Can we rely on judgment to select the best forecasting model?
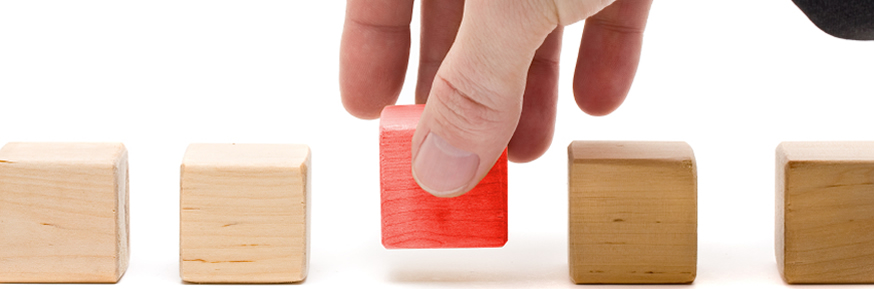
12 February 2014
12 February 2014
How does judgmental model selection perform compared to statistical model selection? Fotios Petropoulos and Nikolaos Kourentzes of the Lancaster Centre for Forecasting explain.
Model selection for forecasting problems has attracted much attention during the last 30 years. Many research studies have examined theoretically and empirically different statistical selection methodologies to identify the ‘optimal’ model. The model selection problem is of major practical importance, because if selections are to be done perfectly, substantial gains will achieved in terms of forecasting performance, usually up to 25-30%, and these gains can be translated into profits.
Conventionally, automatic statistical algorithms perform selection of the most appropriate model from a set of alternatives based on the performance of each model or applying a set of rules. Most forecasting packages employ such automatic algorithms, usually introduced as 'automatic forecasting', 'automatic selection', or 'expert selection'. Not all of these selection techniques are equally good in terms of design or implementation. Nonetheless, it is easy to devise examples where statistical model selection is pretty disastrous. Such examples are common in real forecasting applications.
Key question: How can you improve your statistical model selection routines to increase your forecasting accuracy?
LCF has long experience in forecasting model selection for various applications and industries. Following advances in our understanding of how managerial judgment affects the accuracy of forecasts, we are exploring how to best integrate judgment to support statistical model selection.
In addition to the automatic integrated approaches, several forecasting software systems allow the forecasters/demand planners to manually select a method and specify its parameters. Not to mention that this may be the only alternative for many forecasting modules implemented in major ERP systems. So, forecasting support systems offer what we define as 'judgmental model selection', meaning that the user (practitioner) can, instead of relying on automatic algorithms, judgmentally select the most appropriate model for each time series.
Key question: How does judgmental model selection perform compared to statistical model selection?
Despite judgmental model selection being an option in many of the leading forecasting systems, there is limited, if any, research with regards to the performance of humans in identifying and ‘optimally’ selecting the best model from a set of alternatives. Moreover, the types of information that are relevant and helpful in judgmental model selection have not been identified.
Key question: What types of information can facilitate the judgmental model selection process?
We try to address these questions by utilizing a large scale judgmental forecasting experiment.
If you would like to know more about the research of LCF in the area of judgmental model selection please contact Dr Fotios Petropoulos or Dr Nikolaos Kourentzes. The Centre is always keen to embark on new collaborations with organisations and industrial partners.