
Here you can find details of PhD research projects from STOR-i associated students. You can also access further technical descriptions by clicking on the links to the student's personal webpage below each project description.
Improving consumer demand predictions
Student Devon Barrow Supervisor Sven CroneThe ability to accurately predict the demand for goods and services is important across all sectors of society particularly business, economics and finance. In the business and retail sector, for example, prediction of consumer demand affects both the profitability of suppliers and the quality of service delivered. Unreliable demand forecasts can lead to inefficient order quantities, suboptimal inventory levels, and increased inventory as well as administrative and processing costs which all affect the revenue, profitability and cash flow of a company. Improvements in demand forecasts, therefore, have the potential for major cost savings.
Traditionally a major source of this improvement has come from the selection of the appropriate choice of a forecasting method. Additionally, improvements in accuracy can also be achieved by combining the output of several forecast methods rather than relying on any single best one. This research investigates existing techniques and develops new ones for combining predictions from one or more forecast methods. The potential improvements in accuracy and reliability will help to support management decisions and allow managers to better respond to circumstances, events and conditions affecting seasonal demand, price sensitivities and supply fluctuations for both itself, and competitors.
Analysis and Classification of sounds
Student Karolina Krezmienewska Supervisors Idris Eckley and Paul FearnheadWe are surrounded by a huge number of different sounds in our daily lives. Some of these are generated by natural phenomena, like the sound we hear during seismic activity or when the wind blows. Other sounds are generated by man-made devices. By analysing these sounds we can learn valuable information about their source. This can include either (a) identifying the type of the source or (b) assessing its condition.
Classification of sounds is currently used in a variety of settings e.g. speech recognition, diagnosing cardiovascular diseases through the sound of the heart, and environmental studies. This project involves the development of more accurate methods for analysing and classifying sounds in collaboration with a leading industrial partner.
Extreme risks of financial investments
Student Ye Liu Supervisor Jonathan TawnA few years have passed, but aftershocks of the credit crunch have spread far beyond just the financial sector and influenced everyone's life in many ways - housing, education, jobs etc. Living in a world yet to recover fully from the crisis-led recession, we cannot help but wonder what went wrong and how we can better prepare ourselves for the future.
To resolve the fundamental issue of understanding uncertainty in the financial sector statistical methods have been used for many decades. However standard statistical analysis relies on a good amount of past information, whereas events like the credit crunch have occurred only a few times throughout human history. Traditional risk management tends to use one model for all situations and make simplistic assumptions that rare events like the credit crunch happen in the same way as the normal ups-and-downs in the financial market. This research reveals that such beliefs can lead to a very inaccurate risk assessment, which is the root of many failed investments in the credit crunch.
Analysing the whole financial sector jointly is very difficult and usually assumptions are made that all financial products react similarly to a market crash. This research shows that it is not true and proposes a method which allows flexibility for each financial product to be treated individually. The new method provides a much more accurate risk assessment when multiple financial products are concerned, and is being incorporated by a top UK fund manager to identify the true extent of their risk in future.
Modelling Wind Farm Data and the Short Term Prediction of Wind Speeds
Student Erin Mitchell Supervisors Paul Fearnhead and Idris EckleyWind energy is a fast developing market within the United Kingdom and the entire world. With the ever-looming threat of Earth's fossil fuels drying up, the world is increasingly looking to turn to renewable energy sources; wind energy is a popular and growing market within the renewables sector.
In 2007 only 1.8% of the energy in the United Kingdom came from renewable sources. However, the United Kingdom's Government is aiming to produce 20% of its energy from renewable sources by 2020. With the profile and demand for wind energy constantly increasing there is an expanding market in its analysis and prediction. Due to there being financial penalties for both under and over prediction it is important to make accurate predictions to maximise the profit made from sales to the market. Wind energy producers sell their energy in advance of its production and, as such, it is important to make accurate forecasts of wind speeds and energies up to 36 hours in advance.
Alongside a leading renewables company, this research is looking at developing novel methods for accurate forecasts for wind power output, in particular by implementing dynamic systems with evolving model parameters.
Demand learning and assortment optimization
Student Jochen Schurr Supervisor Kevin GlazebrookIn the retail industry, the most constraining resource is shelf space. Decision-makers in that field should, therefore, pay careful consideration to the question of how to make optimal use of it. In the context of seasonal consumer goods, e.g. fashion, this decision making process becomes dynamic for two reasons: first, as the assortment changes seasonally or even within the season and, second, as the demand for each product is yet to be estimated more precisely with the use of actual sales data.
The purpose of this project is to identify the key quantities and to study their sensitivity in the decision-making process, both in existing and to-be-formulated models.
Modelling and Analysis of Image Texture
Student Sarah Taylor Supervisor Idris EckleyWhen one thinks about texture, a typical example that comes to mind is that of a woven material, straw or a brick wall. More formally, image texture is the visual property of an image region with some degree of regularity or pattern: it describes the variation in the data at smaller scales than the current perspective. In many settings, it is useful to be able to detect differing fabric structure, for example, to identify whether there is an area of uneven wear within a sample of material. To avoid the subjectivity of human inspection of materials it is thus desirable to develop an automatic detection method for uneven wear. Developing such methods is the focus of this PhD project.
Determining the future wave climate of the North Sea
Student Ross Towe Supervisor Jonathan TawnWave heights are of inherent interest to oil firms, given that many of their operations take place offshore. Information about the meteorological processes, which determine the occurrence of extreme waves, influence plans for any future operations. Clarifying the risk of these operations is of importance for oil firms.
This project will analyse the distribution of extreme wave heights and how this distribution will change under future climate change scenarios. Determining the distribution of future wave heights depends on knowledge of other factors such as wind speed and storm direction. Data from global climate models can also be used to provide an insight into the future large scale processes; however, this information has to be downscaled to the local scale to produce site-specific estimates that the oil firms can use. Naturally, past information can be used to predict the distribution at a specific site as well as from other sites across the region.
Facility layout design under uncertainty
Student Yifei Zhao Supervisor Stein W WallaceThe facility layout problem (FLP) considers how to arrange physical locations of facilities (such as machine tools, work centres, manufacturing cells, departments, warehouse, etc.) for a production or delivery system. The layout of facilities is one of the most fundamental and strategic issues in many manufacturing industries. Any modifications or re-arrangements of existing layout involve substantial financial investment and planning efforts. An efficient layout of facilities can reduce operational cost and contribute to the overall production efficiency. One of the most frequently considered criteria for layout design is the minimization of material handling distance/cost. It is claimed that material handling cost contributes from 20 to 50 per cent of the total operating expenses in manufacturing.
Classical FLPs only consider the deterministic cases where flows between each pair of machines are known and certain. However, the real production environment involves uncertain factors such as changes in technology and market requirement. Under the uncertain environment, flows between machines are uncertain and can vary from period to period. We are interested in designing a robust layout which adapts to the flow changes. The criterion of the robust layout is to minimize the expected material handling cost over all possible uncertain production scenarios.
These are just a few of the very real and practical issues our graduates will be well equipped to tackle, giving them the skills and experience to enable their careers to progress rapidly.
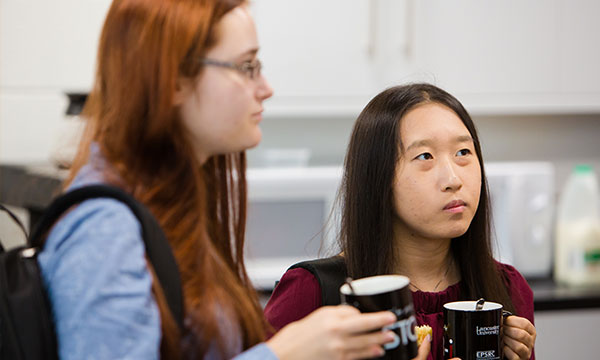
Research Funding Opportunities
As a STOR-i PhD student, there are four key sources of funding:
Your Personal Research Fund
Your own fund to cover attendance at training courses, conferences and books. In addition to this fund, you are supplied with a high specification laptop. You will manage your own research fund spending. It typically covers attendance at 2 international conferences and 2-3 national meetings/conferences across your studies.
The STOR-i Research Fund
You can make a bid for funding for additional research support for more substantial activities, where your Personal Fund cannot cover it. Applications to the Research Fund are competitive and require a full case putting forward. Successful applicants will be responsible for the management of the award and reporting of outcomes. The process of applying for and managing grants gives the opportunity to practice and develop key skills acquired on the STOR-i programme.
STOR-i's Executive Committee is responsible for selecting applications to the research fund. They give full feedback to every applicant.
STOR-i Impact Fellowship
On PhD completion, STOR-i students are able to apply for a 1-year post-doctoral Impact Fellowship. One is typically awarded per cohort. Impact Fellowships are aimed at enhancing STOR-i students' career development and ensuring the rapid impact of their research. Applications are assessed against PhD performance and a written research proposal describing how the fellowship will be used to further develop research ideas and achieve impact.
The current Impact Fellows are:
- James Grant whose project is Optimal Partition-Based Search; and
- Emma Stubington whose project is Supporting the design of radiotherapy treatment plans.
Gwern Owain Bursary Scheme
Why the scheme exists
Gwern Owain joined STOR-i in 2013 having completed a Mathematics degree at Cardiff University. He obtained an MRes in Statistics and Operational Research in 2014, and he started a PhD in statistical modelling for low-count time series, supervised by Nikos Kourentzes and Peter Neal. Gwern died from leukaemia in October 2015.
In recognition of Gwern's happy experiences in STOR-i, his family (Robin, Eirian and Erin) has very generously offered STOR-i substantial funding in Gwern's name. The funding provides bursaries for students to help understand and address humanitarian and environmental problems they wouldn't have considered in their PhD, reflecting Gwern's strong personal interests.
STOR-i students have undertaken activities in memory of Gwern, such as the STOR-i Yorkshire Three Peaks Challenge
What the scheme funds
The purpose of the bursary is to fund Statistics and Operational Research work by STOR-i students that improves humanitarian or environmental causes: doing good for people or the planet in some form.
Examples of what it could fund, include:
- Attendance at humanitarian/environmental meetings, when existing funds wouldn't naturally have covered this.
- A short period to step outside the PhD and use statistics and operational research skills in some form of humanitarian/environmental cause, e.g., to do an analysis for a relevant group or to self-fund an internship.
- Funding for group activities for students on a humanitarian theme, eg school visits interest pupils in environmental/ethical Statistics and Operational Research.
Funding and Reporting Process
There is a deadline of 4pm 1st September annually, with bids to be submitted to the Director. However, if the proposed project or student has particular time-limiting features then those bids can be submitted at any time. In such cases, it is best to discuss this possibility with the Director in advance.
The bid document (1-page max) should explain what is intended to be done, how it will benefit the student awarded, and provide an outline breakdown of how the funding will be used.
Typically successful proposals will be £1K in value, though exceptionally we would be willing to consider proposals of up to £2K (assuming a suitable justification is provided).
Decisions on which bids to fund will be made by a panel consisting of two representatives from STOR-i Management Team, Phil Jonathan (Shell and a close friend of the Owain family), with input from the Owain family as required.
Successful Awards
2016 Awards
Jamie-Leigh Chapman
To work with the Vegan Society, to analyse survey data, helping them develop a profile for the characteristics of vegans and understand regional differences in their numbers. The Vegan Society plans to use this information in a drive to increase veganism in the UK. Award £1K.
Emma Stubington
To work with Coeliac UK, to undertake data analysis that will contribute to evidence supporting their campaign to stop clinical commissioning groups restricting, or even removing, gluten-free prescription services. Award £1K.
Elena Zanini
To work with Mercy Corps (a global humanitarian aid agency) to improve understanding of why people in certain parts of the world use violence. With this understanding, aid programmes can more effectively address these factors and thereby reduce violence. Award £1K.