Improving and reconciling your forecasts using temporal aggregation
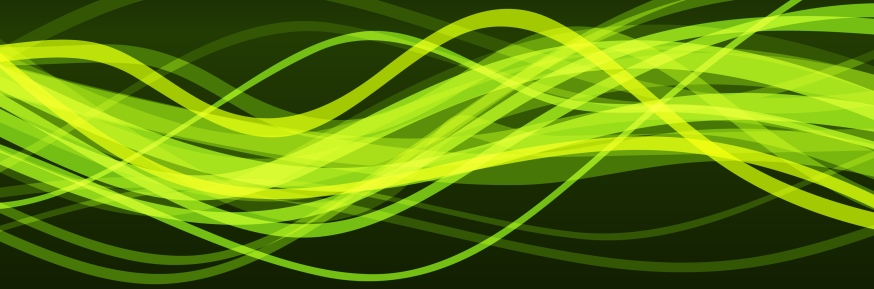
22 June 2014
22 June 2014
In most business forecasting applications, the problem usually directs the granularity of the data that we use for forecasting.
Conventional approaches try to extract information from the historical observations to build a forecasting model. However by transforming the data through temporal aggregation (for example summing monthly data into quarterly and yearly) and using all resulting series simultaneously helps us gather additional information, resulting in more accurate and robust forecasts.
The key idea is that by combining various views/aggregation levels of the series we augment different features of it, helping forecasting models to better capture its structure. The same simple trick can be used to reduce or even remove intermittency in slow moving items making them easier to forecast.
We recently proposed the Multiple Aggregation Prediction Algorithm (MAPA) to take advantage of this idea. The intuition behind the method is simple. Let us assume that we have to forecast demand recorded in monthly buckets. Typically seasonality dominates in such time scales. Most forecasting models, because of the way they are build, will focus on this seasonal element and potentially fit poorly to any trend elements and long term effects, unless the trend is very steep. By aggregating months into quarters, or higher, we reduce the impact of seasonality. This makes it easier for forecasting models to capture the remaining elements of the demand history. More generally, the different elements of a time series are captured better at different levels of aggregation.
This presents us with an opportunity: instead of choosing a single level of temporal aggregation to produce our forecasts, we can use several. This way we achieve two things: first, we capture best the various components of the demand history; and second, we do not rely on a single forecasting model. The second point is somewhat more subtle. The idea is that by not choosing a single model, not only we retain more information, but also we protect our forecasts from potentially choosing the wrong model or parameters.
What are the practical benefits of this approach for your forecasts?
If you would like to give it a try on your own data, LCF has released an implementation of MAPA. Visit this page for examples and to download it. If you want to read more details about this, there will be a non-academic article in the forthcoming issue of Foresight: the international journal of applied forecasting. You can also find the details for fast and slow moving items in two recent LCF publications.
The LCF is always keen to further its collaboration with industry and would be happy to discuss with you how this forecasting approach can help you. If you are interested for more information on this work please contact Dr. Nikolaos Kourentzes or Dr. Fotios Petropoulos.